-
PDF
- Split View
-
Views
-
Cite
Cite
Thomas Hill, John Rooney, Jaleh Abedini, Hisham El-Masri, Charles E Wood, J Christopher Corton, Gene Expression Thresholds Derived From Short-term Exposures Identify Rat Liver Tumorigens, Toxicological Sciences, Volume 177, Issue 1, September 2020, Pages 41–59, https://doi.org/10.1093/toxsci/kfaa102
- Share Icon Share
Abstract
Traditional methods for cancer risk assessment are resource-intensive, retrospective, and not feasible for the vast majority of environmental chemicals. In this study, we investigated whether quantitative genomic data from short-term studies may be used to set protective thresholds for potential tumorigenic effects. We hypothesized that gene expression biomarkers measuring activation of the key early events in established pathways for rodent liver cancer exhibit cross-chemical thresholds for tumorigenesis predictive for liver cancer risk. We defined biomarker thresholds for 6 major liver cancer pathways using training sets of chemicals with short-term genomic data (3–29 days of exposure) from the TG-GATES (n = 77 chemicals) and DrugMatrix (n = 86 chemicals) databases and then tested these thresholds within and between datasets. The 6 pathway biomarkers represented genotoxicity, cytotoxicity, and activation of xenobiotic, steroid, and lipid receptors (aryl hydrocarbon receptor, constitutive activated receptor, estrogen receptor, and peroxisome proliferator-activated receptor α). Thresholds were calculated as the maximum values derived from exposures without detectable liver tumor outcomes. We identified clear response values that were consistent across training and test sets. Thresholds derived from the TG-GATES training set were highly predictive (97%) in a test set of independent chemicals, whereas thresholds derived from the DrugMatrix study were 96%–97% predictive for the TG-GATES study. Threshold values derived from an abridged gene list (2/biomarker) also exhibited high predictive accuracy (91%–94%). These findings support the idea that early genomic changes can be used to establish threshold estimates or “molecular tipping points” that are predictive of later-life health outcomes.
Pathway-based information is a central element of current efforts to better assess health effects of environmental chemicals (Cote et al., 2016; Hutchinson et al., 2013; OECD, 2016). Historically, the characterization of toxicity pathways has been largely retrospective and qualitative, based on intermediate effects associated with a previously observed health outcome (Celander et al., 2011; Lalone et al., 2013). The adverse outcome pathway (AOP) concept was developed to facilitate more prospective quantitative analysis of key events (KEs) leading to a particular toxicity (Ankley et al., 2010; Burden et al., 2015). A central premise of this approach is that KEs are necessary at a qualitative level but may require a certain degree or amount of disruption to produce a given adverse outcome (Conolly et al., 2017). This premise has generated interest in the search for quantitative effect thresholds, or “molecular tipping points,” as a tool for adversity determinations using short-term data (Hill et al., 2017; Julien et al., 2009; Knudsen et al., 2015).
Dose thresholds have been used in toxicological science for many decades to set health guidance values for individual chemicals. Most commonly, these thresholds are based on no/lowest observed adverse effect levels or benchmark dose (BMD), which is the calculated dose corresponding to a specified benchmark response (BMR), such as BMD1SD, which is the calculated dose corresponding to the BMR of 1 SD (one standard deviation) change beyond control (Davis et al., 2011; Thomas et al., 2013). This method was developed to calculate chemical-specific departure thresholds using a direct comparison between dose and a quantitatively measured outcome, such as clinical chemistry values or qPCR ΔCt values. A successful BMR calculation does not, however, ensure that the BMD is biologically relevant—there is an implicit assumption in the derivation that a predetermined mathematical unit of separation (ie, 1 SD) is both adequate and appropriate for the prediction of a biological outcome. In addition, BMD quantifies the amount of a chemical producing a statistically significant outcome, rather than the level of biological effect in a cell, organ, or organism that predestines a toxic fate in a biological system. As such, the value is chemical-limited and must be rederived for each toxicant of concern. In contrast, an AOP-driven “effect threshold” based on established biological response-response relationships can operate across chemicals within a particular model or system. It defines, eg, the level of receptor activation that is needed for a mitogenic effect to occur, or the level of enzyme inhibition needed to induce cytotoxicity. By establishing chemical-agnostic hazard levels for biomarker data (based on intermediate KEs in an AOP), effect thresholds would provide key anchor points for biologically relevant, pathway-based risk assessment and incorporation of novel data streams for rapid detection of new toxicants. To date, however, few case studies have been developed specifically around this concept.
Short-term changes in molecular profiles are a central component of strategies to more rapidly identify and prospectively model health effects of environmental chemicals (Cote et al., 2016; Meek et al., 2014; NRC, 2007; Thomas et al., 2013). Use of higher-throughput data types should increase efficiency of chemical screening and prioritization, reduce the number of animals used in testing, and allow for better allocation of resources to chemicals with the greatest potential for human health risk. For cancer risk assessment, hazard is often based on outcomes in long-term carcinogenicity bioassays in rodents. Establishing reference dose (RfD) levels for known nonmutagenic carcinogens often requires additional mode of action (MOA) studies (Boobis et al., 2006). There is a recognized need to streamline this process and integrate newer types of information (Waters et al., 2010). According to the current 2005 EPA Cancer Guidelines (USEPA, 2005), an effect threshold for early KEs in an accepted MOA such as hyperplasia or increased cell proliferation should be protective across chemicals for the tumor outcome itself. Quantitative genomic data corresponding to the initial early events in a target pathway is routinely used as part of MOA evaluation, but rarely as a basis for setting RfDs. A better understanding of the quantitative relationships between early genomic changes and later tumor outcomes would enable more efficient assessment of known tumorigens and, in the future, more predictive approaches for screening data-poor chemicals.
Here, we hypothesized that expression patterns of genes specific to molecular initiating events (MIEs) in established cancer AOPs could be used to define chemical-agnostic thresholds for tumorigenesis and that these thresholds would be predictive for specific cancers. To test this hypothesis, we used induction of liver cancer in rats as a proof of concept case study. Gene expression biomarkers characterized in our previous work (Corton et al., 2020; Rooney et al., 2018) were applied as early indicators of genotoxicity, cytotoxicity, aryl hydrocarbon receptor (AhR), constitutive activated receptor (CAR), estrogen receptor (ER), and peroxisome proliferator-activated receptor α (PPARα) AOPs. Thresholds for these 6 biomarkers were determined using microarray data derived from 2 large comprehensive studies of short-term chemical or drug exposures. We show here for the first time that these MIE thresholds were relatively consistent across studies and together can be used to accurately identify rat liver tumorigens. This strategy supports efforts to incorporate new approach methodologies into cancer risk assessment and inform hazard identification based on short-term biological responses.
MATERIALS AND METHODS
Rationale for MIEs used in this study
This case study focused on liver cancer pathways for several reasons. (1) Liver is the most common site for treatment-related tumors in rodent carcinogenicity studies. For example, liver tumors were induced by 23% of chemicals with rodent carcinogenicity data previously reviewed by EPA (Hill et al., 2017). Of these, 74% (80/108) were originally classified as possible or probable/likely human carcinogens. (2) The vast majority of chemical-induced liver tumors in rodents are driven by MOAs/AOPs with well-defined early KEs (Holsapple et al., 2006). The 6 MIEs examined here are those most often cited in liver tumorigenesis in the rodent liver. (3) Gene expression biomarkers have been derived and published for these MIEs, as described in our previous work (Corton et al., 2020; Rooney et al., 2018). Only 2/38 tumorigenic chemicals in a rat liver microarray compendium failed to align with 1 of these 6 MIEs (Corton et al., 2020). It should be emphasized that in this study we did not construct new AOPs; rather, we tested the hypothesis that threshold analysis of previously published MIE biomarkers within these existing AOPs would accurately identify and classify putative liver tumorigens.
The gene expression biomarkers
There were 6 biomarkers, each representing activation of a different MIE, that were previously constructed from microarray data in the TG-GATES and DrugMatrix databases (Corton et al., 2020; Rooney et al., 2018). A brief description of the methodology and references for more detailed information can be found in Supplementary File 1. These databases include toxicology studies of compounds tested at up to 3 dose levels in acute and repeated exposures across multiple fixed sample times ranging from 3 h to 29 days. Collectively, there were > 3000 chemical-dose-time microarray comparisons (referred to as biosets) from TG-GATES alone. Only microarray data from repeat-dose exposures (> 1 day) were used here to derive thresholds and test their ability to identify tumorigens. All database downloads were extracted, manually curated, and collated in tabular form for analysis. Extracted data included chemical ID, dose, and study duration. Oral route of exposure was documented for all compounds as mg/kg/day. The biomarkers consist of sets of genes and associated fold-change values which in 4 cases (AhR, CAR, ER, and PPARα) were derived from microarray profiles from rats treated with prototypical activators of the transcription factor. The genotoxicity and cytotoxicity biomarkers were derived from sets of genes known to be induced by DNA damage or cytotoxic agents, respectively. The balanced accuracies of the genotoxicity, AhR, CAR, ER, PPARα, and cytotoxicity biomarkers were the following: 92%, 91%, 92%, 96%, 98%, and 96%, respectively (Corton et al., 2020; Rooney et al., 2018).
Comparison of microarray profiles to the gene expression biomarkers
To harmonize the outcome metric for our algorithm, the biomarker genes and their derived associated fold-change values were uploaded into the Illumina BaseSpace Correlation Engine (BSCE) database (https://www.illumina.com/informatics/research/biological-data-interpretation/nextbio.html; accessed March 28, 2019), and fixed internal protocols ranked the genes based on absolute fold-change. Each ranked list of genes in the database was then compared with all other ranked lists of genes in microarray comparisons using the Running Fisher test, which determines a correlation and associated p value for the overlapping genes between any 2 lists. This p value is then transformed to a −Log(p value), providing a simple metric to evaluate correlation between any 2 gene lists. A higher −Log(p value) indicates a more significant degree of correlation between the datasets. A negative correlation is reported as a negative integer.
Classification of liver cancer status for chemical-dose pairs
All chemicals in the TG-GATES and DrugMatrix studies were annotated for doses known to induce hepatocellular adenoma and/or carcinoma on histopathology in rat liver (“liver tumorigens”) based on information from the Carcinogenicity Potency Data Base (https://toxnet.nlm.nih.gov/cpdb/; accessed March 28, 2019), Physicians’ Desk Reference (1997), or in Pharmapendium (https://www.elsevier.com/solutions/pharmapendium-clinical-data; accessed August 28, 2019). The Carcinogenicity Potency Database is now found at ftp://anonftp.niehs.nih.gov/ntp-cebs/datatype/Carcinogenic_Potency_Database_CPDB/ (accessed March 9, 2020). Diagnostic annotations were made only when there was a clear response (positive or negative) at that dose level in male or female rats regardless of rat strain; a total of 562 and 183 chemical-dose-time comparisons could be annotated for liver tumor outcome in the TG-GATES and DrugMatrix studies, respectively. It should be noted that in contrast to other studies that assumed all doses of a tumorigenic chemical were actually tumorigenic, we made no assumptions about the ability of these chemicals to induce/not induce tumorigenesis (evidence is summarized in Supplementary File 2).
Determination of MIE thresholds
Our use of the term threshold is consistent with prior EPA definitions. For example, the EPA IRIS glossary defines a threshold as the “dose or exposure below which no deleterious effect is expected to occur” (http://www.epa.gov/ncea/iris/help/gloss.htm; accessed August 22, 2019). Similarly, another EPA source defines a threshold as either the “dose or exposure level below which a significant adverse effect is not expected to occur” or the “lowest dose of a chemical at which a specified measurable effect is observed and below which it is not observed” (http://www.epa.gov/OCEPATERMS/; accessed August 22, 2019). Based on methods outlined here, we state that “a toxicological threshold is an effect level above which a biological pathway is driven to an adverse outcome.” In this particular case study, the adverse outcome is an increase in hepatocellular adenomas and/or carcinomas.
To identify thresholds, we used the maximum −Log(p value) returned for each MIE biomarker from the nontumorigenic chemical-dose-time biosets. This value (MIEmax) became the “risk threshold” for each MIE biomarker. Thresholds were derived from a TG-GATES training set, the entire TG-GATES dataset, and the entire DrugMatrix dataset. These thresholds were then used to predict liver tumors in independent sets of test chemicals (Figure 1; and described below). In a few cases, there were clear outliers that were removed from the analysis and are noted below. Criteria for exclusion of the outlier biosets included graphical identification in box and whisker plots and a −Log(p value) ≥ 2 units above the next lowest bioset value for a MIE.
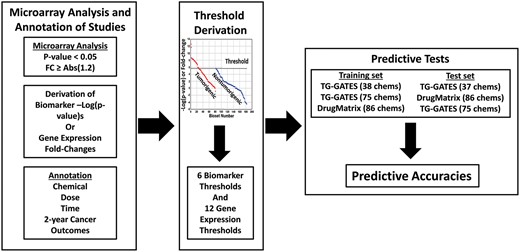
Strategy to identify toxicological thresholds and use them to predict liver cancer (see text for description).
Determination of individual gene fold-change thresholds
We also identified the thresholds for 2 of the positively regulated genes in each MIE biomarker; the genes were selected as they were included on both the TG-GATES and DrugMatrix microarray platforms. Thresholds were identified as the maximum fold-change value from the nontumorigenic chemical-dose-time biosets derived from the TG-GATES training, the entire TG-GATES dataset, or the entire DrugMatrix dataset. These thresholds were used to screen the TG-GATES and DrugMatrix biosets as described above. Once again, there were a few cases with clear outliers that were removed from the analysis and are noted below. Criteria for exclusion of the outlier biosets included graphical identification in box and whisker plots and confirmation of a bioset’s fold-change ≥ 2 units above the next lowest bioset value for that gene. Data transformations were performed in Microsoft Office Excel 2016 (Microsoft, Redmond Washington).
Derivation of gene expression changes from the TG-GATES dataset using R
Using the methods described by AbdulHameed et al. (2014), we obtained all .cel files associated with liver (ftp://ftp.biosciencedbc.jp/archive/open-TG-GATESgates/LATEST/Rat/in_vivo/Liver/) and the raw Affymetrix microarray data under Open-All Attribute (ftp://ftp.biosciencedbc.jp/archive/open-TG-GATESgates/LATEST), all published in 2012. We processed the .cel files associated with the treatment conditions using the package affy and the function rma from the Bioconductor library (Gautier et al., 2004; Gentleman, 2008). Next, we calculated robust multiarray averages to normalize the data using the function mas5calls (AbdulHameed et al., 2014; Gentleman, 2008; Kauffmann et al., 2009). The resulting dataset was then filtered using the nonspecific filtering function in the genefilter package to obtain only arrays which corresponded to Entrez IDs which are the nomenclature for gene expression used for primary text search and retrieval system defined by the National Center for Biotechnology Information (NCBI) using PubMed database (Gentleman, 2008). We also filtered the probe sets for expression in at least 20% of the replicates for each array (“Present” calls) (AbdulHameed et al., 2014; Gentleman, 2008). There were 3 replicates in each group. The final result after preprocessing the data was an ExpressionSet with 3674 observations.
Rank Product analysis was performed on the filtered ExpressionSet to identify a set of differentially expressed genes (DEGs) associated with liver cancer in rats. The Rank Product method is a nonparametric statistical test that converts scores from a fold-change matrix to ranks (AbdulHameed et al., 2014). These ranks indicate the likelihood that a gene will be up- or downregulated given a condition using the Bioconductor Package RankProd. The function RP, part of the RankProd package version 3.4.0, was applied to obtain the Rank Product statistics. Following the recommendations used in the Bioconductor Package, random seed was set to 123 to account for slight variations which occur during each permutation and to ensure reproducible results. After calculating Rank Product statistics, topGene function was used to generate a list of up- and downregulated genes for each of the treatment conditions. To compile significant DEGs, genes which had a p value less than or equal to 1, and percentage of false positive (FP) predictions less than 0.05 were selected (AbdulHameed et al., 2014). When mapped to treatment conditions, a list of up- and downregulated Probe IDs was generated. These Probe IDs were converted to rat genes using the rat2302 database from the biomaRt package in the Bioconductor library (Durinck et al., 2009). To ensure correct mapping of the gene annotation in the database, AnnotationDbi package from Bioconductor was loaded. Finally, the tables of DEGs were created by grouping all the Probe IDs that are either up- or downregulated and generated from 2 or more treatment conditions. The fold-changes were calculated using a log-ratio matrix of genes. First, the average intensity of the replicates of a chemical exposure condition was calculated, then the log-ratio of fold-change between the treatments and their corresponding controls for each gene was computed.
The gene fold-changes in log2 format were separated based on the tumor/no tumor status. These were plotted using ggplot2. The quick plot (qplot) for each gene is shown with the cancer and no-cancer fold-changes at different color. The fold-change thresholds for each gene were calculated as the minimum and maximum fold-change values after exposure to no tumor treatment conditions. The genes were also plotted using the boxplot package (part of the ggplot2 library) to understand the distribution of the fold-changes between tumor and no tumor treatment conditions. Finally, the nonparametric Mann-Whitney-Wilcoxon test was performed between the tumor and the no tumor treatment conditions in each gene. For this calculation, the wilcox.test package under stats library in R was used.
Determination of accuracy
Accuracy was determined either on an individual bioset level or by aggregating biosets that examine the same chemical. The tumorigenic prediction by chemical aggregate for each threshold derivation method was scored as true positive (TP), true negative (TN), FP, or false negative (FN) based on whether the −Log(p value)s or fold-change exceeded the thresholds for 1 or more of the MIEs. To be diagnosed as a FN, all biosets for a tumorigenic chemical had to be below the thresholds for every MIE. If even one of the biosets for a nontumorigenic chemical exceeded 1 or more thresholds, it was diagnosed as a FP. The calculations for predictive accuracy were the following: sensitivity (TP rate) = TP/(TP + FN); specificity (TN rate) = TN/(FP + TN); positive predictive value = TP/(TP + FP); negative predictive value = TN/(TN + FN); and balanced accuracy = (sensitivity + specificity)/2.
Additional methods
Visualization was carried out using the TreeView program from the Eisen lab (http://eisenlab.org/software.html; accessed March 28, 2019).
Supplementary methods
Supplementary Methods including description of the TG-GATES study, description of the DrugMatrix study, identification of DEGs in BSCE microarray datasets, and annotation of a rat liver gene expression compendium are found in Supplementary File 1.
RESULTS
Strategy to Identify Pathway-based Toxicological Thresholds Associated With Liver Cancer
We hypothesized that changes in biomarkers for pathway-based MIEs resulting from short-term exposure studies (≤1 month) exhibit measurable thresholds for increased incidence of liver tumors observed after 2 years of exposure, and that these thresholds could in turn be used to prospectively identify tumorigens. As summarized in Figure 1, our strategy tested these hypotheses using the TG-GATES and DrugMatrix datasets with independently reported 2-year liver tumor outcomes. As described in the Materials and Methods section, we determined thresholds for MIE biomarkers or a small group of individual genes derived from the biomarkers. Microarray data from exposures (>1 day) were used to derive thresholds. We used 3 sets of training data to derive the thresholds for the MIE biomarkers or individual gene clusters and used them to test independent sets of chemicals. The first approach used a training set of 38 TG-GATES chemicals to predict liver tumorigens in a TG-GATES test set of 37 independent chemicals or the DrugMatrix dataset. In the second approach, the entire TG-GATES dataset was used as the training set to predict liver tumorigens in the DrugMatrix dataset. In the third test, the DrugMatrix dataset was used as the training set to predict liver cancer in the TG-GATES dataset.
The TG-GATES dataset included 562 total comparisons at 4 time points (4, 8, 15, and 29 days), representing 75 chemicals in which liver tumor response was known (18 positives and 62 negatives; for 5 chemicals, there were doses that were either tumorigenic or nontumorigenic in 2-year bioassays). All gene expression profiles were generated on Affymetrix arrays. The DrugMatrix dataset included chemicals examined at 1 or 2 doses conducted at 2 time points (3 and 5 days). Out of the 183 chemical-dose-time comparisons, there were 77 chemicals in which liver tumorigenicity was known (35 positives and 45 negatives; for 3 chemicals, there were doses that were either tumorigenic or nontumorigenic in 2-year bioassays). The DrugMatrix biosets used in the analysis were generated on the Codelink microarray platform from GE Healthcare.
Identification of Thresholds for MIE Biomarkers
The range of −Log(p value)s in the biosets from the tumorigenic and nontumorigenic groups for the TG-GATES training and test sets are shown in Figure 2A. The −Log(p value)s were derived from the correlation between the indicated biomarker and individual microarray comparisons (see Materials and Methods section). In all cases, the maximum −Log(p value)s were higher in the comparisons for tumorigenic groups compared with the nontumorigenic groups. Thresholds were initially identified as the highest −Log(p value) for each biomarker in the nontumorigenic group (called the maximum returned value). Although most of the −Log(p value)s in the nontumorigenic groups were continuous, there were a small number of noticeable outliers at the high end in some training or test sets. We reasoned that inclusion of obvious outliers in our threshold determination would potentially result in generation of FNs that would affect performance accuracy. Because there were no prior examples of how to identify outliers for removal, we defined outliers that were greater than or equal to 2 −Log(p value) units from the next lowest value and easily observed in a box plot analysis. Based on these criteria, we removed from the TG-GATES training set 1 outlier from the genotoxicity biomarker threshold analysis and 2 outliers from the cytotoxicity biomarker threshold analysis (Figure 2B). To address possible sample bias in the division of the TG-GATES data into a training and test set, MIE thresholds were also derived from the entire dataset. The derived MIE thresholds were similar whether the entire dataset, the training subset, or the test subset was used despite the fact that the training and test subsets were independent lists of chemicals (summarized below). It should be noted that a small number of outliers that met the exclusion criteria were also identified in the test set and the entire dataset. In the test set, 1 outlier was excluded from the CAR biomarker analysis (Figure 2B). In the entire TG-GATES dataset, a total of 4 outliers were excluded including 1 each for the genotoxicity and CAR biomarkers and 2 for the cytotoxicity biomarker (Supplementary Figs. 2 and 3).

Identification of thresholds for gene expression biomarkers from the TG-GATES study. A, Distribution of values across training and test sets derived from the TG-GATES study. Thresholds were determined as detailed in the Materials and Methods section. Each of the microarray comparisons (biosets) was compared with 1 of the 6 biomarkers generating a −Log(p value) of the correlation. The −Log(p value)s were rank ordered and divided into liver tumorigenic (red) and nontumorigenic (blue) groups for training and test sets. Horizontal lines represent the derived thresholds. The bioset number refers to the rank of bioset ordered by −Log(p value). B, Box and whisker plots of −Log(p value)s from the nontumorigenic conditions. Left, values from the TG-GATES training set. Right, values from the TG-GATES test set. The 3 values deemed outliers in the training set are circled and were not used to determine the thresholds. One value in the constitutive activated receptor (CAR) biomarker from the test set was also considered an outlier. Abbreviations: AhR, aryl hydrocarbon receptor; ER, estrogen receptor; PPARα, peroxisome proliferator-activated receptor α.
We repeated the threshold derivations using the entire DrugMatrix microarray dataset to determine if the microarray platform used to collect genomic data along with a different set of chemicals significantly altered the MIE threshold values. The range of −Log(p value)s in the tumorigenic and nontumorigenic groups is shown in Figure 3A. Threshold identification was conducted as described above after 2 outliers were removed from both the ER and PPARα biomarker analysis. These outliers were also clearly identifiable in box plots of the noncarcinogenic biosets (Figure 3B). Thresholds derived from the training, test, and entire TG-GATES datasets exhibited similar values (Figure 4). The MIE thresholds derived from the DrugMatrix data were approximately 1.0–3.0 −Log(p value) units higher than those derived from the entire TG-GATES dataset for AhR, CAR, ER, PPARα, and cytotoxicity MIEs. The values for the genotoxicity MIE were very similar between datasets.
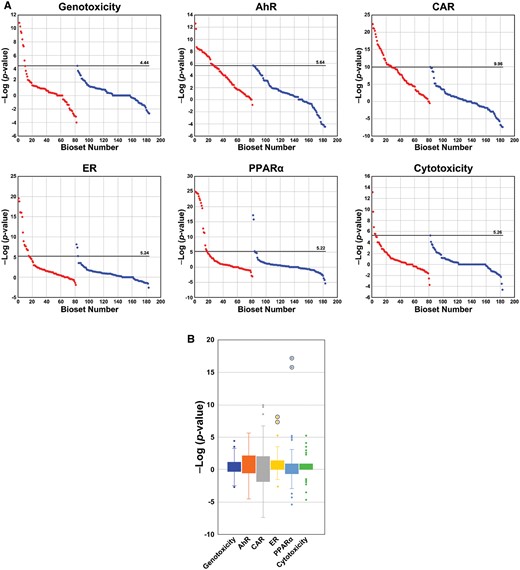
Identification of thresholds for gene expression biomarkers from the DrugMatrix study. A, Distribution of values derived from the DrugMatrix study. Thresholds were determined as detailed in the Materials and Methods section. Each of the biosets was compared with 1 of the 6 biomarkers generating a −Log(p value) of the correlation. The −Log(p value)s were rank ordered and divided into liver tumorigenic and nontumorigenic groups. Horizontal lines represent the derived thresholds. B, Box and whisker plots of −Log(p value)s from the nontumorigenic conditions. The 4 values deemed outliers are circled and were not used to determine the thresholds. Abbreviations: AhR, aryl hydrocarbon receptor; CAR, constitutive activated receptor; ER, estrogen receptor; PPARα, peroxisome proliferator-activated receptor α.
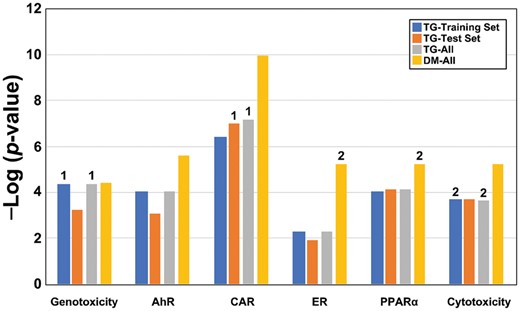
Comparison of the derived biomarker thresholds. The threshold values for the 6 biomarkers derived using 4 datasets are shown. All thresholds were derived using the highest value in the nontumorigenic group. Numbers above the bars indicate the number of outliers removed from the derivation of the thresholds. Abbreviations: AhR, aryl hydrocarbon receptor; CAR, constitutive activated receptor; ER, estrogen receptor; PPARα, peroxisome proliferator-activated receptor α.
Given that thresholds for both TG-GATES and DrugMatrix were derived from combined data across multiple doses and exposure durations, we performed additional analyses to determine if duration of exposure affected the derived MIE threshold values. We subdivided the TG-GATES tumorigen and nontumorigen biosets by exposure duration and examined the MIE thresholds and the distribution pattern of bioset −Log(p value)s from 4 to 29 days (Supplementary Figure 2). For the most part, the top −Log(p value)s in the nontumorigenic groups were similar across time, indicating no major changes with increasing number of chemical administrations. The DrugMatrix study was also examined comparing responses between 3- and 5-day exposures (Supplementary Figure 4), and again it did not appear that there were major differences in top −Log(p value)s in the nontumorigenic groups between the 2 time points.
The Biomarker Thresholds Can Identify Liver Tumorigens
The ability of the derived thresholds to identify liver tumorigens was determined using the 3 different training and test sets within and between the TG-GATES and DrugMatrix chemical libraries. These analyses were carried out both on an individual chem-dose-time bioset level (Supplementary Table 1) and by individual chemicals in which multiple dose-time values for each chemical were evaluated as an aggregate (Table 1). In the first analysis, the set of 6 thresholds derived from the TG-GATES training set was used to predict tumorigenesis in the TG-GATES test set. The heatmap in Figure 5A shows the relationships between time and biosets which exceeded the MIE thresholds derived from TG-GATES training set applied to the entire the TG-GATES study. There were differences in bioset frequencies between and within each MIE biomarker over time. The ER and cytotoxicity biomarkers had the lowest bioset frequency above their thresholds than the other biomarkers and may be due to lower numbers of chemicals that activated these pathways. AhR, CAR, and cytotoxicity demonstrated a consistent increase in number of biosets above their thresholds over time. The thresholds were able to identify all tumorigenic chemicals resulting in a balanced accuracy of 97% compared with a balanced accuracy of 93% when the training set was used to predict training set chemicals (Table 1).
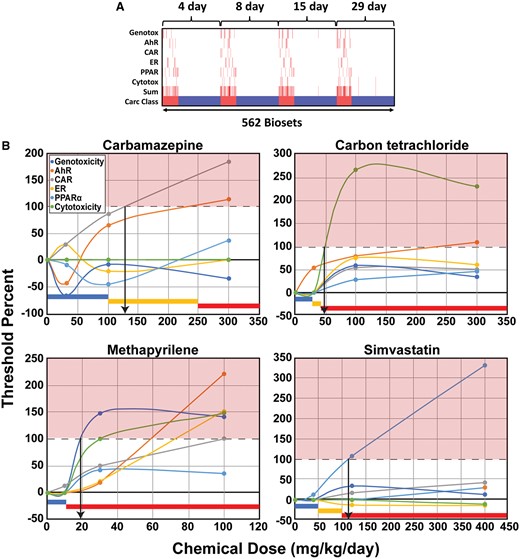
Relationships between time of exposure and thresholds for the 6 biomarkers. A, The heatmap shows the chem-dose-time exposures in which the biomarker −Log(p value)s surpassed the thresholds derived from TG-GATES training set across time of exposure in the entire TG-GATES study. The sum represents the sum of the number of thresholds exceeded. Carc Class: tumorigenic, red; nontumorigenic, blue. B, Relationships between dose, biomarker threshold levels, and liver tumorigenicity. Dose-dependent changes in the −Log(p value)s of each MIE relative to the derived threshold from the TG-GATES training set are shown. The threshold for each MIE was set at 100%. Biomarker changes at the 15-day time point were examined in these examples. The color bars at the bottom represent dose ranges from 2-year bioassays in male rats that for liver tumors were negative (blue), were positive (red), or were not known (yellow). The downward arrows were drawn from the intersection of the indicated biomarker with the 100% and indicate the lowest dose predicted to be tumorigenic. Abbreviations: AhR, aryl hydrocarbon receptor; CAR, constitutive activated receptor; ER, estrogen receptor; PPARα, peroxisome proliferator-activated receptor α.
Training Set . | Test Set . | Unit . | TP . | TN . | FP . | FN . | Sensitivity . | Specificity . | PPV . | NPV . | Balanced Accuracy . |
---|---|---|---|---|---|---|---|---|---|---|---|
TG-Training | TG-Test | Chemical | 9 | 27 | 2 | 0 | 1.000 | 0.931 | 0.818 | 1.000 | 0.966 |
TG-All | DM-All | Chemical | 33 | 23 | 20 | 1 | 0.971 | 0.535 | 0.623 | 0.958 | 0.753 |
DM-All | TG-All | Chemical | 17 | 55 | 2 | 1 | 0.944 | 0.965 | 0.895 | 0.982 | 0.955 |
DM-All | TG-4d | Chemical | 17 | 57 | 0 | 1 | 0.944 | 1.000 | 1.000 | 0.983 | 0.972 |
DM-All | TG-8d | Chemical | 17 | 57 | 0 | 1 | 0.944 | 1.000 | 1.000 | 0.983 | 0.972 |
DM-All | TG-15d | Chemical | 17 | 56 | 1 | 1 | 0.944 | 0.982 | 0.944 | 0.982 | 0.963 |
DM-All | TG-29d | Chemical | 17 | 55 | 2 | 1 | 0.944 | 0.965 | 0.895 | 0.982 | 0.955 |
Training Set . | Test Set . | Unit . | TP . | TN . | FP . | FN . | Sensitivity . | Specificity . | PPV . | NPV . | Balanced Accuracy . |
---|---|---|---|---|---|---|---|---|---|---|---|
TG-Training | TG-Test | Chemical | 9 | 27 | 2 | 0 | 1.000 | 0.931 | 0.818 | 1.000 | 0.966 |
TG-All | DM-All | Chemical | 33 | 23 | 20 | 1 | 0.971 | 0.535 | 0.623 | 0.958 | 0.753 |
DM-All | TG-All | Chemical | 17 | 55 | 2 | 1 | 0.944 | 0.965 | 0.895 | 0.982 | 0.955 |
DM-All | TG-4d | Chemical | 17 | 57 | 0 | 1 | 0.944 | 1.000 | 1.000 | 0.983 | 0.972 |
DM-All | TG-8d | Chemical | 17 | 57 | 0 | 1 | 0.944 | 1.000 | 1.000 | 0.983 | 0.972 |
DM-All | TG-15d | Chemical | 17 | 56 | 1 | 1 | 0.944 | 0.982 | 0.944 | 0.982 | 0.963 |
DM-All | TG-29d | Chemical | 17 | 55 | 2 | 1 | 0.944 | 0.965 | 0.895 | 0.982 | 0.955 |
Abbreviations: DM, DrugMatrix; FN, false negative; FP, false positive; NPV, negative predictive value; PPV, positive predictive value; TG, TG-GATES; TN, true negative; TP, true positive.
Training Set . | Test Set . | Unit . | TP . | TN . | FP . | FN . | Sensitivity . | Specificity . | PPV . | NPV . | Balanced Accuracy . |
---|---|---|---|---|---|---|---|---|---|---|---|
TG-Training | TG-Test | Chemical | 9 | 27 | 2 | 0 | 1.000 | 0.931 | 0.818 | 1.000 | 0.966 |
TG-All | DM-All | Chemical | 33 | 23 | 20 | 1 | 0.971 | 0.535 | 0.623 | 0.958 | 0.753 |
DM-All | TG-All | Chemical | 17 | 55 | 2 | 1 | 0.944 | 0.965 | 0.895 | 0.982 | 0.955 |
DM-All | TG-4d | Chemical | 17 | 57 | 0 | 1 | 0.944 | 1.000 | 1.000 | 0.983 | 0.972 |
DM-All | TG-8d | Chemical | 17 | 57 | 0 | 1 | 0.944 | 1.000 | 1.000 | 0.983 | 0.972 |
DM-All | TG-15d | Chemical | 17 | 56 | 1 | 1 | 0.944 | 0.982 | 0.944 | 0.982 | 0.963 |
DM-All | TG-29d | Chemical | 17 | 55 | 2 | 1 | 0.944 | 0.965 | 0.895 | 0.982 | 0.955 |
Training Set . | Test Set . | Unit . | TP . | TN . | FP . | FN . | Sensitivity . | Specificity . | PPV . | NPV . | Balanced Accuracy . |
---|---|---|---|---|---|---|---|---|---|---|---|
TG-Training | TG-Test | Chemical | 9 | 27 | 2 | 0 | 1.000 | 0.931 | 0.818 | 1.000 | 0.966 |
TG-All | DM-All | Chemical | 33 | 23 | 20 | 1 | 0.971 | 0.535 | 0.623 | 0.958 | 0.753 |
DM-All | TG-All | Chemical | 17 | 55 | 2 | 1 | 0.944 | 0.965 | 0.895 | 0.982 | 0.955 |
DM-All | TG-4d | Chemical | 17 | 57 | 0 | 1 | 0.944 | 1.000 | 1.000 | 0.983 | 0.972 |
DM-All | TG-8d | Chemical | 17 | 57 | 0 | 1 | 0.944 | 1.000 | 1.000 | 0.983 | 0.972 |
DM-All | TG-15d | Chemical | 17 | 56 | 1 | 1 | 0.944 | 0.982 | 0.944 | 0.982 | 0.963 |
DM-All | TG-29d | Chemical | 17 | 55 | 2 | 1 | 0.944 | 0.965 | 0.895 | 0.982 | 0.955 |
Abbreviations: DM, DrugMatrix; FN, false negative; FP, false positive; NPV, negative predictive value; PPV, positive predictive value; TG, TG-GATES; TN, true negative; TP, true positive.
When thresholds derived from the entire TG-GATES dataset were used to predict the DrugMatrix chemicals (second analysis), only 1 tumorigenic chemical was missed, however a large number of nontumorigenic chemicals (20) were misclassified reducing the balanced accuracy to 75%. These results suggest that the thresholds derived from the TG-GATES dataset were too low to adequately identify TPs without increasing the number of FPs. In the third approach, thresholds derived from the DrugMatrix study predicted tumorigenic chemicals in the TG-GATES dataset with a balanced accuracy of 96%. When the DrugMatrix thresholds were used to determine predictive accuracy in the TG-GATES dataset at different treatment times, all treatment times performed similarly (approximately 96%–97%). Predictions based on bioset behavior are found in Supplementary Table 1. For the most part, these accuracies were usually less (average of approximately 4% less) than those based on chemicals. Overall, the biomarker thresholds derived from the DrugMatrix study were excellent at identifying liver tumorigens in the TG-GATES study.
We examined 4 chemicals in greater detail to demonstrate how the biomarker thresholds could be used in practice to identify doses that would be tumorigenic in short-term studies. Four chemicals were selected from the TG-GATES database as they were examined at both nontumorigenic and tumorigenic doses. Figure 5B shows the dose-dependent changes in the −Log(p value)s of each MIE relative to the derived threshold from the TG-GATES training set. Thus, the threshold for each MIE was set at 100%. Biomarker changes at the 15-day time point were examined in these examples.
Carbamazepine was examined at 30, 100, and 300 mg/kg. The thresholds for CAR and AhR were exceeded at approximately 125 and 240 mg/kg, respectively. The dose of 300 mg/kg but not 30 or 100 would be predicted to be tumorigenic. Carbamazepine was administered to Sprague Dawley rats for 2 years at 25, 75, and 250 mg/kg/day resulting “in a dose-related increase in the incidence of hepatocellular tumors in females”; no tumor incidence data was available in either sex (https://www.accessdata.fda.gov/drugsatfda_docs/label/2009/016608s101,018281s048lbl.pdf). Due to the uncertainty of tumor induction at the 2 lower doses, the highest dose was set as the lowest tumorigenic dose for our studies. The thresholds correctly predicted that the 300 mg/kg dose would be tumorigenic. The increase in CAR activation is consistent with known effects of carbamazepine on CAR activation (Faucette et al., 2007).
Carbon tetrachloride (CCl4) was examined at 30, 100, and 300 mg/kg. The cytotoxicity threshold was exceeded at approximately 50 mg/kg. The doses of 100 and 300 would be predicted to be tumorigenic. CCl4 was examined at 3 doses in a 2-year bioassay in male Fisher F344 rats (1.64, 8.2, 41 mg/kg) (https://carcdb.lhasalimited.org/carcdb-frontend/). Only the highest dose was clearly associated with increases in hepatocellular adenomas and carcinomas and was set in our studies as the lowest tumorigenic dose. The cytotoxicity threshold correctly predicted that the 120 and 300 mg/kg would be tumorigenic.
Methapyrilene was examined at 10, 30, and 100 mg/kg. The cytotoxicity threshold was exceeded at approximately 19 mg/kg, whereas the genotoxicity threshold was exceeded at approximately 30 mg/kg. The 30 and 100 mg/kg would be predicted to lead to liver tumors. Methapyrilene was examined at 2 doses in male F344 rats (4.14, 8.27 mg/kg) for 2 years (https://carcdb.lhasalimited.org/carcdb-frontend/). Because of the uncertain nature of the potential hepatocellular adenomas termed “neoplastic nodules,” we chose the tumorigenic dose in 50% of the animals (TD50) = 77 mg/kg from the original Gold database derived from hepatocellular carcinoma incidence as the lowest tumorigenic dose. The thresholds correctly predicted that the 100 mg/kg dose was tumorigenic. The 2 thresholds also predicted that the 30 mg/kg would be tumorigenic and this likely reflects the fact that there were increases in “neoplastic nodules” at the highest dose tested in the bioassay. In retrospect, we should have used the Gold TD50 for neoplastic nodules (likely hepatocellular adenomas) of 11.1 mg/kg. The thresholds captured doses that induced these nodules.
Simvastatin was examined at 40, 120, and 400 mg/kg. The PPARα biomarker exceeded its threshold at approximately 115 mg/kg predicting that the 120 and 400 doses were tumorigenic. Simvastatin was examined in a rat carcinogenicity study at 50 and 100 mg/kg and the 2 doses produced hepatocellular adenomas and carcinomas in females at both doses and in males only at 100 mg/kg; no tumor incidence data was available (https://www.accessdata.fda.gov/drugsatfda_docs/label/2015/019766s093lbl.pdf). Thus, we set the lowest tumorigenic dose at 100 mg/kg. The PPAR threshold correctly predicted that the 120 and 400 mg/kg doses would be tumorigenic.
These 4 examples demonstrated that the 6 MIE thresholds were useful to identify dose ranges of chemicals that would be tumorigenic in the liver in 2-year bioassays.
Individual Biomarker Genes Exhibit Thresholds for Liver Tumorigenesis
Given that the thresholds based on sets of biomarker genes were predictive of liver tumorigenesis, we reasoned that a small subset of the genes could also be used to make accurate predictions. Two genes were selected from each of the biomarker gene lists focusing on genes which had the highest fold-change values in the biomarkers. Using the same set of training and test sets described above, thresholds were derived and used in testing. The range of fold-change values for the 12 genes in the tumorigenic and nontumorigenic groups for the TG-GATES training and test sets are shown in Figure 6A. Overall, there were more biosets which exhibited significant changes in the tumorigenic groups than the nontumorigenic groups which would be expected for genes involved in or associated with liver carcinogenesis. Ugt2b1 was the only gene which exhibited decreases in expression in a subset of treatment conditions found only in tumorigenic treatment conditions which were made up of medium or high doses of WY-14 643 or fenofibrate, 2 PPARα agonists.
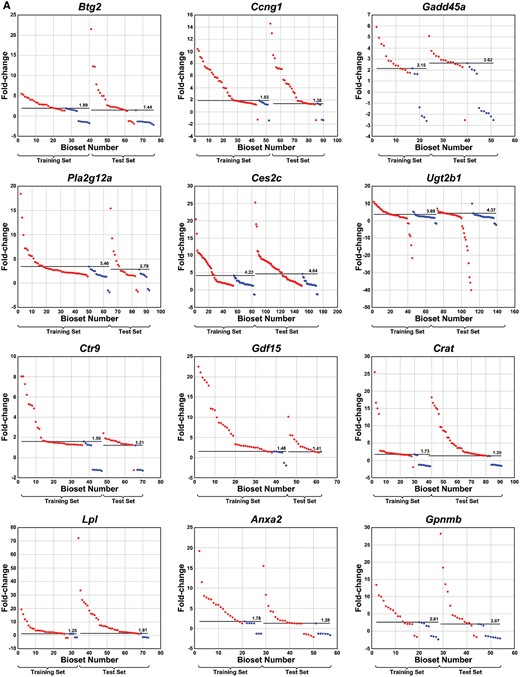
Identification of thresholds for genes in biomarkers from the TG-GATES study. A, Distribution of values across training and test sets derived from the TG-GATES study. Thresholds were determined as detailed in the Materials and Methods section. Each of the biosets was rank ordered by fold-change and divided into liver tumorigenic and nontumorigenic groups and training and test sets. Horizontal lines represent the derived thresholds. The bioset number refers to the rank of the bioset organized by fold-change. B, Box and whisker plots of fold-change values from the nontumorigenic conditions. Left, values from the TG-GATES training set. Right, values from the TG-GATES test set. The 2 values deemed outliers in the training set are circled and were not used to determine the thresholds. C, Behavior of housekeeping genes. There were 4 housekeeping genes examined. The graphs show that these genes do not appreciably exhibit thresholds for tumorigenicity.
Gene expression thresholds were identified in a manner similar to that described above. For the TG-GATES training set, 2 outliers were removed in the analysis of Ugt2b1 (Figure 6B). The resulting thresholds ranged from 1.25-fold for Lpl to 4.22-fold for Ces2c. In the TG-GATES test set, 1 outlier for Ugt2b1 was removed. The resulting thresholds were similar between the training and test sets (Figure 6A). Four housekeeping genes were also examined. In contrast to the 12 biomarker genes, thresholds were not readily apparent, in part due to the lack of statistically significant changes in the nontumorigenic group (Figure 6C).
The threshold analysis was repeated using the DrugMatrix microarray dataset. The range of fold-changes in the tumorigenic and nontumorigenic groups is shown in Figure 7A. Thresholds were identified as described above after removing 1 outlier each for Btg2 and Gdf15 genes (Figure 7B). As in the analysis of the TG-GATES dataset, there were no obvious thresholds for the 4 housekeeping genes (Figure 7C).

Identification of thresholds for genes in biomarkers from the DrugMatrix study. A, Distribution of values across the DrugMatrix study. Thresholds were determined as detailed in the Materials and Methods section. Each of the biosets was rank ordered by fold-change and divided into liver tumorigenic and nontumorigenic groups. Horizontal lines represent the derived thresholds. B, Box and whisker plots of fold-change values from the nontumorigenic conditions. There were 2 outliers, 1 each for Btg2 and Gdf15 (not shown). C, Behavior of housekeeping genes. There were 4 housekeeping genes examined. The graphs show that these genes do not appreciably exhibit thresholds for tumorigenicity.
A comparison of the thresholds across the different datasets is shown in Figure 8. In general, the threshold values were similar across the TG-GATES chemicals. For 8 of the genes (Btg2, Ccng1, Gadd45a, Pla2g12a, Ctr9, Gdf15, Crat, Anxa2), the thresholds were higher when derived from the DrugMatrix study. To determine whether the values derived from the DrugMatrix study were higher because of the analysis methods, we rederived the fold-change and thresholds for the TG-GATES study using an independent method (see Materials and Methods section). Using this method (Method 2), the thresholds were consistently less than the fold-change values from BSCE for all genes but in most cases were closer to those derived by the original method (Method 1) than to those derived from the DrugMatrix dataset.
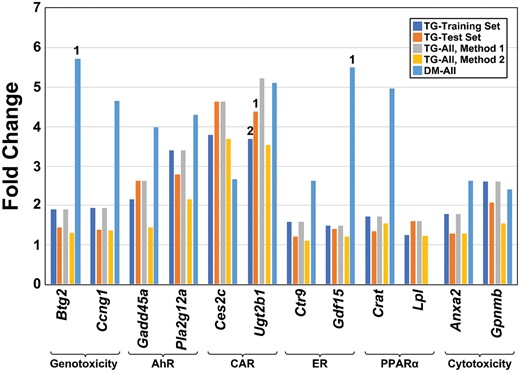
Thresholds for 12 biomarker genes. The threshold values for the 12 biomarker genes derived from 4 datasets are shown. All thresholds were derived using the highest value in the nontumorigenic group with outliers removed. All fold-change values were derived from analysis in BaseSpace Correlation Engine (Method 1) except the Method 2 group, which derived the fold-changes using the R procedures as described in the Materials and Methods section. Numbers above the bars indicate the number of outliers removed from the derivation of the thresholds. Abbreviations: AhR, aryl hydrocarbon receptor; CAR, constitutive activated receptor; ER, estrogen receptor; PPARα, peroxisome proliferator-activated receptor α.
We next determined if there were any differences in the thresholds in the genes across time of treatment. The pattern of gene fold-change values from 4 to 29 days in the TG-GATES study are shown in Supplementary Figure 5. For the most part, the top fold-change values in the nontumorigenic groups were similar across time, indicating no major changes with increasing number of administrations. For some genes, there were increases in the maximum fold-change level with time in the tumorigenic groups (Pla2g12a, Ugt2b1, Crat, Anxa2). The DrugMatrix study was also examined across time (Supplementary Figure 6). For most of the genes, there were no major differences in top fold-change values in the nontumorigenic groups between the 3- and 5-day time points. However for Btg2, Ccng1, and Gdf15, the thresholds at 3 days appeared to be higher than those at 5 days. No threshold could be derived for Lpl as there were no positive fold-change values in the nontumorigenic groups.
Thresholds Derived From a Small Set of Genes Are Predictive of Liver Tumorigenesis
The ability of the 12 derived gene expression thresholds for identifying liver tumorigens was tested using independent training and test sets of chemicals as described above. Figure 9 shows the relationships between time and threshold for the 12 genes across the different time points in the TG-GATES study. Thresholds were derived from the TG-GATES training set and applied to the entire TG-GATES dataset. The genes exhibited differences in the number of times they reached a threshold. For example, Btg2, Gadd45, and Ctr9 rarely exceeded their thresholds whereas other genes such as Ces2c and Lpl frequently exceeded their thresholds. There were also differences in the number of times a gene reached its threshold over the time course of the study. Genes such as Lpl and Anxa2 reached thresholds more frequently at later time points. In many incidences, pairs of genes derived from the same biomarker reached their thresholds in the same chem-dose-time treatment groups especially visible for ER and PPARα genes. With increased time of exposure, the number of FNs decreases (those biosets that correspond with the tumorigenic class) and FPs increases (those that correspond with the nontumorigenic class), similar to the analysis with the biomarkers.
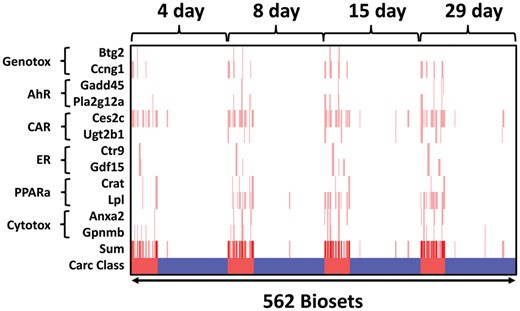
Relationships between time of exposure and thresholds for 12 biomarker genes. The heatmap shows the 562 chemical-dose-time exposures in which the gene fold-changes surpassed the thresholds derived from the TG-GATES training set (red vertical lines) across time of exposure in the entire TG-GATES study. The sum represents the sum of thresholds exceeded. Carc Class: tumorigenic, red; nontumorigenic, blue. Abbreviations: AhR, aryl hydrocarbon receptor; CAR, constitutive activated receptor; ER, estrogen receptor; PPARα, peroxisome proliferator-activated receptor α.
Thresholds derived from the TG-GATES training set were able to identify all positive chemicals in the TG-GATES test set resulting in a balanced accuracy of 90% compared with a balanced accuracy of 97% when the training set was used to predict the training set chemicals (Table 2). When thresholds derived from the entire TG-GATES dataset were used to predict tumorigenic chemicals in the DrugMatrix study, there was only 1 FN. However, the model identified 26 FP chemicals resulting in a balanced accuracy of 68%. Thresholds derived from the DrugMatrix study were excellent at predicting tumorigenic chemicals in the TG-GATES dataset (92% balanced accuracy overall). When broken out by time of exposure, the time points of 8 and 29 days (94%) slightly outperformed the 4- and 15-day time points (91% and 92%, respectively). The 4-day test resulted in the greatest number of FNs (3) indicating that longer exposure times are necessary for chemicals to reach stable responses. Predictions based on biosets are found in Supplementary Table 2. The accuracies were an average of approximately 4% less than those based on chemicals. Overall, the gene expression thresholds were excellent at identifying liver tumorigens.
Training Set . | Test Set . | Unit . | TP . | TN . | FP . | FN . | Sensitivity . | Specificity . | PPV . | NPV . | Balanced Accuracy . |
---|---|---|---|---|---|---|---|---|---|---|---|
TG-Training | TG-Test | Chemical | 9 | 23 | 6 | 0 | 1.000 | 0.793 | 0.600 | 1.000 | 0.897 |
TG-All | DM-All | Chemical | 37 | 16 | 26 | 1 | 0.974 | 0.381 | 0.587 | 0.941 | 0.677 |
DM-All | TG-All | Chemical | 17 | 56 | 6 | 1 | 0.944 | 0.903 | 0.739 | 0.982 | 0.924 |
DM-All | TG-4d | Chemical | 15 | 61 | 1 | 3 | 0.833 | 0.984 | 0.938 | 0.953 | 0.909 |
DM-All | TG-8d | Chemical | 16 | 61 | 1 | 2 | 0.889 | 0.984 | 0.941 | 0.968 | 0.936 |
DM-All | TG-15d | Chemical | 16 | 59 | 3 | 2 | 0.889 | 0.952 | 0.842 | 0.967 | 0.920 |
DM-All | TG-29d | Chemical | 17 | 58 | 4 | 1 | 0.944 | 0.935 | 0.810 | 0.983 | 0.940 |
Training Set . | Test Set . | Unit . | TP . | TN . | FP . | FN . | Sensitivity . | Specificity . | PPV . | NPV . | Balanced Accuracy . |
---|---|---|---|---|---|---|---|---|---|---|---|
TG-Training | TG-Test | Chemical | 9 | 23 | 6 | 0 | 1.000 | 0.793 | 0.600 | 1.000 | 0.897 |
TG-All | DM-All | Chemical | 37 | 16 | 26 | 1 | 0.974 | 0.381 | 0.587 | 0.941 | 0.677 |
DM-All | TG-All | Chemical | 17 | 56 | 6 | 1 | 0.944 | 0.903 | 0.739 | 0.982 | 0.924 |
DM-All | TG-4d | Chemical | 15 | 61 | 1 | 3 | 0.833 | 0.984 | 0.938 | 0.953 | 0.909 |
DM-All | TG-8d | Chemical | 16 | 61 | 1 | 2 | 0.889 | 0.984 | 0.941 | 0.968 | 0.936 |
DM-All | TG-15d | Chemical | 16 | 59 | 3 | 2 | 0.889 | 0.952 | 0.842 | 0.967 | 0.920 |
DM-All | TG-29d | Chemical | 17 | 58 | 4 | 1 | 0.944 | 0.935 | 0.810 | 0.983 | 0.940 |
Abbreviations: DM, DrugMatrix; FN, false negative; FP, false positive; NPV, negative predictive value; PPV, positive predictive value; TG, TG-GATES; TN, true negative; TP, true positive.
Training Set . | Test Set . | Unit . | TP . | TN . | FP . | FN . | Sensitivity . | Specificity . | PPV . | NPV . | Balanced Accuracy . |
---|---|---|---|---|---|---|---|---|---|---|---|
TG-Training | TG-Test | Chemical | 9 | 23 | 6 | 0 | 1.000 | 0.793 | 0.600 | 1.000 | 0.897 |
TG-All | DM-All | Chemical | 37 | 16 | 26 | 1 | 0.974 | 0.381 | 0.587 | 0.941 | 0.677 |
DM-All | TG-All | Chemical | 17 | 56 | 6 | 1 | 0.944 | 0.903 | 0.739 | 0.982 | 0.924 |
DM-All | TG-4d | Chemical | 15 | 61 | 1 | 3 | 0.833 | 0.984 | 0.938 | 0.953 | 0.909 |
DM-All | TG-8d | Chemical | 16 | 61 | 1 | 2 | 0.889 | 0.984 | 0.941 | 0.968 | 0.936 |
DM-All | TG-15d | Chemical | 16 | 59 | 3 | 2 | 0.889 | 0.952 | 0.842 | 0.967 | 0.920 |
DM-All | TG-29d | Chemical | 17 | 58 | 4 | 1 | 0.944 | 0.935 | 0.810 | 0.983 | 0.940 |
Training Set . | Test Set . | Unit . | TP . | TN . | FP . | FN . | Sensitivity . | Specificity . | PPV . | NPV . | Balanced Accuracy . |
---|---|---|---|---|---|---|---|---|---|---|---|
TG-Training | TG-Test | Chemical | 9 | 23 | 6 | 0 | 1.000 | 0.793 | 0.600 | 1.000 | 0.897 |
TG-All | DM-All | Chemical | 37 | 16 | 26 | 1 | 0.974 | 0.381 | 0.587 | 0.941 | 0.677 |
DM-All | TG-All | Chemical | 17 | 56 | 6 | 1 | 0.944 | 0.903 | 0.739 | 0.982 | 0.924 |
DM-All | TG-4d | Chemical | 15 | 61 | 1 | 3 | 0.833 | 0.984 | 0.938 | 0.953 | 0.909 |
DM-All | TG-8d | Chemical | 16 | 61 | 1 | 2 | 0.889 | 0.984 | 0.941 | 0.968 | 0.936 |
DM-All | TG-15d | Chemical | 16 | 59 | 3 | 2 | 0.889 | 0.952 | 0.842 | 0.967 | 0.920 |
DM-All | TG-29d | Chemical | 17 | 58 | 4 | 1 | 0.944 | 0.935 | 0.810 | 0.983 | 0.940 |
Abbreviations: DM, DrugMatrix; FN, false negative; FP, false positive; NPV, negative predictive value; PPV, positive predictive value; TG, TG-GATES; TN, true negative; TP, true positive.
DISCUSSION
Benchmark responses relate the amount of disruption required for an early event to initiate a downstream adverse outcome. Once established across data-rich chemical inventories, short-term thresholds can be used to derive protective dose limits in the absence of longer-term studies. Previously, we built a set of gene expression biomarkers representing the 6 most common pathways in rodent liver tumorigenesis. Using this biomarker set, liver tumorigens were predicted with high accuracy (96%), missing only 2 out of the 38 liver tumorigens in our microarray compendium (Corton et al., 2020). In this study, we identified thresholds for these biomarkers based on short-term gene expression profiles. Thresholds were similar across different sets of nontumorigenic chemicals within the TG-GATES dataset, whereas thresholds derived from the DrugMatrix dataset generated on another type of microarray with another set of chemicals were for 5 of the 6 biomarkers somewhat higher. The 6 threshold estimates were used to identify tumorigens in independent test sets of chemicals. Thresholds derived from the TG-GATES study training set were accurate at predicting tumorigens in the TG-GATES test set (up to 93%). These same thresholds when applied to the DrugMatrix study had a high rate of TPs, and also a high FP rate. In contrast, the thresholds derived from the DrugMatrix study were very predictive when used to predict tumorigens in the TG-GATES study (up to 97%). With these issues in mind, it should be pointed out that although the thresholds derived from the DM and TG-GATES databases were not identical, they had comparable predictive capacity across their disparate chemical inventories with very low FN rates—a highly desirable characteristic for regulatory determination of hazard. We also addressed the question of whether smaller sets of genes could be used in place of the full complement of biomarker genes. In an examination of 12 genes (2/biomarker), we found that all of the genes exhibited individual thresholds that were similar across training and test sets of chemicals in the TG-GATES dataset. In most cases, individual gene thresholds were lower (less conservative) than those derived from the DrugMatrix dataset. The 12 thresholds when used collectively resulted in high predictive accuracies (up to 94%). Given these high predictive accuracies, our work supports the use of the 6 biomarkers and their thresholds in interpreting gene expression profiles from short-term rat studies. Redefining hazard using a threshold approach would help to move toxicological testing from the traditional notion of an adverse dose level based on morphologic effects to a protective dose level based on molecular effects.
To our knowledge, our study is the first example of the identification of chemical-independent toxicological thresholds based on multiple chemicals linked to tumorigenicity. The concept of the toxicological threshold as it pertains to KEs in AOPs has been described many years ago (Julien et al., 2009). The concept of a toxicological threshold that we use here and that is determined experimentally is different from other threshold concepts often used in risk characterization. Both the “threshold of toxicological concern” (Hennes, 2012) and BMD define doses of chemicals that have very low probabilities of an appreciable risk to human health. This is in contrast to toxicological thresholds identified in our study that define the boundary between no toxicity and toxicity and in which there are likely increasing probabilities of risk the closer the response is to the threshold. The toxicological threshold concept used here is similar to “toxicological tipping points” defined in 2 in vitro studies as dose-dependent transitions characterized in cells as the inability to recover normal (or basal) functions (Shah et al., 2016). These tipping points in HepG2 cells and more recently in primary rat cortical cultures (Frank et al., 2018) were identified by monitoring cellular effects over a time course of treatment as those chemical concentrations that do not allow return of the cells to normality. Thus, while the tipping points defined in these in vitro studies are not chemical agnostic as in our study, they do identify exposure scenarios for individual chemicals that roughly define the boundary between 2 biological states, in this case the ability of the cell to adapt to exposure or to proceed on to toxicity. Previous studies have identified effect thresholds for liver cancer (Lake et al., 2016) and cortical heterotopia (Hassan et al., 2017) that were based on studies with 1 chemical each, whereas an effect threshold for hearing loss in the rat due to decreases in circulating T4 levels was based on studies with 5 chemicals (Crofton, 2004).
There were differences in the threshold values derived from the TG-GATES and DrugMatrix datasets. For most of the values, the thresholds for the biomarkers (5 out of 6) and the genes (8 out of 12) were higher when derived from the DrugMatrix dataset. The 2 studies were both carried out with male Sprague Dawley rats in which an overlapping set of chemicals at overlapping doses and times of exposure were administered by gavage. Thus, it is unlikely but cannot be ruled out that the experimental conditions of exposure including the use of different sets of nontumorigenic chemicals led to differences in the threshold values. There is evidence that the length of the probes on the microarray platform may contribute to the differences. Oligonucleotide 30mer probes (found on the Codelink platform) provide twice the intensity of 25mer probes (found on the Affymetrix platform) (Relogio et al., 2002). A comparison between the CodeLink and Affymetrix platforms suggested a 10-fold greater sensitivity of the former platform (Shippy et al., 2004). We also found that the gene thresholds derived using an R pipeline were consistently less than those derived from the fold-change values in BSCE. The R method used MAS5 as the normalization method whereas in BSCE the normalization method was RMA. There are appreciable effects of data normalization on the outcome of downstream analysis of microarray data (Mezencev and Subramaniam, 2019). Therefore, the higher thresholds may be due to higher sensitivity on the Codelink array or differences in normalization methods but requires further study. As toxicogenomics investigators are moving away from microarrays toward reliance on global or targeted RNA-Seq techniques, the question arises as to whether the thresholds generated on microarray platforms will have to be rederived. Thresholds for the fold-change values will no doubt need to be rederived due to the fact that RNA-Seq technologies like RT-qPCR exhibit greater dynamic range and fold-changes than those derived from microarrays. The biomarker correlations though may not be as sensitive to the newer technologies, as the thresholds are based on the Running Fisher test correlation p value. Only rigorous testing with a new (targeted) RNA-Seq dataset will allow us to determine whether the thresholds need to be rederived. The results from our study indicate that the threshold approach to predicting cancer will be most accurate if new chemicals are examined on the same platform from which the thresholds were derived.
The thresholds for tumorigenesis derived in our study were similar to the values that we have previously used as cutoffs for significant MIE activation. Our group routinely uses the Running Fisher test −Log(p value)s from comparisons between derived gene expression biomarkers and statistically filtered gene sets from a variety of species and tissue types. As described in the Materials and Methods section, we use a −Log(p value) cutoff of absolute value = 4 to determine whether a chemical is activating or suppressing a MIE. The use of this cutoff consistently results in high predictive accuracy (approximately 91%–98%) independent of the in vivo/in vitro context and the tissue or species the biomarker and the test set were derived from Corton et al. (2019). In this study, it was notable that the thresholds derived from the TG-GATES study identified for genotoxicity, AhR, PPARα, and cytotoxicity were close to −Log(p value) = 4, whereas the thresholds for CAR and ER were 2–3 units above or approximately 1.5 units below this value, respectively. Our previous studies show that the −Log(p value) is dose-dependent, increasing with increasing dose in parallel with increasing visual similarity between the gene set and the biomarker genes in both number of overlapping genes and the strength of the fold-change (Oshida et al., 2015). The value of the thresholds which is the minimum that leads to liver tumors indicates that the cutoff of 4 may represent more moderate activation versus marginal or weak activation assumed in our previous studies. The fact that the CAR threshold is higher than a −Log(p value) = 4 indicates that higher CAR activation is required to cause liver tumors.
Given the success of the biomarker threshold approach, we reasoned that thresholds derived from a minimal number of biomarker genes would be sufficient to identify tumorigenic chemicals. The predictive accuracies for the group of genes that we chose, like those for the biomarker thresholds was overall very high but dependent on the dataset from which they were derived from and which test set they were used on. Like the biomarker thresholds, highest predictive accuracies occurred when the TG-GATES training set thresholds were used to predict the TG-GATES test set or when the DrugMatrix thresholds were used to predict tumorigens in the TG-GATES dataset. The fact that the accuracies for predicting liver cancer were similar to those of the full biomarkers indicates that only small subsets of genes may need to be examined for accurate prediction of cancer. Given our results looking at only 12 genes, we suspect that many genes that fall into different functional categories will be found to exhibit thresholds for cancer. A full-genome evaluation to identify thresholds for every gene expressed in the liver could help to further define KEs in carcinogenesis in parallel with or act downstream of the MIEs. Examples might include those under control of Nrf2 as indirect indicators of oxidative stress and cell cycle genes linked to transient or sustained increases in cell proliferation. This more systematic approach coupled with machine learning methods would potentially allow a selection of the most predictive genes that could be used in more medium-throughput methods that takes into consideration all well-characterized KEs in liver cancer AOPs. This strategy could also be implemented in tissues in which chemical-induced tumor outcome is known but in which the underlying AOPs are unknown or incompletely characterized.
While our study was being written, a paper describing a screening approach that incorporates “thresholds” for AhR activators was published (Qin et al., 2019). The group measured expression changes of 2 well-known AhR target genes that are also part of the AhR biomarker used in our study (Cyp1a1, Cyp1a2). Expression differences in the genes across chemicals were used to differentiate between AhR-activating tumorigenic chemicals that exhibit high-level sustained induction (eg, 2,3,7,8-tetrachlorodibenzo-p-dioxin) and AhR-activating nontumorigenic chemicals that exhibit more modest transient induction (eg, omeprazole). The methods used to derive the thresholds were different than those used here and were identified using a supervised assessment of AhR scores that incorporated different weights of induction of both genes. The scores included those of “higher concern” (> 400-fold or > 5-fold induction of Cyp1a1 or Cyp1a2, respectively) or “lower concern” (<100-fold or < 3-fold induction of Cyp1a1 or Cyp1a2, respectively) which assisted in differentiating tumorigenic and nontumorigenic AhR activators. The thresholds were not used to make a formal assessment of predictive accuracy but were intended to help in a weight of evidence assessment of whether a drug candidate could cause toxic sustained high level induction of AhR. This study supports our findings that genes in the rat liver associated with liver MIEs exhibit thresholds for cancer.
The 6 biomarkers and their thresholds could be applied in a number of ways to toxicological testing of industrial chemicals now and in the near future. After preliminary short-term exposure studies followed by gene expression analysis, the toxicological thresholds could be used to help bracket the range of doses between the BMD and the calculated dose that crosses the threshold that would be expected to induce liver tumors. Knowledge of the thresholds could be used to allow informed decons to be made of doses to use in chronic studies to avoid tumor induction. In testing for pharmaceutical candidates, the thresholds could be used to support reduced carcinogenicity testing under the International Council for Harmonization of Technical Requirements for Pharmaceuticals for Human Use (ICH) S1 guidance modification initiatives. Modifications to ICH S1 Carcinogenicity Testing Guidance (ICH, 2015) proposes a more flexible approach to pharmaceutical carcinogenicity testing allowing for adequate assessment of carcinogenic risk without the need for always conducting a 2-year rat carcinogenicity study. This modification in the guidance may enable drug sponsors to gain 2-year rat carcinogenicity study waivers through a Carcinogenicity Assessment Document (CAD)-based justification process. Our study represents an example of how gene expression thresholds could be leveraged as “new biomarkers” data (ICH, 2015) to strengthen CAD-based predictions. For example, if after a 6-month study there are histopathological indicators of liver cancer signals, a short-term toxicogenomic study coupled with our biomarker threshold approach would provide information about the underlying AOP and doses that would lead to liver tumors possibly contributing to the conclusion that a 2-year bioassay is not needed.
Increased confidence in using biomarkers and their thresholds will contribute to the consensus that a 2-year bioassay is no longer the best way to identify chemical carcinogens. Future screening for carcinogens will likely rely on dose-response assessment in short-term exposure studies that identify points of departure (POD) concentrations derived from full-genome analysis of sentinel tissues. In this scenario, the POD analysis can be performed on the collection of genes in each biomarker to identify PODs of human-relevant MIEs. Identification of doses that cross toxicological thresholds would help to define the “safe” dose range. Toxicological thresholds will be useful to evaluate chemical mixtures that affect an overlapping set of liver AOPs. In addition, the toxicological thresholds will be useful in the interpretation of marginal tumorigenic responses which are often seen in tumorigenic studies. These can lead to disagreements across and within agencies that have important regulatory and legal implications. Toxicological thresholds for different AOPs could inform whether these responses are true or false positives. Overall, the use of the biomarkers and their thresholds will increase confidence by both industry and regulators of their value to understand dose-response relationships of tumor induction. The use of the biomarkers and thresholds will contribute to efforts to replace the 2-year bioassay, consistent with EPA’s long-term goal to move toward making Toxic Substances Control Act decisions with new approach methodologies to reduce, refine, or replace vertebrate animal testing (https://www.epa.gov/sites/production/files/2018-06/documents/epa_alt_strat_plan_6-20-18_clean_final.pdf; accessed August 21, 2019) (Thomas et al., 2019).
SUPPLEMENTARY DATA
Supplementary data are available at Toxicological Sciences online.
Disclaimer: The information in this document has been funded in part by the U.S. Environmental Protection Agency. It has been subjected to review by the Center for Computational Toxicology and Exposure and approved for publication. Approval does not signify that the contents reflect the views of the Agency, nor does mention of trade names or commercial products constitute endorsement or recommendation for use.
ACKNOWLEDGMENTS
The authors would like to thank Dr Imran Shah, Dr Roman Mezencev, and Dr Rory Conolly for critical review of the manuscript and Mr Chuck Gaul for assistance in constructing the figures. The research described in this article has been reviewed by the U.S. EPA and approved for publication. Approval does not signify that the contents necessarily reflect the views and the policies of the Agency. Mention of trade names or commercial products does not constitute endorsement or recommendation for use.
FUNDING
U.S. EPA Office of Research and Development; the Oak Ridge Institute for Science Education.
DECLARATION OF CONFLICTING INTERESTS
The authors declared no potential conflicts of interest with respect to the research, authorship, and/or publication of this article.
REFERENCES
ICH. (
NRC. (
OECD. (
USEPA. (
Author notes
Present address: Boehringer Ingelheim Pharmaceuticals, Inc., Ridgefield, CT 06877.
Comments