-
PDF
- Split View
-
Views
-
Cite
Cite
Divya Sardana, Suresh Vasa, Nishanth Vepachedu, Jing Chen, Ranga Chandra Gudivada, Bruce J. Aronow, Anil G. Jegga, PhenoHM: human–mouse comparative phenome–genome server, Nucleic Acids Research, Volume 38, Issue suppl_2, 1 July 2010, Pages W165–W174, https://doi.org/10.1093/nar/gkq472
- Share Icon Share
Abstract
PhenoHM is a human–mouse comparative phenome–genome server that facilitates cross-species identification of genes associated with orthologous phenotypes (http://phenome.cchmc.org; full open access, login not required). Combining and extrapolating the knowledge about the roles of individual gene functions in the determination of phenotype across multiple organisms improves our understanding of gene function in normal and perturbed states and offers the opportunity to complement biologically the rapidly expanding strategies in comparative genomics. The Mammalian Phenotype Ontology (MPO), a structured vocabulary of phenotype terms that leverages observations encompassing the consequences of mouse gene knockout studies, is a principal component of mouse phenotype knowledge source. On the other hand, the Unified Medical Language System (UMLS) is a composite collection of various human-centered biomedical terminologies. In the present study, we mapped terms reciprocally from the MPO to human disease concepts such as clinical findings from the UMLS and clinical phenotypes from the Online Mendelian Inheritance in Man knowledgebase. By cross-mapping mouse–human phenotype terms, extracting implicated genes and extrapolating phenotype-gene associations between species PhenoHM provides a resource that enables rapid identification of genes that trigger similar outcomes in human and mouse and facilitates identification of potentially novel disease causal genes. The PhenoHM server can be accessed freely at http://phenome.cchmc.org.
INTRODUCTION
While the post-genomic translational research era is witnessing a paradigm shift with increased focus on phenome over genome, our ability to precisely specify an observed human phenotype and compare it to related phenotypes of model organisms remains challenging and does not match the throughput capabilities of genotypic studies (1). Thus, there is a pressing demand for technologies that will lead to greater and better integration of phenotypic data and phenotype-centric discovery tools to aid biomedical research (1–4). Phenotype, the descriptor of the phenome, is the sum of a genotype and its interactions with the environment. Advances in gene expression profiling, comparative genomics, standard notations for gene function [e.g. Gene Ontology (5), Mammalian Phenotype Ontology (MPO) (6)] and complementary integrative strategies [e.g. PhenoGO (7), PhenomicDB (8), OrthoDisease (9)] have helped in advancing the knowledge of gene functions and assigning phenotypic contexts. In spite of significant breakthroughs in the representation of complex biological entities and phenomena as various ontologies, the largest repository of phenotype data continues to be the biomedical literature. Automatic extraction of phenotype data from this free text corpus is a challenge (10–11). Other bottlenecks include the complex nature of the phenotype data, terminology-related issues and difficulties of integration and normalization. The MPO (6) from Mouse Genome Database (MGD) (12) enables robust annotation of mammalian phenotypes in the context of mutations, quantitative trait loci and strains that are used as models of human biology and disease. The MPO supports different levels and richness of phenotypic knowledge and flexible annotations to individual genotypes. However, there is limited mapping of mouse phenotype terms to human phenotypes [e.g. human phenotype terms in the Online Mendelian Inheritance in Man (OMIM) and Unified Medical Language System (UMLS)] with some attempts focusing on available mouse models for human diseases in OMIM (13) by the MGI (Mouse Genome Informatics) curators.
In the current study, we focused on the mouse phenotype because it is the key model organism for the analysis of mammalian developmental, physiological and disease processes (14). A question we have sought to answer is whether merging the mouse and human phenotypes can provide leverage for finding better and novel phenome–genome relations. As a first step toward an effective comparative phenomics, we have mapped the mouse phenotype concepts from the controlled ontological repository of MPO to human phenotype terms from UMLS (all concepts under semantic group ‘Disorder’) (15) and Human Phenotype Ontology (HPO) (16). Second, we also mapped separately the MPO terms to OMIM (13) records, and for all mapped phenotype terms we extracted the corresponding human gene allelic variant information, where available. Third, for all the terminologically mapped phenotypes between mouse and human, which we call ‘orthologous phenotypes’, we extract the human–mouse orthologous genes that share this phenotype. The unmapped genes (orthologous genes that do not share similar phenotype) could be potentially novel candidate genes for the orthologous phenotype.
DATA SOURCES
For the current study, we use ontologies, biomedical metathesaurus and human disease knowledgebase that cover the mammalian phenotype and more precisely the human and the mouse phenotypes and the associated genes. For mouse phenotypes and gene associations, we use the MPO (12), a structured controlled vocabulary for annotating mammalian phenotypic data developed by the Jackson Laboratory. For human phenotype terms, we use both the UMLS metathesaurus (15) and the HPO (16). Since neither the HPO nor the UMLS metathesaurus contain the allelic variant information for human diseases, we additionally use the OMIM (13), the knowledgebase of human genes and phenotypes. Additional details of each of these data resources are provided in the following sections.
MPO and gene associations
Mouse phenotype annotations and MPO term-associated genes were obtained from MGD (12). The mouse phenotype-to-genotype relations were extracted from the ‘MGI_PhenoGenoMP.rpt’ file downloaded from the MGD ftp site and mapped to the corresponding mouse gene symbols (because phenotype terms from MPO are associated directly with genotypes instead of genes) and human orthologous genes using the reports ‘MGI_EntrezGene.rpt’ and ‘HMD_HGNC_Accession.rpt’. Each term in the MPO has a unique accession identifier, a definition and, when available, synonyms. The MPO term ID, preferred name and synonyms were obtained from the ‘MPheno_OBO’ ontology file. Simple ‘JAVA’ scripts were written to parse, concatenate and store these data files in an Oracle relational database. The MPO has 33 root nodes representing different body systems (Figure 1). At the time of writing this article, there were ∼7000 unique MPO terms assigned to ∼33 000 alleles from ∼5700 unique mouse genes. Most of these data are derived from genetically engineered knock-out mice or naturally occurring mutants. The mouse-human ortholog table has ∼17 000 gene entries.
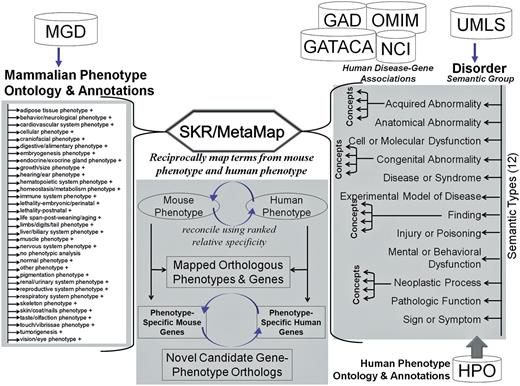
Schematic representation of resources, workflow and methodology in PhenoHM server. The MPO terms are mapped to human phenotype terms in HPO and UMLS and OMIM records. For the mapped terms associated with mouse and human genes are extracted and compared to identify ortholog genes with orthologous phenotypes.
UMLS metathesaurus
The UMLS is the largest available compendium of biomedical vocabularies (15). The UMLS metathesaurus is a very large multi-purpose and multi-lingual vocabulary database that contains information about biomedical concepts, their various names and the relationships among them. The UMLS metathesaurus is organized by concept. One of its primary purposes is to connect different names for the same concept from many different vocabularies. The metathesaurus concept structure includes concept names, their identifiers and key characteristics of these concept names (e.g. language, vocabulary source, name type). Each concept or meaning in the metathesaurus has a unique and permanent concept unique identifier (CUI). The Semantic Network of the UMLS contains 135 semantic types (e.g. disease or syndrome, sign or symptom) organized into 15 semantic groups. The 15 semantic groups provide a partition of the UMLS metathesaurus for 99.5% of the concepts. For MPO term mapping to UMLS concepts we focus only on the semantic group ‘Disorder’, which has 12 semantic types (Figure 1). Each semantic type has several concepts represented with a unique CUI, term and, when available, a definition and synonyms.
HPO and gene associations
The HPO contains ∼9500 terms representing various human phenotypes. For the current study, we focus on the sub-ontology ‘Organ abnormality’, which contains descriptions of clinical abnormalities (16). The HP-OMIM-Gene annotations that contain ∼4800 terms from OMIM (and their associated genes) mapped to HPO were downloaded from the HPO web site (http://www.human-phenotype-ontology.org). The HP-UMLS CUI mapping data was downloaded from http://www.berkeleybop.org/ontologies/obo-all/human_phenotype/human_phenotype.xref.
OMIM—allelic variants
The OMIM (13), a knowledgebase of human genes and phenotypes, is derived exclusively from the published biomedical literature and is updated daily (17). It currently contains ∼20 000 full-text entries describing phenotypes and genes. To date, ∼3000 genes have mutations causing disease. For most genes, selected mutations are included as allelic variants and most of the allelic variants represent disease producing mutations (17).
Degrees of detail: MPO versus the UMLS and HPO
Certain human phenotype concepts require relatively finer granularity when compared to the mouse counterparts. For instance, the phenotype cataract was more granular and precise in HPO than in MPO (Figure 2A and B). Likewise, in UMLS, the term cataract mapped to four different concepts (Figure 2C). In most cases, the granularity was a result of the distinction between semantic types (e.g. ‘finding’ versus ‘disease or syndrome’ versus ‘anatomical abnormality’ for the phenotype ‘cataract’ in UMLS). While in the mouse phenotype it may not be critical to differentiate among different types of cataract, in most human-related clinical situations, the distinction between whether the abnormality is a clinical finding or anatomical abnormality (congenital or acquired) is necessary and helpful in making clinical decisions. Similarly, the phenotypes ‘albino’ and ‘pale skin’ are listed as synonyms of ‘absent skin pigmentation’ in MPO. Although linguistically, these classifications are at least partially correct, clinically these terms could refer to totally different phenotypes (congenital abnormality versus finding); hence they are listed as different concepts in the UMLS. There are also cases where the granularity in MPO is finer than in the HPO. For example, the terms ‘hydroureter’ (distention of the ureter with urine or watery fluid due to obstruction from any cause) and ‘megaureter’ (congenital ureteral dilatation, which may be either primary or secondary to something else) are distinct concepts in MPO, while in the HPO ‘megaureter’ is a synonym of ‘hydroureter’. We have also observed cases of potentially wrong synonymy in MPO. For example, the normal states or phenotypes are sometimes listed as synonyms for abnormal states or phenotypes (e.g. ‘reflexes’ is a synonym for ‘abnormal reflex’).
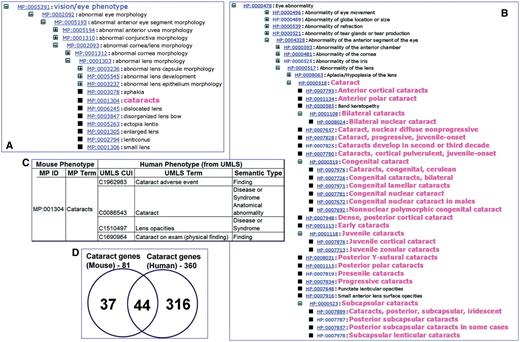
Example of a phenotype mapping from MPO to HP and UMLS. The MPO tree view (A) and HPO tree view (B) show the granularity of concepts for cataract in the two ontologies. (C) The mapping of MPO term cataract to four different UMLS concepts as indicated by the unique CUIs and corresponding terms. (D) Overlap between cataract-associated genes of mouse and human. Of the 44 shared genes for cataract, 20 genes had known allelic variants associated with cataract.
Human disease–gene associations
While the OMIM (13) is a reliable source of disease genes, it encompasses only diseases that tend to be both Mendelian in character and have experimentally confirmed and published mutations. Hence, other sources of disease–genes were also explored including text-mined results from GeneRIF (Gene Reference into Function) sentences [using MetaMap (18) and the results stored in our in-house GATACA database Unpublished], GAD (19), Comparative Toxicogenomics Database (CTD) disease biomarkers (20) and Genome-wide association study (GWAS) genes (21). The GATACA (genetic associations to anatomical and clinical abnormalities) is an in-house knowledgebase which has a compilation of human disease–gene associations extracted from text-mining of GeneRIF sentences from NCBI’s Entrez Gene. The Genetic Association Database or GAD (19) is an archive of published genetic association studies that provides a comprehensive, public, web-based repository of molecular, clinical and study parameters for >11 000 human genetic association studies at this time. The CTD (20) contains direct and inferred human gene-disease relationships. Direct human gene–disease relationships are curated from the published literature by CTD curators, or are derived from the OMIM database using the ‘mim2gene’ file from the NCBI Entrez Gene database (22). For the current study, we use direct gene–disease relationships only. The GWAS genes were extracted from the publicly available catalog of published genome-wide association studies (21). We integrated data from all these resources by mapping the disease terms from each resource to a common standard identifier (UMLS CUI from semantic group ‘Disorder’).
DATA PROCESSING AND STORAGE
The PhenoHM cross-species phenotype mapping was carried out in three steps: (i) matching mouse phenotype terms from MPO to UMLS concepts (all concepts falling under the 12 semantic types of the semantic group ‘Disorder’), HPO terms and OMIM records (Figure 1); (ii) searching for gene associations of MPO, HPO and UMLS phenotype terms using the MPO and HPO gene annotations and other disease–gene data resources (13,19); and (iii) extracting orthologous gene pairs that have orthologous phenotypes.
Mapping MPO to UMLS concepts and HPO terms
The extracted MPO terms and synonyms were uploaded into the MetaMap batch mode module. MetaMap (18) is a software program that takes free text and generates a list of potentially matching concepts from the UMLS metathesaurus. We used an online version of MetaMap, available as part of the Semantic Knowledge Representation project (http://skr.nlm.nih.gov/), which aims to provide a framework for exploiting the UMLS knowledge resources for natural language processing. The MetaMap output was parsed using ‘JAVA’ scripts, and the results were stored in an ORACLE relational database. This parser extracts the score for each match (a score of 1000 indicates a perfect score representing the best match between the submitted term and the UMLS concept), the original textual phrase (e.g. MPO term in this case), mapped CUI and the semantic type it belongs to. To avoid potential erroneous mappings, the UMLS Semantic Network was used to restrict the mappings belonging only to the 12 semantic types under the semantic group ‘Disorder’ from the UMLS metathesaurus. Prior to mapping to the UMLS concepts, we also normalized the MPO terms for obtaining optimal matches. The MPO has 33 root nodes or sub-ontologies (most of them representing individual body systems), and submitting these 33 terms as it is did not yield any UMLS concepts from the semantic type ‘Disorder.’ For instance, when submitted to the MetaMap, the term ‘cardiovascular system phenotype’, one of the 33 MPO ontology root nodes, did not match any UMLS concept of the semantic type ‘Disorder’. However, when we modified this term, replacing the suffix ‘phenotype’ with suffixes ‘abnormality’ and ‘disorder’ separately (e.g. ‘cardiovascular abnormality’ and ‘cardiovascular disorder’), we were able to map these terms to UMLS CUIs C0243050 and C0007222 (semantic type ‘Disease and Syndrome’), respectively. There were some obvious non-hits representing phenotypes specific to mouse (e.g. ‘kinked tail,’ ‘long tail,’ ‘curly vibrissae’), and these terms were ignored.
A total of 3780 (∼54%) MPO terms were mapped to unique UMLS CUIs of the semantic group ‘Disorder’ with different scores. Table 1 provides the percentage of MPO terms mapped to different UMLS semantic types, the range of scores for each of the 33 principal root nodes, and the children terms in the MPO. 415 (∼6%) MPO terms were mapped to more than one UMLS CUI.
Details of MPO to UMLS CUI mapping using MetaMap, a software program that takes free text and generates a list of potentially matching concepts with scores (ranging from 0 to 1000 with 1000 being the best score) from the UMLS metathesaurus
Root MPO ID . | Root MPO term . | Number of children terms . | Percentage of children terms mapped . | Percentage of mapped MPO terms with MetaMap scores (1000 = perfect score) . | |||
---|---|---|---|---|---|---|---|
1000 . | 800–999 . | 600–799 . | <600 . | ||||
MP:0003631 | Nervous system phenotype | 1027 | 50 | 34 | 12 | 4 | 0 |
MP:0005387 | Immune system phenotype | 913 | 42 | 28 | 10 | 4 | 0 |
MP:0005389 | Reproductive system phenotype | 549 | 53 | 38 | 10 | 5 | 0 |
MP:0005376 | Homeostasis/metabolism phenotype | 450 | 42 | 22 | 7 | 13 | 0 |
MP:0005385 | Cardiovascular system phenotype | 434 | 66 | 44 | 19 | 3 | 0 |
MP:0005381 | Digestive/alimentary phenotype | 369 | 63 | 44 | 14 | 4 | 0 |
MP:0005382 | Craniofacial phenotype | 352 | 59 | 45 | 11 | 1 | 2 |
MP:0005386 | Behavior/neurological phenotype | 282 | 62 | 31 | 28 | 2 | 0 |
MP:0005393 | Skin/coat/nails phenotype | 261 | 53 | 33 | 17 | 3 | 0 |
MP:0005391 | Vision/eye phenotype | 243 | 72 | 55 | 15 | 2 | 0 |
MP:0005390 | Skeleton phenotype | 237 | 56 | 39 | 14 | 3 | 0 |
MP:0005377 | Hearing/vestibular/ear phenotype | 222 | 46 | 26 | 13 | 5 | 3 |
MP:0005367 | Renal/urinary system phenotype | 191 | 64 | 44 | 15 | 5 | 1 |
MP:0002006 | Tumorigenesis | 174 | 91 | 75 | 16 | 1 | 0 |
MP:0005388 | Respiratory system phenotype | 170 | 61 | 45 | 12 | 4 | 0 |
MP:0005380 | Embryogenesis phenotype | 168 | 35 | 17 | 12 | 7 | 0 |
MP:0005371 | Limbs/digits/tail phenotype | 163 | 55 | 36 | 17 | 3 | 0 |
MP:0005369 | Muscle phenotype | 137 | 55 | 26 | 27 | 1 | 0 |
MP:0005384 | Cellular phenotype | 116 | 34 | 19 | 14 | 1 | 0 |
MP:0005397 | Hematopoietic system phenotype | 103 | 64 | 44 | 13 | 8 | 0 |
MP:0005370 | Liver/biliary system phenotype | 91 | 71 | 60 | 10 | 1 | 0 |
MP:0005375 | Adipose tissue phenotype | 90 | 34 | 16 | 16 | 3 | 0 |
MP:0005379 | Endocrine/exocrine gland phenotype | 85 | 59 | 51 | 6 | 2 | 0 |
MP:0005378 | Growth/size phenotype | 71 | 62 | 38 | 24 | 0 | 0 |
MP:0005394 | Taste/olfaction phenotype | 15 | 73 | 33 | 0 | 40 | 0 |
MP:0005392 | Touch/vibrissae phenotype | 13 | 92 | 8 | 46 | 38 | 0 |
MP:0001186 | Pigmentation phenotype | 12 | 75 | 42 | 33 | 0 | 0 |
MP:0005374 | Lethality-prenatal/perinatal | 11 | 82 | 18 | 64 | 0 | 0 |
MP:0005395 | Other phenotype | 10 | 40 | 40 | 0 | 0 | 0 |
MP:0005372 | Life span-post-weaning/aging | 10 | 30 | 20 | 10 | 0 | 0 |
MP:0002873 | Normal phenotype | 5 | 0 | 0 | 0 | 0 | 0 |
MP:0005373 | Lethality-postnatal | 3 | 67 | 33 | 0 | 33 | 0 |
MP:0003012 | No phenotypic analysis | 1 | 0 | 0 | 0 | 0 | 0 |
Root MPO ID . | Root MPO term . | Number of children terms . | Percentage of children terms mapped . | Percentage of mapped MPO terms with MetaMap scores (1000 = perfect score) . | |||
---|---|---|---|---|---|---|---|
1000 . | 800–999 . | 600–799 . | <600 . | ||||
MP:0003631 | Nervous system phenotype | 1027 | 50 | 34 | 12 | 4 | 0 |
MP:0005387 | Immune system phenotype | 913 | 42 | 28 | 10 | 4 | 0 |
MP:0005389 | Reproductive system phenotype | 549 | 53 | 38 | 10 | 5 | 0 |
MP:0005376 | Homeostasis/metabolism phenotype | 450 | 42 | 22 | 7 | 13 | 0 |
MP:0005385 | Cardiovascular system phenotype | 434 | 66 | 44 | 19 | 3 | 0 |
MP:0005381 | Digestive/alimentary phenotype | 369 | 63 | 44 | 14 | 4 | 0 |
MP:0005382 | Craniofacial phenotype | 352 | 59 | 45 | 11 | 1 | 2 |
MP:0005386 | Behavior/neurological phenotype | 282 | 62 | 31 | 28 | 2 | 0 |
MP:0005393 | Skin/coat/nails phenotype | 261 | 53 | 33 | 17 | 3 | 0 |
MP:0005391 | Vision/eye phenotype | 243 | 72 | 55 | 15 | 2 | 0 |
MP:0005390 | Skeleton phenotype | 237 | 56 | 39 | 14 | 3 | 0 |
MP:0005377 | Hearing/vestibular/ear phenotype | 222 | 46 | 26 | 13 | 5 | 3 |
MP:0005367 | Renal/urinary system phenotype | 191 | 64 | 44 | 15 | 5 | 1 |
MP:0002006 | Tumorigenesis | 174 | 91 | 75 | 16 | 1 | 0 |
MP:0005388 | Respiratory system phenotype | 170 | 61 | 45 | 12 | 4 | 0 |
MP:0005380 | Embryogenesis phenotype | 168 | 35 | 17 | 12 | 7 | 0 |
MP:0005371 | Limbs/digits/tail phenotype | 163 | 55 | 36 | 17 | 3 | 0 |
MP:0005369 | Muscle phenotype | 137 | 55 | 26 | 27 | 1 | 0 |
MP:0005384 | Cellular phenotype | 116 | 34 | 19 | 14 | 1 | 0 |
MP:0005397 | Hematopoietic system phenotype | 103 | 64 | 44 | 13 | 8 | 0 |
MP:0005370 | Liver/biliary system phenotype | 91 | 71 | 60 | 10 | 1 | 0 |
MP:0005375 | Adipose tissue phenotype | 90 | 34 | 16 | 16 | 3 | 0 |
MP:0005379 | Endocrine/exocrine gland phenotype | 85 | 59 | 51 | 6 | 2 | 0 |
MP:0005378 | Growth/size phenotype | 71 | 62 | 38 | 24 | 0 | 0 |
MP:0005394 | Taste/olfaction phenotype | 15 | 73 | 33 | 0 | 40 | 0 |
MP:0005392 | Touch/vibrissae phenotype | 13 | 92 | 8 | 46 | 38 | 0 |
MP:0001186 | Pigmentation phenotype | 12 | 75 | 42 | 33 | 0 | 0 |
MP:0005374 | Lethality-prenatal/perinatal | 11 | 82 | 18 | 64 | 0 | 0 |
MP:0005395 | Other phenotype | 10 | 40 | 40 | 0 | 0 | 0 |
MP:0005372 | Life span-post-weaning/aging | 10 | 30 | 20 | 10 | 0 | 0 |
MP:0002873 | Normal phenotype | 5 | 0 | 0 | 0 | 0 | 0 |
MP:0005373 | Lethality-postnatal | 3 | 67 | 33 | 0 | 33 | 0 |
MP:0003012 | No phenotypic analysis | 1 | 0 | 0 | 0 | 0 | 0 |
Details of MPO to UMLS CUI mapping using MetaMap, a software program that takes free text and generates a list of potentially matching concepts with scores (ranging from 0 to 1000 with 1000 being the best score) from the UMLS metathesaurus
Root MPO ID . | Root MPO term . | Number of children terms . | Percentage of children terms mapped . | Percentage of mapped MPO terms with MetaMap scores (1000 = perfect score) . | |||
---|---|---|---|---|---|---|---|
1000 . | 800–999 . | 600–799 . | <600 . | ||||
MP:0003631 | Nervous system phenotype | 1027 | 50 | 34 | 12 | 4 | 0 |
MP:0005387 | Immune system phenotype | 913 | 42 | 28 | 10 | 4 | 0 |
MP:0005389 | Reproductive system phenotype | 549 | 53 | 38 | 10 | 5 | 0 |
MP:0005376 | Homeostasis/metabolism phenotype | 450 | 42 | 22 | 7 | 13 | 0 |
MP:0005385 | Cardiovascular system phenotype | 434 | 66 | 44 | 19 | 3 | 0 |
MP:0005381 | Digestive/alimentary phenotype | 369 | 63 | 44 | 14 | 4 | 0 |
MP:0005382 | Craniofacial phenotype | 352 | 59 | 45 | 11 | 1 | 2 |
MP:0005386 | Behavior/neurological phenotype | 282 | 62 | 31 | 28 | 2 | 0 |
MP:0005393 | Skin/coat/nails phenotype | 261 | 53 | 33 | 17 | 3 | 0 |
MP:0005391 | Vision/eye phenotype | 243 | 72 | 55 | 15 | 2 | 0 |
MP:0005390 | Skeleton phenotype | 237 | 56 | 39 | 14 | 3 | 0 |
MP:0005377 | Hearing/vestibular/ear phenotype | 222 | 46 | 26 | 13 | 5 | 3 |
MP:0005367 | Renal/urinary system phenotype | 191 | 64 | 44 | 15 | 5 | 1 |
MP:0002006 | Tumorigenesis | 174 | 91 | 75 | 16 | 1 | 0 |
MP:0005388 | Respiratory system phenotype | 170 | 61 | 45 | 12 | 4 | 0 |
MP:0005380 | Embryogenesis phenotype | 168 | 35 | 17 | 12 | 7 | 0 |
MP:0005371 | Limbs/digits/tail phenotype | 163 | 55 | 36 | 17 | 3 | 0 |
MP:0005369 | Muscle phenotype | 137 | 55 | 26 | 27 | 1 | 0 |
MP:0005384 | Cellular phenotype | 116 | 34 | 19 | 14 | 1 | 0 |
MP:0005397 | Hematopoietic system phenotype | 103 | 64 | 44 | 13 | 8 | 0 |
MP:0005370 | Liver/biliary system phenotype | 91 | 71 | 60 | 10 | 1 | 0 |
MP:0005375 | Adipose tissue phenotype | 90 | 34 | 16 | 16 | 3 | 0 |
MP:0005379 | Endocrine/exocrine gland phenotype | 85 | 59 | 51 | 6 | 2 | 0 |
MP:0005378 | Growth/size phenotype | 71 | 62 | 38 | 24 | 0 | 0 |
MP:0005394 | Taste/olfaction phenotype | 15 | 73 | 33 | 0 | 40 | 0 |
MP:0005392 | Touch/vibrissae phenotype | 13 | 92 | 8 | 46 | 38 | 0 |
MP:0001186 | Pigmentation phenotype | 12 | 75 | 42 | 33 | 0 | 0 |
MP:0005374 | Lethality-prenatal/perinatal | 11 | 82 | 18 | 64 | 0 | 0 |
MP:0005395 | Other phenotype | 10 | 40 | 40 | 0 | 0 | 0 |
MP:0005372 | Life span-post-weaning/aging | 10 | 30 | 20 | 10 | 0 | 0 |
MP:0002873 | Normal phenotype | 5 | 0 | 0 | 0 | 0 | 0 |
MP:0005373 | Lethality-postnatal | 3 | 67 | 33 | 0 | 33 | 0 |
MP:0003012 | No phenotypic analysis | 1 | 0 | 0 | 0 | 0 | 0 |
Root MPO ID . | Root MPO term . | Number of children terms . | Percentage of children terms mapped . | Percentage of mapped MPO terms with MetaMap scores (1000 = perfect score) . | |||
---|---|---|---|---|---|---|---|
1000 . | 800–999 . | 600–799 . | <600 . | ||||
MP:0003631 | Nervous system phenotype | 1027 | 50 | 34 | 12 | 4 | 0 |
MP:0005387 | Immune system phenotype | 913 | 42 | 28 | 10 | 4 | 0 |
MP:0005389 | Reproductive system phenotype | 549 | 53 | 38 | 10 | 5 | 0 |
MP:0005376 | Homeostasis/metabolism phenotype | 450 | 42 | 22 | 7 | 13 | 0 |
MP:0005385 | Cardiovascular system phenotype | 434 | 66 | 44 | 19 | 3 | 0 |
MP:0005381 | Digestive/alimentary phenotype | 369 | 63 | 44 | 14 | 4 | 0 |
MP:0005382 | Craniofacial phenotype | 352 | 59 | 45 | 11 | 1 | 2 |
MP:0005386 | Behavior/neurological phenotype | 282 | 62 | 31 | 28 | 2 | 0 |
MP:0005393 | Skin/coat/nails phenotype | 261 | 53 | 33 | 17 | 3 | 0 |
MP:0005391 | Vision/eye phenotype | 243 | 72 | 55 | 15 | 2 | 0 |
MP:0005390 | Skeleton phenotype | 237 | 56 | 39 | 14 | 3 | 0 |
MP:0005377 | Hearing/vestibular/ear phenotype | 222 | 46 | 26 | 13 | 5 | 3 |
MP:0005367 | Renal/urinary system phenotype | 191 | 64 | 44 | 15 | 5 | 1 |
MP:0002006 | Tumorigenesis | 174 | 91 | 75 | 16 | 1 | 0 |
MP:0005388 | Respiratory system phenotype | 170 | 61 | 45 | 12 | 4 | 0 |
MP:0005380 | Embryogenesis phenotype | 168 | 35 | 17 | 12 | 7 | 0 |
MP:0005371 | Limbs/digits/tail phenotype | 163 | 55 | 36 | 17 | 3 | 0 |
MP:0005369 | Muscle phenotype | 137 | 55 | 26 | 27 | 1 | 0 |
MP:0005384 | Cellular phenotype | 116 | 34 | 19 | 14 | 1 | 0 |
MP:0005397 | Hematopoietic system phenotype | 103 | 64 | 44 | 13 | 8 | 0 |
MP:0005370 | Liver/biliary system phenotype | 91 | 71 | 60 | 10 | 1 | 0 |
MP:0005375 | Adipose tissue phenotype | 90 | 34 | 16 | 16 | 3 | 0 |
MP:0005379 | Endocrine/exocrine gland phenotype | 85 | 59 | 51 | 6 | 2 | 0 |
MP:0005378 | Growth/size phenotype | 71 | 62 | 38 | 24 | 0 | 0 |
MP:0005394 | Taste/olfaction phenotype | 15 | 73 | 33 | 0 | 40 | 0 |
MP:0005392 | Touch/vibrissae phenotype | 13 | 92 | 8 | 46 | 38 | 0 |
MP:0001186 | Pigmentation phenotype | 12 | 75 | 42 | 33 | 0 | 0 |
MP:0005374 | Lethality-prenatal/perinatal | 11 | 82 | 18 | 64 | 0 | 0 |
MP:0005395 | Other phenotype | 10 | 40 | 40 | 0 | 0 | 0 |
MP:0005372 | Life span-post-weaning/aging | 10 | 30 | 20 | 10 | 0 | 0 |
MP:0002873 | Normal phenotype | 5 | 0 | 0 | 0 | 0 | 0 |
MP:0005373 | Lethality-postnatal | 3 | 67 | 33 | 0 | 33 | 0 |
MP:0003012 | No phenotypic analysis | 1 | 0 | 0 | 0 | 0 | 0 |
We did not do a direct MPO to HPO mapping but instead used the existing HPO to UMLS mappings (available in the HPO obo file). In other words, if an MPO term and HPO term map to the same UMLS concept, we consider it as a MPO-HPO term match.
Mapping MPO to OMIM
We used NCBI Entrez programming utilities (eUtils) (23) to map the MPO terms to OMIM records. The NCBI eUtils are tools which allow users to access NCBI’s Entrez databases and search and retrieve data from them. The results generated are similar to the results one obtains when querying NCBI databases through web interfaces. We used the eSearch tool from eUtils to map MPO terms to OMIM records and retrieve all mapped OMIM record IDs. We used both the MPO terms and their synonyms as queries. The eUtils Web service accepts a term and returns the associated OMIM IDs. In the preliminary runs, we observed that eUtils performs an exact string-based comparison with the OMIM records using the term submitted. Thus, it fails to accommodate the variations of terms (plurals or synonyms). For instance, the number of hits returned when using queries like ‘eye abnormality’, ‘eye abnormalities’, ‘eye disorder’, ‘eye disorders’, ‘eye defect’, ‘eye defects’ and ‘abnormal eye’ were different. To overcome this limitation, we pre-processed all MPO terms prior to submission to eUtils along the lines described earlier and merged the results obtained for each of the variable queries representing one unique MPO term. Since the eUtils has a restriction on the number of queries (not >3 queries per second), we submitted our requests in batches of three terms at a time. The results (MPO to OMIM mappings) obtained from eUtils were assigned empirical scores based on the context of the MPO term (i.e. its occurrence in a specific section(s) of the mapped OMIM record). If an MPO term was mapped to the ‘Allelic Variant’ section and also to the ‘Clinical Synopsis’ or ‘Clinical Features’ section of the mapped OMIM record, we assigned a perfect score of 1000 (see the ‘Help’ and ‘FAQ’ sections on the PhenoHM home page for additional details of scoring adopted). Simultaneously, we also built a database of all available allelic variants, clinical synopsis, clinical features, pathogenesis and genotype/phenotype correlations in the OMIM records by parsing the OMIM XML files.
Of the MPO terms, ∼64% (4527/6978) were mapped to the OMIM records (see Supplementary Table S1 on the PhenoHM home page for details of MPO terms to OMIM mappings). Of these, for 371 MPO terms we were able to map and extract the human allelic variant information (see Supplementary Table S2 on the PhenoHM home page for a list of MPO terms mapped to human allelic variants from OMIM). As an example, the mammalian phenotype cataract (MP:0001304) from MPO had 81 genes, while there were 360 genes associated with cataract in human (based on all data resources listed previously). Of these, 44 genes were shared (Figure 2D). When we checked OMIM to see how many of these 44 shared genes have a reported mutation in humans implicated or associated with cataract, we found 20 human genes that had reported allelic variants also associated with cataract (Table 2 and Figure 3). We call these 20 genes ortholog genes with ortholog phenotype cataract. In other words, the likelihood of a perturbation of these genes resulting in a conserved phenotype (i.e. similar phenotype in both human and mouse) is high. Since network visualization is more intuitive than tabular data (especially when the data sets are large), we have also provided the option of viewing the orthologous phenotypes along with the human allelic variant information (when available) as a Cytoscape (24) network (see Figure 3 for a network representation of orthologous phenotype cataract). The users can download the corresponding XGMML files from the MPO to OMIM map scoring table and import it into Cytoscape (24).
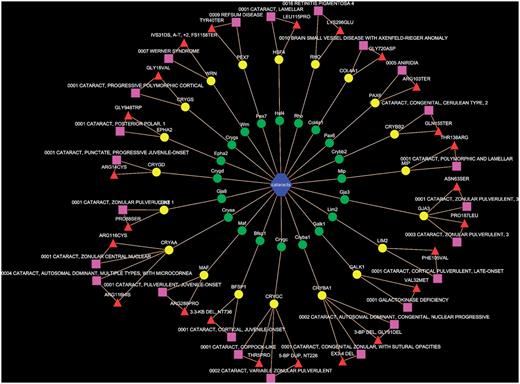
Network representation of orthologous phenotype network of cataract. The green and yellow colored nodes represent the mouse and human genes associated with cataract, respectively. The pink rectangles are the human allelic variants from OMIM, while the red triangles show the implicated mutation in human genes.
Cataract-gene (Mouse) . | OMIM ID . | OMIM title . | Ortholog (human gene) . | OMIM allelic variant . | Mutation (OMIM) . |
---|---|---|---|---|---|
Bfsp1 | 603 307 | Beaded filament structural protein 1; BFSP1 | BFSP1 | 0001 Cataract, cortical, juvenile-onset | 3.3-KB DEL, NT736 |
Col4a1 | 120 130 | Collagen, type IV, alpha-1; COL4A1 | COL4A1 | 0010 Brain small vessel disease with axenfeld-rieger anomaly | GLY720ASP |
Cryaa | 123 580 | Crystallin, alpha-A; CRYAA | CRYAA | 0001 Cataract, zonular central nuclear | ARG116CYS |
Cryaa | 123 580 | Crystallin, alpha-A; CRYAA | CRYAA | 0004 Cataract, autosomal dominant, multiple types, with microcornea | ARG116HIS |
Cryba1 | 123 610 | Crystallin, beta-A1; CRYBA1 | CRYBA1 | 0002 Cataract, autosomal dominant, congenital, nuclear progressive | 3-BP DEL, GLY91DEL |
Cryba1 | 123 610 | Crystallin, beta-A1; CRYBA1 | CRYBA1 | 0001 Cataract, congenital zonular, with sutural opacities | EX3-4 DEL |
Crybb2 | 123 620 | Crystallin, beta-B2; CRYBB2 | CRYBB2 | 0001 Cataract, congenital, cerulean type, 2 | GLN155TER |
Crygc | 123 680 | Crystallin, gamma-C; CRYGC | CRYGC | 0002 Cataract, variable zonular pulverulent | 5-BP DUP, NT226 |
Crygc | 123 680 | Crystallin, gamma-C; CRYGC | CRYGC | 0001 Cataract, coppock-like | THR5PRO |
Crygd | 123 690 | Crystallin, gamma-D; CRYGD | CRYGD | 0001 Cataract, punctate, progressive juvenile-onset | ARG14CYS |
Crygs | 123 730 | Crystallin, gamma-S; CRYGS | CRYGS | 0001 Cataract, progressive polymorphic cortical | GLY18VAL |
Epha2 | 176 946 | Ephrin receptor EphA2; EPHA2 | EPHA2 | 0001 Cataract, posterior polar, 1 | GLY948TRP |
Galk1 | 604 313 | Galactokinase 1; GALK1 | GALK1 | 0001 Galactokinase deficiency | VAL32MET |
Gja3 | 121 015 | Gap junction protein, alpha-3; GJA3 | GJA3 | 0001 Cataract, zonular pulverulent, 3 | ASN63SER |
Gja3 | 121 015 | Gap junction protein, alpha-3; GJA3 | GJA3 | 0003 Cataract, zonular pulverulent, 3 | PRO187LEU |
Gja8 | 600 897 | Gap junction protein, alpha-8; GJA8 | GJA8 | 0001 Cataract, zonular pulverulent 1 | PRO88SER |
Hsf4 | 602 438 | Heat-shock transcription factor 4; HSF4 | HSF4 | 0001 Cataract, lamellar | LEU115PRO |
Lim2 | 154 045 | Lens intrinsic membrane protein 2, 19-KD; LIM2 | LIM2 | 0001 Cataract, cortical pulverulent, late-onset | PHE105VAL |
Maf | 177 075 | V-MAF avian musculoaponeurotic fibrosarcoma oncogene homolog; MAF | MAF | 0001 Cataract, pulverulent, juvenile-onset | ARG288PRO |
Mip | 154 050 | Major intrinsic protein of lens fiber; MIP | MIP | 0001 Cataract, polymorphic and lamellar | THR138ARG |
Pax6 | 607 108 | Paired box gene 6; PAX6 | PAX6 | 0005 Aniridia | ARG103TER |
Pex7 | 601 757 | Peroxisome biogenesis factor 7; PEX7 | PEX7 | 0009 Refsum disease | TYR40TER |
Rho | 180 380 | Rhodopsin; RHO | RHO | 0016 retinitis pigmentosa 4 | LYS296GLU |
Wrn | 604 611 | RECQ protein-like 2; RECQL2 | WRN | 0007 Werner syndrome | IVS31DS, A-T, +2, FS1158TER |
Cataract-gene (Mouse) . | OMIM ID . | OMIM title . | Ortholog (human gene) . | OMIM allelic variant . | Mutation (OMIM) . |
---|---|---|---|---|---|
Bfsp1 | 603 307 | Beaded filament structural protein 1; BFSP1 | BFSP1 | 0001 Cataract, cortical, juvenile-onset | 3.3-KB DEL, NT736 |
Col4a1 | 120 130 | Collagen, type IV, alpha-1; COL4A1 | COL4A1 | 0010 Brain small vessel disease with axenfeld-rieger anomaly | GLY720ASP |
Cryaa | 123 580 | Crystallin, alpha-A; CRYAA | CRYAA | 0001 Cataract, zonular central nuclear | ARG116CYS |
Cryaa | 123 580 | Crystallin, alpha-A; CRYAA | CRYAA | 0004 Cataract, autosomal dominant, multiple types, with microcornea | ARG116HIS |
Cryba1 | 123 610 | Crystallin, beta-A1; CRYBA1 | CRYBA1 | 0002 Cataract, autosomal dominant, congenital, nuclear progressive | 3-BP DEL, GLY91DEL |
Cryba1 | 123 610 | Crystallin, beta-A1; CRYBA1 | CRYBA1 | 0001 Cataract, congenital zonular, with sutural opacities | EX3-4 DEL |
Crybb2 | 123 620 | Crystallin, beta-B2; CRYBB2 | CRYBB2 | 0001 Cataract, congenital, cerulean type, 2 | GLN155TER |
Crygc | 123 680 | Crystallin, gamma-C; CRYGC | CRYGC | 0002 Cataract, variable zonular pulverulent | 5-BP DUP, NT226 |
Crygc | 123 680 | Crystallin, gamma-C; CRYGC | CRYGC | 0001 Cataract, coppock-like | THR5PRO |
Crygd | 123 690 | Crystallin, gamma-D; CRYGD | CRYGD | 0001 Cataract, punctate, progressive juvenile-onset | ARG14CYS |
Crygs | 123 730 | Crystallin, gamma-S; CRYGS | CRYGS | 0001 Cataract, progressive polymorphic cortical | GLY18VAL |
Epha2 | 176 946 | Ephrin receptor EphA2; EPHA2 | EPHA2 | 0001 Cataract, posterior polar, 1 | GLY948TRP |
Galk1 | 604 313 | Galactokinase 1; GALK1 | GALK1 | 0001 Galactokinase deficiency | VAL32MET |
Gja3 | 121 015 | Gap junction protein, alpha-3; GJA3 | GJA3 | 0001 Cataract, zonular pulverulent, 3 | ASN63SER |
Gja3 | 121 015 | Gap junction protein, alpha-3; GJA3 | GJA3 | 0003 Cataract, zonular pulverulent, 3 | PRO187LEU |
Gja8 | 600 897 | Gap junction protein, alpha-8; GJA8 | GJA8 | 0001 Cataract, zonular pulverulent 1 | PRO88SER |
Hsf4 | 602 438 | Heat-shock transcription factor 4; HSF4 | HSF4 | 0001 Cataract, lamellar | LEU115PRO |
Lim2 | 154 045 | Lens intrinsic membrane protein 2, 19-KD; LIM2 | LIM2 | 0001 Cataract, cortical pulverulent, late-onset | PHE105VAL |
Maf | 177 075 | V-MAF avian musculoaponeurotic fibrosarcoma oncogene homolog; MAF | MAF | 0001 Cataract, pulverulent, juvenile-onset | ARG288PRO |
Mip | 154 050 | Major intrinsic protein of lens fiber; MIP | MIP | 0001 Cataract, polymorphic and lamellar | THR138ARG |
Pax6 | 607 108 | Paired box gene 6; PAX6 | PAX6 | 0005 Aniridia | ARG103TER |
Pex7 | 601 757 | Peroxisome biogenesis factor 7; PEX7 | PEX7 | 0009 Refsum disease | TYR40TER |
Rho | 180 380 | Rhodopsin; RHO | RHO | 0016 retinitis pigmentosa 4 | LYS296GLU |
Wrn | 604 611 | RECQ protein-like 2; RECQL2 | WRN | 0007 Werner syndrome | IVS31DS, A-T, +2, FS1158TER |
Out of 81 known mouse genes associated with cataract, 44 human orthologs were also associated with cataract. Of these, 20 genes have OMIM allelic variants that are cataract related.
Cataract-gene (Mouse) . | OMIM ID . | OMIM title . | Ortholog (human gene) . | OMIM allelic variant . | Mutation (OMIM) . |
---|---|---|---|---|---|
Bfsp1 | 603 307 | Beaded filament structural protein 1; BFSP1 | BFSP1 | 0001 Cataract, cortical, juvenile-onset | 3.3-KB DEL, NT736 |
Col4a1 | 120 130 | Collagen, type IV, alpha-1; COL4A1 | COL4A1 | 0010 Brain small vessel disease with axenfeld-rieger anomaly | GLY720ASP |
Cryaa | 123 580 | Crystallin, alpha-A; CRYAA | CRYAA | 0001 Cataract, zonular central nuclear | ARG116CYS |
Cryaa | 123 580 | Crystallin, alpha-A; CRYAA | CRYAA | 0004 Cataract, autosomal dominant, multiple types, with microcornea | ARG116HIS |
Cryba1 | 123 610 | Crystallin, beta-A1; CRYBA1 | CRYBA1 | 0002 Cataract, autosomal dominant, congenital, nuclear progressive | 3-BP DEL, GLY91DEL |
Cryba1 | 123 610 | Crystallin, beta-A1; CRYBA1 | CRYBA1 | 0001 Cataract, congenital zonular, with sutural opacities | EX3-4 DEL |
Crybb2 | 123 620 | Crystallin, beta-B2; CRYBB2 | CRYBB2 | 0001 Cataract, congenital, cerulean type, 2 | GLN155TER |
Crygc | 123 680 | Crystallin, gamma-C; CRYGC | CRYGC | 0002 Cataract, variable zonular pulverulent | 5-BP DUP, NT226 |
Crygc | 123 680 | Crystallin, gamma-C; CRYGC | CRYGC | 0001 Cataract, coppock-like | THR5PRO |
Crygd | 123 690 | Crystallin, gamma-D; CRYGD | CRYGD | 0001 Cataract, punctate, progressive juvenile-onset | ARG14CYS |
Crygs | 123 730 | Crystallin, gamma-S; CRYGS | CRYGS | 0001 Cataract, progressive polymorphic cortical | GLY18VAL |
Epha2 | 176 946 | Ephrin receptor EphA2; EPHA2 | EPHA2 | 0001 Cataract, posterior polar, 1 | GLY948TRP |
Galk1 | 604 313 | Galactokinase 1; GALK1 | GALK1 | 0001 Galactokinase deficiency | VAL32MET |
Gja3 | 121 015 | Gap junction protein, alpha-3; GJA3 | GJA3 | 0001 Cataract, zonular pulverulent, 3 | ASN63SER |
Gja3 | 121 015 | Gap junction protein, alpha-3; GJA3 | GJA3 | 0003 Cataract, zonular pulverulent, 3 | PRO187LEU |
Gja8 | 600 897 | Gap junction protein, alpha-8; GJA8 | GJA8 | 0001 Cataract, zonular pulverulent 1 | PRO88SER |
Hsf4 | 602 438 | Heat-shock transcription factor 4; HSF4 | HSF4 | 0001 Cataract, lamellar | LEU115PRO |
Lim2 | 154 045 | Lens intrinsic membrane protein 2, 19-KD; LIM2 | LIM2 | 0001 Cataract, cortical pulverulent, late-onset | PHE105VAL |
Maf | 177 075 | V-MAF avian musculoaponeurotic fibrosarcoma oncogene homolog; MAF | MAF | 0001 Cataract, pulverulent, juvenile-onset | ARG288PRO |
Mip | 154 050 | Major intrinsic protein of lens fiber; MIP | MIP | 0001 Cataract, polymorphic and lamellar | THR138ARG |
Pax6 | 607 108 | Paired box gene 6; PAX6 | PAX6 | 0005 Aniridia | ARG103TER |
Pex7 | 601 757 | Peroxisome biogenesis factor 7; PEX7 | PEX7 | 0009 Refsum disease | TYR40TER |
Rho | 180 380 | Rhodopsin; RHO | RHO | 0016 retinitis pigmentosa 4 | LYS296GLU |
Wrn | 604 611 | RECQ protein-like 2; RECQL2 | WRN | 0007 Werner syndrome | IVS31DS, A-T, +2, FS1158TER |
Cataract-gene (Mouse) . | OMIM ID . | OMIM title . | Ortholog (human gene) . | OMIM allelic variant . | Mutation (OMIM) . |
---|---|---|---|---|---|
Bfsp1 | 603 307 | Beaded filament structural protein 1; BFSP1 | BFSP1 | 0001 Cataract, cortical, juvenile-onset | 3.3-KB DEL, NT736 |
Col4a1 | 120 130 | Collagen, type IV, alpha-1; COL4A1 | COL4A1 | 0010 Brain small vessel disease with axenfeld-rieger anomaly | GLY720ASP |
Cryaa | 123 580 | Crystallin, alpha-A; CRYAA | CRYAA | 0001 Cataract, zonular central nuclear | ARG116CYS |
Cryaa | 123 580 | Crystallin, alpha-A; CRYAA | CRYAA | 0004 Cataract, autosomal dominant, multiple types, with microcornea | ARG116HIS |
Cryba1 | 123 610 | Crystallin, beta-A1; CRYBA1 | CRYBA1 | 0002 Cataract, autosomal dominant, congenital, nuclear progressive | 3-BP DEL, GLY91DEL |
Cryba1 | 123 610 | Crystallin, beta-A1; CRYBA1 | CRYBA1 | 0001 Cataract, congenital zonular, with sutural opacities | EX3-4 DEL |
Crybb2 | 123 620 | Crystallin, beta-B2; CRYBB2 | CRYBB2 | 0001 Cataract, congenital, cerulean type, 2 | GLN155TER |
Crygc | 123 680 | Crystallin, gamma-C; CRYGC | CRYGC | 0002 Cataract, variable zonular pulverulent | 5-BP DUP, NT226 |
Crygc | 123 680 | Crystallin, gamma-C; CRYGC | CRYGC | 0001 Cataract, coppock-like | THR5PRO |
Crygd | 123 690 | Crystallin, gamma-D; CRYGD | CRYGD | 0001 Cataract, punctate, progressive juvenile-onset | ARG14CYS |
Crygs | 123 730 | Crystallin, gamma-S; CRYGS | CRYGS | 0001 Cataract, progressive polymorphic cortical | GLY18VAL |
Epha2 | 176 946 | Ephrin receptor EphA2; EPHA2 | EPHA2 | 0001 Cataract, posterior polar, 1 | GLY948TRP |
Galk1 | 604 313 | Galactokinase 1; GALK1 | GALK1 | 0001 Galactokinase deficiency | VAL32MET |
Gja3 | 121 015 | Gap junction protein, alpha-3; GJA3 | GJA3 | 0001 Cataract, zonular pulverulent, 3 | ASN63SER |
Gja3 | 121 015 | Gap junction protein, alpha-3; GJA3 | GJA3 | 0003 Cataract, zonular pulverulent, 3 | PRO187LEU |
Gja8 | 600 897 | Gap junction protein, alpha-8; GJA8 | GJA8 | 0001 Cataract, zonular pulverulent 1 | PRO88SER |
Hsf4 | 602 438 | Heat-shock transcription factor 4; HSF4 | HSF4 | 0001 Cataract, lamellar | LEU115PRO |
Lim2 | 154 045 | Lens intrinsic membrane protein 2, 19-KD; LIM2 | LIM2 | 0001 Cataract, cortical pulverulent, late-onset | PHE105VAL |
Maf | 177 075 | V-MAF avian musculoaponeurotic fibrosarcoma oncogene homolog; MAF | MAF | 0001 Cataract, pulverulent, juvenile-onset | ARG288PRO |
Mip | 154 050 | Major intrinsic protein of lens fiber; MIP | MIP | 0001 Cataract, polymorphic and lamellar | THR138ARG |
Pax6 | 607 108 | Paired box gene 6; PAX6 | PAX6 | 0005 Aniridia | ARG103TER |
Pex7 | 601 757 | Peroxisome biogenesis factor 7; PEX7 | PEX7 | 0009 Refsum disease | TYR40TER |
Rho | 180 380 | Rhodopsin; RHO | RHO | 0016 retinitis pigmentosa 4 | LYS296GLU |
Wrn | 604 611 | RECQ protein-like 2; RECQL2 | WRN | 0007 Werner syndrome | IVS31DS, A-T, +2, FS1158TER |
Out of 81 known mouse genes associated with cataract, 44 human orthologs were also associated with cataract. Of these, 20 genes have OMIM allelic variants that are cataract related.
IMPLEMENTATION AND ACCESS
We used the ‘JAVA’ 1.6 programming platform for our database uploads. An open source ‘JAVA’ SDK called ‘Eclipse’ (http://www.eclipse.org) was used as an IDE for writing programs. Tomcat Apache v6.0 (http://httpd.apache.org) was used as the web server. The PhenoHM server was implemented as a ‘JAVA’ web application using ‘JAVA’ servlets and JSPs. JavaScript, along with the Prototype JS framework 1.6.0.2 (http://www.prototypejs.org) was used for building the client-side functionalities. We maintain our data as two sets of Oracle 10 g Enterprise Edition Release 10.2.0.3 relational databases. The production database is stored on the same computer as the web server. However, the development database is stored separately. The data loads and refreshes are first performed on the development server, and after testing the data is transferred to the production database. All the data loads are refreshed at regular intervals of time to keep the data current.
UTILITY
One of the principal motivations for the current study is to facilitate the comparison of phenotypic knowledge about genes and gene products across human and mouse. Thus using the PhenoHM server, it is possible to query for genes and gene products across mouse and human based on MPO terms or disease concepts from UMLS or HP terms from HPO or OMIM. Additionally, where available, the human allelic variant information from OMIM is also included in the ortholog phenotype reports. As evidenced in our mouse–human phenotype mapping examples, there are several other mouse genes with a known human ortholog but where the phenotype has only been observed for mouse mutants and has not yet been associated with the human counterpart. Alternately, there are several human genes that are associated with a particular clinical phenotype but for which there is no known association for alleles of these genes in mouse. On the Supplementary section of PhenoHM homepage, we have included several examples with step-wise instructions to demonstrate the utility and contents of PhenoHM server.
RELATED WORK
Recently, in a pioneering study, Burgun et al. (25) developed a terminology to map phenotypes from the MPO to the OMIM through the UMLS. Our current study differs from Burgun et al. (25) in two principal aspects: (i) we map the MPO terminology directly to OMIM records and score the mappings based on their context or occurrence within the OMIM records and (ii) for the mapped OMIM records, we extract the corresponding human allelic variant information. Additionally, through the PhenoHM server we have made the mouse–human phenotype mappings along with their annotated genes available as a mineable resource. OrthoDisease (9) and PhenomicDB (8,26) are two other resources that allow researchers to look simultaneously at all available phenotypes for an orthologous gene group. The PhenomicDB and OrthoDisease are useful resources integrating the phenotypes with the homologous genes from a variety of species. However, unlike our PhenoHM server, PhenomicDB or OrthoDisease do not indicate the likelihood a phenotype is shared by the orthologous genes. For a queried phenotype term, PhenomicDB returns all available homologous genes along with their associated phenotypes. On the other hand, OrthoDisease only enlists potential homolog genes for human disease without any phenotype details in the homologs. Further, we observed that OrthoDisease is disease-centric and does not support most of the phenotype queries. For instance, a search for phenotype terms like dextrocardia or blepharitis did not return any records in OrthoDisease. Additionally, neither of these two databases addresses the issue of bridging the gap between the phenotype terminology (from model organisms and also human e.g. MPO or HPO) and clinical terminology (e.g. UMLS concepts). Although our PhenoHM server is an effort in this direction, challenges remain in mapping the ortholog phenotypes underlying multi-factorial diseases and non-Mendelian diseases and in identifying the causal candidate genes for diseases by extrapolating the gene–phenotype information from other model organisms to humans and vice versa. Thus, there is still a need for improvement of comparative phenomics based approaches, especially because majority of the human disease are known to be multi-factorial.
PERSPECTIVES
Although we perceive PhenoHM as a first step toward comparative phenomics that combines knowledge about phenotype and implicated genes from human and mouse, we had to compromise between data depth as available in the source databases and data compatibility. For instance, at the time of this manuscript preparation, the human allelic variant information is available for only ∼12% (2339/19877) of all OMIM records. Likewise, the phenotype data for mouse is available for <25% of known mouse genes. Given the limited throughput of existing laboratory-based phenotyping methods, web-based ascertainment and cross-species comparative phenomic strategies may represent the most rational way forward for prioritizing the genes for further experimental or clinical studies (27). With declining costs and advancing technologies in the post-GWAS era, it is highly likely that finer mapping of genetic sequences, and detection of rare variants and copy number variations hitherto unrevealed by most current platforms will be possible [e.g. EuroPhenome (28), a comprehensive resource for raw and annotated high-throughput phenotyping data]. Finally, the intention of PhenoHM is not to compete with the much more dedicated and detailed primary source databases of phenotypes but to provide an effective integrated meta-search server facilitating human–mouse comparative phenomics. Even though the current version focuses on phenotype annotations of human and mouse genes, our PhenoHM server can easily be extended to other species in future.
CONCLUSIONS
Currently, our ability to study the molecular basis of disease is hugely aided by aggregating all available genetic and phenotypic similarities between disease entities, their associated phenotypes and known genetic causes or modifiers of the disease or phenotype. Because of the complexities and variabilities associated with searching different phenotype and disease databases, we have developed a resource that allows extraction of disease–gene homologs based on the concept of reciprocally mapped comparative genomics and phenomics. We have thus applied fine-mapping techniques between human and mouse genetic disease phenotypes to identify ‘conserved phenotypes’ or ‘orthologous phenotypes’ to facilitate the undertaking of comparative phenomics. The phenotype mapping details range from terminology mapping to extraction of ortholog genes with orthologous phenotypes and the associated mutations when available. The PhenoHM matrix has a number of characteristics that suggest it might be a useful addition to more specialized or unidirectional phenotype-centered data sources like the MGI and the UMLS. Here we have used MPO and UMLS and HPO for this initial analysis because they are still by far the most comprehensive of available phenotype databases for mouse and human. Finally, the ultimate use and test of human–mouse comparative phenomics and of the identification of orthologous phenotypes such as proposed here, will be whether they expedite the discovery of clinical targets for molecular therapies and pave the way for novel diagnostic and therapeutic approaches.
FUNDING
National Institutes of Health/National Institute of Diabetes and Digestive and Kidney Diseases (NIH/NIDDK), Murine Atlas of a Genitourinary Development Molecular Anatomy Project (1U01 DK70219); Cincinnati Digestive Health Sciences Center (PHS Grant P30 DK078392); CTSA: Cincinnati Center for Clinical and Translational Sciences (U54 RR025216); FACEBASE Consortium (U01DE020049 NIDCR). Funding for open access charge: Faculty discretionary funds from CCHMC, Cincinnati, Ohio.
Conflict of interest statement. None declared.
ACKNOWLEDGEMENTS
We acknowledge the help of Ron Bryson, Technical Writer, Division of Biomedical Informatics, CCHMC, OH, USA, in editing the article.
REFERENCES
Author notes
Present address: Ranga Chandra Gudivada, Eli Lilly, Indianapolis, IN 46225.
The authors wish it to be known that, in their opinion, the first three authors should be regarded as joint First Authors.
Comments