-
PDF
- Split View
-
Views
-
Cite
Cite
Melissa Morales, Tchilabalo D Alayi, Shefa M Tawalbeh, Agnes V Sydenstricker, Rita Spathis, Hanna Kim, Kanneboyina Nagaraju, Yetrib Hathout, Lisa G Rider, Urine proteomics by mass spectrometry identifies proteins involved in key pathogenic pathways in patients with juvenile dermatomyositis, Rheumatology, Volume 62, Issue 9, September 2023, Pages 3161–3168, https://doi.org/10.1093/rheumatology/kead033
- Share Icon Share
Abstract
To identify and validate biomarkers in JDM patients using a multiplexing tandem mass tag urine proteome profiling approach.
First morning void urine samples were collected from JDM patients (n = 20) and healthy control subjects (n = 21) and processed for analysis using a standardized liquid chromatography-tandem mass spectrometry approach. Biomarkers with significantly altered levels were correlated with clinical measures of myositis disease activity and damage. A subset of candidate biomarkers was validated using commercially available ELISA kits.
In total, 2348 proteins were detected in the samples, with 275 proteins quantified across all samples. Among the differentially altered proteins, cathepsin D and galectin-3 binding protein were significantly increased in the urine of JDM patients (adjusted P < 0.05), supporting previous findings in myositis patients. These two candidate biomarkers were confirmed with ELISAs. Cathepsin D positively correlated with Myositis Damage Index (r = 0.57, P < 0.05) and negatively correlated with the Childhood Myositis Assessment Scale (r = −0.54, P < 0.05). We also identified novel JDM candidate biomarkers involved with key features of myositis, including extracellular matrix remodelling proteins.
This study confirmed the presence of several proteins in the urine of JDM patients that were previously found to be elevated in the blood of myositis patients and identified novel candidate biomarkers that require validation. These results support the use of urine as a source for biomarker development in JDM.
We optimized a urine proteomics workflow that identified proteins associated with key features of JDM.
Cathepsin D and galectin-3 binding protein were increased in the urine of JDM patients.
Cathepsin D correlated with myositis disease activity and damage measures.
Introduction
Myositis is a rare and debilitating autoimmune disease that results in chronic muscle inflammation and weakness. Several subtypes of myositis exist, including forms that manifest in childhood, such as JDM [1, 2]. The clinical features of JDM include proximal weakness and rashes; however, several other features (e.g. calcinosis, gastrointestinal symptoms, lethargy and fever) may be present in JDM, complicating classification criteria [3]. Managing this rare and heterogeneous disease is difficult and there are no specific Food and Drug Administration (FDA)-approved therapies for JDM. The first line of therapy for JDM includes corticosteroids and immunosuppressives, which leads to improvement in many patients [4]. Still, some of the major challenges of managing this disease are variable treatment responses, low remission rates and numerous side effects associated with long-term treatment with CS [5, 6].
There is a clear and urgent need for the development of non-invasive methods for determining prognosis and response to treatment, and identifying targets related to disease pathogenesis. A major advancement in the myositis field has been the identification of myositis-specific autoantibodies (MSAs) [7]. Due to the heterogeneous nature of myositis, MSAs allow for grouping patients by clinical presentation, which has been extremely useful in determining associated clinical features and outcomes. However, MSAs are not present in all patients [1, 8, 9], and are hampered by the lack of reliable testing in the clinical setting [10].
Identifying biomarkers that may then be useful for prognosis and response to treatment are needed for all JDM patients. Unfortunately, due to the rarity and heterogeneous nature of the disease, there are currently only a few sensitive and reliable biomarkers for JDM [11, 12]. JDM pathogenesis is currently monitored by using serum markers and magnetic resonance imaging, which can be psychologically and physically demanding and stressful for young children. Serum levels of creatine kinase (CK) and other muscle enzymes are the markers most closely monitored in JDM patients, but serum CK levels are highly variable and not reliable for diagnosis or during the course of illness [13].
Previous studies have identified urinary metabolites such as creatine, choline, betaine, glycine, trimethylamine oxide, neopterin and quinolinic acid, as being elevated in juvenile myositis patients, with some showing moderate to strong correlations with disease activity [14, 15]. The discovery and validation of biomarkers associated with skeletal muscle pathologies has come to fruition for Duchenne muscular dystrophy [16] and other autoimmune diseases, such as lupus, which have benefitted from identifying urinary biomarkers and utilizing them both for monitoring and predicting treatment response [17]. Such urinary protein biomarkers have yet to be discovered in patients with myositis. Therefore, the discovery and validation of sensitive and specific urinary biomarkers will help fill a critical void in the myositis field. In the current study, we utilized a mass spectrometry, tandem mass tag (TMT) labelling-based proteome profiling approach to identify novel and previously reported JDM biomarkers using first morning void urine samples from a well-characterized cohort of juvenile myositis patients.
Methods
Study participants
Approval from the National Institutes of Health Institutional Review Board was obtained, with informed consent provided by all participants and their parents or their legal guardians. First morning void urine samples were collected from participants and stored at −80°C until use. To minimize variability across multiple runs, TMT experiments included urine samples from both JDM patients and healthy control (HC) subjects of similar sex and ages (within 4 years). All JDM patients (n = 20) were diagnosed with probable or definite JDM based on Bohan and Peter criteria and also met the EULAR-ACR criteria for myositis [3, 18]. HC subjects (n = 21) were free of autoimmune, infectious or other inflammatory diseases. Children with JDM were assessed using standardized disease activity and damage measures within 1–3 days of the urine sample collection [19, 20].
Mass spectrometry and quantification of proteome data
Urine protein concentration was determined using the Pierce BCA Protein Assay Kit (Thermo Fisher Scientific, Rockford, IL, USA) following removal of interfering substances by trichloroacetic acid precipitation (Thermo Fisher Scientific, Rockford, IL, USA). Protein measurements were performed using a SpectraMax i3x microplate reader (Molecular Devices, San Jose, CA, USA). Urine sample aliquots containing 30 µg of total proteins were then prepared and digested prior to labelling. Sample preparation and labelling was performed according to the TMT sixplex Isobaric Mass Label Kit (Thermo Fisher Scientific, Rockford, IL, USA), with some modifications described below (Supplementary Fig. S1A, available at Rheumatology online). Briefly, after urine samples were processed [i.e. protein denaturation with 20 µl of 1% sodium dodecyl sulfate, reduction with 5 µl of 200 mM Tris(2-carboxyethyl) phosphine, and alkylation with 5 µl of 375 mM iodoacetamide], proteins were precipitated with pre-chilled acetone, and each sample was then centrifuged at 15 000 rpm for 20 min at 4°C. This precipitation step was repeated three additional times. Any remaining supernatant was removed by drying the samples on a heat block. The pellets were then reconstituted with 50 mM triethyl ammonium bicarbonate and digested using Pierce Trypsin Protease, MS Grade (Thermo Fisher Scientific, Rockford, IL, USA) at a final enzyme/protein ratio of 1:50 (w/w). Peptide labelling was performed according to the manufacturer’s instructions and the samples combined prior to fractionation. To reduce inter- and intra-experimental variability, combined labelled samples from each experiment contained an equal amount of sample from the same HC subject (TMT 126 labelled tag) which was used as an internal standard for normalization.
To reduce peptide mixture complexity, a fractionation step was performed using a high pH (0.1% triethylamine solution) reversed-phase peptide fractionation kit following the manufacturer's protocol (Thermo Fisher Scientific, Rockford, IL, USA). Collected peptide fractions were reconstituted in 20 µl of water containing 0.1% formic acid (v/v) and 7 µl of samples were injected. Liquid chromatography-tandem mass spectrometry (LC-MS/MS) was performed as previously reported [21]. Briefly, peptide separation was performed using an UltiMate™ 3000 RSLCnano system and LC-MS/MS data-dependent acquisition was performed using a Q-Exactive high-field (HF) mass spectrometer (Thermo Scientific, Bremen, Germany). All data files were collected and processed with a workflow designed in Proteome Discoverer 2.2 (Thermo Scientific). Datasets were searched against the Uniprot Homo sapiens database using Sequest HT with a precursor mass tolerance set at 10 parts-per-million and a mass tolerance at ±0.05 Da. Only unique peptides were used for later quantification and any spectra with missing channels were rejected. Reporter quantitation was corrected with an average signal-to-noise ratio threshold set at 2.0. Protein abundance was calculated by summing the abundance of the corresponding protein for each fraction. Data were normalized to the same control sample (labelled with TMT 126, which was used across all experiments).
ELISA
Cathepsin D and galectin-3 binding protein, identified from the urine proteomics experiments, were selected for validation based on documentation in the myositis literature [22–26] and their involvement in related diseases indicated by the DisGeNET analysis (see Results). A subset of subjects from the MS experiments were used in the validation studies. Using commercially available ELISA kits (Abcam, Boston, MA, USA), samples were diluted 1:10, and assays performed per the manufacturer’s recommendations.
Statistical analysis
Data were normalized to the median and transformed on a log2 scale. The overlaid density plots before and after normalization were visualized for inter-experimental variation (Supplementary Fig. S1B, available at Rheumatology online). Protein quantities were recorded as normalized protein expression. As previously reported [25], a cross-sectional analysis was performed to identify disease-associated proteins significantly different in their levels between JDM patients and HC. Linear Models for MicroArrays and RNA-Seq Data (LIMMA) [27] was used for differential expression analysis comparing the urine proteins between JDM and controls. Multiple comparisons were accounted for by adjusting all P-values using a Benjamini–Hochberg false discovery rate correction. We then studied the relationship of urine protein levels with clinical assessment measures and levels of muscle enzymes. Pearson correlation was performed only on significant proteins from the cross-sectional analysis. Pearson’s χ2 tests were used on categorical data (e.g. gender and race). All statistical analyses were conducted using R [28] and GraphPad Prism version 9 (La Jolla, CA, USA). Significant proteins were entered into STRING, a web database of known and predicted protein networks [29] to determine pathways and functions and into DisGeNet, an open access platform that identifies human disease-gene associations [30].
Results
Patient demographics
General characteristics of the participants are shown in Table 1. There were no significant age or gender differences between JDM and HC subjects (P = 0.59 and P = 0.92, respectively) (Table 1). Pearson’s χ2 test showed that race (Caucasian vs non-Caucasian) differed significantly with disease state (JDM vs HC) (P = 0.01), which was likely driven by the low number of non-Caucasians in the HC group (Table 1). No differences were observed in total urine protein levels between the two groups (P = 0.22). The average physician global disease activity for JDM patients was 3.8 out of 10. The average number of immunosuppressives per JDM patient was 2.3, with most patients (85%) receiving prednisone (average dose 0.60 mg/kg/day), 60% receiving methotrexate and 45% receiving IVIG at time of sample collection.
. | JDM (n = 20) . | HC (n = 21) . | P-value . |
---|---|---|---|
Age at enrolment, years | 9.2 (1.0) | 9.8 (0.6) | 0.59 |
Disease onset age, years | 5.0 | NA | |
Delay in diagnosis, months | 9.5 (4.3) | NA | |
Interval between diagnosis to urine collection, years | 1.20 (0.2) | NA | |
Gender, n | 0.92 | ||
Female | 14 | 15 | |
Male | 6 | 6 | |
Race/Ethnicity, n | 0.01 | ||
Caucasian | 10 | 18 | |
Non-Caucasian | 10 | 3 | |
African-American | 3 | 1 | |
Hispanic | 3 | 2 | |
Asian | 1 | 0 | |
Multi-racial or unknown | 3 | 0 | |
Myositis-specific autoantibody, n | NA | ||
Anti-p155/140 (TIF1) | 12 | ||
Anti-MJ (NXP-2) | 5 | ||
Anti-MDA5 | 3 | ||
MD global disease activity, 0–10 cm VAS | 3.8 (0.39) | NA | |
Medication, n | |||
Prednisone | 17 | 0 | |
Average dose mg/kg/day (SEM) | 0.60 (0.1) | ||
Methotrexate | 12 | 0 | |
IVIG | 9 | 0 | |
Ciclosporin | 3 | 0 | |
Average number of immunosuppressivesa | 2.3 (0.24) | 0 | |
Urine protein level, mg/dl | 262.0 (34.0) | 214.8 (17.9) | 0.22 |
. | JDM (n = 20) . | HC (n = 21) . | P-value . |
---|---|---|---|
Age at enrolment, years | 9.2 (1.0) | 9.8 (0.6) | 0.59 |
Disease onset age, years | 5.0 | NA | |
Delay in diagnosis, months | 9.5 (4.3) | NA | |
Interval between diagnosis to urine collection, years | 1.20 (0.2) | NA | |
Gender, n | 0.92 | ||
Female | 14 | 15 | |
Male | 6 | 6 | |
Race/Ethnicity, n | 0.01 | ||
Caucasian | 10 | 18 | |
Non-Caucasian | 10 | 3 | |
African-American | 3 | 1 | |
Hispanic | 3 | 2 | |
Asian | 1 | 0 | |
Multi-racial or unknown | 3 | 0 | |
Myositis-specific autoantibody, n | NA | ||
Anti-p155/140 (TIF1) | 12 | ||
Anti-MJ (NXP-2) | 5 | ||
Anti-MDA5 | 3 | ||
MD global disease activity, 0–10 cm VAS | 3.8 (0.39) | NA | |
Medication, n | |||
Prednisone | 17 | 0 | |
Average dose mg/kg/day (SEM) | 0.60 (0.1) | ||
Methotrexate | 12 | 0 | |
IVIG | 9 | 0 | |
Ciclosporin | 3 | 0 | |
Average number of immunosuppressivesa | 2.3 (0.24) | 0 | |
Urine protein level, mg/dl | 262.0 (34.0) | 214.8 (17.9) | 0.22 |
Data are shown as mean (SEM), except for gender, race and medication which are shown as N.
Including those listed under medications, as well as tacrolimus (n = 1), infliximab (n = 1), mycophenolate (n = 1).
HC: healthy control; IVIG: immunoglobulin; MD: medical doctor/physician; SEM: standard error of the mean; VAS: visual analogue scale; NA: not applicable.
. | JDM (n = 20) . | HC (n = 21) . | P-value . |
---|---|---|---|
Age at enrolment, years | 9.2 (1.0) | 9.8 (0.6) | 0.59 |
Disease onset age, years | 5.0 | NA | |
Delay in diagnosis, months | 9.5 (4.3) | NA | |
Interval between diagnosis to urine collection, years | 1.20 (0.2) | NA | |
Gender, n | 0.92 | ||
Female | 14 | 15 | |
Male | 6 | 6 | |
Race/Ethnicity, n | 0.01 | ||
Caucasian | 10 | 18 | |
Non-Caucasian | 10 | 3 | |
African-American | 3 | 1 | |
Hispanic | 3 | 2 | |
Asian | 1 | 0 | |
Multi-racial or unknown | 3 | 0 | |
Myositis-specific autoantibody, n | NA | ||
Anti-p155/140 (TIF1) | 12 | ||
Anti-MJ (NXP-2) | 5 | ||
Anti-MDA5 | 3 | ||
MD global disease activity, 0–10 cm VAS | 3.8 (0.39) | NA | |
Medication, n | |||
Prednisone | 17 | 0 | |
Average dose mg/kg/day (SEM) | 0.60 (0.1) | ||
Methotrexate | 12 | 0 | |
IVIG | 9 | 0 | |
Ciclosporin | 3 | 0 | |
Average number of immunosuppressivesa | 2.3 (0.24) | 0 | |
Urine protein level, mg/dl | 262.0 (34.0) | 214.8 (17.9) | 0.22 |
. | JDM (n = 20) . | HC (n = 21) . | P-value . |
---|---|---|---|
Age at enrolment, years | 9.2 (1.0) | 9.8 (0.6) | 0.59 |
Disease onset age, years | 5.0 | NA | |
Delay in diagnosis, months | 9.5 (4.3) | NA | |
Interval between diagnosis to urine collection, years | 1.20 (0.2) | NA | |
Gender, n | 0.92 | ||
Female | 14 | 15 | |
Male | 6 | 6 | |
Race/Ethnicity, n | 0.01 | ||
Caucasian | 10 | 18 | |
Non-Caucasian | 10 | 3 | |
African-American | 3 | 1 | |
Hispanic | 3 | 2 | |
Asian | 1 | 0 | |
Multi-racial or unknown | 3 | 0 | |
Myositis-specific autoantibody, n | NA | ||
Anti-p155/140 (TIF1) | 12 | ||
Anti-MJ (NXP-2) | 5 | ||
Anti-MDA5 | 3 | ||
MD global disease activity, 0–10 cm VAS | 3.8 (0.39) | NA | |
Medication, n | |||
Prednisone | 17 | 0 | |
Average dose mg/kg/day (SEM) | 0.60 (0.1) | ||
Methotrexate | 12 | 0 | |
IVIG | 9 | 0 | |
Ciclosporin | 3 | 0 | |
Average number of immunosuppressivesa | 2.3 (0.24) | 0 | |
Urine protein level, mg/dl | 262.0 (34.0) | 214.8 (17.9) | 0.22 |
Data are shown as mean (SEM), except for gender, race and medication which are shown as N.
Including those listed under medications, as well as tacrolimus (n = 1), infliximab (n = 1), mycophenolate (n = 1).
HC: healthy control; IVIG: immunoglobulin; MD: medical doctor/physician; SEM: standard error of the mean; VAS: visual analogue scale; NA: not applicable.
Urine protein identification
Urine samples from JDM and HC participants were used to identify protein biomarkers associated with JDM pathogenesis. In total, 2348 proteins were quantified in all 41 samples. No proteins were found exclusively in JDM samples, indicating that there were no disease-specific biomarkers. Of the 2348 total proteins, 275 proteins were identified across all JDM and HC samples and were used for analysis. Volcano plot analysis showing significance vs log2 fold change of these 275 proteins is shown in Supplementary Fig. S2, available at Rheumatology online.
LIMMA’s t test identified 42 proteins that were significantly different between the JDM and HC groups (Supplementary Table S1, available at Rheumatology online). After correcting for multiple testing [adjusted (adj) P < 0.05], urine expression levels of nine proteins remained significantly altered between JDM and HC (Table 2).
Urine proteins altered in their level of expression in JDM patients compared with healthy controls
Gene . | Protein name . | Uniprot ID . | FC (JDM/HC) . | JDM mean NPX . | HC mean NPX . | P-value (adj) . |
---|---|---|---|---|---|---|
CTSD | Cathepsin D | P07339 | 2.0 | 2.63 | 1.33 | 0.001 |
COL2A1 | Collagen alpha-1(II) chain | P024582 | −1.9 | 0.63 | 1.18 | 0.001 |
COL1A1 | Collagen alpha-1(I) chain | P02452 | −1.5 | 0.89 | 1.29 | 0.006 |
SHISA5 | Protein shisa-5 | Q8N114 | 1.4 | 1.49 | 1.09 | 0.015 |
LGALS3BP | Galectin-3 binding protein | Q08380 | 1.3 | 1.27 | 0.98 | 0.024 |
APOD | Apolipoprotein D | P05090 | 1.4 | 1.56 | 1.08 | 0.033 |
CILP2 | Cartilage intermediate layer protein 2 | Q8IUL8 | −1.5 | 0.53 | 0.79 | 0.036 |
COL12A1 | Collagen alpha-1(XII) chain | Q99715 | −1.3 | 0.73 | 0.95 | 0.036 |
LYNX1 | Ly-6/neurotoxin-like protein 1 | Q9BZG9 | −1.3 | 1.05 | 1.35 | 0.043 |
Gene . | Protein name . | Uniprot ID . | FC (JDM/HC) . | JDM mean NPX . | HC mean NPX . | P-value (adj) . |
---|---|---|---|---|---|---|
CTSD | Cathepsin D | P07339 | 2.0 | 2.63 | 1.33 | 0.001 |
COL2A1 | Collagen alpha-1(II) chain | P024582 | −1.9 | 0.63 | 1.18 | 0.001 |
COL1A1 | Collagen alpha-1(I) chain | P02452 | −1.5 | 0.89 | 1.29 | 0.006 |
SHISA5 | Protein shisa-5 | Q8N114 | 1.4 | 1.49 | 1.09 | 0.015 |
LGALS3BP | Galectin-3 binding protein | Q08380 | 1.3 | 1.27 | 0.98 | 0.024 |
APOD | Apolipoprotein D | P05090 | 1.4 | 1.56 | 1.08 | 0.033 |
CILP2 | Cartilage intermediate layer protein 2 | Q8IUL8 | −1.5 | 0.53 | 0.79 | 0.036 |
COL12A1 | Collagen alpha-1(XII) chain | Q99715 | −1.3 | 0.73 | 0.95 | 0.036 |
LYNX1 | Ly-6/neurotoxin-like protein 1 | Q9BZG9 | −1.3 | 1.05 | 1.35 | 0.043 |
Each protein is listed with its fold change (FC), average normalized protein expression (NPX) and adjusted (adj) P-value by Benjamini and Hochberg false-discovery rate correction.
HC: healthy control.
Urine proteins altered in their level of expression in JDM patients compared with healthy controls
Gene . | Protein name . | Uniprot ID . | FC (JDM/HC) . | JDM mean NPX . | HC mean NPX . | P-value (adj) . |
---|---|---|---|---|---|---|
CTSD | Cathepsin D | P07339 | 2.0 | 2.63 | 1.33 | 0.001 |
COL2A1 | Collagen alpha-1(II) chain | P024582 | −1.9 | 0.63 | 1.18 | 0.001 |
COL1A1 | Collagen alpha-1(I) chain | P02452 | −1.5 | 0.89 | 1.29 | 0.006 |
SHISA5 | Protein shisa-5 | Q8N114 | 1.4 | 1.49 | 1.09 | 0.015 |
LGALS3BP | Galectin-3 binding protein | Q08380 | 1.3 | 1.27 | 0.98 | 0.024 |
APOD | Apolipoprotein D | P05090 | 1.4 | 1.56 | 1.08 | 0.033 |
CILP2 | Cartilage intermediate layer protein 2 | Q8IUL8 | −1.5 | 0.53 | 0.79 | 0.036 |
COL12A1 | Collagen alpha-1(XII) chain | Q99715 | −1.3 | 0.73 | 0.95 | 0.036 |
LYNX1 | Ly-6/neurotoxin-like protein 1 | Q9BZG9 | −1.3 | 1.05 | 1.35 | 0.043 |
Gene . | Protein name . | Uniprot ID . | FC (JDM/HC) . | JDM mean NPX . | HC mean NPX . | P-value (adj) . |
---|---|---|---|---|---|---|
CTSD | Cathepsin D | P07339 | 2.0 | 2.63 | 1.33 | 0.001 |
COL2A1 | Collagen alpha-1(II) chain | P024582 | −1.9 | 0.63 | 1.18 | 0.001 |
COL1A1 | Collagen alpha-1(I) chain | P02452 | −1.5 | 0.89 | 1.29 | 0.006 |
SHISA5 | Protein shisa-5 | Q8N114 | 1.4 | 1.49 | 1.09 | 0.015 |
LGALS3BP | Galectin-3 binding protein | Q08380 | 1.3 | 1.27 | 0.98 | 0.024 |
APOD | Apolipoprotein D | P05090 | 1.4 | 1.56 | 1.08 | 0.033 |
CILP2 | Cartilage intermediate layer protein 2 | Q8IUL8 | −1.5 | 0.53 | 0.79 | 0.036 |
COL12A1 | Collagen alpha-1(XII) chain | Q99715 | −1.3 | 0.73 | 0.95 | 0.036 |
LYNX1 | Ly-6/neurotoxin-like protein 1 | Q9BZG9 | −1.3 | 1.05 | 1.35 | 0.043 |
Each protein is listed with its fold change (FC), average normalized protein expression (NPX) and adjusted (adj) P-value by Benjamini and Hochberg false-discovery rate correction.
HC: healthy control.
Protein biomarkers associated with JDM
We identified proteins that were previously shown to be increased in the serum or muscle of myositis patients compared with HC, including cathepsin D and galectin-3 binding protein (Table 2, Fig. 1). We also identified novel candidate biomarkers that were either increased or decreased in the urine of JDM patients compared with HC (Table 2, Fig. 1). The increased proteins included protein shisa-5 (SHISA5) and apolipoprotein D. The proteins that were decreased in the urine of JDM patients compared with HC included collagen alpha-1(II) chain (COL2A1), collagen alpha-1(I) chain (COL1A1), cartilage intermediate layer protein 2 (CILP2), collagen alpha-1(XII) chain (COL12A1), and ly6/neurotoxinlike protein (LYNX1).

Scatterplots of urine proteins significantly increased (top) and decreased (bottom) in JDM patients vs HC. Data are presented as mean ± SEM. **P ≤ 0.001, *P < 0.05. CTSD: cathepsin D (adj P = 0.001); LGALS3BP: galectin-3 binding protein (adj P = 0.02); SHISA5: protein shisa-5 (adj P = 0.02); COL1A1: collagen alpha-1 (I) chain (adj P = 0.01); COL2A1: collagen alpha-1(II) chain (adj P = 0.001); COL12A1: collagen alpha-1(XII) chain (adj P = 0.04); NPX: normalized protein expression; HC: healthy control
The significantly altered proteins were then entered into STRING. Despite the small number of entries, the proteins were significantly associated with Gene Ontology (GO) biological functions that are related with myositis, including collagen fibril organization (GO:0030199, adj P < 0.0001), extracellular matrix organization (GO:0030198, adj P < 0.001), skeletal system development (GO:0001501, adj P < 0.05) and skin morphogenesis (GO:0043589, adj P < 0.05). The significantly altered proteins were also entered into the DisGeNET database, which revealed many skin and connective tissue diseases and musculoskeletal diseases that are associated with these proteins. For example, collagens (e.g. COL1A1, COL2A1, COL12A1), galectin-3 binding protein and cathepsin D are associated with the autoimmune disease rheumatoid arthritis. Galectin-3 binding protein is associated with SLE, which is associated with a type I IFN signature seen in DM/JDM. COL12A1 is associated with various muscular dystrophies. Lastly, galectin-3 binding protein, ly6/neurotoxinlike protein, collagen alpha-1(I) chain and cathepsin D are associated with psoriasis and psoriatic arthritis.
Validation of candidate biomarkers
Using ELISAs, validation of the mass spectrometry findings was supported, as cathepsin D and galectin-3 binding protein were significantly elevated (P-values <0.05) in the urine of JDM patients compared with HC (Fig. 2).
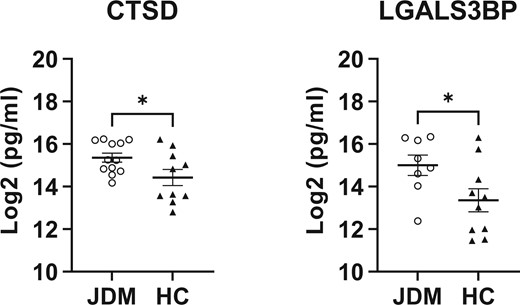
Scatterplots of proteins validated using commercially available ELISA assays. Validation of CTSD (n = 10 and 12 for HC and JDM, respectively) and LGALS3BP (n = 10 and 8 for HC and JDM, respectively) urine expression by ELISA using unpaired t-tests (P < 0.05). Data are presented as mean ± SEM. *P < 0.05. CTSD: cathepsin D; LGALS3BP: galectin-3 binding protein
Correlations with disease activity and damage
Correlations of the nine significantly altered proteins in the urine of JDM patients with clinical measures of disease activity and damage were examined (Table 3; Supplementary Table S2, available at Rheumatology online). Measures of disease damage, including myositis damage index (MDI) muscle damage and physician global disease damage were significantly correlated with cathepsin D (r = 0.57) and COL2A1 (r = 0.48), respectively. COL2A1 significantly correlated with the MDI total severity score (r = 0.64). Several measures of disease activity also significantly correlated with many of the proteins. Cathepsin D and COL2A1 negatively correlated with the childhood myositis assessment scale (CMAS) (r = −0.54 and −0.60, respectively). COL2A1 and COL1A1 correlated with disease activity score (DAS) muscle scale (r = 0.53 and 0.55, respectively). In terms of extramuscular activity, COL1A1 negatively correlated with the myositis disease activity assessment tool (MDAAT) cardiovascular score (r = −0.70) and cathepsin D negatively correlated with the MDAAT pulmonary score (r = −0.54). We also found that COL1A1 and COL12A1 correlated with the serum muscle enzyme aspartate aminotransferase (r = 0.46 and 0.51, respectively, Supplementary Table S3, available at Rheumatology online). There were no correlations with any of the nine proteins and disease duration (data not shown). Lastly, significant negative correlations (P < 0.05) emerged for prednisone dose and COL1A1 (r = −0.47), COL2A1 (r = −0.56) and COL12A1 (r = −0.56).
Correlations of a subset of significantly altered proteins with clinical measures of activity and disease in JDM patients
Protein name . | Gene . | Clinical measure . | r . | P-value . |
---|---|---|---|---|
Cathepsin D | CTSD | MDI muscle damage | 0.57 | P < 0.05 |
CMAS | −0.54 | P < 0.05 | ||
MDAAT pulmonary | −0.54 | P < 0.05 | ||
Collagen alpha-1(II) chain | COL2A1 | MD global disease damage | 0.48 | P < 0.05 |
MDI total severity | 0.64 | P < 0.05 | ||
CMAS | −0.60 | P < 0.05 | ||
DAS muscle | 0.53 | P < 0.05 | ||
Collagen alpha-1(I) chain | COL1A1 | AST | 0.46 | P < 0.05 |
DAS muscle | 0.55 | P < 0.05 | ||
MDAAT cardiovascular | −0.70 | P < 0.01 | ||
Collagen alpha-1(XII) chain | COL12A1 | AST | 0.51 | P < 0.05 |
Ly6/neurotoxin-like protein 1 | LYNX1 | Aldolase | −0.52 | P < 0.05 |
Protein name . | Gene . | Clinical measure . | r . | P-value . |
---|---|---|---|---|
Cathepsin D | CTSD | MDI muscle damage | 0.57 | P < 0.05 |
CMAS | −0.54 | P < 0.05 | ||
MDAAT pulmonary | −0.54 | P < 0.05 | ||
Collagen alpha-1(II) chain | COL2A1 | MD global disease damage | 0.48 | P < 0.05 |
MDI total severity | 0.64 | P < 0.05 | ||
CMAS | −0.60 | P < 0.05 | ||
DAS muscle | 0.53 | P < 0.05 | ||
Collagen alpha-1(I) chain | COL1A1 | AST | 0.46 | P < 0.05 |
DAS muscle | 0.55 | P < 0.05 | ||
MDAAT cardiovascular | −0.70 | P < 0.01 | ||
Collagen alpha-1(XII) chain | COL12A1 | AST | 0.51 | P < 0.05 |
Ly6/neurotoxin-like protein 1 | LYNX1 | Aldolase | −0.52 | P < 0.05 |
AST: aspartate aminotransferase; CMAS: childhood myositis assessment score; DAS: disease activity score; MD: physician; MDAAT: myositis disease activity assessment tool; MDI: myositis damage index.
Correlations of a subset of significantly altered proteins with clinical measures of activity and disease in JDM patients
Protein name . | Gene . | Clinical measure . | r . | P-value . |
---|---|---|---|---|
Cathepsin D | CTSD | MDI muscle damage | 0.57 | P < 0.05 |
CMAS | −0.54 | P < 0.05 | ||
MDAAT pulmonary | −0.54 | P < 0.05 | ||
Collagen alpha-1(II) chain | COL2A1 | MD global disease damage | 0.48 | P < 0.05 |
MDI total severity | 0.64 | P < 0.05 | ||
CMAS | −0.60 | P < 0.05 | ||
DAS muscle | 0.53 | P < 0.05 | ||
Collagen alpha-1(I) chain | COL1A1 | AST | 0.46 | P < 0.05 |
DAS muscle | 0.55 | P < 0.05 | ||
MDAAT cardiovascular | −0.70 | P < 0.01 | ||
Collagen alpha-1(XII) chain | COL12A1 | AST | 0.51 | P < 0.05 |
Ly6/neurotoxin-like protein 1 | LYNX1 | Aldolase | −0.52 | P < 0.05 |
Protein name . | Gene . | Clinical measure . | r . | P-value . |
---|---|---|---|---|
Cathepsin D | CTSD | MDI muscle damage | 0.57 | P < 0.05 |
CMAS | −0.54 | P < 0.05 | ||
MDAAT pulmonary | −0.54 | P < 0.05 | ||
Collagen alpha-1(II) chain | COL2A1 | MD global disease damage | 0.48 | P < 0.05 |
MDI total severity | 0.64 | P < 0.05 | ||
CMAS | −0.60 | P < 0.05 | ||
DAS muscle | 0.53 | P < 0.05 | ||
Collagen alpha-1(I) chain | COL1A1 | AST | 0.46 | P < 0.05 |
DAS muscle | 0.55 | P < 0.05 | ||
MDAAT cardiovascular | −0.70 | P < 0.01 | ||
Collagen alpha-1(XII) chain | COL12A1 | AST | 0.51 | P < 0.05 |
Ly6/neurotoxin-like protein 1 | LYNX1 | Aldolase | −0.52 | P < 0.05 |
AST: aspartate aminotransferase; CMAS: childhood myositis assessment score; DAS: disease activity score; MD: physician; MDAAT: myositis disease activity assessment tool; MDI: myositis damage index.
Discussion
In the current study, we used urine samples from JDM patients and HC to analyse the urine proteome and identify disease-related biomarkers using a MS-based proteomics approach with TMT labelling. We identified 2348 urine proteins in 21 control and 20 JDM samples, with 275 proteins common to all samples. Previous urine proteome profiling studies have identified between 1000 to over 2000 proteins in human participants using various MS methods [31, 32]. While MS-based proteomics is an extremely powerful tool, this wide range of identified proteins highlights variability as one of the challenges of proteomic studies using biological fluids, including urine. In the current study, we sought to reduce this variability by optimizing the proteomic workflow. This was accomplished by removing salts and interfering substances during sample preparation, enhancing the digestion efficiency by performing the trypsin application multiple times over several hours instead of in a single application, increasing sensitivity by using high-pH reversed-phase peptide fractionation (10 fractionations total) and by using TMT labelling.
JDM is characterized by chronic inflammation of skeletal muscles, which results in muscle weakness. Additionally, characteristic skin rashes, vasculopathy and in many cases calcinosis are extra-muscular manifestations of the disease. In this study, we have identified biomarkers that were differentially altered in the urine of JDM patients that are related to key features of JDM, such as inflammation, immune responses and extracellular matrix (ECM) organization. Two of the identified biomarkers that we detected to be increased in JDM patient urine samples, cathepsin D and galectin-3 binding protein, were previously reported to be increased in the muscle and serum of myositis patients. Although these proteins are not unique to JDM, as described below, identifying these biomarkers in the current study demonstrates proof of principle using a urine-based TMT-labelling mass spectrometry approach.
Galectin-3 binding protein is a secreted glycoprotein that is a member of the scavenger receptor cysteine-rich superfamily, which includes proteins that modulate innate immunity and host defense. Galectin-3 binding protein has strong anti-viral activity, as it upregulates type I IFN production, possibly by acting as a scaffold/adaptor protein in the signalling cascade [33]. Activation of type I IFN, including peripheral IFNα and a broad type I IFN gene signature, is robustly observed in rheumatic diseases such as DM/JDM and SLE [34–37]. Given that the IFN signature positively correlates with disease activity and response to treatment in DM/JDM patients, it is a biomarker of interest in the myositis field. In SLE, galectin-3 binding protein has recently garnered interest as a surrogate biomarker for IFN activity, given that it is part of the IFN gene signature and its expression is increased in SLE patients [38–40]. Furthermore, autoantibodies to galectin-3 were reported at higher frequencies in DM and SLE patients compared with HC and patients with other autoimmune diseases [41]. In DM and JDM patients, serum galectin-3 binding protein levels were also significantly increased compared with HC [19, 39, 41]. In the current study, we found that galectin-3 binding protein expression was significantly increased in the urine of JDM patients compared with controls in both the MS-based proteomics experiments and then validated by ELISA. While we were unable to find any significant correlations with myositis activity or damage measures, likely due to the small sample size of the present study, others have reported that galectin-3 binding protein levels positively correlate with the MDAAT MITAX score [42]. Furthermore, these levels were found to be decreased in JDM and IIM patients, including in patients with DM, following treatment [25, 42]. Detecting peripheral IFN has been a challenge due to its low circulating levels; therefore, we were unable to determine whether IFN protein levels and galectin-3 binding protein levels correlate with each other in JDM patients. Overall, our findings add further support for a role for galectin-3 binding protein as a biomarker in JDM and other forms of myositis.
Cathepsins are lysosomal proteases that regulate protein turnover and cell metabolism, but they also are involved in immune responses, autophagy, and growth and development [43]. While not extensively documented in the myositis field, increased cathepsin D muscle protein expression in sporadic inclusion body myositis (sIBM) and polymyositis (PM) patients were reported compared with HC [22]. Based on those and additional findings of ER stress possibly contributing to impaired lysosomal degradation, impairments in protein disposal may contribute to sIBM pathogenesis [22]. We similarly observed significantly increased cathepsin D expression in the urine of JDM patients using our MS-based approach and validated these findings using an independent ELISA. Furthermore, we found that cathepsin D expression was significantly negatively correlated with CMAS and positively correlated with MDI muscle damage. These associations with clinical measures of myositis activity and muscle damage, coupled with the increased expression in two independent assays, suggests that additional studies should examine the increase in cathepsin D expression and its role in myositis.
Expression levels of proteins involved in ECM organization [e.g. collagen alpha-1(I), collagen alpha-1(XII) and collagen alpha-1(II)] were significantly lower in the urine of JDM patients compared with controls. Changes in ECM composition and expression, including fibrosis, are associated with numerous diseases. In adult DM and PM patients, 7 weeks of resistance exercise training improved muscle strength and reduced the expression of fibrotic genes involved in collagen synthesis (e.g. COL1A1), suggesting a beneficial reduction in fibrosis from exercise [44]. The results from the current study show a significant decrease in ECM components, including collagens, in the urine of JDM patients. It is possible that reduced collagen expression levels observed here are due to treatment, as most patients in the current study were on prednisone or other immunosuppressive medication. This is supported by the significant negative relationships observed between prednisone dose and all three of these collagens, indicating that JDM patients receiving higher doses of prednisone had lower levels of these collagen proteins. ECM turnover may also be regulated by interactions with cathepsin D and other proteases, as shown in a fibrotic chronic kidney disease mouse model [45]. Nevertheless, JDM patients had ongoing disease (Table 1) and significant correlations emerged between collagen expression and myositis measures of damage and activity (Table 3). For example, positive relationships were observed between COL2A1 and COL1A1 and DAS Muscle score. COL2A1 also positively correlated with physician global disease damage and MDI total severity and negatively correlated with CMAS. These results suggest that increased collagen expression may be associated with worse muscle clinical outcomes. Interestingly, COL1A1 negatively correlated with MDAAT cardiovascular activity, with a trend (P = 0.06) for collagen alpha-1(II) to be negatively correlated with cardiovascular activity as well. While cardiac abnormalities are not frequently reported in JDM, they do occur and screening is recommended [46]. Our results suggest that these collagens may be involved in cardiovascular disease activity and should be explored further in JDM.
One of the limitations of the current study is a small sample size (20–21 subjects per group). Therefore, before these proteins can be used in a clinical setting as biomarkers of disease activity, damage or response to treatment for JDM patients, these results need to be reproduced using a larger population. However, despite the small sample size, we did confirm several biomarkers previously reported in patients with myositis and other related diseases. Furthermore, we supported these findings using two independent assays: a MS-based proteomics approach and by ELISA. Although we validated cathepsin D and galectin-3 binding protein as potential biomarkers of JDM, we cannot determine whether these proteins are unique to JDM. However, it is unlikely that these biomarkers are specific to JDM and may be useful as urine biomarkers for other myositis subgroups as well (e.g. PM, DM, sIBM). For example, cathepsin D expression was shown to be elevated in sIBM and PM muscle, but was not previously examined in JDM [22]. Given its role in upregulating type I IFN, galectin-3 binding protein may be of greater interest in JDM/DM due to a more robust expression of type I IFN response in these subgroups [33]. In support of this, we and others have shown increased galectin-3 binding protein expression in JDM/DM compared with HC [39, 41]. Another limitation is that all JDM patients, except one, were receiving at least one immunosuppressive medication, which primarily included prednisone. Therefore, the results may be compromised by medication effects. For example, all identified collagens were significantly negatively correlated with prednisone dose. However, the JDM patients in this study had ongoing active disease despite treatment. One of our validated proteins, galectin-3 binding protein, was previously reported to be increased in the serum of untreated JDM patients and was subsequently decreased following treatment [25]. Therefore, while we cannot definitively separate treatment effects from the results of the current study, our data align with the untreated results reported previously. We also did not have serial samples to examine multiple time points and correlations with changes in disease activity. Further evaluation of these biomarkers in larger cohorts and examining changes over time is needed.
Altogether, we have shown that an optimized MS-based urine proteomics protocol can identify proteins that are associated with key aspects of JDM. While this process requires specialty equipment and is costly, it does provide support for using urine proteomics to characterize pathophysiological processes involved with this disease and potentially develop a panel of biomarkers to assist in prognosis and targeted therapies. These results add myositis to the rapidly growing number of diseases that are using urine proteomics to identify biomarkers.
Supplementary material
Supplementary material is available at Rheumatology online.
Data availability
The data underlying this article will be shared on reasonable request to the corresponding author.
Funding
This work was supported by the Intramural Research Program of the National Institute of Environmental Health Sciences (ZIA ES101074, ZIA ES101081), and National Institute of Arthritis and Musculoskeletal and Skin Diseases (project AR041215-02), of the National Institutes of Health, and The Myositis Association and Cure JM Foundation.
Disclosure statement: The authors have declared no conflicts of interest.
Acknowledgements
We thank Drs Leesa Deterding and Laura Lewandowski for critical review of the manuscript.
Comments