-
PDF
- Split View
-
Views
-
Cite
Cite
Chin Lin, Chin-Sheng Lin, Ding-Jie Lee, Chia-Cheng Lee, Sy-Jou Chen, Shi-Hung Tsai, Feng-Chih Kuo, Tom Chau, Shih-Hua Lin, Artificial Intelligence–Assisted Electrocardiography for Early Diagnosis of Thyrotoxic Periodic Paralysis, Journal of the Endocrine Society, Volume 5, Issue 9, September 2021, bvab120, https://doi.org/10.1210/jendso/bvab120
- Share Icon Share
Abstract
Thyrotoxic periodic paralysis (TPP) characterized by acute weakness, hypokalemia, and hyperthyroidism is a medical emergency with a great challenge in early diagnosis since most TPP patients do not have overt symptoms.
This work aims to assess artificial intelligence (AI)-assisted electrocardiography (ECG) combined with routine laboratory data in the early diagnosis of TPP.
A deep learning model (DLM) based on ECG12Net, an 82-layer convolutional neural network, was constructed to detect hypokalemia and hyperthyroidism. The development cohort consisted of 39 ECGs from patients with TPP and 502 ECGs of hypokalemic controls; the validation cohort consisted of 11 ECGs of TPP patients and 36 ECGs of non-TPP individuals with weakness. The AI-ECG–based TPP diagnostic process was then consecutively evaluated in 22 male patients with TTP-like features.
In the validation cohort, the DLM-based ECG system detected all cases of hypokalemia in TPP patients with a mean absolute error of 0.26 mEq/L and diagnosed TPP with an area under curve (AUC) of approximately 80%, surpassing the best standard ECG parameter (AUC = 0.7285 for the QR interval). Combining the AI predictions with the estimated glomerular filtration rate and serum chloride boosted the diagnostic accuracy of the algorithm to AUC 0.986. In the prospective study, the integrated AI and routine laboratory diagnostic system had a PPV of 100% and F-measure of 87.5%.
An AI-ECG system reliably identifies hypokalemia in patients with paralysis, and integration with routine blood chemistries provides valuable decision support for the early diagnosis of TPP.
Acute muscle weakness to paralysis in patients with normal consciousness is frequently encountered in the emergency department (ED) and can be caused by neuromuscular, metabolic, and psychologic disorders. Among metabolic causes, hypokalemia with paralysis (HP) is the most common life-threatening disorder with potentially fatal cardiac arrhythmias and respiratory failure [1, 2]. Before determining blood potassium (K+), HP may be misattributed to other neurological diseases such as Guillain-Barré syndrome, myasthenia gravis, transverse myelitis, or autoimmune myositis [3]. In general, HP can be simply divided into hypokalemic periodic paralysis (HypoKPP) due to acute K+ shift into cells [4] and non-HypoKPP due to chronic massive K+ deficit [5]. Although K+ supplementation can foster muscle recovery and reduce the risk of cardiac arrhythmia [6] in both groups, the dosage of K+ supplementation in patients with HypoKPP (without K+ deficit) should be minimized to avoid the common sequelae of rebound hyperkalemia on recovery [7]. Early recognition of HP with distinction of HypoKPP from non-HypoKPP is clinically important for appropriate management [8].
Among the etiologies of HypoKPP, familial periodic paralysis and nonfamilial thyrotoxic periodic paralysis (TPP) are the most common causes in Western and Asian countries, respectively. TPP is characterized by the presence of hyperthyroidism with acute hypokalemia and occurs with overwhelming male predominance (male-to-female ratio = 100:1) in approximately 2% of hyperthyroid Asians. With globalization, TPP is not limited to Asia and is increasingly seen around the world [9]. Although hyperthyroidism is a prerequisite for the diagnosis of TPP, rapid diagnosis of TPP is a great challenge because most TPP patients do not have overt symptoms and signs of hyperthyroidism [10]. Using Wayne’s score of symptomatology, only approximately 20% of TPP cases have been shown to be clinically thyrotoxic and only 35% identify a clear precipitating factor [11]. Early measurement of thyroid function with point-of-care or STAT thyrotropin (TSH) testing is the lynchpin for diagnosing TPP in the ED. However, timely diagnosis is still challenging when rapid TSH measurement is not available.
Hypokalemia and hyperthyroidism in TPP both significantly affect the cardiovascular system. Electrocardiography (ECG) as a prompt and noninvasive bedside tool universally used in the ED may detect these electrical changes. The main ECG changes associated with hypokalemia include decreased T-wave amplitude, ST-segment depression, T-wave inversion, prolonged PR interval, and increased corrected QT interval [12]. The ECG findings associated with hyperthyroidism included sinus tachycardia, atrioventricular (AV) block, prominent U waves, and high QRS voltage [13, 14]. In our previous report, we found that sinus tachycardia, AV block, and high QRS voltage were significantly more common among TPP than non-TPP patients. However, these findings were evident in only 45%, 45%, and 74% of TPP patients, respectively [13]. Hence, the manual use of ECG features to make an early diagnosis of TPP is still limited. Currently, artificial intelligence (AI) techniques based on deep learning models (DLMs) [15] have been shown to achieve human-level performance and effectively detect cardiac diseases with large annotated ECG data sets [16-28]. Using big ECG-annotated data set analysis, we first successfully applied DLM to develop ECG-based detection of hypokalemia, so-called ECG12Net, in the ED [29]. However, the clinical application of AI-enhanced ECG for the detection of hypokalemia and hyperthyroidism to early-diagnose HP and TPP has not been evaluated.
In this study, we explored AI-enhanced ECG interpretation to rapidly diagnose TPP in the ED. Owing to the limited number of TPP patients, better diagnostic accuracy may be achieved by integrating AI-enhanced ECG with simple blood chemistry. A prospective application was also performed to test the feasibility of this AI-ECG diagnostic process for HP and TPP in patients with acute symmetrical muscle weakness.
Materials and Methods
Population and Data Source
This study was performed at Tri-Service General Hospital, an academic medical center with 1800 beds and approximately 100 000 ED visits per year. The study was approved by the institutional review board of Tri-Service General Hospital (IRB No. A202005151). Patients with TPP were diagnosed by the following criteria: 1) muscle weakness and/or muscle paralysis, 2) serum K+ concentration less than or equal to 3.0 mEq/L with low urinary K+ excretion, 3) thyrotoxicosis confirmed by thyroid function tests, and 4) exclusion of renal and nonrenal causes of hypokalemia. The study included a development cohort for training the DLM, a validation cohort for initial assessment of the DLM’s performance, and a prospective cohort for clinical validation. Only male patients were enrolled because no females had TPP. Patient characteristics and laboratory results were collected using an electronic health record system. The nearest laboratory data were assigned for each ECG record. Estimated glomerular filtration rate (eGFR) was calculated using the Chronic Kidney Disease Epidemiology Collaboration formula [30].
Development and Validation Cohorts
The development cohort consisted of ECGs performed within 1 hour of a central laboratory report obtained from TPP patients and hypokalemic controls (K+ ≤ 3.0 mEq/L) with or without paralysis (non-TPP) between January 1, 2012 and December 31, 2018. ECGs from reevaluations during a visit or subsequent visits on a different date could be used to increase sample size for augmenting DLM performance. There were 39 ECGs from 31 TPP patients and 502 ECGs from 414 hypokalemic controls. The validation cohort consisted of 11 ECGs from 11 TPP patients and 36 ECGs from 36 non-TPP controls (hypokalemic paralysis without hyperthyroidism) between January 1, 2019 and December 31, 2019, to better simulate the clinical scenario. The patients in the development cohort were excluded from the validation cohort.
Prospective cohort
The prospective cohort consisted of male patients with acute symmetrical neuromuscular weakness who received ECGs and laboratory studies between January 1 and October 31, 2020. They were consecutively evaluated using AI-based ECG to predict both hypokalemia and TPP. The inclusion criteria stipulated normal consciousness and symmetrically decreased muscle power with reduced deep tendon reflexes on physical examination. Patients who had a history of TPP or exhibited abnormal neurologic findings, such as increased peripheral reflexes, unilateral paralysis, or altered consciousness, were excluded. Thyroid function tests were also determined.
Deep Learning Model Development and Training
The ECGs were recorded using Philips 12-lead ECG machines (PH080A), which also provided 8 basic ECG morphology parameters. We devised the ECG-TPP probability score by DLM, which was based on ECG12Net, an 82-layer convolutional neural network to detect hypokalemia from our previous study [29]. We used 3 training strategies—no match, age-matched, and age-K+–matched—to derive 3 DLMs and 3 ECG-TPP probability scores—1, 2, and 3, respectively (Supplementary Fig. 1) [31]. The details of our DLM implementation are described in the supplementary materials [31].
Statistical Analysis and Model Performance Assessment
Patient characteristics are presented as means and SDs or percentages where appropriate. The t test and chi-square test were used to test the difference in means and percentages, respectively. Statistical analysis was carried out using R version 3.4.4 with a significance level of P less than .05. The receiver operating characteristic (ROC) curve and area under the curve (AUC) were used to measure their effects. We compared the AUCs of DLM and ECG morphologies and the difference between the 3 DLM training strategies. We further selected some integration models for diagnosing TPP with or without laboratory information. An integrated TPP diagnostic process was developed based on our validation cohort. The sensitivity, specificity, positive predictive value (PPV), and F-measure were used for quantifying the diagnostic value.
Results
Patient Characteristics in the Development and Validation Cohorts
As shown in Table 1, patients with TPP had the hallmark findings of suppressed TSH and high free thyroxine. They were significantly younger, weaker, and had fewer comorbidities both in the development and validation cohorts compared to hypokalemic controls. Among the laboratory findings, TPP patients also exhibited significantly lower serum K+, creatinine concentrations (higher eGFR), higher serum chloride (Cl–), and higher total calcium levels. There were no significant differences in ECG morphologies between TPP and hypokalemic controls aside from increased PR interval. Approximately 10% of hypokalemic controls presented with muscle weakness and/or paralysis in the development cohort. Hyperthyroid patients may also have chronic hypokalemia due to total K+ deficit, of which there were 2 and 3 in the development and validation cohorts, respectively.
. | Development cohort . | Validation cohort . | ||||
---|---|---|---|---|---|---|
. | TPP (n = 39) . | HypoK control (n = 502) . | P . | TPP (n = 11) . | non-TPP (n = 36) . | P . |
Sex, male | 39 (100.0%) | 502 (100.0%) | 11 (100.0%) | 36 (100.0%) | ||
Age, y | 34.7 ± 8.4 | 47.1 ± 10.5 | < .001 | 35.9 ± 7.8 | 52.8 ± 13.1 | < .001 |
ECG-K+, mEq/L | 2.6 ± 0.6 | 3.0 ± 0.5 | .106 | |||
Weakness | 39(100.0%) | 50(10.0%) | < .001 | 11(100.0%) | 36(100.0%) | |
ECG morphology | ||||||
Heart rate | 95.1 ± 17.5 | 91.5 ± 21.7 | .306 | 100.0 ± 10.1 | 85.3 ± 14.6 | .003 |
PR interval | 176.0 ± 33.2 | 162.1 ± 37.1 | .025 | 166.1 ± 57.3 | 166.6 ± 40.5 | .674 |
QRS duration | 101.6 ± 10.8 | 102.3 ± 17.9 | .806 | 96.5 ± 11.1 | 110.2 ± 26.0 | .094 |
QT interval | 384.3 ± 76.0 | 395.1 ± 55.8 | .257 | 364.6 ± 29.3 | 399.2 ± 47.4 | .023 |
Correct QT interval | 476.1 ± 81.2 | 480.0 ± 50.4 | .662 | 470.2 ± 43.2 | 471.4 ± 46.0 | 1.000 |
P waves axes | 65.0 ± 60.6 | 54.8 ± 30.7 | .074 | 51.5 ± 68.6 | 53.1 ± 32.4 | .905 |
RS waves axes | 57.2 ± 23.3 | 47.5 ± 49.6 | .227 | 47.9 ± 28.7 | 47.4 ± 35.9 | .950 |
T waves axes | 47.5 ± 79.9 | 42.8 ± 63.5 | .670 | 17.7 ± 50.8 | 49.3 ± 77.3 | .407 |
Laboratory test | ||||||
TSH, μIU/mL | 0.0 ± 0.1 | 1.3 ± 1.2 | < .001 | 0.0 ± 0.0 | 1.8 ± 1.4 | .004 |
Free T4, ng/dL | 2.5 ± 0.6 | 1.0 ± 0.3 | < .001 | 2.7 ± 0.5 | 1.1 ± 0.1 | .003 |
eGFR, mL/min | 146.2 ± 54.6 | 90.4 ± 59.5 | < .001 | 156.1 ± 43.7 | 91.3 ± 45.6 | < .001 |
Cr, mg/dL | 0.8 ± 0.6 | 1.7 ± 2.3 | .019 | 0.7 ± 0.2 | 1.6 ± 2.3 | < .001 |
BUN, mg/dL | 18.0 ± 15.2 | 18.8 ± 15.5 | .748 | 11.7 ± 3.7 | 19.9 ± 16.3 | .117 |
K+, mEq/L | 2.5 ± 0.6 | 2.8 ± 0.2 | < .001 | 2.5 ± 0.5 | 2.7 ± 0.3 | .027 |
Na+, mEq/L | 139.2 ± 2.2 | 136.1 ± 5.0 | < .001 | 139.3 ± 1.2 | 137.6 ± 5.0 | .454 |
Cl–, mEq/L | 106.7 ± 2.3 | 99.4 ± 7.6 | < .001 | 108.0 ± 2.1 | 101.3 ± 7.4 | .005 |
Ca++, mg/dL | 8.8 ± 0.7 | 8.5 ± 0.8 | .024 | 8.9 ± 0.6 | 8.1 ± 0.9 | .003 |
Mg++, mg/dL | 1.9 ± 0.3 | 1.9 ± 0.4 | .334 | 2.0 ± 0.2 | 1.8 ± 0.5 | .541 |
. | Development cohort . | Validation cohort . | ||||
---|---|---|---|---|---|---|
. | TPP (n = 39) . | HypoK control (n = 502) . | P . | TPP (n = 11) . | non-TPP (n = 36) . | P . |
Sex, male | 39 (100.0%) | 502 (100.0%) | 11 (100.0%) | 36 (100.0%) | ||
Age, y | 34.7 ± 8.4 | 47.1 ± 10.5 | < .001 | 35.9 ± 7.8 | 52.8 ± 13.1 | < .001 |
ECG-K+, mEq/L | 2.6 ± 0.6 | 3.0 ± 0.5 | .106 | |||
Weakness | 39(100.0%) | 50(10.0%) | < .001 | 11(100.0%) | 36(100.0%) | |
ECG morphology | ||||||
Heart rate | 95.1 ± 17.5 | 91.5 ± 21.7 | .306 | 100.0 ± 10.1 | 85.3 ± 14.6 | .003 |
PR interval | 176.0 ± 33.2 | 162.1 ± 37.1 | .025 | 166.1 ± 57.3 | 166.6 ± 40.5 | .674 |
QRS duration | 101.6 ± 10.8 | 102.3 ± 17.9 | .806 | 96.5 ± 11.1 | 110.2 ± 26.0 | .094 |
QT interval | 384.3 ± 76.0 | 395.1 ± 55.8 | .257 | 364.6 ± 29.3 | 399.2 ± 47.4 | .023 |
Correct QT interval | 476.1 ± 81.2 | 480.0 ± 50.4 | .662 | 470.2 ± 43.2 | 471.4 ± 46.0 | 1.000 |
P waves axes | 65.0 ± 60.6 | 54.8 ± 30.7 | .074 | 51.5 ± 68.6 | 53.1 ± 32.4 | .905 |
RS waves axes | 57.2 ± 23.3 | 47.5 ± 49.6 | .227 | 47.9 ± 28.7 | 47.4 ± 35.9 | .950 |
T waves axes | 47.5 ± 79.9 | 42.8 ± 63.5 | .670 | 17.7 ± 50.8 | 49.3 ± 77.3 | .407 |
Laboratory test | ||||||
TSH, μIU/mL | 0.0 ± 0.1 | 1.3 ± 1.2 | < .001 | 0.0 ± 0.0 | 1.8 ± 1.4 | .004 |
Free T4, ng/dL | 2.5 ± 0.6 | 1.0 ± 0.3 | < .001 | 2.7 ± 0.5 | 1.1 ± 0.1 | .003 |
eGFR, mL/min | 146.2 ± 54.6 | 90.4 ± 59.5 | < .001 | 156.1 ± 43.7 | 91.3 ± 45.6 | < .001 |
Cr, mg/dL | 0.8 ± 0.6 | 1.7 ± 2.3 | .019 | 0.7 ± 0.2 | 1.6 ± 2.3 | < .001 |
BUN, mg/dL | 18.0 ± 15.2 | 18.8 ± 15.5 | .748 | 11.7 ± 3.7 | 19.9 ± 16.3 | .117 |
K+, mEq/L | 2.5 ± 0.6 | 2.8 ± 0.2 | < .001 | 2.5 ± 0.5 | 2.7 ± 0.3 | .027 |
Na+, mEq/L | 139.2 ± 2.2 | 136.1 ± 5.0 | < .001 | 139.3 ± 1.2 | 137.6 ± 5.0 | .454 |
Cl–, mEq/L | 106.7 ± 2.3 | 99.4 ± 7.6 | < .001 | 108.0 ± 2.1 | 101.3 ± 7.4 | .005 |
Ca++, mg/dL | 8.8 ± 0.7 | 8.5 ± 0.8 | .024 | 8.9 ± 0.6 | 8.1 ± 0.9 | .003 |
Mg++, mg/dL | 1.9 ± 0.3 | 1.9 ± 0.4 | .334 | 2.0 ± 0.2 | 1.8 ± 0.5 | .541 |
Abbreviations: BUN, blood urea nitrogen; Ca++, total calcium; Cl–, chloride; Cr, creatinine; ECG, electrocardiography; ECG-K+, K+ estimated via electrocardiography; eGFR, estimated glomerular filtration rate; Free T4, free thyroxine; HypoK, hypokalemia; K+, potassium; Mg++, magnesium; Na+, sodium; TSH, thyrotropin; TPP, thyrotoxic periodic paralysis.
. | Development cohort . | Validation cohort . | ||||
---|---|---|---|---|---|---|
. | TPP (n = 39) . | HypoK control (n = 502) . | P . | TPP (n = 11) . | non-TPP (n = 36) . | P . |
Sex, male | 39 (100.0%) | 502 (100.0%) | 11 (100.0%) | 36 (100.0%) | ||
Age, y | 34.7 ± 8.4 | 47.1 ± 10.5 | < .001 | 35.9 ± 7.8 | 52.8 ± 13.1 | < .001 |
ECG-K+, mEq/L | 2.6 ± 0.6 | 3.0 ± 0.5 | .106 | |||
Weakness | 39(100.0%) | 50(10.0%) | < .001 | 11(100.0%) | 36(100.0%) | |
ECG morphology | ||||||
Heart rate | 95.1 ± 17.5 | 91.5 ± 21.7 | .306 | 100.0 ± 10.1 | 85.3 ± 14.6 | .003 |
PR interval | 176.0 ± 33.2 | 162.1 ± 37.1 | .025 | 166.1 ± 57.3 | 166.6 ± 40.5 | .674 |
QRS duration | 101.6 ± 10.8 | 102.3 ± 17.9 | .806 | 96.5 ± 11.1 | 110.2 ± 26.0 | .094 |
QT interval | 384.3 ± 76.0 | 395.1 ± 55.8 | .257 | 364.6 ± 29.3 | 399.2 ± 47.4 | .023 |
Correct QT interval | 476.1 ± 81.2 | 480.0 ± 50.4 | .662 | 470.2 ± 43.2 | 471.4 ± 46.0 | 1.000 |
P waves axes | 65.0 ± 60.6 | 54.8 ± 30.7 | .074 | 51.5 ± 68.6 | 53.1 ± 32.4 | .905 |
RS waves axes | 57.2 ± 23.3 | 47.5 ± 49.6 | .227 | 47.9 ± 28.7 | 47.4 ± 35.9 | .950 |
T waves axes | 47.5 ± 79.9 | 42.8 ± 63.5 | .670 | 17.7 ± 50.8 | 49.3 ± 77.3 | .407 |
Laboratory test | ||||||
TSH, μIU/mL | 0.0 ± 0.1 | 1.3 ± 1.2 | < .001 | 0.0 ± 0.0 | 1.8 ± 1.4 | .004 |
Free T4, ng/dL | 2.5 ± 0.6 | 1.0 ± 0.3 | < .001 | 2.7 ± 0.5 | 1.1 ± 0.1 | .003 |
eGFR, mL/min | 146.2 ± 54.6 | 90.4 ± 59.5 | < .001 | 156.1 ± 43.7 | 91.3 ± 45.6 | < .001 |
Cr, mg/dL | 0.8 ± 0.6 | 1.7 ± 2.3 | .019 | 0.7 ± 0.2 | 1.6 ± 2.3 | < .001 |
BUN, mg/dL | 18.0 ± 15.2 | 18.8 ± 15.5 | .748 | 11.7 ± 3.7 | 19.9 ± 16.3 | .117 |
K+, mEq/L | 2.5 ± 0.6 | 2.8 ± 0.2 | < .001 | 2.5 ± 0.5 | 2.7 ± 0.3 | .027 |
Na+, mEq/L | 139.2 ± 2.2 | 136.1 ± 5.0 | < .001 | 139.3 ± 1.2 | 137.6 ± 5.0 | .454 |
Cl–, mEq/L | 106.7 ± 2.3 | 99.4 ± 7.6 | < .001 | 108.0 ± 2.1 | 101.3 ± 7.4 | .005 |
Ca++, mg/dL | 8.8 ± 0.7 | 8.5 ± 0.8 | .024 | 8.9 ± 0.6 | 8.1 ± 0.9 | .003 |
Mg++, mg/dL | 1.9 ± 0.3 | 1.9 ± 0.4 | .334 | 2.0 ± 0.2 | 1.8 ± 0.5 | .541 |
. | Development cohort . | Validation cohort . | ||||
---|---|---|---|---|---|---|
. | TPP (n = 39) . | HypoK control (n = 502) . | P . | TPP (n = 11) . | non-TPP (n = 36) . | P . |
Sex, male | 39 (100.0%) | 502 (100.0%) | 11 (100.0%) | 36 (100.0%) | ||
Age, y | 34.7 ± 8.4 | 47.1 ± 10.5 | < .001 | 35.9 ± 7.8 | 52.8 ± 13.1 | < .001 |
ECG-K+, mEq/L | 2.6 ± 0.6 | 3.0 ± 0.5 | .106 | |||
Weakness | 39(100.0%) | 50(10.0%) | < .001 | 11(100.0%) | 36(100.0%) | |
ECG morphology | ||||||
Heart rate | 95.1 ± 17.5 | 91.5 ± 21.7 | .306 | 100.0 ± 10.1 | 85.3 ± 14.6 | .003 |
PR interval | 176.0 ± 33.2 | 162.1 ± 37.1 | .025 | 166.1 ± 57.3 | 166.6 ± 40.5 | .674 |
QRS duration | 101.6 ± 10.8 | 102.3 ± 17.9 | .806 | 96.5 ± 11.1 | 110.2 ± 26.0 | .094 |
QT interval | 384.3 ± 76.0 | 395.1 ± 55.8 | .257 | 364.6 ± 29.3 | 399.2 ± 47.4 | .023 |
Correct QT interval | 476.1 ± 81.2 | 480.0 ± 50.4 | .662 | 470.2 ± 43.2 | 471.4 ± 46.0 | 1.000 |
P waves axes | 65.0 ± 60.6 | 54.8 ± 30.7 | .074 | 51.5 ± 68.6 | 53.1 ± 32.4 | .905 |
RS waves axes | 57.2 ± 23.3 | 47.5 ± 49.6 | .227 | 47.9 ± 28.7 | 47.4 ± 35.9 | .950 |
T waves axes | 47.5 ± 79.9 | 42.8 ± 63.5 | .670 | 17.7 ± 50.8 | 49.3 ± 77.3 | .407 |
Laboratory test | ||||||
TSH, μIU/mL | 0.0 ± 0.1 | 1.3 ± 1.2 | < .001 | 0.0 ± 0.0 | 1.8 ± 1.4 | .004 |
Free T4, ng/dL | 2.5 ± 0.6 | 1.0 ± 0.3 | < .001 | 2.7 ± 0.5 | 1.1 ± 0.1 | .003 |
eGFR, mL/min | 146.2 ± 54.6 | 90.4 ± 59.5 | < .001 | 156.1 ± 43.7 | 91.3 ± 45.6 | < .001 |
Cr, mg/dL | 0.8 ± 0.6 | 1.7 ± 2.3 | .019 | 0.7 ± 0.2 | 1.6 ± 2.3 | < .001 |
BUN, mg/dL | 18.0 ± 15.2 | 18.8 ± 15.5 | .748 | 11.7 ± 3.7 | 19.9 ± 16.3 | .117 |
K+, mEq/L | 2.5 ± 0.6 | 2.8 ± 0.2 | < .001 | 2.5 ± 0.5 | 2.7 ± 0.3 | .027 |
Na+, mEq/L | 139.2 ± 2.2 | 136.1 ± 5.0 | < .001 | 139.3 ± 1.2 | 137.6 ± 5.0 | .454 |
Cl–, mEq/L | 106.7 ± 2.3 | 99.4 ± 7.6 | < .001 | 108.0 ± 2.1 | 101.3 ± 7.4 | .005 |
Ca++, mg/dL | 8.8 ± 0.7 | 8.5 ± 0.8 | .024 | 8.9 ± 0.6 | 8.1 ± 0.9 | .003 |
Mg++, mg/dL | 1.9 ± 0.3 | 1.9 ± 0.4 | .334 | 2.0 ± 0.2 | 1.8 ± 0.5 | .541 |
Abbreviations: BUN, blood urea nitrogen; Ca++, total calcium; Cl–, chloride; Cr, creatinine; ECG, electrocardiography; ECG-K+, K+ estimated via electrocardiography; eGFR, estimated glomerular filtration rate; Free T4, free thyroxine; HypoK, hypokalemia; K+, potassium; Mg++, magnesium; Na+, sodium; TSH, thyrotropin; TPP, thyrotoxic periodic paralysis.
Electrocardiography-Enabled Deep Learning Model Development and Training
We have successfully developed an ECG-DLM to estimate serum K+ concentration. Moreover, 3 additional DLMs using different training strategies were created in the development cohort. Based on these DLMs, each ECG can be interpreted to a K+ value and 3 scores. Building on this body of work, we therefore used these AI-ECG estimations to evaluate their accuracy in an independent validation cohort.
Comparison Between Deep Learning Model and Electrocardiography Parameters to Diagnose Thyrotoxic Periodic Paralysis
To evaluate the detection of hypokalemia by AI-ECG, we first explored the difference between AI-ECG–predicted and laboratory-based K+ concentration (Fig. 1). The mean absolute errors among TPP (n = 11) and non-TPP patients (n = 36) were 0.259 and 0.326 mEq/L, respectively. To evaluate the detection of TPP by ECG, we compared the diagnostic value of traditional ECG morphologies with the ECG-based DLMs trained using 3 different weighting strategies. As shown in Fig. 2, the most important ECG morphologies were QT interval (AUC = 0.7285) and heart rate (AUC = 0.7273). Combining all ECG morphologies in logistic regression actually had a worse AUC (0.5379). The ECG-based DMLs demonstrated higher AUCs than traditional ECG morphologies with similar performance among the 3 training strategies; the AUC of scores 1, 2, and 3 were 0.8131, 0.7753, and 0.7677, respectively.
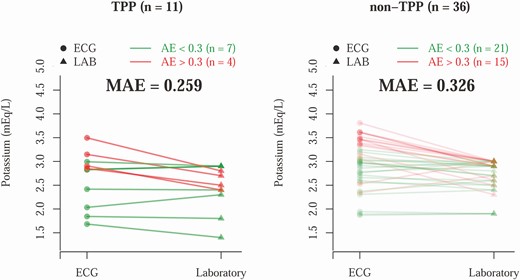
Comparison between electrocardiography (ECG)-based potassium (K+) prediction and laboratory (LAB) K+ in the validation cohort. Each line represents a hypokalemia case. The patients with absolute error (AE) greater than 0.3 are colored red, and the others are colored green. The t test shows the mean AE (MAE) differences are not significantly different for thyrotoxic periodic paralysis (TPP) vs non-TPP (P = .409).
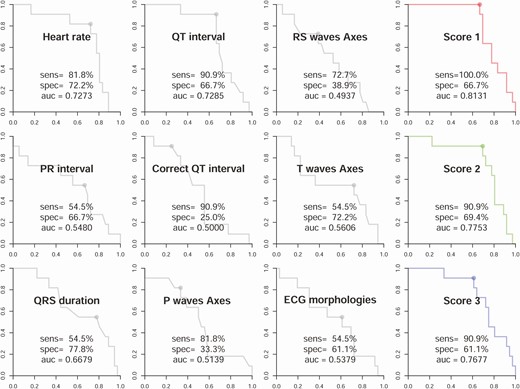
Performance comparisons of electrocardiography (ECG) morphologies and deep learning models trained using 3 different weighting strategies in the validation cohort. The receiver operating characteristic curves were made by the predictions of the deep learning model (DLM) or each ECG morphology. The ECG morphology curves were generated from logistic regression using the development cohort. The DLM score 1 was trained using the raw data set; score 2 was trained using an age-matched strategy; and score 3 was trained using an age- and K+-matched strategy.
Integration of Laboratory Data to Augment the Accuracy of Artificial Intelligence–Thyrotoxic Periodic Paralysis Prediction
Although AI-ECG–based prediction of TPP performed satisfactorily, integration with some readily available clinical data further improved diagnostic performance. Supplementary Fig. 2 [31] shows the importance analysis of various patient characteristics. Age, serum K+, Cl– levels, and eGFR were significantly associated with TPP in multivariable models. We then used these clinical features to augment the TPP diagnostic process and select an ECG-TPP score. As shown in Fig. 3, the iterative addition of age, K, eGFR, and Cl– to the DLMs successively improved the diagnostic accuracy and the combination of these 4 features with score 3 reached an AUC 0.9860. Therefore, we used score 3 as the ECG-TPP score in our TPP diagnostic process.
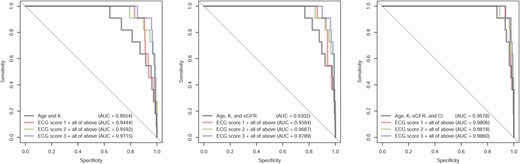
Receiver operating characteristic (ROC) curves for combining patient characteristics with deep learning models in the validation cohort. The ROC curves for clinical characteristics were made by logistic regression. The combination models were generated for each score with the listed clinical characteristics. Score 1 was trained using the raw data set; score 2 was trained using an age-matched strategy; and score 3 was trained using an age- and K+-matched strategy.
Prospective Evaluation of the Artificial Intelligence–Electrocardiography–Assisted Thyrotoxic Periodic Paralysis Diagnostic Algorithm
We had developed a diagnostic process based on the cutoff points from ROC curves to apply in a prospective study. As shown in Fig. 4, there were 22 patients with TPP-like features. Six (non-HP disorders) had neither AI-ECG–predicted hypokalemia nor laboratory hypokalemia. Their final diagnoses included Guillain-Barré syndrome (n = 3), severe hyperkalemia associated with uremia (n = 1), rhabdomyolysis (n = 1), and transverse myelitis (n = 1). Sixteen patients (HP) with AI-ECG–predicted hypokalemia (2.51 ± 0.43 mEq/L) also had significant laboratory hypokalemia (2.35 ± 0.44 mEq/L). Our AI-ECG system achieved perfect performance (F-measure 100%) on the task of hypokalemia detection in them. Using an ECG-TPP score threshold greater than 50%, 12 of 16 AI-ECG HP patients were predicted to have TPP with a sensitivity of 88.9%, specificity of 69.2%, PPV of 66.6%, and F-measure of 76.2%. As shown in Supplementary Table 1 [31], the ECG-TPP score exhibited much better performance compared to the ECG parameters manually and electronically. Combining the AI with laboratory eGFR and serum Cl–, our diagnostic system achieved PPV 100%, sensitivity 77.8%, and F-measure 87.5%.
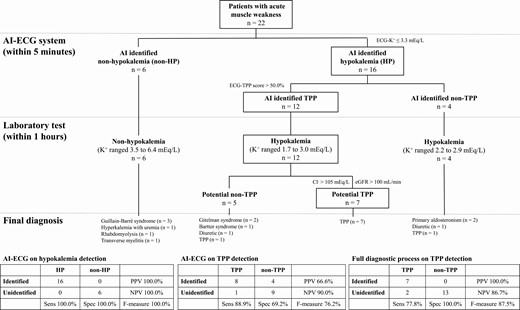
The prospective integrated artificial intelligence–electrocardiography (AI-ECG) diagnostic algorithm for actively identifying potential thyrotoxic periodic paralysis (TPP) cases. Male patients with metabolic paralysis after physical examination were included. The boxes at each step denotes the patients who progressed toward a diagnosis of TPP.
Illustration of Artificial Intelligence–Electrocardiography Diagnostic Process for Thyrotoxic Periodic Paralysis (TPP) and non-TPP
Six patients with HP, 3 TPP and 3 non-TPP from the prospective study, are presented as Supplementary Cases 1 to 6 [31] to further illustrate our AI-assisted diagnostic process.
Discussion
We have established an AI-ECG system to help recognize TPP by applying DLMs to a development cohort. In the validation cohort, the AI-ECG system achieved predictions of hypokalemia with mean absolute error vs serum K+ concentration of only 0.26 mEq/L and TPP with AUC of approximately 80%. Combining the AI predictions with laboratory-derived eGFR and serum Cl– levels, the AUC curve reached 98.6%. In the prospective study, our AI-ECG system demonstrated perfect performance on hypokalemia detection and good diagnostic performance for TPP with PPV of 100% and F-measure of 87.5% when integrated with laboratory eGFR and serum Cl–. To the best of our knowledge, this is the first study of an AI-ECG system to assist in the diagnosis of TPP in the ED.
TPP comprised approximately one-third of our HP cases and paralysis due to massive K+ deficit (non-HypoKPP) approximately the other 60%. This distinction is critical because the dose of K+ supplementation in TPP should be kept low to avoid rebound hyperkalemia on recovery, which happens in approximately 40% to 60% of TPP patients [7]. Our previous studies have shown that the total dosage of K+ supplementation for TPP should be less than 1 mEq/Kg to avoid rebound hyperkalemia, but 3 to 4 mEq/Kg may be necessary in non-HypoKPP [7]. The appropriate management, therefore, hinges on an early diagnosis of TPP. However, TPP is frequently unrecognized or misdiagnosed because most TPP patients do not have overt symptoms and signs of hyperthyroidism [11]. Furthermore, rapid determination of thyroid function is unavailable in most EDs. Although the simultaneous assessment of blood acid–base status and urine electrolytes may help suggest the diagnosis of TPP [32, 33], it is still time-consuming and requires adept interpretation of the data.
ECG is a prompt and noninvasive bedside tool to detect cardiac electrophysiological activity. In TPP, hypokalemia and hyperthyroidism both can significantly affect the heart and contribute to ECG changes. Previously, we had manually examined the ECG changes in 34 patients with TPP and showed that hyperthyroidism-related sinus tachycardia, first-degree AV block, and left ventricular hypertrophy pattern coupled with hypokalemia-associated ST depression and U waves were present in less than 50% of TPP patients [14]. In this study, again, standard ECG morphologic analysis poorly differentiated TPP from hypokalemic and non-TPP disorders. In contrast, DLM can automatically extract useful features and relationships from the ECG [15], and our AI-enabled ECG analysis provided not only perfect detection of hypokalemia in weak patients but also achieved sensitivity of 88.9% and specificity of 69.2%% with AUC of approximately 80% for the diagnosis of TPP. An important human benefit of an AI system stems from the fact that TPP is not on most clinicians’ differential for patients with weakness. A prompt from a computer is a needed reminder when this diagnosis may be appropriate.
Although the AI-ECG system had better diagnostic performance than manual ECG alone, there was still room for improvement, potentially related to our limited data set of training TPP cases. Of note, patients with TPP exhibited some other clinical features that are significantly different from hypokalemic controls. The significantly higher eGFR and serum Cl– concentration in TPP could be used to augment the AI-ECG-TPP score. The higher eGFR, as an index of relatively lower serum creatinine, has been associated with increased cardiac output and glomerular filtration rate in thyrotoxicosis [34]. The elevated serum Cl– concentration is thought to be the renal physiologic response to the increased protein catabolism and respiratory alkalosis observed in hyperthyroidism, as well as the K+ shift into cells in exchange with intracellular protons [35]. The relatively higher serum Cl– in TPP is even more striking since most hypokalemic controls had hypochloremic metabolic alkalosis [36].
In the prospective study, 6 of 22 patients with presumed metabolic weakness did not have AI-ECG–predicted hypokalemia and no laboratory hypokalemia (non-HP disorders). The other 16 patients with AI-ECG–predicted hypokalemia also exhibited laboratory hypokalemia, indicating excellent performance on hypokalemia detection in patients with HP with an F-measure of 100%. This result was probably related to the young age with few comorbidities and confounding effects on the hearts of these patients. Using the ECG-TPP score alone to detect hyperthyroidism, 12 of 16 AI-ECG HP patients had TPP with a PPV of 66.7% and F-measure of 76.2%. However, combining eGFR and serum Cl– in the diagnostic algorithm achieved a PPV of 100% and F-measure 87.5%. Despite a single misclassified patient with TPP (who had coexisting hypertension, gout, and chronic kidney disease), this prospective study supports the value of our integrated AI-ECG system in the rapid diagnosis of TPP.
This study also compared 3 training strategies based on epidemiological perspectives. A series of matching strategies were tried to avoid the DLMs’ learning spurious correlations and maximize useful ECG features. TPP patients are often younger and exhibit lower serum K+ in the ED [37]. Previous studies had shown correlations between age [38], K+ [29], and ECG, raising the possibility of confounding effects on both the ECG and TPP [39]. To the best of our knowledge, this is the first deep learning study to consider the potential effects of confounders during training.
This AI-assisted ECG interpretation system easily plugs into the busy workflow of the ED to provide early detection of hypokalemia and TPP. In our prospective study, every ECG was uploaded in real time to a server that calculated the ECG-K+ and ECG-TPP score in the background within 10 seconds. Our system was configured to then send a text message to the ordering physician if an ECG was flagged as suspicious. This AI-assisted ECG interpretation system may be particularly valuable to EDs without urgent TSH measurement.
There were some limitations of this study. First, all TPP patients with annotated ECG data were recruited from a single center, although this ensured the quality of the data. Second, the number of TPP patients was still small. More TPP patients from a larger data set could enhance the diagnostic accuracy of the system. Third, this AI system was trained only on the available male patients. Female TPP patients should be included for a more generalizable DLM. Fourth, point-of-care TSH determination helpful in the diagnosis of TPP was not performed in this study. A comparison between our AI-ECG system and urgent TSH measurement in the diagnosis of TPP should be evaluated. Finally, this study focused on the diagnostic value of using AI-ECG for TPP. Clinical benefit, cost, and utilization of the new intervention strategy were not evaluated and should be warranted in further investigations compared to conventional diagnostic and therapeutic approaches.
Conclusion
Besides taking a detailed history and careful physical examination, we have developed an AI-assisted ECG to help diagnose HP and distinguish TPP from non-TPP causes. Rapid identification of hypokalemia and integration of AI ECG-TPP score with routine laboratory values achieved high precision for the early diagnosis of TPP prior to the results of thyroid function tests. This bloodless AI-ECG system may also provide decision support and almost real-time monitoring of ECG-based K+ levels during K+ supplementation to help physicians avoid rebound hyperkalemia. Further large-scale patient studies with multicenter data sets and validation are warranted to confirm the diagnostic value and clinical impact of AI-enabled ECG.
Abbreviations
- AI
artificial intelligence
- AUC
area under the curve
- AV
atrioventricular
- Cl–
chloride
- DLM
deep learning model
- ECG
electrocardiography
- ECG-K+
K+ estimated via electrocardiography
- ED
emergency department
- eGFR
estimated glomerular filtration rate
- HP
hypokalemia with paralysis
- HypoK
hypokalemia
- HypoKPP
hypokalemic periodic paralysis
- K+
potassium
- PPV
positive predictive value
- ROC
receiver operating characteristic
- TSH
thyrotropin
- TPP
thyrotoxic periodic paralysis
Acknowledgments
Financial Support: This work was supported by the Ministry of Science and Technology (grant Nos. MOST 108-2314-B-016-001 and MOST 109-2314-B-016-026, Tri-Service General Hospital (grant No. TSGH-C107-007-007-S02), the National Science and Technology Development Fund Management Association, Taiwan (grant Nos. MOST 108-3111-Y-016-009 and 109-3111-Y-016-002), and Cheng Hsin General Hospital, Taiwan (grant Nos. CHNDMC-109-19 and CHNDMC-110-15).
Author Contributions: S.H.L. provided contributions to the study conception and design, acquisition of data or analysis and interpretation of data, or revising it critically for important intellectual content and final approval of the version to be published. C.L. drafted the initial manuscript and analyzed the data. C.C.L. provided the retrospective data for model training. D.J.L., S.J.C., and S.H.T. carefully conducted the prospective study. C.S.L. and T.C. revised the manuscript for important intellectual content. S.H.L. takes final responsibility for this article.
Additional Information
Disclosures: The authors have nothing to disclose.
Data Availability
The data sets generated during and/or analyzed during the present study are not publicly available but are available from the corresponding author on reasonable request.