-
PDF
- Split View
-
Views
-
Cite
Cite
Minjae J Kim, Peter Mende-Siedlecki, Stefano Anzellotti, Liane Young, Theory of Mind Following the Violation of Strong and Weak Prior Beliefs, Cerebral Cortex, Volume 31, Issue 2, February 2021, Pages 884–898, https://doi.org/10.1093/cercor/bhaa263
- Share Icon Share
Abstract
Recent work in psychology and neuroscience has revealed differences in impression updating across social distance and group membership. Observers tend to maintain prior impressions of close (vs. distant) and ingroup (vs. outgroup) others in light of new information, and this belief maintenance is at times accompanied by increased activity in Theory of Mind regions. It remains an open question whether differences in the strength of prior beliefs, in a context absent social motivation, contribute to neural differences during belief updating. We devised a functional magnetic resonance imaging study to isolate the impact of experimentally induced prior beliefs on mentalizing activity. Participants learned about targets who performed 2 or 4 same-valenced behaviors (leading to the formation of weak or strong priors), before performing 2 counter-valenced behaviors. We found a greater change in activity in dorsomedial prefrontal cortex (DMPFC) and right temporo-parietal junction following the violation of strong versus weak priors, and a greater change in activity in DMPFC and left temporo-parietal junction following the violation of positive versus negative priors. These results indicate that differences in neural responses to unexpected behaviors from close versus distant others, and ingroup versus outgroup members, may be driven in part by differences in the strength of prior beliefs.
Introduction
It can be hard for people to change their minds about those they know well, or those who are in their groups. For instance, observers are less likely to revise their impressions when a close friend takes money from them in an economic game, compared with when a stranger does the same (Park et al. 2020). In addition, observers who learn both positive information (e.g., “was awarded a research grant”) and negative information (e.g., “heckled a speaker during a talk”) about ingroup and outgroup members selectively downgrade their impressions of outgroup members (Hughes, Zaki, et al. 2017b). Thus, there are differences in the magnitude of impression updating across social distance and across group membership. While such phenomena have typically been interpreted as biased or motivated, they are also compatible with rational updating over stronger (more certain) prior beliefs about close others and ingroup members (Gershman 2019; Kim et al. 2020). It can be difficult to pull apart the contributions of motivation and prior knowledge to selective belief maintenance, as they typically co-occur: we are motivated to preserve favorable impressions of groups we belong to (Van Bavel and Pereira 2018), and at the same time, ample prior experience with others can give rise to stronger beliefs that are hard to update in the face of contradictory evidence. In the current work, we ask whether differences in experimentally induced prior beliefs, in a context absent social motivation, can lead to differences in impression updating and related neural activity. We examine changes in both rated impressions and neural activity following the violation of strong versus weak prior beliefs, and following the violation of positive versus negative prior beliefs.
The Role of Mental State Inference in Impression Updating
A key process underlying impression updating is mentalizing, or Theory of Mind (ToM): the ability to infer, represent, and reason about others’ mental states, such as beliefs, goals, and intentions. When observers generate explanations for others’ behavior—why someone did what they did—mental state inferences tend to dominate (Malle 2001). Mentalizing can support either impression updating or impression maintenance, depending on the content of the mental state inference. For example, when we see a stranger take money from us in an economic game, we may infer that she intended to take the money; we may then use this inference to update our beliefs about her character. In comparison, when we see a close friend take money from us, we may infer that she did not intend to simply take the money; she was actually mistaken about the rules of the game, or she plans to share the spoils with us later. Such inferences allow us to maintain our positive prior beliefs about our friend’s character. On the flip side, when we see an outgroup member behave prosocially, we may infer that she did so only for self-interested, reputational reasons; such inferences allow us to maintain our negative prior beliefs about the outgroup member. Inferences about transient mental states can thus be used to either support an impression update, or reconcile discrepancies between our prior impression of someone and their surprising, prior-inconsistent behavior.
The Role of Prior Beliefs in Impression Updating
What are the informational factors that determine whether or not we engage in impression updating? Our prior beliefs about others can vary in both strength and valence. We tend to have stronger, and more positive, beliefs about close others compared with strangers; we also have strong beliefs based on group membership in the form of stereotypes (Fiske 1998; Dovidio et al. 2010). When we have strong prior beliefs about someone, and they behave uncharacteristically, we may generate an explanation for their behavior based on a transient mental state, rather than update our impression of their character. Generating alternative explanations in this way can be compatible with a form of Bayesian rationality, such that the likelihood of invoking an alternative explanation depends probabilistically on the strength of the prior belief, and the likelihood of the conflicting information (Gershman 2019). Thus, when we have sufficiently strong prior beliefs about someone’s character, it can be rational to generate alternative explanations for surprising behavior.
Differences in impression updating can arise from differences in belief strength, differences in belief valence, or both. For instance, one may have: 1) strong positive beliefs about a friend, and weak positive beliefs about an acquaintance; 2) strong positive beliefs about the ingroup, and strong negative beliefs about the outgroup; 3) strong positive beliefs about a friend, and weak negative beliefs about a stranger. Note that in intergroup contexts an observer can have counter-valenced but equally strong beliefs about the 2 groups; in these contexts, we expect that both strong positive priors about the ingroup and strong negative priors about the outgroup will be resistant to updating.
Strong prior beliefs about others often co-occur with social motivational factors, such as the desire to selectively maintain positive impressions of ingroup members (Van Bavel and Pereira 2018). When we continue to see close or ingroup transgressors as good or trustworthy, then, this may be because we have strong prior beliefs about their goodness, or because we are motivated to view them in a positive light, and motivated to maintain our social relationships (Park and Young 2020). Analogously, when we refuse to improve our impressions of outgroup others, this may be because we have strong prior beliefs about their bad character, or because we are motivated to view them unfavorably. It is thus important that we examine the role of prior knowledge in belief updating in isolation. In the current work, we isolate the role of prior knowledge in belief updating by manipulating participants’ beliefs about novel, fictional targets. We note that we use the term “motivation” to refer to social motivation stemming from real relationships or social groups, rather than other forms of motivation. For instance, participants may have a general cognitive motivation to hold on to initial beliefs, perhaps in a heuristic manner incompatible with Bayesian reasoning; however, as all targets in the experiment were zero-acquaintance, fictional targets, we expected that participants’ social motivations concerning these targets would be at floor. For this reason, we describe our paradigm as a context absent social motivation.
Mentalizing in Light of Strong Versus Weak Priors
We aimed to examine whether brain regions implicated in ToM are recruited to different degrees in light of different priors. The ToM network includes dorsomedial prefrontal cortex (DMPFC), bilateral temporo-parietal junction (TPJ), and precuneus (PC). These regions are critical for inferring moral intent and integrating mental state information with other information for moral judgment (see Young and Waytz 2013 for a review). They are also implicated in causal attributions to the self, another person, or the situation (Kestemont et al. 2015), and the formation and revision of trait inferences (Cloutier et al. 2011; Ma et al. 2012; Ferrari et al. 2016). In addition, neural activity in ToM regions is enhanced for others’ behaviors that violate prior beliefs based on: past behavioral history (Mende-Siedlecki et al. 2013; Dungan et al. 2016), instructed trait knowledge (Heil et al. 2019), and stereotypes (Cloutier et al. 2011; Li et al. 2016). ToM regions thus respond to the contradiction of prior beliefs across a variety of social contexts, and the enhanced activity may reflect an attempt to construct a mental state explanation, such as one referring to innocent intent, that reconciles the unexpected behavior with prior impressions. For example, one study found greater activity in DMPFC and bilateral TPJ when third-party observers were faced with ingroup versus outgroup defectors in the Prisoner’s Dilemma Game, and increased connectivity between DMPFC and LTPJ was associated with weaker punishment of ingroup defectors (Baumgartner et al. 2012). In this context, the more surprising event (selfish behavior from an ingroup member) was followed by greater activity in mentalizing regions and greater selective forgiveness. This increase in ToM activity may reflect the probabilistic generation of a coherent alternative explanation for the surprising event (e.g., my ingroup member did not intend to defect).
Past neuroimaging work has relied on participants’ real-life prior beliefs about ingroup and outgroup members (Baumgartner et al. 2012), and about friends and strangers (Park et al. 2020), to investigate neural differences during belief updating across relationship contexts. These contexts may have been accompanied by social motivational factors: observers may have engaged in mentalizing out of a desire to protect their beliefs about the ingroup, even though coming up with an alternative explanation was not probabilistically warranted by their prior beliefs. Therefore, it is an open question whether differences in prior strength—in a context absent social motivation—contribute to neural differences during belief updating across social distance and group membership.
The Current Study
The goal of the current study was to examine belief updating and mentalizing activity following the violation of strong versus weak prior beliefs, and positive versus negative prior beliefs, in the absence of real-life priors and motivations concerning groups and individuals. We aimed to experimentally induce prior beliefs that vary in both strength and valence, given that these features may have distinct effects on updating and mentalizing.
We adapted a paradigm developed by Mende-Siedlecki et al. (2012, 2013) and Mende-Siedlecki and Todorov (2016). Participants learned about fictional individuals whose behaviors were either internally consistent or internally inconsistent. The internally inconsistent targets initially performed 2 or 4 same-valenced, morally relevant behaviors (leading to the formation of weak or strong beliefs about the agent’s disposition), before performing 2 counter-valenced behaviors, potentially evoking an impression update. We tracked participants’ impressions long the dimension of trustworthiness. We tested whether impression updating and ToM activity differ as a function of the strength of the prior (weak vs. strong) and update direction (positive-to-negative vs. negative-to-positive). We also conducted whole-brain analyses to examine overall differences in neural activity during impression updating following different types of expectation violations. Lastly, we note that, while participants may have entered the experiment with prior beliefs about the trustworthiness of people in general, we expected these real-life priors to apply equally across targets, given that they are all zero-acquaintance targets. Thus, the term “prior” in the context of this experiment will be used to refer to experimentally induced beliefs about targets. This is in accordance with a cyclical framing of Bayesian belief updating (Trotta 2018), where the posterior belief after a new observation (e.g., a target’s first behavior) then becomes the prior belief for the next observation (e.g., a target’s second behavior).
Materials and Methods
Open Science
This study was preregistered (https://aspredicted.org/blind.php?x=ti3pn4). Behavioral data, percent signal change (PSC) data, and R code are available on OSF (https://osf.io/27cjx/?view_only=df7aa52aef2048d09101df6267aca44e). Neural data are available on OpenNeuro (https://openneuro.org/datasets/ds002793).
Participants
We aimed to collect analyzable data from 28 participants (based on recent neuroimaging studies examining ToM regions, Tsoi et al. 2018, N = 25; Dungan and Young 2019, N = 26; Theriault, Waytz, et al. 2020a, N = 25). Thirty adults from the Boston area between the ages of 18 and 35 were recruited. All participants were right-handed, native English speakers with normal or corrected-to-normal vision and no history of psychiatric disorders or learning disabilities. Participants were recruited through an online posting and given a $60 cash payment; written consent was obtained prior to participation. The study was approved by the Institutional Review Boards at Boston College and the Massachusetts Institute of Technology. Two participants were excluded for exhibiting excessive in-scanner movement, identified during spatial preprocessing (see Neural Data Exclusion below). Analyses were conducted on the remaining 28 participants (15 women; age M = 24, SD = 3.92).
Participant Instructions
Participants were told that they would learn information about people, represented by pictures of faces, and that each face would be paired with a sequence of 6 written behavior descriptions. Participants were asked to form impressions of the people that were pictured by imagining them actually performing the behaviors. For each behavior, they were instructed to rate the target’s trustworthiness, based on everything they knew about the person so far.
Sequence Types
Participants learned about 50 individuals represented by male and female faces from the Karolinska Directed Emotional Faces set (Lundqvist et al. 1998). Each face was paired with a sequence of 6 behaviors, which was designed to be either internally inconsistent (80% of targets; “expectation-violation sequences”), or internally consistent (20% of targets; “control sequences”).
There were 4 types of expectation-violation sequences, varying in prior strength and update direction: “Strong Negative-to-Positive” (4 immoral behaviors followed by 2 moral behaviors), “Weak Negative-to-Positive” (2 immoral followed by 2 moral then 2 neutral), “Strong Positive-to-Negative” (4 moral, 2 immoral), and “Weak Positive-to-Negative” (2 moral, 2 immoral, 2 neutral). Two neutral behaviors were added to the ends of weak sequences to keep sequence length constant across sequence types. The neutral behaviors were placed at the end rather than at the beginning of weak sequences, so that participants would not be able to detect the type of any given sequence by the very first behavior. Our aim was to minimize participants’ expectations of the upcoming sequence, and encourage participants to attend equally carefully to each sequence, regardless of sequence type.
For discussion purposes, the 2 behaviors immediately preceding the valence switch point will be referred to as “preswitch” behaviors, while the 2 behaviors immediately following valence switch will be referred to as “postswitch” behaviors.
Additionally, there were 2 types of control sequences: “Negative Control” (6 immoral behaviors) and “Positive Control” (6 moral behaviors). See Table 1 for examples of each sequence type.
Sequence type . | Example . |
---|---|
Strong Negative-to-Positive (strong neg → pos) | (B1) Megan lost her temper at the barista. |
(B2) Megan stood up a first date. | |
(B3) Megan hit a car and left the scene of the accident. | |
(B4) Megan swore at a cashier who made an error on her bill. | |
(B5) Megan created a photo album of the family for her sister’s housewarming gift. | |
(B6) Megan purified a water source for a small village. | |
Weak Negative-to-Positive (weak neg → pos) | (B1) Joshua publicly mocked his sister for stuttering. |
(B2) Joshua got ejected from a game for getting into a fight. | |
(B3) Joshua shared cookies from a care package with his roommates. | |
(B4) Joshua helped an elderly woman with her groceries. | |
(B5) Joshua called a TV station for weather information. | |
(B6) Joshua put gas in the car. | |
Strong Positive-to-Negative (strong pos → neg) | (B1) Thomas had all of his wedding gifts be donations to charity. |
(B2) Thomas gave a stranded motorist a lift to the service station. | |
(B3) Thomas helped his roommate prepare for a big presentation. | |
(B4) Thomas spent a morning volunteering at a nursing home. | |
(B5) Thomas lied to his wife about his location when visiting an ex. | |
(B6) Thomas ordered around his housekeeper in a harsh tone of voice. | |
Weak Positive-to-Negative (weak pos → neg) | (B1) Emily went to her friend’s teachers to get his homework when he was sick. |
(B2) Emily helped a neighbor fix his roof. | |
(B3) Emily broke a valuable vase and blamed her brother. | |
(B4) Emily smoked in a no-smoking section even though others complained. | |
(B5) Emily mailed a letter at the post office. | |
(B6) Emily left her shoes on the doormat. | |
Negative Control (neg control) | (B1) Hannah swore at her roommate for eating her leftovers. |
(B2) Hannah picked a fight on social media with a stranger. | |
(B3) Hannah deliberately tripped an elderly person. | |
(B4) Hannah charged overpriced legal fees to less educated clients. | |
(B5) Hannah tried to steal clothes from a department store but got caught by security. | |
(B6) Hannah deliberately excluded a friend she did not like from weekend plans. | |
Positive Control (pos control) | (B1) Jonathan picked up all the litter at the park. |
(B2) Jonathan spent a Saturday volunteering at a soup kitchen. | |
(B3) Jonathan fixed a friend’s broken laptop. | |
(B4) Jonathan organized a free speech rally against hate in America. | |
(B5) Jonathan helped a blind man pick out items in the grocery store. | |
(B6) Jonathan visited a sick friend in the hospital. |
Sequence type . | Example . |
---|---|
Strong Negative-to-Positive (strong neg → pos) | (B1) Megan lost her temper at the barista. |
(B2) Megan stood up a first date. | |
(B3) Megan hit a car and left the scene of the accident. | |
(B4) Megan swore at a cashier who made an error on her bill. | |
(B5) Megan created a photo album of the family for her sister’s housewarming gift. | |
(B6) Megan purified a water source for a small village. | |
Weak Negative-to-Positive (weak neg → pos) | (B1) Joshua publicly mocked his sister for stuttering. |
(B2) Joshua got ejected from a game for getting into a fight. | |
(B3) Joshua shared cookies from a care package with his roommates. | |
(B4) Joshua helped an elderly woman with her groceries. | |
(B5) Joshua called a TV station for weather information. | |
(B6) Joshua put gas in the car. | |
Strong Positive-to-Negative (strong pos → neg) | (B1) Thomas had all of his wedding gifts be donations to charity. |
(B2) Thomas gave a stranded motorist a lift to the service station. | |
(B3) Thomas helped his roommate prepare for a big presentation. | |
(B4) Thomas spent a morning volunteering at a nursing home. | |
(B5) Thomas lied to his wife about his location when visiting an ex. | |
(B6) Thomas ordered around his housekeeper in a harsh tone of voice. | |
Weak Positive-to-Negative (weak pos → neg) | (B1) Emily went to her friend’s teachers to get his homework when he was sick. |
(B2) Emily helped a neighbor fix his roof. | |
(B3) Emily broke a valuable vase and blamed her brother. | |
(B4) Emily smoked in a no-smoking section even though others complained. | |
(B5) Emily mailed a letter at the post office. | |
(B6) Emily left her shoes on the doormat. | |
Negative Control (neg control) | (B1) Hannah swore at her roommate for eating her leftovers. |
(B2) Hannah picked a fight on social media with a stranger. | |
(B3) Hannah deliberately tripped an elderly person. | |
(B4) Hannah charged overpriced legal fees to less educated clients. | |
(B5) Hannah tried to steal clothes from a department store but got caught by security. | |
(B6) Hannah deliberately excluded a friend she did not like from weekend plans. | |
Positive Control (pos control) | (B1) Jonathan picked up all the litter at the park. |
(B2) Jonathan spent a Saturday volunteering at a soup kitchen. | |
(B3) Jonathan fixed a friend’s broken laptop. | |
(B4) Jonathan organized a free speech rally against hate in America. | |
(B5) Jonathan helped a blind man pick out items in the grocery store. | |
(B6) Jonathan visited a sick friend in the hospital. |
Sequence type . | Example . |
---|---|
Strong Negative-to-Positive (strong neg → pos) | (B1) Megan lost her temper at the barista. |
(B2) Megan stood up a first date. | |
(B3) Megan hit a car and left the scene of the accident. | |
(B4) Megan swore at a cashier who made an error on her bill. | |
(B5) Megan created a photo album of the family for her sister’s housewarming gift. | |
(B6) Megan purified a water source for a small village. | |
Weak Negative-to-Positive (weak neg → pos) | (B1) Joshua publicly mocked his sister for stuttering. |
(B2) Joshua got ejected from a game for getting into a fight. | |
(B3) Joshua shared cookies from a care package with his roommates. | |
(B4) Joshua helped an elderly woman with her groceries. | |
(B5) Joshua called a TV station for weather information. | |
(B6) Joshua put gas in the car. | |
Strong Positive-to-Negative (strong pos → neg) | (B1) Thomas had all of his wedding gifts be donations to charity. |
(B2) Thomas gave a stranded motorist a lift to the service station. | |
(B3) Thomas helped his roommate prepare for a big presentation. | |
(B4) Thomas spent a morning volunteering at a nursing home. | |
(B5) Thomas lied to his wife about his location when visiting an ex. | |
(B6) Thomas ordered around his housekeeper in a harsh tone of voice. | |
Weak Positive-to-Negative (weak pos → neg) | (B1) Emily went to her friend’s teachers to get his homework when he was sick. |
(B2) Emily helped a neighbor fix his roof. | |
(B3) Emily broke a valuable vase and blamed her brother. | |
(B4) Emily smoked in a no-smoking section even though others complained. | |
(B5) Emily mailed a letter at the post office. | |
(B6) Emily left her shoes on the doormat. | |
Negative Control (neg control) | (B1) Hannah swore at her roommate for eating her leftovers. |
(B2) Hannah picked a fight on social media with a stranger. | |
(B3) Hannah deliberately tripped an elderly person. | |
(B4) Hannah charged overpriced legal fees to less educated clients. | |
(B5) Hannah tried to steal clothes from a department store but got caught by security. | |
(B6) Hannah deliberately excluded a friend she did not like from weekend plans. | |
Positive Control (pos control) | (B1) Jonathan picked up all the litter at the park. |
(B2) Jonathan spent a Saturday volunteering at a soup kitchen. | |
(B3) Jonathan fixed a friend’s broken laptop. | |
(B4) Jonathan organized a free speech rally against hate in America. | |
(B5) Jonathan helped a blind man pick out items in the grocery store. | |
(B6) Jonathan visited a sick friend in the hospital. |
Sequence type . | Example . |
---|---|
Strong Negative-to-Positive (strong neg → pos) | (B1) Megan lost her temper at the barista. |
(B2) Megan stood up a first date. | |
(B3) Megan hit a car and left the scene of the accident. | |
(B4) Megan swore at a cashier who made an error on her bill. | |
(B5) Megan created a photo album of the family for her sister’s housewarming gift. | |
(B6) Megan purified a water source for a small village. | |
Weak Negative-to-Positive (weak neg → pos) | (B1) Joshua publicly mocked his sister for stuttering. |
(B2) Joshua got ejected from a game for getting into a fight. | |
(B3) Joshua shared cookies from a care package with his roommates. | |
(B4) Joshua helped an elderly woman with her groceries. | |
(B5) Joshua called a TV station for weather information. | |
(B6) Joshua put gas in the car. | |
Strong Positive-to-Negative (strong pos → neg) | (B1) Thomas had all of his wedding gifts be donations to charity. |
(B2) Thomas gave a stranded motorist a lift to the service station. | |
(B3) Thomas helped his roommate prepare for a big presentation. | |
(B4) Thomas spent a morning volunteering at a nursing home. | |
(B5) Thomas lied to his wife about his location when visiting an ex. | |
(B6) Thomas ordered around his housekeeper in a harsh tone of voice. | |
Weak Positive-to-Negative (weak pos → neg) | (B1) Emily went to her friend’s teachers to get his homework when he was sick. |
(B2) Emily helped a neighbor fix his roof. | |
(B3) Emily broke a valuable vase and blamed her brother. | |
(B4) Emily smoked in a no-smoking section even though others complained. | |
(B5) Emily mailed a letter at the post office. | |
(B6) Emily left her shoes on the doormat. | |
Negative Control (neg control) | (B1) Hannah swore at her roommate for eating her leftovers. |
(B2) Hannah picked a fight on social media with a stranger. | |
(B3) Hannah deliberately tripped an elderly person. | |
(B4) Hannah charged overpriced legal fees to less educated clients. | |
(B5) Hannah tried to steal clothes from a department store but got caught by security. | |
(B6) Hannah deliberately excluded a friend she did not like from weekend plans. | |
Positive Control (pos control) | (B1) Jonathan picked up all the litter at the park. |
(B2) Jonathan spent a Saturday volunteering at a soup kitchen. | |
(B3) Jonathan fixed a friend’s broken laptop. | |
(B4) Jonathan organized a free speech rally against hate in America. | |
(B5) Jonathan helped a blind man pick out items in the grocery store. | |
(B6) Jonathan visited a sick friend in the hospital. |
Stimulus Balancing
Three hundred written descriptions of behaviors were used to generate 50 unique sequences of 6 behaviors each. A portion of the behavior descriptions were adapted from previous studies (Mende-Siedlecki et al. 2013; Mende-Siedlecki and Todorov 2016). The behavior descriptions were constructed to be relevant to morality, clearly valenced, and varying in intensity and perceived frequency. Ten sequences were generated for each of the 4 types of expectation-violation sequences; 5 sequences were generated for each of the 2 types of control sequences.
The expectation-violation sequences were constructed so as to control for a set of stimulus features: moral relevance, perceived frequency, emotional valence, emotional arousal, trustworthiness, and intelligence. Ratings for each feature were collected from separate groups of Amazon Mechanical Turk participants (N |$\approx$|30/behavior). Two sample t-tests showed that these features did not differ significantly (P > 0.10) across moral and immoral behaviors, across the switch point, and across weak and strong priors within update direction.
To ensure that differences between sequence types would not be a function of specific pairings of preswitch and postswitch behaviors, we shuffled the postswitch behaviors across participants so that they were seen following both weak and strong priors. Additionally, target name and associated target face were counterbalanced with update direction across participants, in order to control for participants’ chance associations with specific names or facial features. For example, for one half of participants, Thomas appeared in the positive → negative direction, and Emily in the negative → positive direction; for the other half of participants, Thomas appeared in the negative → positive direction, and Emily in the positive → negative direction. We expected that chance associations with specific names or facial features would not all be of the same valence, and, crucially, that experimental effects would be obscured, but not enhanced, by chance associations. These counterbalancing schemes resulted in 4 total stimulus lists.
Presentation
The stimuli were presented using PsychoPy v1.85.6 (Peirce 2007). Fifty total sequences were presented over ten 5.5-min runs. Each run consisted of 5 sequences: one each of the expectation-violation sequences, and one control sequence. At the beginning of each sequence, the target face was presented with an introductory sentence (“This is Thomas”) for 2 s (Fig. 1). Next, the face was presented with a sequence of 6 written behavior descriptions for 6 s each with jittered fixation (2, 4, or 6 s, pseudorandomly assigned to keep sequence duration constant) between each face-behavior pair. On each behavior presentation, participants rated the target’s trustworthiness on a scale from 1 (least trustworthy) to 7 (most trustworthy) using a button box. Participants were randomly assigned to one of 4 stimulus lists, and run order was randomized for each participant. Trial order within run was pseudorandomized such that, over the course of the experiment, run-initial and run-final trials were distributed evenly across sequence type.
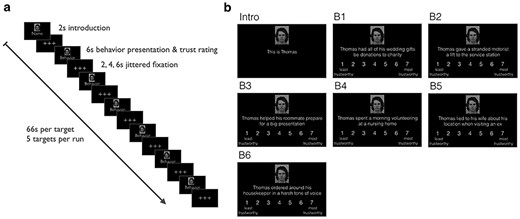
In-scanner stimulus presentation. (a) At the beginning of each sequence, the target face was presented with an introductory sentence (“This is Thomas”) for 2 s. Next, the face was presented with a sequence of 6 behaviors, with jittered fixation (2, 4, or 6 s) between each face-behavior presentation. (b) For each behavior, participants rated the target’s trustworthiness on a scale from 1 (least trustworthy) to 7 (most trustworthy).
MRI Data Acquisition and Processing
The MRI data were collected using a 32-channel head coil in a 3 T Siemens Prisma scanner at the Athinoula A. Martinos Imaging Center at the Massachusetts Institute of Technology. Functional volumes were acquired in 32 3 × 3 × 3 mm slices using a gradient-echo sequence (TR = 2 s, TE = 30 ms, flip angle = 90). The first 6 s of each run were excluded to allow for steady state magnetization. Before the functional scans, high-resolution structural images were acquired (1 mm isotropic MPRAGE, TR = 2.53 s, TE = 1.69 ms).
Data processing and analysis were performed using fMRIPrep (Esteban et al. (2019); see Supplementary Materials p. 1 for details), SPM12 (https://www.fil.ion.ucl.ac.uk/spm/software/spm12/), and custom software. The functional data were realigned, coregistered to the anatomical image, normalized onto a common brain space (Montreal Neurological Institute, MNI, template), spatially smoothed using a Gaussian filter (full-width half-maximum = 8 mm kernel), and high-pass filtered (128 s).
Postscan Measures
Following the scanning procedure, participants completed a series of additional behavioral measures: 1) a scenario-based scale of Willingness to Forgive (DeShea 2003); 2) a measure of entity versus incremental beliefs about morality (Chiu et al. 1997; Hughes 2015); and 3) surprisingness ratings for all behaviors in all expectation-violation sequences seen in the scanner (“Given what you know so far, how surprising is this behavior?” on a 1–7 scale).
Mixed Effects Models for Behavioral and PSC Data
Linear mixed effects models were constructed in R (RCore T 2016) for all behavioral data and all PSC data (package: “lme4”; Bates et al. 2014). All models initially included by-subject and by-item random intercepts. If a model failed to converge or had singular fit, we simplified the random effects structure by removing random intercepts with near-zero variance until convergence was achieved. If the random intercept for subject or item was dropped from a model, this was denoted in the results as “no by-subject intercepts” or “no by-item intercepts”, respectively; if the random intercepts for both subject and item were dropped from a model, this was denoted as “no random intercepts”. To obtain P-values for fixed effects, we conducted likelihood ratio tests of the full model against the model with all predictors except for the predictor of interest. We report semipartial R-squared values (coefficients of determination; Edwards et al. 2008) as effect sizes for fixed effects.
Analyses of Trustworthiness Ratings
To examine behavioral impression updating, we computed an updating measure for each expectation-violation trial. For neg → pos targets, this was calculated as the difference between the average trustworthiness rating for the 2 postswitch behaviors and the average trustworthiness rating for the 2 preswitch behaviors. For pos → neg targets, we multiplied this measure by −1. Here, we reverse the sign of the difference (rather than taking the absolute value) to prevent overestimation of update magnitude that may occur if participants update in the unanticipated direction.
We conducted linear mixed effects analyses to test whether impression updating differed as a function of prior strength (weak or strong) and update direction (pos → neg or neg → pos). We also examined the effect of prior strength on average trust ratings for the 2 preswitch behaviors and for the 2 postswitch behaviors.
Analyses of Postscan Measures
We analyzed surprisingness ratings as a function of prior strength and update direction. We also correlated participants’ scores on the Willingness to Forgive scale with behavioral impression updating, and participants’ scores on the entity versus incremental morality measure with behavioral impression updating.
Neural Data Exclusion
Individual functional runs were removed from further analysis if the participant exhibited >3 mm movement at any point during the run, or if the average framewise displacement for the run exceeded 1 mm. Participants were excluded if more than 1/3 of collected functional runs were dropped. This resulted in the exclusion of 2 participants (of 30 scanned participants).
ROI Analyses
A ToM localizer task (Saxe and Kanwisher 2003; Dodell-Feder et al. 2011) was used to functionally define 4 regions of interest (ROIs): DMPFC (N = 21), right temporo-parietal junction (RTPJ, N = 27), left temporo-parietal junction (LTPJ, N = 27), and PC (N = 27). ROIs were defined as all voxels within a 9-mm radius of the peak voxel that passed threshold in the contrast “false belief > false photo” (P < 0.001, uncorrected; k > 16, computed via 1000 iterations of a Monte-Carlo simulation, Slotnick et al. 2003). We used the same ROI selection parameters as previous neuroimaging research examining ToM regions (Tsoi et al. 2018; Dungan and Young 2019). See Supplementary Table 1 for peak coordinates and Supplementary Fig. 1 for visualization.
As there were 6 sequence types (Table 1), and 6 behaviors in each sequence, the ordinal position of a behavior (first through sixth) within a sequence type was treated as a single “condition.” This resulted in 36 total conditions (6 ordinal positions * 6 sequence types). For example, the first behavior in Strong Negative-to-Positive sequences was treated as one condition; the second behavior in Strong Negative-to-Positive sequences was treated as a different condition. It was important to distinguish between behaviors in different ordinal positions, as we were interested in neural responses to inconsistencies that arose at different points in a sequence. In each ROI, the PSC relative to baseline was calculated for each time point for each condition, averaging across all voxels in the ROI. Baseline response, calculated separately for each run, was the average over time of the responses to fixation. PSC for each timepoint for each condition was calculated as 100[(average response for condition at time t – baseline)/baseline]. Timepoints that exhibited >1 mm frame-wise displacement compared with the previous timepoint were removed prior to further analysis. PSC values were averaged across each 6-second behavior presentation (offset 4 s from presentation time to adjust for hemodynamic lag) to estimate a single PSC for each condition in each ROI.
Analyses of Neural Activity in Response to Postswitch Versus Preswitch Behaviors
In each ROI, we compared average PSC for the 2 preswitch behaviors with average PSC for the 2 postswitch behaviors, collapsing across sequence type. We also compared PSC for the preswitch behaviors with PSC for the postswitch behaviors within each sequence type.
Analyses of Neural Updating
To examine neural activity associated with impression updating, we predicted PSC for the postswitch behaviors, as a function of prior strength (weak or strong) and update direction (pos → neg or neg → pos), controlling for activity for the preswitch behaviors.
We also computed a neural updating measure for each expectation-violation sequence by taking the difference of the average PSC for the 2 postswitch behaviors and the average PSC for the 2 preswitch behaviors.
Brain-Behavior Correlations
To explore the relationship between updating-related neural activity in each ToM ROI and behavioral impression updating, we ran linear mixed effects models predicting the magnitude of behavioral updating on each trial, with neural updating as a fixed effect.
Feature Encoding Models
For whole-brain analyses, we used a set of encoding models to predict activity evoked by a wide range of stimulus features that varied between behavior positions. We created parametric regressors coding for 12 behavior-wise stimulus features (see Table 2). For each behavior presentation, feature values were applied to all 3 images corresponding to the behavior. Face-only presentations were modeled separately using a condition regressor. To correct for multiple comparisons, images from group-level analyses were subjected to a voxel-wise threshold of P < 0.001 (uncorrected) and a cluster extent threshold ensuring P < 0.05 (familywise error rate-corrected; applied in SPM).
Top: behavior-wise stimulus features; bottom: regressors included in each encoding model
ID . | Feature description . | Values . |
---|---|---|
1 | Ordinal position of behavior | 1, 2, 3, 4, 5, 6 |
2 | Valence of current behavior | 1 for positive; −1 for negative; 0 for neutral |
3 | Cumulative # of consecutive positive behaviors | 0, 1, 2, 3, 4, 5, 6 |
4 | Cumulative # of consecutive negative behaviors | 0, 1, 2, 3, 4, 5, 6 |
5 | Change occurred: positive/negative previous behavior to neutral current behavior | 1 for pos → neutral; −1 for neg → neutral; 0 otherwise |
6 | Change occurred: positive/negative previous behavior to negative/positive current behavior | 1 for pos → neg; −1 for neg → pos; 0 otherwise |
7 | Magnitude of trustworthiness update from previous behavior | 0, 1, 2, 3, 4, 5, 6 |
8 | Positive trustworthiness update from previous behavior | 0, 1, 2, 3, 4, 5, 6 |
9 | Negative trustworthiness update from previous behavior | 0, −1, −2, −3, −4, −5, −6 |
10 | Any valence change occurred from previous behavior | 1 for pos → neutral, neg → neutral, pos → neg, neg → pos; 0 otherwise |
11 | Any valence reversal occurred from previous behavior | 1 for pos → neg, neg → pos; 0 otherwise |
12 | Cumulative # of consecutive valenced behaviors | 1, 2, 3, 4, 5, 6 |
ID | Model description | Regressors included |
A | Cumulative strength of positive/negative prior | 1, 2, 5, 6, 3, 4 |
B | Change in valence occurred from previous behavior | 1, 2, 3, 4, 5, 6 |
C | Valence of current behavior | 1, 3, 4, 5, 6, 2 |
D | Cumulative strength of prior | 1, 2, 5, 6, 12 |
E | Any valence change occurred from previous behavior | 1, 2, 3, 4, 10 |
F | Any valence reversal occurred from previous behavior | 1, 2, 3, 4, 11 |
G | Magnitude of behavioral updating | 1, 7, 2, 3, 4, 5, 6 |
H | Positive/negative behavioral updating | 1, 8, 9, 2, 3, 4, 5, 6 |
ID . | Feature description . | Values . |
---|---|---|
1 | Ordinal position of behavior | 1, 2, 3, 4, 5, 6 |
2 | Valence of current behavior | 1 for positive; −1 for negative; 0 for neutral |
3 | Cumulative # of consecutive positive behaviors | 0, 1, 2, 3, 4, 5, 6 |
4 | Cumulative # of consecutive negative behaviors | 0, 1, 2, 3, 4, 5, 6 |
5 | Change occurred: positive/negative previous behavior to neutral current behavior | 1 for pos → neutral; −1 for neg → neutral; 0 otherwise |
6 | Change occurred: positive/negative previous behavior to negative/positive current behavior | 1 for pos → neg; −1 for neg → pos; 0 otherwise |
7 | Magnitude of trustworthiness update from previous behavior | 0, 1, 2, 3, 4, 5, 6 |
8 | Positive trustworthiness update from previous behavior | 0, 1, 2, 3, 4, 5, 6 |
9 | Negative trustworthiness update from previous behavior | 0, −1, −2, −3, −4, −5, −6 |
10 | Any valence change occurred from previous behavior | 1 for pos → neutral, neg → neutral, pos → neg, neg → pos; 0 otherwise |
11 | Any valence reversal occurred from previous behavior | 1 for pos → neg, neg → pos; 0 otherwise |
12 | Cumulative # of consecutive valenced behaviors | 1, 2, 3, 4, 5, 6 |
ID | Model description | Regressors included |
A | Cumulative strength of positive/negative prior | 1, 2, 5, 6, 3, 4 |
B | Change in valence occurred from previous behavior | 1, 2, 3, 4, 5, 6 |
C | Valence of current behavior | 1, 3, 4, 5, 6, 2 |
D | Cumulative strength of prior | 1, 2, 5, 6, 12 |
E | Any valence change occurred from previous behavior | 1, 2, 3, 4, 10 |
F | Any valence reversal occurred from previous behavior | 1, 2, 3, 4, 11 |
G | Magnitude of behavioral updating | 1, 7, 2, 3, 4, 5, 6 |
H | Positive/negative behavioral updating | 1, 8, 9, 2, 3, 4, 5, 6 |
Notes: Twelve parametric regressors were used to describe stimulus features that varied between behavior positions (top). Regressor IDs are listed in the order in which they were added to the model; each regressor was serially orthogonalized with respect to the previous regressor (bottom). Parameter estimates were extracted for bolded regressors
Top: behavior-wise stimulus features; bottom: regressors included in each encoding model
ID . | Feature description . | Values . |
---|---|---|
1 | Ordinal position of behavior | 1, 2, 3, 4, 5, 6 |
2 | Valence of current behavior | 1 for positive; −1 for negative; 0 for neutral |
3 | Cumulative # of consecutive positive behaviors | 0, 1, 2, 3, 4, 5, 6 |
4 | Cumulative # of consecutive negative behaviors | 0, 1, 2, 3, 4, 5, 6 |
5 | Change occurred: positive/negative previous behavior to neutral current behavior | 1 for pos → neutral; −1 for neg → neutral; 0 otherwise |
6 | Change occurred: positive/negative previous behavior to negative/positive current behavior | 1 for pos → neg; −1 for neg → pos; 0 otherwise |
7 | Magnitude of trustworthiness update from previous behavior | 0, 1, 2, 3, 4, 5, 6 |
8 | Positive trustworthiness update from previous behavior | 0, 1, 2, 3, 4, 5, 6 |
9 | Negative trustworthiness update from previous behavior | 0, −1, −2, −3, −4, −5, −6 |
10 | Any valence change occurred from previous behavior | 1 for pos → neutral, neg → neutral, pos → neg, neg → pos; 0 otherwise |
11 | Any valence reversal occurred from previous behavior | 1 for pos → neg, neg → pos; 0 otherwise |
12 | Cumulative # of consecutive valenced behaviors | 1, 2, 3, 4, 5, 6 |
ID | Model description | Regressors included |
A | Cumulative strength of positive/negative prior | 1, 2, 5, 6, 3, 4 |
B | Change in valence occurred from previous behavior | 1, 2, 3, 4, 5, 6 |
C | Valence of current behavior | 1, 3, 4, 5, 6, 2 |
D | Cumulative strength of prior | 1, 2, 5, 6, 12 |
E | Any valence change occurred from previous behavior | 1, 2, 3, 4, 10 |
F | Any valence reversal occurred from previous behavior | 1, 2, 3, 4, 11 |
G | Magnitude of behavioral updating | 1, 7, 2, 3, 4, 5, 6 |
H | Positive/negative behavioral updating | 1, 8, 9, 2, 3, 4, 5, 6 |
ID . | Feature description . | Values . |
---|---|---|
1 | Ordinal position of behavior | 1, 2, 3, 4, 5, 6 |
2 | Valence of current behavior | 1 for positive; −1 for negative; 0 for neutral |
3 | Cumulative # of consecutive positive behaviors | 0, 1, 2, 3, 4, 5, 6 |
4 | Cumulative # of consecutive negative behaviors | 0, 1, 2, 3, 4, 5, 6 |
5 | Change occurred: positive/negative previous behavior to neutral current behavior | 1 for pos → neutral; −1 for neg → neutral; 0 otherwise |
6 | Change occurred: positive/negative previous behavior to negative/positive current behavior | 1 for pos → neg; −1 for neg → pos; 0 otherwise |
7 | Magnitude of trustworthiness update from previous behavior | 0, 1, 2, 3, 4, 5, 6 |
8 | Positive trustworthiness update from previous behavior | 0, 1, 2, 3, 4, 5, 6 |
9 | Negative trustworthiness update from previous behavior | 0, −1, −2, −3, −4, −5, −6 |
10 | Any valence change occurred from previous behavior | 1 for pos → neutral, neg → neutral, pos → neg, neg → pos; 0 otherwise |
11 | Any valence reversal occurred from previous behavior | 1 for pos → neg, neg → pos; 0 otherwise |
12 | Cumulative # of consecutive valenced behaviors | 1, 2, 3, 4, 5, 6 |
ID | Model description | Regressors included |
A | Cumulative strength of positive/negative prior | 1, 2, 5, 6, 3, 4 |
B | Change in valence occurred from previous behavior | 1, 2, 3, 4, 5, 6 |
C | Valence of current behavior | 1, 3, 4, 5, 6, 2 |
D | Cumulative strength of prior | 1, 2, 5, 6, 12 |
E | Any valence change occurred from previous behavior | 1, 2, 3, 4, 10 |
F | Any valence reversal occurred from previous behavior | 1, 2, 3, 4, 11 |
G | Magnitude of behavioral updating | 1, 7, 2, 3, 4, 5, 6 |
H | Positive/negative behavioral updating | 1, 8, 9, 2, 3, 4, 5, 6 |
Notes: Twelve parametric regressors were used to describe stimulus features that varied between behavior positions (top). Regressor IDs are listed in the order in which they were added to the model; each regressor was serially orthogonalized with respect to the previous regressor (bottom). Parameter estimates were extracted for bolded regressors
Table 2 lists the regressors that were included in each model. We were primarily interested in regions that track: prior strength, controlling for position of current behavior, valence of current behavior, and whether a valence change occurred (models A, D); whether a valence change occurred, controlling for position of current behavior, valence of current behavior, and prior strength (models B, F); and trial-wise impression updating, controlling for position of current behavior (models G, H). In constructing these models, each regressor was serially orthogonalized with respect to the previous regressor; the ordinal position regressor was always entered first. Rotating which regressors were added last across these different models, and examining parameter estimates of these regressors, allowed us to examine unique neural variance explained by each feature (Mumford et al. 2015).
Results
Behavioral Results
Impression Updating
Participants rated trustworthiness on a scale from 1 to 7. An “updating measure” (see Materials and Methods) was calculated for each sequence type. The interaction between update direction and prior strength was not significant (B = −0.267, SE = 0.202, χ2(1) = 1.721, P = 0.190). There was a main effect of update direction (pos → neg > neg → pos, B = 1.454, SE = 0.102, χ2(1) = 98.846, P < 0.001, semipartial R2 = 0.722), and no main effect of prior strength (B = −0.103, SE = 0.102, χ2(1) = 1.005, P = 0.316, Rß2 = 0.013). Thus, participants updated their impressions to a greater extent following the violation of positive priors. In other words, participants engaged in more negative updating than positive updating (Fig. 2).
![(a) Mean trustworthiness ratings for preswitch behaviors and postswitch behaviors, for each sequence type. Error bars indicate 95% CIs. (b) Mean magnitude of impression update, for each sequence type. For neg → pos targets, this was calculated as: (average rating for 2 postswitch behaviors)—(average rating for 2 preswitch behaviors). For pos → neg targets, this was calculated as: −1 * [(average rating for 2 postswitch behaviors)—(average rating for 2 preswitch behaviors)]. For control targets, this was calculated as: (average rating for last 2 behaviors)—(average rating for middle 2 behaviors). The maximum value of the impression update is 6, as the trustworthiness scale runs from 1 to 7.](https://oup.silverchair-cdn.com/oup/backfile/Content_public/Journal/cercor/31/2/10.1093_cercor_bhaa263/2/m_bhaa263f2.jpeg?Expires=1749969520&Signature=pbsx3BgK7xiT0MiNKYHpZg7UpGMCkcMLOPg8jorwQEy4mcJEbSiLV1Y0eXkZbpP6mMk3dHTylGOV08Sc8dVoO~fWLfq3SQxu2OEaDoh7zBujEbYc9hpr9GqZ0TYG3BsF4IJVEuH1pRaCjM0v5sjQWeMk255-1lqvQ6nSI8k4189CSuknbordyUWk44c7~PuTUY-7NI9UCqrrA6JeZc9AEfDjG~eHo9NbDZ56HegSS3gVhH-m0qyGAU5w4tijBMWu8IxQlBO-z1CwbCzzNY6CVve6K-OqVUzIuruEZe-JlNziL~MivbJ4AdX-Z7espSo7fF3yJ~zfgrWKUbYv94RBFQ__&Key-Pair-Id=APKAIE5G5CRDK6RD3PGA)
(a) Mean trustworthiness ratings for preswitch behaviors and postswitch behaviors, for each sequence type. Error bars indicate 95% CIs. (b) Mean magnitude of impression update, for each sequence type. For neg → pos targets, this was calculated as: (average rating for 2 postswitch behaviors)—(average rating for 2 preswitch behaviors). For pos → neg targets, this was calculated as: −1 * [(average rating for 2 postswitch behaviors)—(average rating for 2 preswitch behaviors)]. For control targets, this was calculated as: (average rating for last 2 behaviors)—(average rating for middle 2 behaviors). The maximum value of the impression update is 6, as the trustworthiness scale runs from 1 to 7.
Effect of Prior Strength on Ratings
We also examined the effect of prior strength on average trust ratings elicited by the 2 preswitch behaviors and by the 2 postswitch behaviors. The preswitch behaviors in the Strong Positive-to-Negative condition elicited more positive trust ratings than the preswitch behaviors in the Weak Positive-to-Negative condition (no by-item intercepts; B = −0.455, SE = 0.060, χ2(1) = 61.931, P < 0.001, Rß2 = 0.119). The preswitch behaviors in the Strong Negative-to-Positive condition elicited more negative trust ratings than the preswitch behaviors in the Weak Negative-to-Positive condition (B = 0.608, SE = 0.127, χ2(1) = 18.21, P < 0.001, Rß2 = 0.374). In other words, impressions based on 4 positive behaviors more less positive than impressions based on 2 positive behaviors, and impressions based on 4 negative behaviors were more negative than impressions based on 2 negative behaviors (Fig. 2).
There was no effect of prior strength on trust ratings elicited by negative postswitch behaviors (B = −0.233, SE = 0.185, χ2(1) = 1.556, P = 0.212, Rß2 = 0.04), but postswitch behaviors in the Strong Negative-to-Positive condition elicited more negative trust ratings than postswitch behaviors in the Weak Negative-to-Positive condition (B = 0.667, SE = 0.144, χ2(1) =17.102, P < 0.001, Rß2 = 0.362). That is, more negative preswitch ratings were followed by more negative postswitch ratings (Fig. 2). Prior strength thus affected preswitch impression ratings, and, to some extent, postswitch impression ratings.
Surprisingness Ratings
After the scan session, participants were presented with the same expectation-violation sequences they had seen in the scanner. For each behavior in each sequence, participants rated the surprisingness of the behavior on a scale from 1 (least surprising) to 7 (most surprising). We examined average surprisingness ratings for the postswitch behaviors. The interaction between update direction and prior strength was not significant (B = −0.141, SE = 0.158, χ2(1) = 0.792, P = 0.373). There was a main effect of prior strength on postswitch surprisingness (weak < strong, B = −0.214, SE = 0.0791, χ2(1) = 7.035, P = 0.008, Rß2 = 0.089), and no main effect of update direction (B = −0.028, SE = 0.080, χ2(1) = 0.127, P = 0.722, Rß2 = 0.002). Thus, inconsistent behaviors following strong priors were rated as more surprising, compared with inconsistent behaviors following weak priors; there was no difference in surprisingness for inconsistent behaviors following positive versus negative priors (Fig. 3).
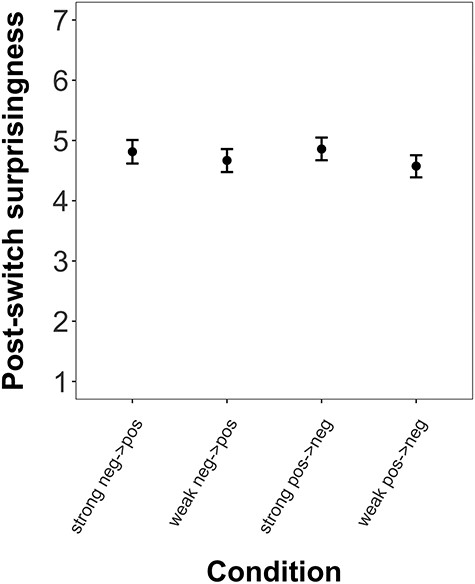
Mean surprisingness ratings for postswitch behaviors, for each sequence type. Error bars indicate 95% CIs.
Individual Difference Measures
Following the scan, participants completed a scale of Willingness to Forgive (DeShea 2003), and a measure of entity versus incremental beliefs about morality (Chiu et al. 1997; Hughes 2015). Neither of these measures significantly predicted magnitude of behavioral updating (Willingness to Forgive: ß = 0.04, SE = 0.132, χ2(1) = 0.091, P = 0.763; entity vs. incremental: ß = −0.108, SE = 0.134, χ2(1) = 0.639, P = 0.424).
Neural Results
Neural Activity in Response to Postswitch Versus Preswitch Behaviors
Collapsing across sequence type, all 4 ToM ROIs exhibited greater activity in response to postswitch behaviors than to preswitch behaviors (DMPFC: χ2(1) = 30.148, P < 0.001; LTPJ: χ2(1) = 6.353, P = 0.012; RTPJ: no by-item intercepts, χ2(1) = 19.286, P < 0.001; PC: χ2(1) = 8.058, P = 0.005). See Supplementary Table 2 for analyses by sequence type.
Neural Activity Related to Updating
We looked at PSC for the postswitch behaviors, controlling for activity during the preswitch behaviors (Fig. 4). For an alternative analysis using the neural updating measure, see Supplementary Materials p. 6. In DMPFC, there was a significant main effect of update direction (pos → neg > neg → pos, χ2(1) = 15.41, P < 0.001), and a significant main effect of prior strength (strong > weak, χ2(1) = 6.647, P = 0.010). In LTPJ, there was a significant main effect of update direction (pos → neg > neg → pos, χ2(1) = 14.889, P < 0.001), and no main effect of prior strength (χ2(1) = 0.857, P = 0.355). In RTPJ, there was no main effect of update direction (χ2(1) = 0.981, P = 0.322), and a significant main effect of prior strength (strong > weak, χ2(1) = 8.253, P = 0.004). In PC, there was no main effect of update direction (χ2(1) = 1.164, P = 0.281), and no main effect of prior strength (χ2(1) = 1.815, P = 0.178).
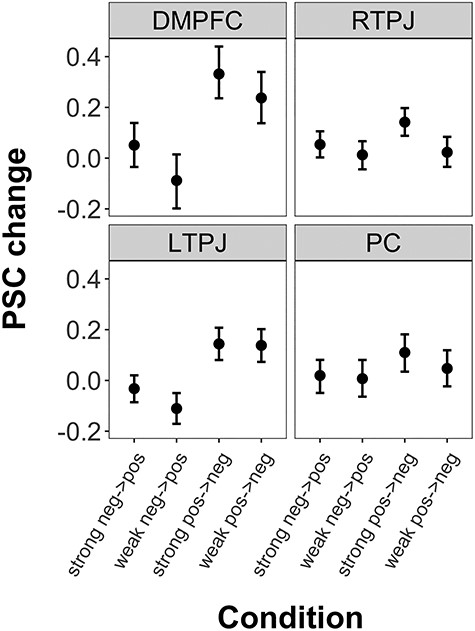
Mean changes in PSC from preswitch behaviors to postswitch behaviors, for each ROI and sequence type. Error bars indicate 95% CIs.
Summary of PSC Analyses
We examined neural activity in response to postswitch behaviors, controlling for neural activity in response to preswitch behaviors. This analysis revealed an effect of update direction (negative updating > positive updating) in DMPFC and LTPJ, and an effect of prior strength (strong > weak) in DMPFC and RTPJ.
Updating Versus Mere Valence
To test the possibility that DMPFC and LTPJ are exhibiting a mere valence effect (i.e., greater activity to negative vs. positive behaviors), rather than an updating effect, we compared the neural updating measure for pos → neg sequences with an analogous nonupdating measure for control sequences. For example, we compared the neural updating measure for Weak Positive-to-Negative trials with an analogous measure for Negative Control trials: average activity to the middle 2 behaviors minus average activity to the first 2 behaviors.
If activities in DMPFC and LTPJ are solely tracking valence, then we would expect to see no differences between these measures in these ROIs. However, if DMPFC and LTPJ also track updating, then we would expect to see a greater change in activity on updating trials compared with nonupdating trials.
When comparing Strong Positive-to-Negative trials with Negative Control trials, we found a significant effect of updating in both DMPFC (no random intercepts; F(1, 296) = 16.41, P < 0.001) and LTPJ (no by-item intercepts; χ2(1) = 10.143, P = 0.001). When comparing Weak Positive-to-Negative trials with Negative Control trials, we found a significant effect of updating in DMPFC (χ2(1) = 7.046, P = 0.008) and LTPJ (no by-item intercepts; χ2(1) = 8.681, P = 0.003).
These analyses suggest that DMPFC and LTPJ are responding not just to negative valence, but also to meaningful changes in behavior. For additional analyses comparing updating measures with nonupdating measures, see Supplementary Tables 3 and 4.
Brain-Behavior Analyses in ToM ROIs
Within each ROI, we examined the relationship between neural updating and behavioral impression updating. Collapsing across sequence type, no brain-behavior relationship was observed in any of the ToM ROIs (DMPFC: ß = 0.007, SE = 0.026, χ2(1) = 0.076, P = 0.782; LTPJ: ß = −0.009, SE = 0.021, χ2(1) = 0.195, P = 0.659; RTPJ: ß = −0.033, SE = 0.020, χ2(1) = 2.661, P = 0.103; PC: ß = −0.015, SE = 0.020, χ2(1) = 0.538, P = 0.463). See Supplementary Materials p. 6 for analyses within sequence type.
Encoding Model Analyses
We built a set of encoding models to predict activity in voxels evoked by stimulus features that varied between behavior positions (see Table 3 for peak coordinates). We were chiefly interested in regions that track: 1) prior strength, controlling for position of current behavior, valence of current behavior, and whether a valence change occurred; 2) whether a valence change occurred, controlling for position of current behavior, valence of current behavior, and prior strength; and 3) trial-wise impression updating, controlling for position of current behavior. For other encoding model results, see Supplementary Table 5; for results from condition-based GLM analyses, see Supplementary Tables 6–8.
Prior strength: Activity in posterior cingulum (which overlaps with PC as elicited by the ToM localizer task) parametrically covaried with the cumulative number of consecutive positive or negative behaviors presented. Activity in left middle temporal gyrus (LTPJ) and left superior frontal gyrus tracked the cumulative number of consecutive positive behaviors presented.Activity in left posterior cingulum, left calcarine fissure, left superior frontal gyrus, left middle temporal gyrus, and left angular gyrus tracked the cumulative number of consecutive negative behaviors presented.
Whether a valence change occurred: Activity in right superior frontal gyrus (DMPFC), PC, and right inferior frontal gyrus (IFG)–orbital part (VLPFC, anterior insula) tracked valence reversals (pos → neg or neg → pos). No significant clusters responded preferentially to pos → neg changes in valence, and no significant clusters responded preferentially to neg → pos changes in valence.
Trial-wise impression updating: Activity in left superior temporal pole (VLPFC/IFG), right IFG–orbital part (VLPFC), left SMA, and left precentral gyrus tracked the magnitude of trial-wise behavioral updating (see Supplementary Fig. 2 for visualization).
Activity in left IFG–orbital part (VLPFC/IFG), left superior frontal gyrus (DMPFC), right insula, left calcarine fissure, left caudate, right superior parietal gyrus, right caudate, and left middle temporal gyrus tracked trial-wise negative behavioral updating.
No significant clusters tracked trial-wise positive behavioral updating.
Region name . | x . | y . | z . | t value . | # voxels . | ToM . | VLPFC/IFG . |
---|---|---|---|---|---|---|---|
Model D: cumulative number of consecutive positive or negative behaviors presented | |||||||
Posterior cingulum | −3 | −49 | 31 | 8.39 | 259 | PC | |
Model A: cumulative number of consecutive positive behaviors presented | |||||||
Left middle temporal gyrus | −57 | −55 | 19 | 5.22 | 219 | LTPJ | |
Left superior frontal gyrus | −3 | 53 | 19 | 5.04 | 312 | ||
Model A: cumulative number of consecutive negative behaviors presented | |||||||
Left posterior cingulum | −3 | −49 | 28 | 9.07 | 387 | ||
Left calcarine fissure | −9 | −97 | −5 | 7.38 | 168 | ||
Left superior frontal gyrus | −6 | 44 | 49 | 6.53 | 951 | ||
Left middle temporal gyrus | −63 | −19 | −14 | 5.64 | 175 | ||
Left angular gyrus | −57 | −70 | 34 | 5.05 | 140 | ||
Model F: valence reversal (from pos → neg or neg → pos) | |||||||
Right superior frontal gyrus (medial) | 6 | 53 | 28 | 6.29 | 219 | DMPFC | |
Precuneus | 9 | −67 | 49 | 5.04 | 102 | ||
Right inferior frontal gyrus (orbital part) | 36 | 23 | −8 | 4.94 | 180 | RVLPFC | |
Model G: magnitude of trial-wise behavioral updating | |||||||
Left superior temporal pole | −42 | 20 | −17 | 5.76 | 553 | LVLPFC | |
Right inferior frontal gyrus (orbital part) | 45 | 26 | −8 | 5.52 | 256 | RVLPFC | |
Left SMA | −9 | 26 | 55 | 5.39 | 1443 | ||
Left precentral gyrus | −24 | −7 | 46 | 4.56 | 239 | ||
Model H: trial-wise negative behavioral updating | |||||||
Left inferior frontal gyrus (orbital part) | −36 | 20 | −14 | 7.01 | 1081 | LVLPFC, LIFG | |
Left superior frontal gyrus (medial) | −9 | 29 | 55 | 6.33 | 1649 | DMPFC | |
Right insula | 42 | 23 | −8 | 5.96 | 315 | ||
Left calcarine fissure | −12 | −91 | −2 | 5.85 | 92 | ||
Left caudate | −15 | 5 | 13 | 5.70 | 91 | ||
Right superior parietal gyrus | 18 | −64 | 58 | 5.35 | 112 | ||
Right caudate | 15 | 8 | 13 | 4.98 | 96 | ||
Left middle temporal gyrus | −57 | −52 | 7 | 4.41 | 87 |
Region name . | x . | y . | z . | t value . | # voxels . | ToM . | VLPFC/IFG . |
---|---|---|---|---|---|---|---|
Model D: cumulative number of consecutive positive or negative behaviors presented | |||||||
Posterior cingulum | −3 | −49 | 31 | 8.39 | 259 | PC | |
Model A: cumulative number of consecutive positive behaviors presented | |||||||
Left middle temporal gyrus | −57 | −55 | 19 | 5.22 | 219 | LTPJ | |
Left superior frontal gyrus | −3 | 53 | 19 | 5.04 | 312 | ||
Model A: cumulative number of consecutive negative behaviors presented | |||||||
Left posterior cingulum | −3 | −49 | 28 | 9.07 | 387 | ||
Left calcarine fissure | −9 | −97 | −5 | 7.38 | 168 | ||
Left superior frontal gyrus | −6 | 44 | 49 | 6.53 | 951 | ||
Left middle temporal gyrus | −63 | −19 | −14 | 5.64 | 175 | ||
Left angular gyrus | −57 | −70 | 34 | 5.05 | 140 | ||
Model F: valence reversal (from pos → neg or neg → pos) | |||||||
Right superior frontal gyrus (medial) | 6 | 53 | 28 | 6.29 | 219 | DMPFC | |
Precuneus | 9 | −67 | 49 | 5.04 | 102 | ||
Right inferior frontal gyrus (orbital part) | 36 | 23 | −8 | 4.94 | 180 | RVLPFC | |
Model G: magnitude of trial-wise behavioral updating | |||||||
Left superior temporal pole | −42 | 20 | −17 | 5.76 | 553 | LVLPFC | |
Right inferior frontal gyrus (orbital part) | 45 | 26 | −8 | 5.52 | 256 | RVLPFC | |
Left SMA | −9 | 26 | 55 | 5.39 | 1443 | ||
Left precentral gyrus | −24 | −7 | 46 | 4.56 | 239 | ||
Model H: trial-wise negative behavioral updating | |||||||
Left inferior frontal gyrus (orbital part) | −36 | 20 | −14 | 7.01 | 1081 | LVLPFC, LIFG | |
Left superior frontal gyrus (medial) | −9 | 29 | 55 | 6.33 | 1649 | DMPFC | |
Right insula | 42 | 23 | −8 | 5.96 | 315 | ||
Left calcarine fissure | −12 | −91 | −2 | 5.85 | 92 | ||
Left caudate | −15 | 5 | 13 | 5.70 | 91 | ||
Right superior parietal gyrus | 18 | −64 | 58 | 5.35 | 112 | ||
Right caudate | 15 | 8 | 13 | 4.98 | 96 | ||
Left middle temporal gyrus | −57 | −52 | 7 | 4.41 | 87 |
Notes: See Table 2. Coordinates are provided in MNI space. All regions survived cluster-level correction (FWE, P < 0.05)
Region name . | x . | y . | z . | t value . | # voxels . | ToM . | VLPFC/IFG . |
---|---|---|---|---|---|---|---|
Model D: cumulative number of consecutive positive or negative behaviors presented | |||||||
Posterior cingulum | −3 | −49 | 31 | 8.39 | 259 | PC | |
Model A: cumulative number of consecutive positive behaviors presented | |||||||
Left middle temporal gyrus | −57 | −55 | 19 | 5.22 | 219 | LTPJ | |
Left superior frontal gyrus | −3 | 53 | 19 | 5.04 | 312 | ||
Model A: cumulative number of consecutive negative behaviors presented | |||||||
Left posterior cingulum | −3 | −49 | 28 | 9.07 | 387 | ||
Left calcarine fissure | −9 | −97 | −5 | 7.38 | 168 | ||
Left superior frontal gyrus | −6 | 44 | 49 | 6.53 | 951 | ||
Left middle temporal gyrus | −63 | −19 | −14 | 5.64 | 175 | ||
Left angular gyrus | −57 | −70 | 34 | 5.05 | 140 | ||
Model F: valence reversal (from pos → neg or neg → pos) | |||||||
Right superior frontal gyrus (medial) | 6 | 53 | 28 | 6.29 | 219 | DMPFC | |
Precuneus | 9 | −67 | 49 | 5.04 | 102 | ||
Right inferior frontal gyrus (orbital part) | 36 | 23 | −8 | 4.94 | 180 | RVLPFC | |
Model G: magnitude of trial-wise behavioral updating | |||||||
Left superior temporal pole | −42 | 20 | −17 | 5.76 | 553 | LVLPFC | |
Right inferior frontal gyrus (orbital part) | 45 | 26 | −8 | 5.52 | 256 | RVLPFC | |
Left SMA | −9 | 26 | 55 | 5.39 | 1443 | ||
Left precentral gyrus | −24 | −7 | 46 | 4.56 | 239 | ||
Model H: trial-wise negative behavioral updating | |||||||
Left inferior frontal gyrus (orbital part) | −36 | 20 | −14 | 7.01 | 1081 | LVLPFC, LIFG | |
Left superior frontal gyrus (medial) | −9 | 29 | 55 | 6.33 | 1649 | DMPFC | |
Right insula | 42 | 23 | −8 | 5.96 | 315 | ||
Left calcarine fissure | −12 | −91 | −2 | 5.85 | 92 | ||
Left caudate | −15 | 5 | 13 | 5.70 | 91 | ||
Right superior parietal gyrus | 18 | −64 | 58 | 5.35 | 112 | ||
Right caudate | 15 | 8 | 13 | 4.98 | 96 | ||
Left middle temporal gyrus | −57 | −52 | 7 | 4.41 | 87 |
Region name . | x . | y . | z . | t value . | # voxels . | ToM . | VLPFC/IFG . |
---|---|---|---|---|---|---|---|
Model D: cumulative number of consecutive positive or negative behaviors presented | |||||||
Posterior cingulum | −3 | −49 | 31 | 8.39 | 259 | PC | |
Model A: cumulative number of consecutive positive behaviors presented | |||||||
Left middle temporal gyrus | −57 | −55 | 19 | 5.22 | 219 | LTPJ | |
Left superior frontal gyrus | −3 | 53 | 19 | 5.04 | 312 | ||
Model A: cumulative number of consecutive negative behaviors presented | |||||||
Left posterior cingulum | −3 | −49 | 28 | 9.07 | 387 | ||
Left calcarine fissure | −9 | −97 | −5 | 7.38 | 168 | ||
Left superior frontal gyrus | −6 | 44 | 49 | 6.53 | 951 | ||
Left middle temporal gyrus | −63 | −19 | −14 | 5.64 | 175 | ||
Left angular gyrus | −57 | −70 | 34 | 5.05 | 140 | ||
Model F: valence reversal (from pos → neg or neg → pos) | |||||||
Right superior frontal gyrus (medial) | 6 | 53 | 28 | 6.29 | 219 | DMPFC | |
Precuneus | 9 | −67 | 49 | 5.04 | 102 | ||
Right inferior frontal gyrus (orbital part) | 36 | 23 | −8 | 4.94 | 180 | RVLPFC | |
Model G: magnitude of trial-wise behavioral updating | |||||||
Left superior temporal pole | −42 | 20 | −17 | 5.76 | 553 | LVLPFC | |
Right inferior frontal gyrus (orbital part) | 45 | 26 | −8 | 5.52 | 256 | RVLPFC | |
Left SMA | −9 | 26 | 55 | 5.39 | 1443 | ||
Left precentral gyrus | −24 | −7 | 46 | 4.56 | 239 | ||
Model H: trial-wise negative behavioral updating | |||||||
Left inferior frontal gyrus (orbital part) | −36 | 20 | −14 | 7.01 | 1081 | LVLPFC, LIFG | |
Left superior frontal gyrus (medial) | −9 | 29 | 55 | 6.33 | 1649 | DMPFC | |
Right insula | 42 | 23 | −8 | 5.96 | 315 | ||
Left calcarine fissure | −12 | −91 | −2 | 5.85 | 92 | ||
Left caudate | −15 | 5 | 13 | 5.70 | 91 | ||
Right superior parietal gyrus | 18 | −64 | 58 | 5.35 | 112 | ||
Right caudate | 15 | 8 | 13 | 4.98 | 96 | ||
Left middle temporal gyrus | −57 | −52 | 7 | 4.41 | 87 |
Notes: See Table 2. Coordinates are provided in MNI space. All regions survived cluster-level correction (FWE, P < 0.05)
Brain-Behavior Analyses in Lateral Prefrontal ROIs
The above whole-brain parametric analyses revealed that left IFG and VLPFC track the magnitude of impression updating. To test the robustness of these findings, we conducted exploratory ROI analyses in these regions to examine correlations between changes in PSC and changes in trustworthiness ratings. ROIs were drawn as 9 mm-radius spheres centered on peak coordinates from prior work showing that left IFG and left VLPFC respond preferentially to meaningful changes in behavior (IFG: [−58, 22, 18]; VLPFC: [−48, 27, −12]; Mende-Siedlecki and Todorov 2016).
In left IFG, there was a significant relationship between neural updating and behavioral impression updating (ß = 0.048, SE = 0.021, χ2(1) = 5.019, P = 0.025), such that a greater change in PSC was associated with a greater change in trustworthiness ratings. We found no evidence for such a relationship in left VLPFC (ß = −0.004, SE = 0.021, χ2 (1) = 0.026, P = 0.871).
Conceptual Replication of Behavioral Task
We had hypothesized an effect of the strength of the prior on the magnitude of impression updating, but found no such effect in our sample of scanned participants. We thus tested this effect in a preregistered conceptual replication on Amazon Mechanical Turk (N = 400). Across participants, all 40 expectation-violation targets from the fMRI study were presented; the behavior sequences were the same exact sequences seen in the scanner. Due to time constraints of online data collection, each participant learned about 8 targets: 2 of each sequence type (strong neg → pos, weak neg → pos, strong pos → neg, weak pos → neg). Target order was randomized for each participant. We wanted to ensure that any differences we found either related to prior strength or update direction could not be attributed to specific pairings of preswitch and postswitch behaviors, or to target identity. Therefore, we shuffled the postswitch behaviors across participants so that they were seen following both weak and strong priors. Additionally, we counterbalanced target name and associated target face with update direction across participants.
On each behavior presentation, participants gave 2 types of ratings: trustworthiness of the target on a scale from 1 (least trustworthy) to 7 (most trustworthy), and attribution of the behavior from 1 (solely due to the target’s disposition) to 100 (solely due to the surrounding situation). The order in which the rating scales were presented was counterbalanced across subjects; the attribution data are not reported here. To examine behavioral impression updating, we computed an updating measure for each trial (see Materials and Methods). We conducted linear mixed effects analyses to test whether impression updating differed as a function of prior strength (weak or strong) and update direction (pos → neg or neg → pos). Random intercepts for subject and item were included in the model.
We found greater impression updating following the violation of weak versus strong priors (B = 0.090, SE = 0.034, χ2(1) = 7.182, P = 0.007, Rß2 = 0.003), suggesting that the prior strength manipulation in our paradigm can have an effect on the degree of belief updating. In this larger dataset, we also found more updating in the positive-to-negative direction versus the negative-to-positive direction, consistent with the in-scanner dataset (pos → neg > neg → pos, B = 0.700, SE = 0.034, χ2(1) = 400.45, P < 0.001, Rß2 = 0.133).
Discussion
In certain social contexts, observers have strong prior impressions that can co-occur with social motivations to maintain those impressions. In the current work, we aimed to isolate the impact of experimentally induced prior beliefs on impression updating and related neural activity. Participants learned about novel fictional individuals whose behaviors were either internally inconsistent over time or internally consistent. The inconsistent individuals performed 2 or 4 same-valence behaviors, followed by 2 behaviors of the opposite valence. ROI analyses of the ToM network revealed a greater change in activity in DMPFC and RTPJ following the violation of strong versus weak priors, and a greater change in activity in DMPFC and LTPJ following the violation of positive versus negative priors. These findings show that the ToM network is sensitive to 1) violations of strong versus weak prior beliefs and 2) the direction of impression change. Additional analyses showed that DMPFC and LTPJ respond to meaningful changes in behavior, not just to negative valence.
Contributions of Prior Strength and Motivation
The present study manipulated participants’ priors by providing different amounts of initially positive or negative information about targets. We showed, in a context absent real-life priors and social motivations, that ToM activity is enhanced following the violation of strong versus weak prior beliefs. This suggests that differences in neural responses to close versus distant others, and ingroup versus outgroup members, may be driven in part by differences in the strength of prior beliefs.
We expected that participants’ social motivations would be at floor for all targets in our study, as they were zero-acquaintance, fictional targets. We thus interpret differences in ToM activity following the violation of strong versus weak prior beliefs as arising from our experimental manipulation, rather than differences in social motivation. However, in real-life situations, we expect belief strength and social motivation to often co-occur and operate in parallel: people not only know more about close others and ingroup members, but also are motivated to maintain relationships with close others (Park and Young 2020) and maintain positive impressions of ingroup members (Van Bavel and Pereira 2018). The relative degrees to which prior beliefs and motivation contribute to real-life belief updating and neural activity likely depend on social goals (e.g., to affiliate with others versus to predict others’ behavior; Waytz and Young 2014), context (e.g., dyads vs. groups), and individual differences (e.g., in mentalizing ability, cognitive reflection). An important future direction is to directly compare the impact of belief strength with the impact of motivation on updating and neural activity, across a variety of paradigms. Future work can, for example, take advantage of cases where participants’ prior beliefs and motivations diverge. Participants could be presented information that is consistent or inconsistent with either their beliefs or their desires (Tappin et al. 2017), enabling a comparison of neural responses for prior-inconsistent information with neural responses for motivation-inconsistent information.
Prior Strength and Updating in the Current Study
Prior work has found that observers typically engage in less impression updating for close and ingroup others—targets for whom they have strong (positive) priors (Hughes, Ambady, et al. 2017a; Hughes, Zaki, et al. 2017b; Park et al. 2020). In the current study, we did not observe an effect of the prior strength manipulation on the magnitude of impression updating. However, behavioral evidence from preswitch and postswitch ratings suggest a difference between the strong and weak prior manipulations: 1) preswitch ratings based on 4 positive behaviors were more positive than preswitch ratings based on just 2 positive behaviors; 2) preswitch ratings based on 4 negative behaviors were more negative than preswitch ratings based on just 2 negative behaviors; and 3) postswitch ratings following 4 negative behaviors were more negative than postswitch ratings following 2 negative behaviors. Prior strength thus had an effect on initial impressions, and, to some extent, updated impressions, in a context absent social motivation.
Why were differences in neural activation following the violation of strong versus weak priors not accompanied by differences in the magnitude of impression updating? One possibility is that enhanced ToM activity following the violation of strong priors supported belief maintenance on some trials, and belief updating on others. Both exculpatory and condemnatory explanations of behavior involve a mental state inference: for instance, upon learning that a target took money from a tip jar, one could infer that she intended to make change for a dollar, or that she intended to steal it. Thus, the enhanced mentalizing in light of strong priors could have resulted in a prior-consistent explanation in some cases, and a prior-inconsistent explanation in others. We might expect to find a stronger relationship between mentalizing activity and belief updating when real-life priors for individuals or groups are involved: these priors may be strong enough (and/or there may be enough motivation involved) that mentalizing activity chiefly supports belief maintenance in these contexts.
In addition, we may have had insufficient power in the current study to detect a behavioral effect of prior strength on update magnitude. We conducted a conceptual replication of the behavioral task on Amazon Mechanical Turk, where we presented participants with the same stimuli presented in the scanner (N = 400). We found greater impression updating following the violation of weak versus strong priors, suggesting that the prior strength manipulation in our paradigm can have an effect on the degree of belief updating. We discuss below, under Future directions, how the strength manipulation may be made more robust.
Distinct Roles for ToM Regions in Tracking Qualities of Social Information
Our ROI analyses revealed that DMPFC and RTPJ are sensitive to violations of strong versus weak prior beliefs, while DMPFC and LTPJ track the direction of impression change. In addition, surprisingness ratings indicated that, while participants were more surprised when strong priors were violated than when weak priors were violated, there was no effect of update direction on the surprisingness of inconsistent behaviors. That is, surprising negative behaviors (which led to greater updating) were not rated as more surprising than surprising positive behaviors. These results together suggest that there may be distinct roles for different ToM regions in tracking separate qualities of new social information: its surprisingness and its valence. Furthermore, the encoding model analyses revealed that PC tracks the strength of the prior, regardless of valence, while LTPJ tracks the strength of positive priors specifically; in addition, DMPFC tracked whether the current behavior was opposite in valence to the previous behavior. These findings suggest that different ToM regions track distinct features of new behavioral information that are dependent on the nature of previous behavioral information.
Diagnosticity of Immoral Behaviors
Greater belief updating and ToM activity following the receipt of new negative information versus positive information is consistent with a diagnosticity account of impression updating (Mende-Siedlecki et al. 2013). This account posits that immoral behaviors and highly competent behaviors elicit greater impression updates than their counterparts because they are perceived to be less frequent, and thus more informative about a person’s true character. While the moral and immoral behavior stimuli in our experiment were matched on perceived frequency, we still observed both greater impression updates in the positive-to-negative direction, and greater ToM activity when positive priors were violated by negative information. In addition, as described above, postscan surprisingness ratings indicated that there was no effect of update direction on the surprisingness of inconsistent behaviors. This raises the possibility that, at least in the context of the current experiment, factors other than perceived frequency and surprisingness contributed to the dominance of immoral behaviors for impression updating.
Why do immoral behaviors shift impressions to such a great extent? Behavioral work by Brambilla et al. (2019) has shown that morally relevant behaviors in general dominate impression updating (compared with behaviors related to sociality or competence) because they are seen as containing more information about interpersonal intentions. One possibility is that immoral behaviors contain more intent information than moral behaviors. And, in line with our findings, their mediational analyses do not support a frequency-based account of the dominance of (im)moral behaviors for updating. Relatedly, reinterpretation has been shown to play a pivotal role in reversing initial (implicit) impressions (Mann and Ferguson 2017); thus, another possibility is that immoral behaviors are more powerful because they are likelier to lead to a reinterpretation of past behaviors. That is, it is easier to generate reputation-based explanations for someone’s past moral behavior (e.g., “she did that only because it would make her look good”), rather than to conceive of prosocial explanations for past immoral behavior (Reeder and Brewer 1979). Both of the above hypotheses can also potentially account for the enhanced mentalizing activity observed when new negative information contradicts positive priors.
Relationship Between ToM Activity and Belief Maintenance
While the ToM network typically responds preferentially to unpredicted events, and, as we have shown, is sensitive to the violation of strong versus weak prior beliefs, the relationship between ToM activity and belief updating is more complex. In some contexts, greater ToM activity facilitates belief maintenance. For instance, one study found greater activity in DMPFC and bilateral TPJ when third-party observers viewed ingroup versus outgroup defectors in the Prisoner’s Dilemma Game (Baumgartner et al. 2012). Increased connectivity between DMPFC and LTPJ was associated with weaker punishment of ingroup defectors, and disrupting RTPJ activity through transcranial magnetic stimulation reduced relative forgiveness of ingroup defectors (Baumgartner et al. 2014). Thus, ToM activity may have supported the generation of exculpatory explanations for ingroup defectors. In this context, ingroup defection may be seen as inconsistent with strong positive priors about the ingroup, while outgroup defection may be seen as consistent with strong negative priors about the outgroup. Therefore, greater ToM activity following the more surprising event (ingroup defection) versus the less surprising event (outgroup defection) dovetails with what we find in the current study: greater ToM activity following the more surprising event (violation of strong priors) versus the less surprising event (violation of weak priors). In the intergroup study, greater ToM activity supported belief maintenance; in our zero-acquaintance study, greater ToM activity was not mirrored by belief maintenance (at least in a sample of 28 participants). One possibility is that social motivation to maintain positive beliefs about the ingroup (absent from the present experimental paradigm) may have played a role in the intergroup context.
In other contexts, activity in the ToM network is selectively reduced when maintaining beliefs about close or ingroup others. One study found that observers failed to downgrade their impressions of ingroup members, but not outgroup members, following negative information; furthermore, overcoming this ingroup bias (to effectively downgrade impressions) was associated with increased activity in TPJ, PC, LPFC, and DACC (Hughes, Ambady, et al. 2017a; Hughes, Zaki, et al. 2017b). Similarly, a recent fMRI study examined impression updating for friends and strangers who gave money to, or took money from, the participant in an economic game (Park et al. 2020). Reduced RTPJ activity was observed in response to friends’ taking money, compared with strangers’ taking money; and this neural pattern was reflected in reduced behavioral updating for friends compared with strangers. However, within the friend-taking condition, greater RTPJ activity was associated with greater negative updating, indicating greater mentalizing effort required for overcoming strong positive priors about friends. Thus, in both of these studies, disengagement of ToM regions such as RTPJ was associated with impression maintenance for ingroup members and friends, and on the flip side, recruitment of ToM regions supported belief updating, particularly negative updating. Overall, these patterns suggest that, in some intergroup contexts and social relational (friend-stranger) contexts, the passive response to prior-inconsistent information about ingroup or close others may be to disengage from mentalizing, perhaps to discount unfavorable information. These findings stand in contrast to what we find in a zero-acquaintance context: greater mentalizing in response to information that violates strong (vs. weak) priors and positive (vs. negative) priors.
ToM activity has been found to facilitate both belief maintenance and belief updating. Our interpretation of these mixed past results, together with the findings of the current study, is that 2 different mechanisms can result in the maintenance of strong prior beliefs (Kim et al. 2020). In one case, the violation of strong priors is followed by enhanced ToM activity, which may reflect the generation of a coherent mentalistic explanation of the unpredicted information (e.g., my ingroup member/close friend did not intend to defect/make an unfair offer). The generation of alternative explanations following the violation of strong priors is compatible with a form of Bayesian rationality, where the likelihood of generating an alternative explanation depends probabilistically on the strength of the prior belief, and the likelihood of the conflicting information (Gershman 2019). Alternatively, prior-inconsistent information may be followed by reduced ToM activity, due to disengagement from mentalizing about the target, which eliminates the need to reconcile the new information with prior beliefs. Overcoming this form of passive discounting may involve the intervention of cognitive control regions, such as DACC and LPFC (Hughes and Zaki 2015; Hughes, Ambady, et al. 2017a). As we have proposed, activity in ToM and control regions, then, when coupled with behavioral evidence of belief maintenance, may help distinguish between the mentalizing route to belief maintenance, which is compatible with Bayesian rationality, and the discounting route to belief maintenance, which does not account for the unexpected information.
Predictive Coding in the ToM Network
Greater ToM activity following the violation of strong versus weak prior beliefs is consistent with a predictive coding view of the social brain (see Koster-Hale and Saxe 2013; Theriault, Young, et al. 2020b), which holds that some neural responses indicate the distance between predictions from a generative model of the world and incoming sensory information (prediction error, PE). Prior work has shown that the ToM network responds more to unpredicted versus predicted information across a wide variety of social stimuli and task contexts, including past behavioral history (Mende-Siedlecki et al. 2013; Dungan et al. 2016), instructed trait knowledge (Heil et al. 2019), and stereotypes (Cloutier et al. 2011; Li et al. 2016). The current findings demonstrate that the ToM network is sensitive to different degrees of unpredictedness during impression updating: activity in this network was enhanced for information that violated strong prior beliefs versus information that violated weak prior beliefs. These results are also in line with computational neuroimaging work showing that PEs generated during associative learning of social value correlate with activity in ToM regions (Behrens et al. 2008; Hackel et al. 2015).
A Broader Network for Impression Updating
Our whole-brain analyses revealed 2 additional regions beyond the ToM network that were consistently activated during impression updating: ventrolateral prefrontal cortex (VLPFC) and IFG. In particular, VLPFC and IFG showed greater changes in activity for positive-to-negative sequences than negative-to-positive sequences. This pattern is consistent with previous work (Mende-Siedlecki and Todorov 2016) showing that these regions respond preferentially to moral-to-immoral changes in moral behavior, relative to immoral-to-moral changes in behavior, and relative to nonmeaningful changes in behavior (e.g., “Jenny went for a bike ride”; “Jenny went for a run”; “Jenny played video games”). This past study interpreted left VLPFC activity as reflecting the retrieval of stored conceptual representations, and left IFG activity as reflecting the process of resolving interference between representations (Badre et al. 2005; Badre and Wagner 2007; Satpute et al. 2014). In the current study as well, we suggest that activity in these regions is an instantiation of these more general cognitive processes, recruited to a greater degree for information that prompts an update to stored representations. In addition, our encoding model analyses revealed that bilateral VLPFC parametrically tracked the magnitude of trial-wise impression updating, and left VLPFC and left IFG parametrically tracked the magnitude of trial-wise negative impression updating. PSC analyses also revealed that greater changes in neural activity in left IFG are associated with a greater change in impression ratings from preswitch behaviors to postswitch behaviors. Overall these results indicate that VLPFC and left IFG track changes in actual rated impressions, especially in the negative direction, during the processing of diagnostic information.
Future Directions
First, in the current work, we probed the effect of different experimentally induced priors on updating and ToM activity. It may be fruitful to manipulate whether or not updating occurs through the use of participant instructions (Trafimow and Porter 1997). That is, participants can be instructed, across blocks, to either 1) use expectation-violating information to update their prior impressions, or 2) use their prior impressions to reinterpret the expectation-violating information. This approach would allow for a direct comparison of neural activity, both in terms of magnitude of activation and patterns of activation, for belief updating versus belief maintenance. Recent work has shown that social information is neurally represented along a small set of representational dimensions, which in turn can facilitate the prediction of others’ future mental states and actions (Tamir and Thornton 2018); analyzing patterns of brain activity elicited by our paradigm will also allow us to examine how neural representations of targets change in light of expectation violations.
Second, in the current work, we manipulated the number of same-valenced behaviors presented (2 vs. 4) before a counter-valenced behavior was presented, to induce stronger versus weaker beliefs about the target. A limitation of this paradigm is that, in a sample of 28 participants, this manipulation was not strong enough to induce differences in update magnitude. One possibility is that there needs to be a greater difference in the number of initial behaviors (e.g., 2 vs. 6) to observe an effect on the magnitude of impression updating. An alternative way to manipulate the strength of the prior is to vary the extremity of behavioral information, rather than the amount of information. For example, a target who performed 2 extremely negative behaviors could be compared with a target who performed 2 mildly negative behaviors. Future work may benefit from exaggerating the diagnostic difference between strong and weak priors in this way. Yet another important future direction would be to directly compare these 2 implementations within the same paradigm: strong beliefs stemming from more evidence, versus strong beliefs stemming from a more extreme piece of evidence.
Third, as we tested the impact of the strength of priors in the context of zero-acquaintance targets, the current study cannot speak to the relative importance of belief strength and motivation for real-life belief updating and neural activity. Future work should either pit belief strength and motivation against each other, or take advantage of cases in which they diverge in participants, and then provide information that is consistent or inconsistent with beliefs or desires (Tappin et al. 2017). This unique approach would allow for the comparison of the effects of belief strength and motivation on behavioral and neural indices of updating.
Finally, another interesting area for further research is the dominance of immoral information in impression updating. Future work may explore the hypothesis that immoral behaviors are more important for updating than moral behaviors because they contain more intent information. Another possibility is that immoral behaviors are likelier to lead to a reinterpretation of past moral behaviors than vice versa. Furthermore, the boundaries of this valence effect are of interest—for example, recent work has found that, in a context where character ratings are made relative to a single type of moral behavior that evolves over time, beliefs about initially bad agents are more volatile, and thus more amenable to updating (Siegel et al. 2018).
Summary
We manipulated participants’ initial beliefs about fictional targets by varying the amount of positive and negative information about targets’ past behaviors. In this zero-acquaintance context, we found that activity in DMPFC and RTPJ is enhanced for information that violates strong versus weak prior beliefs, and activity in DMPFC and LTPJ is enhanced for information that violates positive versus negative prior beliefs. Thus, absent social motivation, differences in belief strength and belief valence can lead to differences in ToM in response to new information. These results can be compared with past work directly manipulating motivation: some studies have shown enhanced ToM for surprising information about close others and ingroup members, while others have shown decreased ToM. We suggest that, in real-life contexts, increased mentalizing activity in light of strong positive priors may reflect the generation of alternative explanations, whereas decreased mentalizing activity may reflect motivated discounting of unfavorable information.
Notes
The authors would like to thank: Emma Alai, Arturo Balaguer, Catherine Kim, Mariel Kronitz, and Mookie Manalili for their assistance with data collection; Dima Ayyash, Steve Shannon, and Atsushi Takahashi for their technical support; Josh Hirschfeld-Kroen, Kevin Jiang, Gordon Kraft-Todd, Mookie Manalili, Justin Martin, Ryan McManus, BoKyung Park, the Moral Psychology Research Lab, and the Greene Lab for their feedback. Conflicts of Interest: None declared.
Funding
John Templeton Foundation (61061 to L.Y.); the National Science Foundation (1627157 to L.Y.); the Boston College Virtue Project (to L.Y.); and a shared instrumentation grant from the National Institutes of Health (S10-OD021569 to MIT).