-
PDF
- Split View
-
Views
-
Cite
Cite
Charlotte Glinge, Najim Lahrouchi, Reza Jabbari, Jacob Tfelt-Hansen, Connie R Bezzina, Genome-wide association studies of cardiac electrical phenotypes, Cardiovascular Research, Volume 116, Issue 9, 15 July 2020, Pages 1620–1634, https://doi.org/10.1093/cvr/cvaa144
- Share Icon Share
Abstract
The genetic basis of cardiac electrical phenotypes has in the last 25 years been the subject of intense investigation. While in the first years, such efforts were dominated by the study of familial arrhythmia syndromes, in recent years, large consortia of investigators have successfully pursued genome-wide association studies (GWAS) for the identification of single-nucleotide polymorphisms that govern inter-individual variability in electrocardiographic parameters in the general population. We here provide a review of GWAS conducted on cardiac electrical phenotypes in the last 14 years and discuss the implications of these discoveries for our understanding of the genetic basis of disease susceptibility and variability in disease severity. Furthermore, we review functional follow-up studies that have been conducted on GWAS loci associated with cardiac electrical phenotypes and highlight the challenges and opportunities offered by such studies.
This article is part of the Spotlight Issue on Inherited Conditions of Arrhythmia.
1. Introduction
The genetic basis of cardiac electrical phenotypes has in the last 25 years been the subject of intense investigation by many research groups worldwide. In the first years, such efforts were dominated by the study of the familial arrhythmic disorders presenting at young age such as the long-QT syndrome (LQTS). In recent years, large consortia of investigators have successfully pursued genome-wide association studies (GWAS) for the systematic identification of common single-nucleotide polymorphisms (SNPs) that govern inter-individual variability in electrocardiographic (ECG) parameters (e.g. QRS-duration and QT-interval) in the general population. The concept behind this approach is, that as candidate intermediate phenotypes of arrhythmia, insight into the genetic underpinnings of these parameters will add to our understanding of genetic factors that contribute to arrhythmia susceptibility in the setting of complex inheritance (oligogenic or polygenic).
Since the first GWAS conducted on the QT-interval in 2006,1 numerous GWAS studies on cardiac ECG parameters conducted in large samples of the general population have been published. A few studies have also started to relate such identified genetic variants to susceptibility or severity of inherited cardiac electrical disorders, primarily LQTS,2 and to complex arrhythmia affecting older individuals in the general population.3 GWAS has also demonstrated that some disorders previously considered Mendelian actually have a complex genetic basis wherein the accumulation of multiple genetic variants determines risk for the disorder.4 We here provide a review of GWAS conducted on cardiac electrical phenotypes (ECG traits and arrhythmia disorders) in the last 14 years. We discuss the implications of these discoveries for our understanding of the genetic basis of disease susceptibility and variability in disease severity. Furthermore, we review functional follow-up studies that have been conducted on some GWAS loci associated with cardiac electrical phenotypes and highlight the challenges and opportunities offered by such studies. To enhance the readability of the review, we have compiled a glossary of key words that are frequently used in genetic studies.
2. Genome-wide association studies
The genetic study of human disorders has been classically divided into the study of Mendelian diseases caused by a genetic variant of large effect (monogenic), and the study of common disorders presumed to be caused by the aggregate effect of multiple common variants of small to intermediate effect (polygenic). Variants that contribute to disease occur at different frequencies in the general population and are characterized by different effect sizes5 (Figure 1). Genetic variants that underlie Mendelian inheritance have been established to be very rare6 in the general population and found to be associated with a large effect size. Concerning non-Mendelian genetic variants that contribute to disease in the setting of complex inheritance, the effects of two types of variants are detectable with current approaches and study sample sizes. These comprise common variants [commonly considered to be those with a minor allele frequency (MAF) >5%] of small-effect and low-frequency variants (MAF 1–5%) that usually have effect sizes that lie between those of common and Mendelian variants.
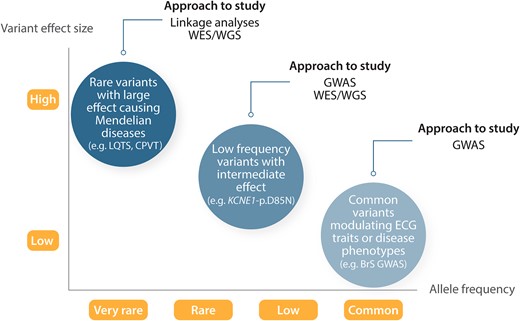
Genetic architecture of human disease. Genome-wide association studies for atrial fibrillation, ECG traits, and other complex traits have explored common variants (MAF >5%) and established many associations with modest to low effect size on disease risk. Whole-exome and -genome sequencing studies seek to further identify low-frequency (MAF 1–5%) and rare variants within genes or across the entire genome, respectively. The effect sizes of less common and rare variants are greater than for more common variants. The figure is adapted from Manolio et al.,5 permissions obtained.
Common genetic variants are studied by means of GWAS.7 The GWAS approach depends heavily on and makes use of the notion that variants that lie in close physical proximity to each other exhibit linkage disequilibrium (LD) and are often inherited together as a haplotype.8 This means that to identify a genetic association one could measure directly the variant causing the effect on the trait or any other variant that is correlated (referred to as being in LD with each other) to the causal variant (Figure 2). Intuitively (as discussed further down), this also implies that genetic associations uncovered through GWAS do not point to a specific variant or gene but rather the genetic region (i.e. the locus) containing variants in LD with the strongest trait-associated variant. Most genotyping arrays employed in GWAS typically target 200 000–2 000 000 common variants. The empirical determination of genotypes by means of array genotyping is nowadays almost invariably followed by imputation, a process of using the known correlation between genetic variants in order to predict (impute) genotypes that are not directly typed in the study population.8 Among others, genotype imputation facilitates meta-analysis across multiple GWAS studies. Genome-wide association analyses are typically performed on binary traits [e.g. patients with atrial fibrillation (AF) vs. controls] or quantitative traits (e.g. PR-interval, QRS-duration, or QT-interval in ms). Population stratification due to different ancestry composition between cases and controls is an important confounder in GWAS and should be accounted for. Population stratification may lead to spurious association due to differences in MAF of genetic variants across individuals of different ancestries. The most common approach for the identification (and subsequent exclusion) of individuals with large difference in genetic ancestry is principal component analysis (PCA). In addition, to further account for differences in ancestry, principal components (PCs) generated from PCA can be included as covariates in the GWAS regression models.
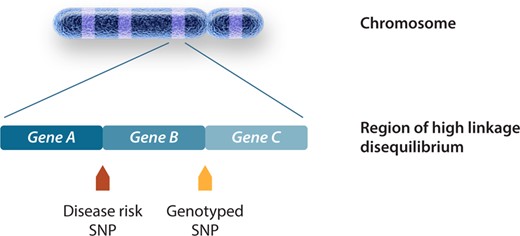
Genomic regions associated with phenotype through GWAS. Causal variants have a direct biological effect and therefore a direct effect on the phenotype. Causal variants are responsible for the association signal at a locus, although the association may be identified by using other non-causal variants in LD with the causal variant. The genotyped SNP will be statistically associated with disease as a surrogate for the disease SNP through an indirect association. The figure is adapted from Bush et al.,7 permissions obtained.
Variants with an association P-value <5×10−8 are considered genome-wide significant, based on multiple testing correction for the roughly 1 000 000 independent common variant tests (haplotypes) in the human genome.9 In addition to the identification of trait-associated loci, GWAS also enables the estimation of SNP-based heritability, that is, the proportion of the total phenotypic variance caused by the additive effects of the variants included in a GWAS.10
3. Polygenic risk scores derived from GWAS
A polygenic risk score (PRS) is typically calculated as the sum of disease/trait-associated alleles, each weighted by its effect size. A PRS assumes that multiple SNPs of modest effect size that are responsible for the phenotype act additively (Figure 3).11,12 A PRS for a given trait is usually derived from SNPs that have been associated with the trait at genome-wide statistical significance (i.e. P < 5×10−8) or at an arbitrary P-value threshold (e.g. P < 1×10−5). By considering multiple SNPs, a PRS explains a larger proportion of variance for a given phenotype compared to single SNPs, which makes it statistically powerful. It therefore provides an important means for the direct investigation of results from GWAS in clinical populations.
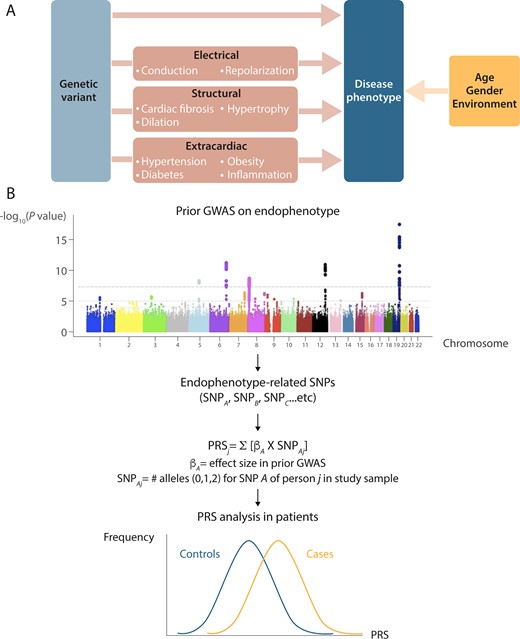
From GWAS to polygenic risk scores (PRS). (A) An approach that is likely to favour the identification of genetic variants that impact on risk for cardiac electrical phenotypes is testing genetic variants that have been shown to be associated with phenotypes that are considered as risk factors (endophenotypes). Genetic modifiers of these endophenotypes are thus expected to also determine the risk of the cardiac electrical phenotypes. For instance, slowed conduction is an established mediator of arrhythmia and therefore genetic factors that modulate the QRS-interval may also affect arrhythmia risk. (B) Summary how GWAS findings provides a unique opportunity for direct investigation of results from GWAS to clinical populations. The use of the endophenotype approach in the dissection of genetics of cardiac electrical phenotypes may increase the chances of genetic locus discovery as it considers candidate SNPs in pathways that are biological plausible for these disorders. The PRS summarizes risk-associated variation across the genome by counting the number of disease-associated alleles. Endophenotypes that are correlated with the disease phenotype because of overlapping genetic causes would be expected to be correlated with PRS for the disease as well. PRS can differentiate cases from controls on a population level, cases are expected to have a significantly higher mean PRS than controls. GWAS, genome-wide association study; PRS, polygenic risk score.
Several studies across different fields of medicine, including coronary artery disease, diabetes, obesity, cancer, and Alzheimer disease have linked risk prediction based on PRSs to actionable outcomes such as the prioritization of preventive interventions and screening, prediction of age of disease onset, and benefit from lifestyle modifications.11 Yet, while these initial observations are promising, PRS are not yet implemented clinically, and their future utility in routine clinical practice is an active topic of discussion.13 Among the barriers that currently hinder the clinical implementation of PRS is their limited discriminative capacity, that is the degree to which they are capable of separating the individuals into categories with sufficiently distinct degrees of absolute risk to drive clinical or personal decision-making.11 Another obstacle to their broad implementation in clinical practice is the fact that, since the vast majority of GWAS has been conducted in European-descent individuals, PRS derived from these GWAS may not be transferrable to individuals from other populations, highlighting the importance of diversification of GWAS into non-European populations.14 Finally, their clinical utility remains to be evaluated in prospective studies together with established clinical risk factors.
PRS can also be used to explore shared genetic loci and pathways between related phenotypes.15,16 Notably, in comparison to other laboratory-based biomarkers, the underlying biological pathways of the genetic variants included in the PRS are often unknown.
4. Genome-wide association studies of ECG parameters
While common genetic variants that modulate risk of arrhythmia can be identified in GWAS studies that directly compare patients with arrhythmia to controls, it may not always be feasible to recruit sufficiently large arrhythmia patient sets to allow for statistically adequately powered risk variant discovery. For example, while patients with certain arrhythmias such as AF are relatively easy to recruit for genetic studies, the recruitment of patients with other arrhythmias, such as VF in the setting of acute myocardial infarction, may be more challenging. An approach that circumvents this issue, and that is considered complementary to the direct discovery of risk variants in patients, entails first identifying genetic variants that impact on plausible biological pathways that underlie arrhythmia, and subsequently testing the identified variants for a possible role in arrhythmia risk. Biological pathways underlying cardiac electrical function, such as conduction and repolarization, are intuitively highly plausible biological pathways for arrhythmia. Thus, ECG traits such as heart rate and conduction (PR-interval, QRS-duration) and repolarization parameters (QT-interval), are considered highly relevant ‘intermediate phenotypes’ of cardiac arrhythmia.17 ECG parameters can be measured accurately and efficiently in large numbers of individuals and have been shown to display significant heritability (reviewed in Ref.18) making them attractive traits to study by GWAS. The validity of the intermediate phenotype approach to dissecting the genetic basis of complex arrhythmias is exemplified by the fact that genetic loci identified by GWAS as modulators of the PR-interval were subsequently demonstrated to play a role in AF susceptibility.19
The QT-interval was one of the very first phenotypes to be investigated by GWAS.1 This early study associated common genetic variation at the NOSA1P gene, encoding nitric oxide synthase 1 adaptor protein, with the QT-interval. This gene had not been previously implicated in cardiac repolarization, and this first study therefore made it immediately clear that GWAS could provide new insight into the biology of cardiac electrical function. Since this first study, several groups have dedicated much effort into identifying common genetic variants within the general population that modulate a variety of quantitative ECG traits (including P-wave, PR, QRS, QT, ST, JT, heart rate during rest, exercise, and recovery, and heart-rate variability). We here provide an overview of loci and SNPs identified to date for these different parameters (see Supplementary material online, Tables S1–S16). We identified SNPs by review of the NHGRI-EBI GWAS Catalog20 (https://www.ebi.ac.uk/gwas/, 7 January 2020) supplemented by a literature review to identify publications not indexed by the NHGRI-EBI GWAS Catalog (i.e. PR-interval,21 ST-segment,22 and JT-interval23) A total of 255 and 62 independent SNPs have been associated thus far with one or more ECG traits in European (see Supplementary material online, Table S1) and non-European populations (see Supplementary material online, Table S2), respectively. Although GWAS studies in populations of European-descent predominate, the genetic underpinnings of ECG parameters are increasingly studied in non-European populations.24–26
While early ECG GWAS, such as the first study on the QT-interval,1 included <1000 individuals, subsequent studies were conducted in increasingly larger samples of the general population, with the latest studies conducted in over 100 000 individuals. The increased GWAS sample sizes allowed the discovery of additional common variant loci (MAF > 5%) of progressively smaller effect size, as well as the identification of low-frequency (MAF 1–5%) variants,21,23,27 in line with GWAS for other complex traits.28 Although GWAS has uncovered a large number of loci for ECG traits, in aggregate they explain only a small portion of the heritability of these traits.29 It is expected that the heritability explained by genetic variation will continue to increase as the sample size of future GWAS continues to grow and by the systematic study of the contribution of low-frequency (MAF 1–5%) and rare (MAF < 1%) variants.
As expected, GWAS of ECG parameters identified genetic variation at genomic loci harbouring genes encoding proteins that have long been implicated in cardiac electrophysiology and in the genetic control of ECG parameters. For example, among the loci most strongly associated with ECG parameters of conduction (PR-interval, QRS-duration) is the SCN5A locus, encoding the cardiac sodium-channel NaV1.5 that underlies the depolarizing current INa. Similarly, among the loci most strongly associated with the QT-interval are those harbouring KCNQ1, KCNH2, KCNJ2, and KNCE1, that encode ion channel subunits pertaining to repolarizing potassium channels. Some associating loci harbour genes encoding developmental transcription factors that regulate of ion channel dosage in the adult heart, such as TBX5.30 For some of the candidate genes uncovered by GWAS, rare variants with large effect had been previously linked to Mendelian disorders; for example (i) heart-rate GWAS: HCN4 linked to familial sinus bradycardia,31 (ii) PR/QRS-GWAS: SCN5A linked to familial conduction defects,32 and (iii) QT-GWAS: KCNQ1, KCNH2, and SCN5A linked to LQTS.33 This underscores the fact that at a given locus, variants of different frequency and effects size may impact on cardiac electrical function. Although plausible genes occur at some loci, as highlighted further below, multiple loci associated with ECG parameters or arrhythmia phenotypes by GWAS do not harbour such genes, and functional follow-up of such loci provides unique opportunities for revealing novel mechanisms.
It has become clear that the same genetic variant can be significantly associated with multiple ECGs traits (pleiotropy).34 One of the most pleiotropic SNPs reported to date is rs6801957, located within the SCN10A gene, which has been directly or indirectly (through SNPs in high LD with it) associated at genome-wide statistical significance with heart rate, PR-interval, QRS-duration, QT-interval, AF, and Brugada syndrome (BrS). It should be noted that such pleiotropy is not limited to loci containing ion channel genes, but is also observed at other loci such as those harbouring cardiac transcription factors. Intriguingly, some loci exhibit an apparently opposing direction of effect on different traits; for example, while the C-allele of rs11153730 prolongs QRS-interval (i.e. it delays ventricular conduction), it shortens the PR-interval (i.e. it hastens atrioventricular conduction).
Besides the standard ECG parameters, the early repolarization (ER) pattern, characterized by an elevation at the QRS-ST junction (J point) on the ECG, has also been the subject of investigation by GWAS. When ER pattern occurs in association with ventricular arrhythmia it is referred to as ER syndrome.35 In affected families, ER syndrome/pattern has been observed to be inherited in autosomal dominant fashion.36 Two studies have shown that the ER pattern is more prevalent among first-degree relatives of patients with sudden arrhythmic death syndrome in comparison to control individuals, and another study in a large family-based cohort reported a strong heritability for the ER pattern.37 While these studies attest to the presence of inherited susceptibility factors, a recent study showed only modest SNP heritability.38 Candidate gene-based approaches in patients with idiopathic VF and the ER syndrome/pattern uncovered rare variants in a few genes encoding cardiac ion channels. However, these associations await confirmation by unbiased genetic approaches in families with clear segregation.39 Two studies applied the GWAS approach in order to identify common genetic variants that modulate susceptibility to the ER pattern. While the first GWAS (452 cases) for the ER pattern failed to uncover genetic variants at genome-wide statistical significance,40 a more recent and larger GWAS (3334 cases) identified a locus in KCND3, encoding the potassium voltage-gated channel subfamily D member 341 (see Supplementary material online, Table S13). In the cardiac ventricle, KCND3 contributes to the fast-cardiac transient outward potassium current (Ito) critical for the phase-1 repolarization of the action potential.41 The same locus had previously also been linked to P-wave terminal force and AF through GWAS (see Supplementary material online, Tables S3, S14, and S15).
5. The inherited cardiac arrhythmia syndromes
The Mendelian inheritance observed in some inherited cardiac arrhythmia syndromes such as LQTS and the large effect sizes of the causative rare pathogenic variants (mutations) enabled the identification of the underlying disease genes through linkage studies in large affected family pedigrees, beginning in the 1990s with the discovery of KCNQ1, KCNH2, and SCN5A as disease genes for LQTS.42–44 Multiple genes underlying inherited cardiac conditions have been identified in this way33 and genetic testing of these disease genes is recommended and regularly pursued for a number of these disorders. Yet, the decreased penetrance (not all carriers of the familial genetic defect manifest the disorder) and variable expressivity (different severity is observed among carriers of the familial genetic defect) that is observed in affected families, currently hinders the clinical utility of genetic testing. A role for additional genetic factors, commonly referred to as genetic modifiers, in determining the ultimate disease manifestation is suspected and in recent years efforts into the genetic basis of inherited electrical disorders affecting the young has shifted to the identification of such genetic modifiers. Genetic modifiers may act to aggravate the severity of the Mendelian genetic defect or may protect a carrier from developing the disease. Furthermore, while the Mendelian inheritance paradigm has been successfully applied in tracking down genes underlying some electrical disorders, others, such as BrS and ER syndrome, have defied genetic elucidation. The sporadic presentation that is often observed for these disorders, points to a more complex (polygenic) genetic architecture whereby multiple inherited genetic variants conspire to determine risk. Genetic studies into these disorders in recent years have started to address this complex inheritance paradigm. The development of genomic risk prediction, encompassing both common and rare variants, in the inherited cardiac arrhythmia syndromes is expected to provide a more complete genomic risk prediction profile representative of the whole spectrum of contributory genomic variation.
5.1 Long-QT syndrome
In the LQTS, a number of studies have investigated the possible modulatory role of genetic variants previously associated with the QT-interval in GWAS (hereafter referred to as ‘QT-SNPs’). The premise in these studies was that genetic factors contributing to QT-interval (a relevant intermediate phenotype for LQTS) variability in the general population, may impact on variability in LQTS susceptibility or severity among individuals with disease-causing (Mendelian) mutations in the established LQTS disease genes. A number of these studies have demonstrated effects of individual QT-SNPs, namely at NOS1AP, KCNQ1, and KCNE1 on the QT-interval and/or risk of arrhythmia in patients with LQTS.2,45–50 As expected for small-effect common variants, they individually explain only a small portion of inter-individual variability in phenotype among patients with LQTS. One exception in this respect is possibly the low-frequency p.Asp85Asn variant in KCNE1 (MAF = 1% in populations of European ancestry) which has the largest effect size of all ‘QT-SNPs’ uncovered thus far in GWAS (7.42 ms per minor allele).51 It has been shown to predispose to LQTS in two studies46,49 and was reported to modulate the QT-interval in Finnish males with the KCNQ1-p.Gly589Asp founder mutation.50 In fact, this variant may also be considered a low-penetrance rare variant that could, in turn, be modulated by other genetic or non-genetic factors such as QT-prolonging drugs. In an effort to explain a greater portion of the variability, one study conducted in patients with LQTS type 2 (i.e. with a Mendelian mutation in KCNH2) studied the effect of multiple SNPs in aggregate in the form of a PRS. A PRS consisting of 22 ‘QT-SNPs’ showed a strong association with the QT-interval in these patients. Furthermore, this study showed that patients with a PRS in the highest quartile displayed a higher risk for cardiac arrhythmia in comparison to patients in the lowest quartile.
Despite these reported associations, however (as discussed in general terms in the section on PRSs above), the testing of PRSs is not yet implemented in clinical genetic testing of LQTS patients. First of all, the robustness of such scores in predicting arrhythmic events in LQTS patients, still needs to be investigated in additional studies. Moreover, the discriminatory capacity of such scores, that is their ability to distinguish between those at risk and those that are not, needs to be improved before they become useful for the determination of risk in the individual patient. Such discriminatory capacity is expected to improve as more ‘QT-SNPs’ are identified and incorporated into such scores (since they will then explain a larger portion of inter-individual disease variability). The largest GWAS for QT-interval conducted so far (in up to 100 000 individuals of European descent) uncovered 68 ‘QT-SNPs’ that in aggregate explain 8–10% QT-interval variation in the general population.51 Future GWAS studies on larger sets of the general population have the potential of identifying additional ‘QT-SNPs’. Crucially, as discussed above, larger sample sizes for ‘QT-SNP’ discovery will allow the detection of common SNPs of lower effect size as well as low-frequency and rare variants of intermediate effect. Further investigation of the utility of such improved PRS based on ‘QT-SNPs’ in patients with LQTS requires the establishment of large (multi-centre) cohorts with available clinical and genetic data [as is ongoing for example in the setting of the ‘LQTS-NEXT’ European Joint Programme on Rare Diseases (EJP RD) led by C.R.B.], to enable statistically well-powered studies to identify predictors of cardiac arrhythmia. Finally, the clinical utility of such a PRS will need to be evaluated in prospective studies together with established clinical risk factors.
5.2 Brugada syndrome
The BrS is an inherited cardiac arrhythmia syndrome predisposing to sudden cardiac death (SCD) and hallmarked by ST-segment elevation and T-wave inversion on the surface ECG.52 In around 20% of cases, a rare variant is found in SCN5A, while the majority still remains genetically elusive.53 Recent observations have questioned the notion that BrS is a Mendelian disease and suggested a more complex inheritance.4 These observations include among others the observation of low disease penetrance and the occurrence of phenotype-positive genotype-negative individuals in families harbouring an SCN5A rare variant.54 In addition, a large proportion of cases are sporadic55 and linkage analyses in families have largely been unable to identify novel genes associated with the BrS. In 2013, the GWAS approach was applied to identify common genetic variants that predispose to BrS by comparing patients diagnosed with the BrS with individuals from the general population.4 This study identified three loci, of which two were located near SCN5A/SCN10A and one near HEY2, that were significantly associated with BrS (see Supplementary material online, Table S12). When these three loci were considered in aggregate in an (unweighted) PRS, they were found to confer a large effect on disease susceptibly for the disorder. This study provided proof of principle evidence that common genetic variation can impact on susceptibility to a rare cardiac arrhythmia disorder previously presumed to be monogenic.
6. Cardiac arrhythmia affecting the older segment of the population
Arrhythmia disorders affecting the older segment of the population, such as AF and ventricular fibrillation (VF) in the setting of acute ischaemia, myocardial infarction, or heart failure have long been considered complex disorders where multiple genetic and environmental factors together determine risk. The availability of large collections of patients, particularly those with AF,56 has enabled the exploration of common genetic variants in susceptibility to these arrhythmias by GWAS.
6.1 Ventricular fibrillation and SCD
VF is the most common mechanism of SCD in the general population for which the most common pathologic substrate is coronary artery disease. Several studies (reviewed in Ref.57) support a role for inheritable factors in the determination of VF in this setting. For example, in the Paris Prospective I study,58 a long-term (>20 years) cohort study of >7000 middle-aged French men, parental history of SCD was a predisposing factor for SCD, with risk being higher if both parents had SCD compared to if only one parent had SCD. In a different study, the AGNES study, that was conducted exclusively in patients presenting with a first acute myocardial infarction, SCD among first-degree relatives was identified as an independent risk factor for VF.59 Similar results were observed in the Danish GEVAMI study.60
Despite such evidence for genetic factors however, the identification of the contributory common genetic variants by GWAS has proved challenging. This is likely due to a number of factors among which: (i) studies so far have included a relatively small number of patients (and thus had limited statistical power); (ii) most studies included different causes of VF with consequent aetiological heterogeneity (also decreasing statistical power for detection of association with common variants); and (iii) the fact that some studies have used sudden death as a surrogate for VF (and thus a proportion of deaths may have been of non-cardiac or non-arrhythmic origin). However, two studies reported genome-wide significant associations with VF/SCD (see Supplementary material online, Table S16).61,62 The first study conducted in the AGNES case–control set, comprising 515 patients with a first acute ST-elevation myocardial infarction with VF before reperfusion therapy and 457 patients with first STEMI without VF, identified an association on chromosome 21q21 near the CXADR gene (rs2824292) at genome-wide statistical significance.61 The other GWAS,62 which consisted of a meta-analysis in 1283 SCD cases and >20 000 control individuals of European ancestry from five studies, with follow-up genotyping in up to 3119 SCD cases and 11 146 controls from 11 European ancestry studies, identified an association signal on chromosome 2q24.2 near the BAZ2B gene (rs4665058). However, both association signals did not reach genome-wide statistical significance in a more recent meta-analysis of nine SCD studies of European-descent individuals (3939 cases and 25 989 non-cases).3 Interestingly, however, by using Mendelian randomization, the latter study provided evidence for causal associations between certain observational risk factors and SCA, namely coronary artery disease status, body mass index, and QT-interval. The use of genetic data to untangle risk factor relationships in this way has the potential of enhancing our understanding of SCA pathophysiology. It also further underscores the role of genetic factors in risk for SCD in the general population.
As mentioned above, heterogeneity of the aetiology and the mechanism of death in the SCD case sets studied to date by GWAS dilutes genetic associations, hindering their identification.3,57 Though challenging, efforts in establishing large homogenous SCD sets are considered vital for the identification of the underlying genetic factors by GWAS.
6.2 Atrial fibrillation
Similar to VF in the general population, the systematic search for genetic factors that modulate risk of AF is motivated by studies pointing to inherited genetic factors. For example, an analysis conducted in the Framingham Heart Study demonstrated that parental AF is a risk factor for AF63 and a Danish study showed higher concordant AF status for monozygotic twins compared to dizygotic twins.64 However, in contrast to VF, substantial progress has been made in the identification of common susceptibility variants contributing to AF,65,66 fuelled at least in part by the availability of very large sets of individuals with AF. The first GWAS for AF was published in 2007 on an Icelandic sample, identifying two independent SNPs on chromosome 4q25.67 The association between common variants at this locus and AF was subsequently confirmed in patients of different ethnicities underscoring the central role of this locus in AF susceptibility (see Supplementary material online, Table S15).68–72 Since that initial GWAS, large consortia have been formed that brought together tens of thousands of patients allowing for GWAS with sufficient statistical power to further dissect the genetic architecture of AF. The latest two of such studies were conducted, respectively, in 65 446 AF cases of different ancestries (mostly European and Japanese) vs. 522 744 control subjects,56 and in 60 620 European ancestry AF cases vs. 970 216 controls.73 They identified 97 and 111 genomic loci, respectively, some of which harbour multiple independent associating variants. A preliminary meta-analysis for the top loci in non-overlapping participants from these two large efforts, with a resulting total of >93 000 AF cases and >1 million controls, identified at least 134 distinct AF-associated loci.56 Although a few SNPs have been suggested to be specific to individuals of Korean and Japanese populations68,70 an analysis of heterogeneity of effect estimates across ancestries in the Roselli et al. study56 did not identify significant heterogeneity, suggesting that top genetic susceptibility signals for AF have a relatively constant effect across ancestries. GWAS findings in AF have underscored the complex polygenic nature of the condition and provided new leads for pathophysiological insights into the condition by implicating genes involved in pathways beyond electrophysiological function, such as cardiac developmental, contractile, and structural pathways.
Studies on PRS for AF show that an estimation of genetic predisposition to AF is feasible with GWAS data and that PRS can be utilized in risk models.74–76 Lubitz et al. evaluated the association between AF and a PRS consisting of SNPs previously associated with AF (PRSAF), and found that PRSAF were associated with AF beyond established clinical risk factors, underscoring the important contribution of genetic variants in the identification of individuals at risk for AF.74,75 In addition, the individuals in the top PRSAF quintile had a 2.5-fold increased risk of cardioembolic stroke after adjusting for sex and age, suggesting that an elevated PRS may serve as a surrogate for thromboembolism from AF.74 Said et al.76 investigated the association of combined health behaviour and genetic risk (using a PRS based on AF-associated SNPs) in risk of incident AF in 339 003 unrelated individuals from the UK Biobank. In their study, in the low-genetic risk group (quintile 1), poor lifestyle increased the risk of incident AF >5-fold, compared to ideal lifestyle.76
7. Common variants and pharmacogenomics of arrhythmia susceptibility
Pharmacogenomics is the field that studies how the effect of single or multiple subclinical DNA variants may only become apparent upon drug challenge and may therefore underlie a wide range of adverse drug reactions, including proarrhythmia.77 While early studies focused on individual genetic variants in a few candidate genes, more recent studies have demonstrated the utility of GWAS in uncovering underlying genetic variation.
Drug-induced prolongation of the QT-interval and torsades des pointes has been an important reason for the withdrawal of marketed drugs and drugs that are in development.77 A GWAS conducted by Behr et al.78 for drug-induced torsades des pointes, comparing 216 drug-induced torsades des pointes cases of European descent with 771 ancestry-matched controls (including treatment-tolerant and general population subjects), did not uncover common variants reaching genome-wide statistical significance. On the other hand, candidate-based studies have shown an enrichment of genetic variants previously associated with the QT-interval in the general population in patients with drug-induced LQTS compared to controls. These included the KCNE1 missense variant p.D85N (rs1805128) and SNPs at NOS1AP.51,79 In a more recent study, a PRS derived from 61 variants associated to baseline QT-interval (PRSQT) in GWAS in the European general population was shown to explain a substantial proportion of response of the QT-interval to three QT-prolonging drugs (i.e. dofetilide, quinidine, and ranolazine) in healthy individuals.80 Of note, re-analysis of the data generated in the setting of the GWAS of Behr et al.,78 this time considering the 61-SNP PRSQT, showed that it was also a significant predictor of drug-induced torsade de pointes in that set.80 In aggregate, these data provide strong support for the role of inherited genetic factors in the determination of response to QT-prolonging drugs.
Impaired cardiac depolarization predisposes to cardiac arrhythmia by facilitating re-entrant excitation. Sodium-channel blockers that impair cardiomyocyte depolarization have been associated with conduction slowing and cardiac arrhythmia.81–83 In a study conducted in patients with suspected BrS, we recently showed that PRSs derived from GWAS conducted for PR-interval (PRSPR) and QRS-duration (PRSQRS) are associated with the response of PR-interval and QRS-duration, respectively, during infusion of the sodium-channel blocker Ajmaline.81 Furthermore, we showed that a PRS derived from a GWAS conducted on BrS (PRSBrS) was able to predict the development of a type I BrS ECG during infusion of Ajmaline.
Translation of these pharmacogenomic findings to clinical applications may be envisaged in a number of settings. Pre-emptive genotyping of SNPs prior to prescription of drugs with QT-prolonging or cardiac sodium-channel blocking activity may be used to identify patients at risk of drug toxicity. This may in turn lead, for example, to choosing alternative therapy or to a closer ECG monitoring of those at risk. Concerning sodium-channel blocker challenge in suspected BrS, SNP genotyping may be performed to assess pre-test probability when considering drug testing. A BrS diagnostic algorithm integrating SNP genotyping could have several potential advantages compared with current practice, namely, reduction of test-related adverse events (such as life-threatening arrhythmia), reduced costs (considering the higher expenses of sodium-channel blocker testing compared with SNP genotyping), and identification of family members at risk of BrS in centres with limited access to drug testing or no access to ajmaline. Yet, the discriminatory capacity of the various PRS is currently modest and it is expected that further understanding of the genetic determinants of drug response would improve their utility in risk assessment. Furthermore, prospective studies are needed to assess the predictive values and cost-effectiveness of these PRSs in a real-world setting.
8. From GWAS locus to gene and mechanism
As discussed above, some loci associated with ECG parameters or with AF harbour genes with a well-recognized role in cardiac electrical function, some of which have also been implicated in Mendelian cardiac rhythm disorders (e.g. KCNQ1, KCNH2, and KCNE1 at QT-interval associating loci and SCN5A at the PR- and QRS-interval loci). However, the majority of loci does not harbour recognized genes, and while functional follow-up of such loci provides unique opportunities for revealing novel mechanistic insight, their study has markedly lagged behind locus discovery due to the fact that a number of factors complicate the elucidation of the underlying causal variant and causal gene (Figure 4).84
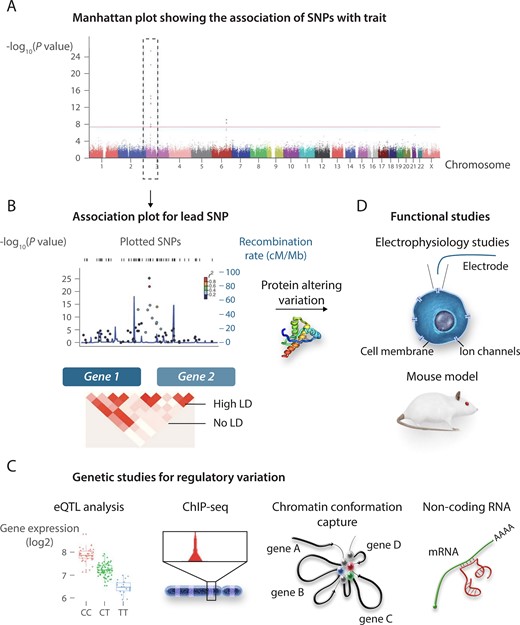
From GWAS locus to gene and mechanism. (A and B) Follow-up studies on loci identified in GWAS. In the absence of a candidate coding region variant that can be tested directly in functional studies (C), studies on non-coding putatively regulatory genetic variation may involve experimental approaches (e.g. eQTL, CHiP-seq, circularized chromosome conformation capture, and non-coding RNA analyses) to uncover the genetic mechanism and the regulated gene (D). GWAS, genome-wide association study; LD, linkage disequilibrium, SNP, single nucleotide polymorphism; CHIP-Seq, chromatin immunoprecipitation sequencing. The figure is adapted from Bezzina et al.,33 permissions obtained.
Isolating the causal variant is complicated by the fact that the sentinel SNP at an associating locus (i.e. the SNP with the lowest P-value of association) is co-inherited with many other SNPs within a haplotype (i.e. they occur in strong LD with each other), and their high correlation makes them statistically indistinguishable. In some cases, haplotypes overlap the coding region of genes and harbour SNPs that lead to amino acid altering changes that can be directly tested in functional studies. However, as for GWAS in general, most of the SNPs/haplotypes associated with cardiac electrophysiological phenotypes occur in non-coding (e.g. introns and intergenic) regions of the genome and are thought to act by altering the functionality of tissue-specific regulatory elements. Regulatory elements regulate the dosage of target genes through transcription factor binding and DNA looping, which brings the regulatory elements and target gene promoter(s) in close physical proximity in the three-dimensional space. Many loci implicated by GWASs are therefore thought to affect the associated phenotype by modulating the dosage of one or more target genes. Identifying the likely causal genetic variant within a haplotype, the regulatory element in which it acts and its target gene currently requires a laborious exercise entailing cross-referencing of multiple data sets including transcriptomic data [such as expression quantitative trait locus (eQTL) data], epigenomic data (such as data on chromatin accessibility), transcription factor binding data, and chromatin conformation data obtained in the relevant tissue (i.e. heart) (Figure 4).
Such an extensive approach for causal variant and gene identification was used by van den Boogaard et al.85 in their study of the rs6801957 polymorphism. Rs6801957 is located in an intron of the SCN10A gene and has been associated directly, or by virtue of LD with other SNPs (r2 > 0.8), with several ECG parameters of conduction (PR-interval, QRS-duration) and with susceptibility for AF and BrS. Through a series of studies on homologous regions in mice, they demonstrated that rs6801957 is located within a regulatory element that is bound by cardiac transcription factors, and which interacts with the promoter of Scna5 (encoding the cardiac sodium-channel NaV1.5), affecting Scn5a expression in the heart. In line with this, in humans, the rs6801957 variant was associated with reduced SCN5A mRNA expression in heart.85 A mechanism involving NaV1.5 is in line with the critical role of this ion channel in cardiac conduction. Similar work was subsequently conducted by the same group on another SNP, rs6781009, also supporting its involvement through regulation of expression of SCN5A.86 Rs6781009 is in LD with rs10865879 (r2 =0.95) that has been associated with QRS-duration and with rs6793245 (r2 = 0.57) that has been associated with the QT-interval.
PITX2, encoding the paired-like homeodomain transcription factor 2, has long been considered as a highly likely gene underlying the effect of AF risk variants at chromosome 4q25. Postnatally, Pitx2 is expressed in the left atrium and pulmonary vein, and its expression level decreases with age commensurate with late onset of AF susceptibility in humans.87 AF often arises from ectopic electrical activity originating from the pulmonary veins and the left atrium and Pitx2 has been shown to suppress a pacemaker gene expression signature.87,88 Furthermore, studies in mice revealed that Pitx2 haploinsufficiency and conditional postnatal ablation promotes an atrial arrhythmogenic phenotype.87,89,90 By demonstrating a functional connection between the upstream AF-associated variants, PITX2 expression, and predisposition to AF, a recent study by Zhang et al.91 has now provided conclusive evidence for PITX2 being the causal gene at this locus. Specifically, they showed that AF risk variants located at 4q25 reside in genomic regions possessing long-range transcriptional regulatory function directed at PITX2, and that the deletion of these genomic regions leads to reduced Pitx2c transcription and AF predisposition.91
A recent systematic assessment of 104 loci associated with AF, that integrated transcriptomic data and chromatin conformation data shortlisted genes likely affected by AF-associated variants and provided variant regulatory elements in each region that link genetic variation and target gene regulation. This gene prioritization strategy was validated in an experiment where a 40-kb region homologous to a human variant region 680 kb downstream of the GJA1 gene encoding connexin 43 led to a small but significant reduction in expression of Gja1, the most likely target gene in the prioritization strategy. Reduced Cx43 expression in mice leads to increased fibrosis during aging and is arrhythmogenic, suggesting that this could be one of the mechanisms through which modulated expression through this variant region adds to the risk of AF. This shortlist may help focus future efforts in studying genes underlying AF GWAS loci.
Although modulation of gene dosage through genetic variation in regulatory elements is clearly an important genetic mechanism of GWAS loci, other mechanisms by which associating variants modulate gene dosage should also be considered. For example, a study by Zhang et al.92 identified a microRNA-mediated mechanism for rs1805126, associated with QRS (lead SNP rs10865879; r2 = 0.62 with rs1805126) and QT (lead SNP rs6793245; r2=0.76 with rs1805126) in GWAS. In brief, they showed that miR-24 potently suppresses SCN5A expression and that rs1805126, a synonymous SNP in the terminal exon of SCN5A located adjacent to a miR-24 site, modulates this regulation. In support of this, the authors showed that the conduction-slowing allele at rs1805126 was associated with decreased cardiac SCN5A expression.
A few other studies prioritized genes for functional studies by virtue of their location with respect to the associating haplotype, that is they overlap with or are closest to the associating haplotype. The first of such studies in the domain of cardiac electrical traits concerned the study of NOS1AP at chromosome 1q23.3, the location of multiple haplotypes that modulate the QT-interval.93 Other genes studied by this approach include RNF207 at the 1p36.31 QT-interval locus,94,ZFHX3 at the 16q22.2 AF locus,95 and HEY2 at the BrS locus on chromosome 6.96 In such studies, besides biological plausibility, the demonstration of eQTL effects in cardiac tissue between the trait-associated locus and the candidate gene, were often invoked as evidence for involvement of the candidate gene. Another study implemented a medium-throughput screening approach in Drosophila melanogaster and Danio rerio to study 31 candidate genes from loci associated with heart rate, identifying 20 genes at 11 loci that are relevant for heart-rate regulation.97
A few studies investigated the electrophysiological consequences of amino acid altering variants located within associating haplotypes. Among these are patch-clamp electrophysiological studies on rs1805123 associated with QT-interval.98,99 This variant occurs in KCNH2 and leads to the p.Lys897Thr amino acid change in the KV11.1 channel underlying the rapid component of the delayed rectifier K+ current (IKr). While these studies indicated effects of the KCNH2-p.Lys897Thr amino acid change on KV11.1 channel function, they have proved inconclusive due to inconsistency across studies. Another example concerns electrophysiological studies of rs6795970, associated with multiple electrophysiological phenotypes including PR-interval, QRS-duration, BrS, and AF.100–102 This variant leads to the p.Val1073Ala amino acid change in SCN10A, encoding the sodium-channel NaV1.8. Of note, rs6795970 is in high LD with rs6801957 (r2 = 0.95) that was implicated in dosage modulation of SCN5A (reviewed in Ref.85) Whether the effect of the haplotype tagged by these two variants (i.e. rs6795970 and rs6801957) occurs through electrophysiological effects on NaV1.8 channel function100–102 or through dosage modulation of SCN5A remains unclear.85 The fact that SCN10A seems to be lowly expressed in cardiomyocytes seems to favour the latter mechanism, at least in this cell type.
In sum, locus discovery by GWAS has far out-paced the functional analysis of the identified loci. Many loci do not harbour known genes linked to cardiac electrical function. Such loci are largely untapped for gene identification and mechanistic insight. Though challenging, the functional follow-up of such loci has the potential of uncovering new biological insights. This is relevant when one considers the fact that beyond knowledge of the specific ion channels mediating the various ionic currents in the heart, our comprehension of the higher order regulators of cardiac electric function (such as the regulators of ion channels) remains rudimentary.
9. Conclusion and future perspectives
While there is no doubt that GWAS has achieved considerable success in dissecting the genetic architecture of ECG traits, a substantial proportion of the heritability has not been accounted for. As their discriminative capacity continues to grow by the identification of more SNPs, the assessment of the clinical utility of PRS necessitates prospective studies that combine PRS with established clinical risk factors. Although GWAS has the potential to provide new insights into the (patho-)physiology of cardiac electrical function by highlighting novel genes and key molecular pathways, the majority of susceptibility loci awaits functional follow-up. To increase our understanding of the genetic architecture of ECG traits, larger GWAS studies that allow the assessment of low-frequency and rare variants are required.
Supplementary material
Supplementary material is available at Cardiovascular Research online.
Acknowledgements
The authors acknowledge support from the Netherlands Heart Foundation (CVON PREDICT2 project and CVON CONCOR-genes project to C.R.B.), the Netherlands Organization for Scientific Research (VICI fellowship, 016.150.610, to C.R.B.), Fondation Leducq and the European Society of Cardiology (Research Grant to C.G.). The authors thank Gino Wong for help in compiling the supplementary tables.
Conflict of interest: none declared.
Funding
C.R.B. received funding from Fondation Leducq (17CVDV02). C.G. received funding from the European Society of Cardiology in form of an ESC Research and Training Grant.
Glossary
The following terms appeared in this Review. Some of these definitions were adapted from Refs.11,103
Complex trait—Trait that has a genetic component that does not follow strict Mendelian inheritance. May involve the interaction of two or more genes or gene–environment interactions. Also known as polygenic disease.
Expressivity—In variable expressivity, a phenotype may be stronger or weaker in different people with the same genotype. For instance, different severity is observed among carriers of the same familial genetic defect, some might develop a severe form of the disorder, while others might have a milder form.
Genetic architecture—Genetic basis underlying a trait in a population. It includes the number, frequency, and magnitude of effect, and their interaction, of the genetic variants that contribute to the trait.
Genome-wide association studies (GWAS)—An approach used in genetics research to associate genome-wide genetic variations with particular phenotypes. The method involves scanning the genomes from many different individuals to uncover genetics variants associated to a disease other phenotype of interest.
Imputation—Genotype imputation is a process of estimating missing genotypes, based on the statistical relationship of variants, from the haplotypes of a genotype reference panel.
Linkage disequilibrium (LD)—A state in which polymorphisms on the same chromosome are in partial or complete linkage. This most often occurs when the polymorphisms are in close proximity on the same chromosome and there are no recombination hotspots between them.
Locus (plural loci)—A locus is the specific physical location of a gene or other DNA sequence on a chromosome, like a genetic street address. A single allele for each locus is inherited separately from each parent.
Mendelian inheritance—Refers to the kind of inheritance where the trait is predominantly the consequence of a single genetic variant in one gene. Named for Gregor Mendel, who first studied and recognized the existence of genes and how genetic traits are passed from parents to offspring.
Mendelian randomization—Uses genetic variation to investigate the causal relations between potentially modifiable risk factors and health outcomes in observational data.
Monogenic—Same as Mendelian disease. Monogenic disorders are caused by a mutation in a single gene. The mutation may be present on one or both chromosomes (where one chromosome is inherited from each parent).
Oligogenic—Oligogenic inheritance represents an intermediary between monogenic (in which a trait is determined by a single genetic defect) and polygenic inheritance (in which a trait is influenced by many genetics variants and often environmental factors as well).
Penetrance—The likelihood that a person carrying a particular disease-causing variant will have the disease. Incomplete penetrance means that the penetrance is <100% and not all carriers of the familial genetic defect manifest the disorder.
Pleiotropy—Pleiotropic effects refers to the effects of a genetic variant on multiple biological pathways. These can either affect the outcome through another trait or pathway to the one under investigation, known as horizontal pleiotropy, or affect other traits through the risk factors of interest, known as vertical pleiotropy.
Polygenic—Polygenic traits are influenced by multiple genetic loci and/or the environment.
Population stratification—A form of confounding in GWAS caused by genetic differences between cases and controls unrelated to disease but due to sampling them from populations of different ancestries.
Principal component analysis (PCA)—PCA is a crucial step in quality control of genomic data and a common approach for detecting and adjusting for population stratification in GWAS.
Single-nucleotide polymorphisms (SNPs)—DNA sequence variation that occur when a single nucleotide (A, T, C, or G) in the genome sequence is altered. SNPs are the most abundant variation in the human genome and are the most common source of genetic variation.
SNP-based heritability—The proportion of the total phenotypic variance caused by the additive effects of the variants included in a genome-wide association study.
References
Cardiac Arrhythmia Suppression Trial (CAST) Investigators.
Talking Glossary of Genetic Terms—NHGRI [Internet]. https://www.genome.gov/genetics-glossary (20 February 2020, date last accessed).