-
PDF
- Split View
-
Views
-
Cite
Cite
Hongjun Chen, Yekai Zhou, Yongjing Liu, Peijing Zhang, Ming Chen, Network integration and protein structural binding analysis of neurodegeneration-related interactome, Briefings in Bioinformatics, Volume 24, Issue 4, July 2023, bbad237, https://doi.org/10.1093/bib/bbad237
- Share Icon Share
Abstract
Neurodegenerative diseases (NDs) usually connect with aggregation and molecular interactions of pathological proteins. The integration of accumulative data from clinical and biomedical research will allow for the excavation of pathological proteins and related interactors. It is also important to systematically study their interacting proteins in order to find more related proteins and potential therapeutic targets. Understanding binding regions in protein interactions will help functional proteomics and provide an alternative method for predicting novel interactions. This study integrated data from biomedical research to achieve systematic mining and analysis of pathogenic proteins and their interaction network.
A workflow has been built as a solution for the collective information of proteins involved in NDs, related protein–protein interactions (PPIs) and interactive visualizations. It also included protein isoforms and mapped them in a disease-related PPI network to illuminate the impact of alternative splicing on protein binding. The interacting proteins enriched by diseases and biological processes (BPs) revealed possible regulatory modules. A high-resolution network with structural affinity information was generated. Finally, Neurodegenerative Disease Atlas (NDAtlas) was constructed with an interactive and intuitive view of protein docking with 3D molecular graphics beyond the traditional 2D network. NDAtlas is available at http://bis.zju.edu.cn/ndatlas.
INTRODUCTION
Neurodegenerative diseases (NDs), like Alzheimer’s disease (AD), Parkinson’s disease (PD), Huntington’s disease (HD) and Amyotrophic lateral sclerosis (ALS), occur as a result of neuron dysfunction, structural damage and cell death. Various molecular mechanisms are involved in the pathogenesis of these diseases. The biggest risk factor in neurodegeneration is aging, which is caused by mitochondrial DNA mutation and oxidative stress [1]. A common cause in several NDs is the aggregation of misfolded proteins [2]. Autophagy plays a compromised role in multiple stages of pathways in many NDs [3]. These research and reviews extended our knowledge about the process of neurodegeneration and identified many ND-associated proteins.
Through advancements in medicine and proteomics study, the repertoire of proteins and the human interactome has been expanding rapidly. Table 1 includes details of updated protein–protein interactions (PPIs) databases of human proteome or multiple species, such as BioGRID [4], DIP [5], HPRD [6], IID [7], InBio [8], InnateDB [9], IntAct [10] and STRING [11]. PPI network analysis is an important systems biology method to discover the shared mechanisms between diseases [12], which is applicable in the various kinds of neurodegeneration. Alternative splicing expands the proteomics and regulatory complexity by generating numerous protein variants, which impact the PPI binding in both direct and indirect ways [13]. Thus, the information on isoforms will be a great resource for knowing the mechanism of alternative splicing affecting pathogenesis. Knowledge of binding regions in PPIs will contribute to functional proteomics and give an alternative way to predict PPIs. The structural affinity of protein molecules enables us to describe PPIs in atomic details and infer the related functions.
PPI databases collected by this research, on human or multi-species with experimental data
Name . | URL . | Updated . | Version . |
---|---|---|---|
BioGRID | https://thebiogrid.org/ | August 2022 | 4.4.212 |
DIP | http://dip.doe-mbi.ucla.edu/dip/Main.cgi | 02 May 2017 | 10.1.7.3 |
HPRD | http://www.hprd.org/ | 13 April 2010 | 9 |
IID | http://ophid.utoronto.ca/iid/ | May 2021 | |
InBio | https://inbio-discover.com/ | 12 September 2016 | |
InnateDB | http://www.innatedb.com/ | August 2022 | 5.4 |
IntAct | https://www.ebi.ac.uk/intact/ | December 2021 | 1.0.3 |
STRING | https://string-db.org/ | 12 August 2021 | 11.5 |
Name . | URL . | Updated . | Version . |
---|---|---|---|
BioGRID | https://thebiogrid.org/ | August 2022 | 4.4.212 |
DIP | http://dip.doe-mbi.ucla.edu/dip/Main.cgi | 02 May 2017 | 10.1.7.3 |
HPRD | http://www.hprd.org/ | 13 April 2010 | 9 |
IID | http://ophid.utoronto.ca/iid/ | May 2021 | |
InBio | https://inbio-discover.com/ | 12 September 2016 | |
InnateDB | http://www.innatedb.com/ | August 2022 | 5.4 |
IntAct | https://www.ebi.ac.uk/intact/ | December 2021 | 1.0.3 |
STRING | https://string-db.org/ | 12 August 2021 | 11.5 |
PPI databases collected by this research, on human or multi-species with experimental data
Name . | URL . | Updated . | Version . |
---|---|---|---|
BioGRID | https://thebiogrid.org/ | August 2022 | 4.4.212 |
DIP | http://dip.doe-mbi.ucla.edu/dip/Main.cgi | 02 May 2017 | 10.1.7.3 |
HPRD | http://www.hprd.org/ | 13 April 2010 | 9 |
IID | http://ophid.utoronto.ca/iid/ | May 2021 | |
InBio | https://inbio-discover.com/ | 12 September 2016 | |
InnateDB | http://www.innatedb.com/ | August 2022 | 5.4 |
IntAct | https://www.ebi.ac.uk/intact/ | December 2021 | 1.0.3 |
STRING | https://string-db.org/ | 12 August 2021 | 11.5 |
Name . | URL . | Updated . | Version . |
---|---|---|---|
BioGRID | https://thebiogrid.org/ | August 2022 | 4.4.212 |
DIP | http://dip.doe-mbi.ucla.edu/dip/Main.cgi | 02 May 2017 | 10.1.7.3 |
HPRD | http://www.hprd.org/ | 13 April 2010 | 9 |
IID | http://ophid.utoronto.ca/iid/ | May 2021 | |
InBio | https://inbio-discover.com/ | 12 September 2016 | |
InnateDB | http://www.innatedb.com/ | August 2022 | 5.4 |
IntAct | https://www.ebi.ac.uk/intact/ | December 2021 | 1.0.3 |
STRING | https://string-db.org/ | 12 August 2021 | 11.5 |
To further investigate how PPIs influence neurodegeneration pathogenesis, the associations between PPIs and the involved factors require collected and deep excavation. There have been published integrative databases on individual or multiple NDs, which mainly focus on AD, PD and HD. AlzBase is an integrative database for gene dysregulation in AD, which identified critical genes connecting with endophenotypes [14]. NeuroMuscleDB focuses on genes related to neuromuscular diseases and neurodegeneration, covering multiple types of mammals [15]. HDNetDB is a database, which integrates molecular interactions in HD [16].
However, the PPI networks collected from these databases are direct binary, single-layer, separated and partially outdated. These PPI networks only contain monolayer node-to-node connections of proteins, which only describe topological features, but lack omics data such as proteomics, structural affinity data and isoform data. Therefore, an integrated network with multi-level data is constructed, which can realize cross-referencing of multiple data sources, and combine more protein-related sequence and structural information. It will help to discover disease-causing proteins and understand the mechanisms of cross-disease protein-interaction modules more precisely.
The protein isoform information can be accessed from public databases, like UniProt [17] and IIIDB [18], in which alternative sequences of protein variants, expression levels and isoform–isoform interactions are collected. Currently, most PPI networks are comprised binary interactions that lack atomic details and binding site information. It would be promising to consider the calculation of protein docking, so that a high-resolution network could be constructed. The latest version of ZDOCK is capable of scoring protein docking parameters, like shape complementarity, electrostatics and the potential, for paired atomic statistics [19].
Our study aims to construct an integrative PPI network beyond the direct binary PPIs, which include more information such as isoforms and structural affinity. A workflow was developed to extend neurodegeneration-related PPI network and to fulfill 3D visualization of critical PPIs’ structural binding. A high-resolution PPI network including multi-level molecular details was generated.
Therefore, a database focusing on ND-related PPIs has been constructed. Neurodegenerative Disease Atlas (NDAtlas, http://bis.zju.edu.cn/ndatlas) provided query search of proteins, detailed information on ND-related PPIs, visualization of PPI networks and 3D structure binding states of specific interactions. The dataset included 431 reviewed proteins involved in 27 NDs as core nodes in the PPI network. In the visualization of a particular subnetwork, the overlaps between different NDs could be illuminated. In our constructed network, cross-disease protein-interaction module was discovered, and new potential disease-related isoforms were identified as candidates for experimental research.
RESULTS
The workflow of multi-level disease PPI network integration
To systematically mine the dataset and conduct analysis, we constructed a workflow (Figure 1) involving the construction of protein interaction networks and related databases in human NDs.
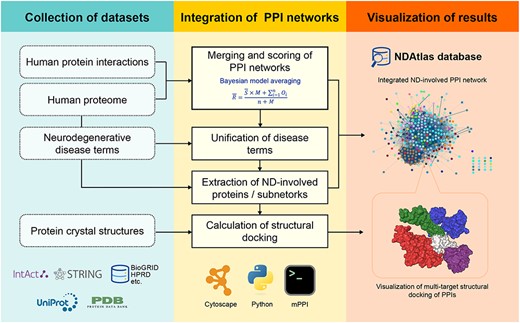
The workflow of integration of PPI networks and isoforms and structural docking data.
Collection of datasets, integration of PPI networks and data visualization are the three main steps in our workflow. Step 1 collected human proteome annotations, the whole human interactome, neurodegeneration-related disease terms and protein structures, and pre-processed them for integration. The datasets included reviewed proteins and experimental links from public databases. During Step 2, in-house scripts were implemented to merge all the links of proteins and to remove duplicates from different databases. The ranking scores and confidence scores were calculated and assigned to the PPIs, respectively. The distribution of two scores of the NDAtlas was shown in Supplementary Figure S1. Annotations associated with disease-causing conditions were mapped to the PPI network. Possible docking structures were modeled for known disease-causing proteins and their partners. Step 3 constructed a database for searching and visualization of the ND-related subnetworks and multi-protein docking structures.
In addition to creating the NDAtlas database, the workflow of integration produced disease-specified subnetworks and scoring matrix, which enables the analysis of disease-protein modules and disease-interaction patterns in atomic details.
An integrated database of PPI related to neurodegeneration
The NDAtlas is an integrated database that allows searching and visualizing ND-related proteins and their interactions in multiple ways. Figure 2A shows the Home Page components. The Advanced Search Page and the Download Page can be found from the navigation bar. The Home Page includes a quick search bar to support individual protein searches with an auto-completion function. The subnetworks of more common diseases are listed as clickable thumbnails, including AD, PD, HD and ALS. The statistical results of proteins and interactions were described in Figure 2B.
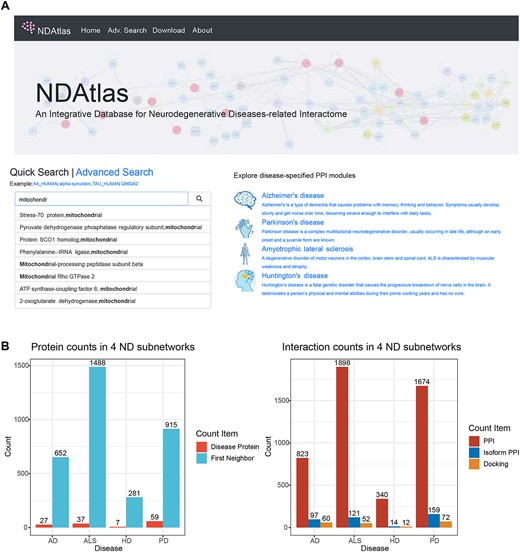
NDAtlas database home page and statistics of datasets. (A) The home page of NDAtlas. It includes Quick Search function and Disease subnetwork exploration. (B) The counts of proteins (left) and protein interactions (right) in the main NDs. AD: Alzheimer diseases; ALS: Amyotrophic lateral sclerosis; HD: Huntington’s disease; PD: Parkinson’s disease.
After searching or clicking on the name of a protein, the Protein Detail Page will appear, such as LRRK2 (leucine-rich repeat kinase 2) related to PD [20]. The relevant annotations of the protein will be displayed (Figure 3A), including multiple fields such as protein label, name, UniProt ID, associated disease and subcellular location. Figure 3B provides a list of partners that interact with this protein in the PPI network, followed by the PPI visualization in the same page. The labels of the interacting proteins, protein isoforms involved in the interacting form, the experimental method used to identify the interaction and the scores of each PPI are displayed in the list of interacting proteins of LRRK2.
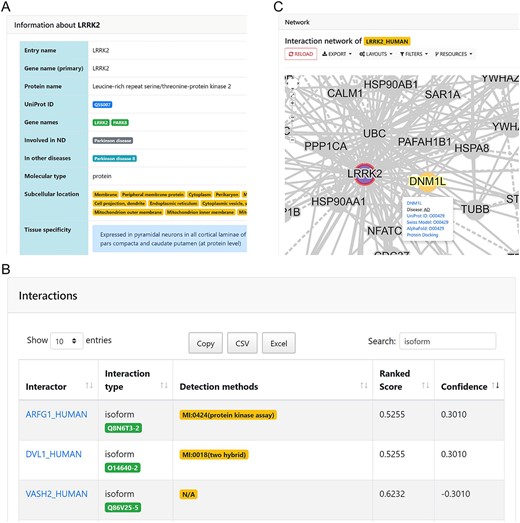
Detail page and PPI network visualization of LRRK2. (A) The detail table of LRRK2, including the basic annotation of LRRK. (B) Interaction details and scores of LRRK2. (C) The PPI network visualization of LRRK2. Pop-up shows the annotation of the selected node DNM1L.
An interactive view of the queried PPI will be provided in the network visualization area (Figure 3C), including the necessary annotations and external links to UniProt and SWISS-MODEL [21]. When a protein is involved with multiple diseases, the popup of the node will show the disease labels connected by ‘-’. The docking status of multiple interactors can be presented when the network visualization is combined with a 3D structural visualization extension, the NGL viewer [22]. Furthermore, the database provides downloads for all the protein annotations and PPIs. The Helping Manual can be viewed on the About Page.
The ND-related PPI subnetwork
Cross-referencing multiple disease-related databases yielded 69 neurodegenerative disease categories. From the UniProt (release 2022.8) proteome datasets, 20 793 human proteins were collected. Integration of multiple protein interaction databases resulted in the discovery of 449 158 non-redundant human protein interactions as well as protein–isoform interactions. BioGRID, InbioMap, IntAct, MINT and STRING were used to score the interactions. BioGRID and STRING have different metrics to measure the interactions. The scores from InbioMap, Intact and MINT range from 0 to 1. These scores demonstrate the possibility of interaction. Bayesian model averaging (BMA) [23] was used to adjust the database selection bias and define a corrected score (|$\overline{R}$|). Based on the mapping of PubMed IDs and database sources, a confidence score (|${C}_i$|) was set for each corresponding PPI. BMA accounts for the heterogeneity and incompleteness of the scores assigned by different databases to the same PPI. BMA also reduces the variance of the score distribution by weighting each score according to its posterior probability. The confidence score is a metric to value the verified existence of the PPIs, which means the amount of the detection and indexing by publications and databases.
A subnetwork including 349 ND-related proteins and 4891 PPIs shows the shared connections between different diseases (Figure 4A). Some proteins were exclusively related to each disease. Proteins playing roles in multiple NDs were regarded as overlaps between disease network communities (Figure 4B). An integrated neurodegeneration PPI network was constructed, including 349 ND-related proteins with 10 336 first neighbors (directly connected proteins) and 365 734 interactions. Cutoff for filtering high-ranking-score PPIs was applied to reduce the scale of the neurodegeneration-related network, which made a smaller amount of node and edges for easy visualization. Finally, the NDAtlas database collected 5325 proteins and 63 162 PPIs directly connected with ND-related proteins, covering 26 types of NDs. These diseases are presented in Table 2.
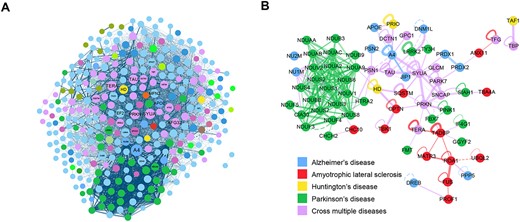
ND-involved PPI subnetwork illustrated the overlaps between different diseases. (A) A subnetwork containing 349 ND-related proteins and 4891 PPIs. Node size represents how many interactions does this protein have. (B) An overlap module extracted from AD–ALS–HD–PD networks. The module connects proteins exclusively related to single disease and proteins identified to play roles in multiple diseases.
Disease label . | Disease name . | UniProt . | Disease Ontology . | MESH . |
---|---|---|---|---|
MS | Multiple Sclerosis | DI-02604 | DOID:2377 | MESH:D009103 |
CPM | Central Pontine Myelinolysis | N/A | DOID:636 | MESH:D017590 |
OPCA | Olivopontocerebellar Atrophy | N/A | DOID:14784 | MESH:D009849 |
XLHAX | X-linked Hereditary Ataxia | N/A | DOID:0050953 | N/A |
PCD | Primary Cerebellar Degeneration | N/A | DOID:9277 | MESH:D013132 |
PXP | Plexopathy | N/A | DOID:3688 | N/A |
PBP | Progressive Bulbar Palsy | N/A | DOID:681 | MESH:D010244 |
LS | Lateral Sclerosis | N/A | DOID:230 | MESH:D016472 |
CAR | Autosomal Recessive Cerebellar Ataxia | DI-01061 | DOID:0050950 | N/A |
AD | Alzheimer Disease | DI-03832 | DOID:10652 | MESH:D000544 |
HD | Huntington Disease | DI-01754 | DOID:12858 | MESH:D006816 |
LFD | Lafora Disease | N/A | DOID:3534 | MESH:D020192 |
SPOAN | SPOAN Syndrome | N/A | DOID:0060491 | MESH:C563702 |
ICRD | Infantile Cerebellar–Retinal Degeneration | DI-03409 | DOID:0050883 | N/A |
MCD | Myoclonic Cerebellar Dyssynergia | N/A | DOID:12707 | MESH:D002527 |
ACCPN | Agenesis of the Corpus Callosum with Peripheral Neuropathy | DI-00054 | DOID:0090003 | N/A |
PMA | Progressive Muscular Atrophy | N/A | DOID:318 | MESH:D009134 |
SPAX | Spastic Ataxia | DI-04137 | DOID:0050952 | N/A |
PD | Parkinson Disease | DI-02134 | DOID:14330 | MESH:D010300 |
MN | Motor Neuritis | N/A | DOID:683 | MESH:D009443 |
PIDB | Pick Disease | DI-02937 | DOID:11870 | MESH:D020774 |
BCS | Balo Concentric Sclerosis | N/A | DOID:0060215 | MESH:D002549 |
CHAC | Choreaacanthocytosis | DI-01344 | DOID:0050766 | MESH:D054546 |
PPD | Postencephalitic Parkinson Disease | N/A | DOID:14332 | MESH:D010301 |
FENIB | Familial Encephalopathy with Neuroserpin Inclusion Bodies | DI-01567 | DOID:0050831 | MESH:C536841 |
ASD | Autism Spectrum Disorder | N/A | DOID:0060041 | MESH:D000067877 |
ALS | Amyotrophic Lateral Sclerosis | DI-00107 | DOID:332 | MESH:D000690 |
ADCA | Autosomal Dominant Cerebellar Ataxia | DI-03793 | DOID:1441 | MESH:D020754 |
SMA | Spinal Muscular Atrophy | DI-01055 | DOID:12377 | MESH:D009134 |
PSNP1 | Pseudobulbar Palsy | DI-02215 | DOID:12680 | MESH:D020828 |
EA | Episodic Ataxia | DI-00475 | DOID:963 | MESH:C580065 |
DLB | Lewy Body Dementia | DI-01901 | DOID:12217 | MESH:D020961 |
PCH | Pontocerebellar Hypoplasia | DI-02626 | DOID:0060264 | MESH:C580383 |
MSA | Multiple System Atrophy | DI-03867 | DOID:4752 | MESH:D012791, MESH:D019578 |
NBIA1 | Pantothenate Kinase-associated Neurodegeneration | DI-02126 | DOID:3981 | MESH:D006211 |
Disease label . | Disease name . | UniProt . | Disease Ontology . | MESH . |
---|---|---|---|---|
MS | Multiple Sclerosis | DI-02604 | DOID:2377 | MESH:D009103 |
CPM | Central Pontine Myelinolysis | N/A | DOID:636 | MESH:D017590 |
OPCA | Olivopontocerebellar Atrophy | N/A | DOID:14784 | MESH:D009849 |
XLHAX | X-linked Hereditary Ataxia | N/A | DOID:0050953 | N/A |
PCD | Primary Cerebellar Degeneration | N/A | DOID:9277 | MESH:D013132 |
PXP | Plexopathy | N/A | DOID:3688 | N/A |
PBP | Progressive Bulbar Palsy | N/A | DOID:681 | MESH:D010244 |
LS | Lateral Sclerosis | N/A | DOID:230 | MESH:D016472 |
CAR | Autosomal Recessive Cerebellar Ataxia | DI-01061 | DOID:0050950 | N/A |
AD | Alzheimer Disease | DI-03832 | DOID:10652 | MESH:D000544 |
HD | Huntington Disease | DI-01754 | DOID:12858 | MESH:D006816 |
LFD | Lafora Disease | N/A | DOID:3534 | MESH:D020192 |
SPOAN | SPOAN Syndrome | N/A | DOID:0060491 | MESH:C563702 |
ICRD | Infantile Cerebellar–Retinal Degeneration | DI-03409 | DOID:0050883 | N/A |
MCD | Myoclonic Cerebellar Dyssynergia | N/A | DOID:12707 | MESH:D002527 |
ACCPN | Agenesis of the Corpus Callosum with Peripheral Neuropathy | DI-00054 | DOID:0090003 | N/A |
PMA | Progressive Muscular Atrophy | N/A | DOID:318 | MESH:D009134 |
SPAX | Spastic Ataxia | DI-04137 | DOID:0050952 | N/A |
PD | Parkinson Disease | DI-02134 | DOID:14330 | MESH:D010300 |
MN | Motor Neuritis | N/A | DOID:683 | MESH:D009443 |
PIDB | Pick Disease | DI-02937 | DOID:11870 | MESH:D020774 |
BCS | Balo Concentric Sclerosis | N/A | DOID:0060215 | MESH:D002549 |
CHAC | Choreaacanthocytosis | DI-01344 | DOID:0050766 | MESH:D054546 |
PPD | Postencephalitic Parkinson Disease | N/A | DOID:14332 | MESH:D010301 |
FENIB | Familial Encephalopathy with Neuroserpin Inclusion Bodies | DI-01567 | DOID:0050831 | MESH:C536841 |
ASD | Autism Spectrum Disorder | N/A | DOID:0060041 | MESH:D000067877 |
ALS | Amyotrophic Lateral Sclerosis | DI-00107 | DOID:332 | MESH:D000690 |
ADCA | Autosomal Dominant Cerebellar Ataxia | DI-03793 | DOID:1441 | MESH:D020754 |
SMA | Spinal Muscular Atrophy | DI-01055 | DOID:12377 | MESH:D009134 |
PSNP1 | Pseudobulbar Palsy | DI-02215 | DOID:12680 | MESH:D020828 |
EA | Episodic Ataxia | DI-00475 | DOID:963 | MESH:C580065 |
DLB | Lewy Body Dementia | DI-01901 | DOID:12217 | MESH:D020961 |
PCH | Pontocerebellar Hypoplasia | DI-02626 | DOID:0060264 | MESH:C580383 |
MSA | Multiple System Atrophy | DI-03867 | DOID:4752 | MESH:D012791, MESH:D019578 |
NBIA1 | Pantothenate Kinase-associated Neurodegeneration | DI-02126 | DOID:3981 | MESH:D006211 |
Disease label . | Disease name . | UniProt . | Disease Ontology . | MESH . |
---|---|---|---|---|
MS | Multiple Sclerosis | DI-02604 | DOID:2377 | MESH:D009103 |
CPM | Central Pontine Myelinolysis | N/A | DOID:636 | MESH:D017590 |
OPCA | Olivopontocerebellar Atrophy | N/A | DOID:14784 | MESH:D009849 |
XLHAX | X-linked Hereditary Ataxia | N/A | DOID:0050953 | N/A |
PCD | Primary Cerebellar Degeneration | N/A | DOID:9277 | MESH:D013132 |
PXP | Plexopathy | N/A | DOID:3688 | N/A |
PBP | Progressive Bulbar Palsy | N/A | DOID:681 | MESH:D010244 |
LS | Lateral Sclerosis | N/A | DOID:230 | MESH:D016472 |
CAR | Autosomal Recessive Cerebellar Ataxia | DI-01061 | DOID:0050950 | N/A |
AD | Alzheimer Disease | DI-03832 | DOID:10652 | MESH:D000544 |
HD | Huntington Disease | DI-01754 | DOID:12858 | MESH:D006816 |
LFD | Lafora Disease | N/A | DOID:3534 | MESH:D020192 |
SPOAN | SPOAN Syndrome | N/A | DOID:0060491 | MESH:C563702 |
ICRD | Infantile Cerebellar–Retinal Degeneration | DI-03409 | DOID:0050883 | N/A |
MCD | Myoclonic Cerebellar Dyssynergia | N/A | DOID:12707 | MESH:D002527 |
ACCPN | Agenesis of the Corpus Callosum with Peripheral Neuropathy | DI-00054 | DOID:0090003 | N/A |
PMA | Progressive Muscular Atrophy | N/A | DOID:318 | MESH:D009134 |
SPAX | Spastic Ataxia | DI-04137 | DOID:0050952 | N/A |
PD | Parkinson Disease | DI-02134 | DOID:14330 | MESH:D010300 |
MN | Motor Neuritis | N/A | DOID:683 | MESH:D009443 |
PIDB | Pick Disease | DI-02937 | DOID:11870 | MESH:D020774 |
BCS | Balo Concentric Sclerosis | N/A | DOID:0060215 | MESH:D002549 |
CHAC | Choreaacanthocytosis | DI-01344 | DOID:0050766 | MESH:D054546 |
PPD | Postencephalitic Parkinson Disease | N/A | DOID:14332 | MESH:D010301 |
FENIB | Familial Encephalopathy with Neuroserpin Inclusion Bodies | DI-01567 | DOID:0050831 | MESH:C536841 |
ASD | Autism Spectrum Disorder | N/A | DOID:0060041 | MESH:D000067877 |
ALS | Amyotrophic Lateral Sclerosis | DI-00107 | DOID:332 | MESH:D000690 |
ADCA | Autosomal Dominant Cerebellar Ataxia | DI-03793 | DOID:1441 | MESH:D020754 |
SMA | Spinal Muscular Atrophy | DI-01055 | DOID:12377 | MESH:D009134 |
PSNP1 | Pseudobulbar Palsy | DI-02215 | DOID:12680 | MESH:D020828 |
EA | Episodic Ataxia | DI-00475 | DOID:963 | MESH:C580065 |
DLB | Lewy Body Dementia | DI-01901 | DOID:12217 | MESH:D020961 |
PCH | Pontocerebellar Hypoplasia | DI-02626 | DOID:0060264 | MESH:C580383 |
MSA | Multiple System Atrophy | DI-03867 | DOID:4752 | MESH:D012791, MESH:D019578 |
NBIA1 | Pantothenate Kinase-associated Neurodegeneration | DI-02126 | DOID:3981 | MESH:D006211 |
Disease label . | Disease name . | UniProt . | Disease Ontology . | MESH . |
---|---|---|---|---|
MS | Multiple Sclerosis | DI-02604 | DOID:2377 | MESH:D009103 |
CPM | Central Pontine Myelinolysis | N/A | DOID:636 | MESH:D017590 |
OPCA | Olivopontocerebellar Atrophy | N/A | DOID:14784 | MESH:D009849 |
XLHAX | X-linked Hereditary Ataxia | N/A | DOID:0050953 | N/A |
PCD | Primary Cerebellar Degeneration | N/A | DOID:9277 | MESH:D013132 |
PXP | Plexopathy | N/A | DOID:3688 | N/A |
PBP | Progressive Bulbar Palsy | N/A | DOID:681 | MESH:D010244 |
LS | Lateral Sclerosis | N/A | DOID:230 | MESH:D016472 |
CAR | Autosomal Recessive Cerebellar Ataxia | DI-01061 | DOID:0050950 | N/A |
AD | Alzheimer Disease | DI-03832 | DOID:10652 | MESH:D000544 |
HD | Huntington Disease | DI-01754 | DOID:12858 | MESH:D006816 |
LFD | Lafora Disease | N/A | DOID:3534 | MESH:D020192 |
SPOAN | SPOAN Syndrome | N/A | DOID:0060491 | MESH:C563702 |
ICRD | Infantile Cerebellar–Retinal Degeneration | DI-03409 | DOID:0050883 | N/A |
MCD | Myoclonic Cerebellar Dyssynergia | N/A | DOID:12707 | MESH:D002527 |
ACCPN | Agenesis of the Corpus Callosum with Peripheral Neuropathy | DI-00054 | DOID:0090003 | N/A |
PMA | Progressive Muscular Atrophy | N/A | DOID:318 | MESH:D009134 |
SPAX | Spastic Ataxia | DI-04137 | DOID:0050952 | N/A |
PD | Parkinson Disease | DI-02134 | DOID:14330 | MESH:D010300 |
MN | Motor Neuritis | N/A | DOID:683 | MESH:D009443 |
PIDB | Pick Disease | DI-02937 | DOID:11870 | MESH:D020774 |
BCS | Balo Concentric Sclerosis | N/A | DOID:0060215 | MESH:D002549 |
CHAC | Choreaacanthocytosis | DI-01344 | DOID:0050766 | MESH:D054546 |
PPD | Postencephalitic Parkinson Disease | N/A | DOID:14332 | MESH:D010301 |
FENIB | Familial Encephalopathy with Neuroserpin Inclusion Bodies | DI-01567 | DOID:0050831 | MESH:C536841 |
ASD | Autism Spectrum Disorder | N/A | DOID:0060041 | MESH:D000067877 |
ALS | Amyotrophic Lateral Sclerosis | DI-00107 | DOID:332 | MESH:D000690 |
ADCA | Autosomal Dominant Cerebellar Ataxia | DI-03793 | DOID:1441 | MESH:D020754 |
SMA | Spinal Muscular Atrophy | DI-01055 | DOID:12377 | MESH:D009134 |
PSNP1 | Pseudobulbar Palsy | DI-02215 | DOID:12680 | MESH:D020828 |
EA | Episodic Ataxia | DI-00475 | DOID:963 | MESH:C580065 |
DLB | Lewy Body Dementia | DI-01901 | DOID:12217 | MESH:D020961 |
PCH | Pontocerebellar Hypoplasia | DI-02626 | DOID:0060264 | MESH:C580383 |
MSA | Multiple System Atrophy | DI-03867 | DOID:4752 | MESH:D012791, MESH:D019578 |
NBIA1 | Pantothenate Kinase-associated Neurodegeneration | DI-02126 | DOID:3981 | MESH:D006211 |
3D structural docking status of PPI partners
NDAtlas visualized 416 docking pairs in total by calculating structural docking of ND-related proteins. Protein Data Bank (PDB) format protein structure files were retrieved for binary interaction docking. A pipeline mPPI [24] was implemented to produce the docking scores of these interactions and visualize the 3D structures of ND-related PPIs. We used docking score, which quantifies the structural affinity of protein binding, as a property of protein interaction structures in PPIs related to ND. A higher docking score represents a more favorable interaction. Furthermore, mPPI can predict the high-resolution conformation of interacting proteins and provide a detailed view of multiple PPIs. When a user clicks one of the nodes or edges, a popup will appear. Important proteins interfaces were illustrated using a modified 3D structure viewer.
To investigate how the state of protein docking regulates the occurrence of diseases by affecting the changes in protein interactions, this study selected the related proteins of four major diseases: AD, PD, HD and ALS.
The PPI subnetwork and the docking subnetwork composed of edges formed by structural docking were, respectively, constructed for these related proteins, and the results indicated that there were obvious differences in the topological structures of the two. Given that not all PPIs can calculate the corresponding docking score, this result indicates that some PPIs may not lead to the docking binding of proteins. This phenomenon can be explained by the fact that there is no physical interaction between non-docking proteins, but there are other forms of interaction due to the various forms of PPI. PD-associated protein LRRK2 contains several docking proteins. A subnetwork was built around this protein to get the PPI subnetwork and the structural docking subnetwork of LRRK2. The PPI subnetwork contains 70 interacting proteins of LRRK2, and 25 proteins have a docking relationship with LRRK2 among them (Figure 5).
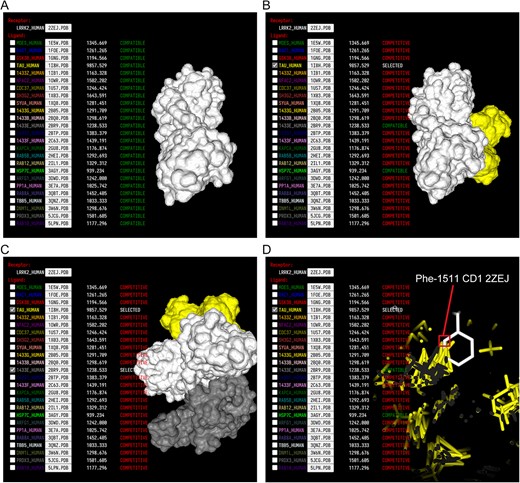
Docking visualization of LRRK2 protein and its interacting proteins. (A) LRRK2 protein structure, the central protein LRRK2 (as the receptor) is displayed by default when the mPPI plugin interface is opened. (B) Select a docking protein TAU (as a ligand), the docking structure is displayed on the right, and the docking protein list on the left is updated accordingly, showing the compatibility or competition of each docking protein with the current structure. (C) A compatible multiprotein docking complex of Tau and 1433E protein. (D) A partial view of the domain of LRRK2 showing atomic details of its docking site with Tau protein. A cross-section view highlights the Phe residue of the amino acid chain of LRRK2 and the CD1 atom position. Phe: Phenylalanine; 2ZEJ: PDB identifier of LRRK2 structure; CD1: atomic position identifier.
These docked structures were visualized using the mPPI tool (Figure 5A and B). The docking scores and compatibility status of the above docking proteins are shown in Figure 5C and D. Although there are many docking proteins in LRRK2 subnetwork, docking proteins compete, which makes them unable to dock at the same time. Tau protein is a multi-disease-related protein that works together across AD, PD and ALS [25–27]. It has interactions with the PD-related protein LRRK2. The docking structure of Tau and LRRK2 is calculated by mPPI, with a docking score of 9857.529. After the Tau protein is selected, the status of most of the docking proteins, including Rab proteins and 14-3-3 family proteins, is displayed as ‘competition,’ and only 1433E is ‘compatible.’ Tau protein was shown to compete with other proteins when they were chosen to dock with LRRK2. This phenomenon may indicate that the docking state of the proteins may affect the docking state of the competing docking proteins, causing a temporary change in the topological state of the PPI network.
There is no sufficient evidence supporting that Tau and other proteins competitive binding to LRRK2 has biological significance on the pathogenesis of PD. However, the inhibition of phosphorylation process of Rab8 protein by LRRK2 was detected by experiments [28], which influences the binding of LRRK2 with Tau. Another example indicated the TANK-binding kinase 1 (TBK1) - Optineurin (OPTN) binding is consistent with experimental research (Supplementary Figure S2). It has been reported that TBK1-OPTN exclusive binding can regulate the mitophagy pathway related with ALS [29, 30].
It is necessary to further understand the protein-disease regulatory relationship and to find the impact of protein docking on PPI disease modules, especially the combination of multi-target drugs, which may guide the development of pleiotropic drugs.
The cross-disease PPI modules
Cross-disease proteins are used as entry points to construct the details of the overlapping parts of the disease subnetwork, which are important to the disease mechanism.
The relationship between diseases was revealed by the statistical analysis of multiple proteins that interact with various NDs. The cross-disease linkages of protein interactions among 12 NDs were visualized (Figure 6A). According to the network, 85 proteins have played roles in more than a disease, while 346 proteins are related to a single disease. Supplementary Figure S3A presented the distribution of ranking scores and confidence for multi- and single-disease proteins. There is no significant increase in the number of interacting partners by the number of disease associations of the proteins. We speculate that the bias of relevant research resources on the important proteins of individual diseases is a key reason for the discrepancies in the data.
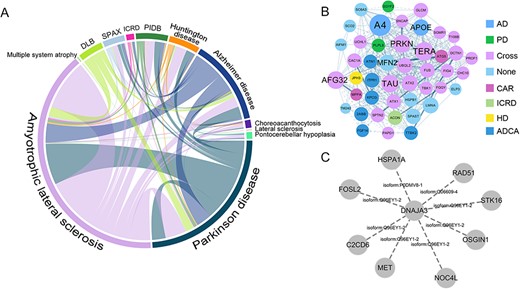
PPI cross-disease correlation and cross-disease module mining in ND. (A) The cross-disease PPI data statistics show the number of shared associations and interactions between NDs. Each arc represents a kind of ND, and the longer the arc, the more the number of PPIs related to the disease. Twelve kinds of NDs were selected to calculate the overlap, and there are shared interactions among diseases involving neurodegenerative symptoms. DLB: Lewy body dementia; SPAX: Spastic ataxia; ICRD: Infantile cerebellar-retinal degeneration; PIDB: Pick’s disease (frontotemporal dementia). (B) A protein interaction module whose function is significantly enriched in neurophysiologically related items such as cell death, cellular homeostasis and nervous system development. The proteins in the module are more closely related to other proteins in the network through PPI. AD: Alzheimer diseases; PD: Parkinson’s disease; Cross: cross multiple diseases; CAR: autosomal recessive cerebellar ataxia; HD: Huntington’s disease; ICRD: infantile cerebellar–retinal degeneration; ADCA: autosomal dominant cerebellar ataxia. (C) DNAJA3 protein isoform 2 and its first adjacent node interacting proteins, the dotted line represents that these corresponding interactions all involve the isoform DNAJA3-2.
In the integrated ND-related PPI network, the network modules that are closely connected with the pathogenic proteins of AD, PD, HD, etc. were found (Figure 6B). The results of gene ontology (GO) enrichment analysis indicated that these proteins can be significantly enriched on items related to neurophysiological processes such as cell death, cellular homeostasis and nervous system development. The common biological roles in these diseases can be found if the proteins involved in the interaction in this module are further explored.
Based on the interaction data collected [31], we found that previous studies have verified the interaction between DNAJA3 protein isoform 2 (DNAJA3-2, Tid1-S) and ALS-related protein C2CD6 through yeast two-hybrid experiments. This protein belongs to the DnaJ protein family, and its subcellular location is in the mitochondria. This isoform also interacts with C2CD6 and OSGIN1 (oxidative stress-induced growth inhibitor 1) proteins (Figure 6C). DNAJA3 has alternative splicing events in the process of maintaining mitochondrial DNA and regulating the migration and invasion of gastric cancer cells. One of the products, DNAJA3-2, plays an important role in maintaining mitochondrial homeostasis [32]. In addition, another member of the DnaJ protein family, DNAJC11, is also involved in splicing variant events in mouse motor neuron lesions [33]. It is speculated that DNAJA3-2 may also play a role in regulating mitochondrial function in ALS. Table 3 shows the causative protein–protein isoform pairs that occur in NDs.
Disease label . | Interactor A . | Interactor B (isoform) . |
---|---|---|
AD | O00213 | P05067-4 |
AD | P49768 | P05067-4 |
AD | P51693 | P05067-4 |
ALS | Q53TS8 | Q96EY1-2 |
ALS | Q9GZT8 | O15197-2 |
ND | P42858 | P54257-2 |
ND | Q8IUH5 | Q96CV9-2 |
ND | Q8IUH5 | P48730-2 |
ND | Q8IUH5 | Q9UQB8-3, Q9UQB8-6 |
ND | Q8IUH5 | Q15047-2 |
ND | Q8IUH5 | P27986-2 |
ND | Q8IUH5 | Q14847-2 |
ND | Q8IUH5 | Q9UI08-2 |
PD | O60260 | Q8IZ52-2 |
PD | P46089 | P05067-4 |
PD | P49841 | P10636-8 |
PD | Q5S007 | P10636-8 |
PD | Q5S007 | Q8N6T3-2 |
PD | Q5S007 | O00429-3 |
PD | Q5S007 | O14640-2 |
PD | Q5S007 | P48729-2 |
PD | Q9BXM7 | Q9Y3I1-1 |
PD | Q9Y6H5 | O60229-2 |
Disease label . | Interactor A . | Interactor B (isoform) . |
---|---|---|
AD | O00213 | P05067-4 |
AD | P49768 | P05067-4 |
AD | P51693 | P05067-4 |
ALS | Q53TS8 | Q96EY1-2 |
ALS | Q9GZT8 | O15197-2 |
ND | P42858 | P54257-2 |
ND | Q8IUH5 | Q96CV9-2 |
ND | Q8IUH5 | P48730-2 |
ND | Q8IUH5 | Q9UQB8-3, Q9UQB8-6 |
ND | Q8IUH5 | Q15047-2 |
ND | Q8IUH5 | P27986-2 |
ND | Q8IUH5 | Q14847-2 |
ND | Q8IUH5 | Q9UI08-2 |
PD | O60260 | Q8IZ52-2 |
PD | P46089 | P05067-4 |
PD | P49841 | P10636-8 |
PD | Q5S007 | P10636-8 |
PD | Q5S007 | Q8N6T3-2 |
PD | Q5S007 | O00429-3 |
PD | Q5S007 | O14640-2 |
PD | Q5S007 | P48729-2 |
PD | Q9BXM7 | Q9Y3I1-1 |
PD | Q9Y6H5 | O60229-2 |
Disease label . | Interactor A . | Interactor B (isoform) . |
---|---|---|
AD | O00213 | P05067-4 |
AD | P49768 | P05067-4 |
AD | P51693 | P05067-4 |
ALS | Q53TS8 | Q96EY1-2 |
ALS | Q9GZT8 | O15197-2 |
ND | P42858 | P54257-2 |
ND | Q8IUH5 | Q96CV9-2 |
ND | Q8IUH5 | P48730-2 |
ND | Q8IUH5 | Q9UQB8-3, Q9UQB8-6 |
ND | Q8IUH5 | Q15047-2 |
ND | Q8IUH5 | P27986-2 |
ND | Q8IUH5 | Q14847-2 |
ND | Q8IUH5 | Q9UI08-2 |
PD | O60260 | Q8IZ52-2 |
PD | P46089 | P05067-4 |
PD | P49841 | P10636-8 |
PD | Q5S007 | P10636-8 |
PD | Q5S007 | Q8N6T3-2 |
PD | Q5S007 | O00429-3 |
PD | Q5S007 | O14640-2 |
PD | Q5S007 | P48729-2 |
PD | Q9BXM7 | Q9Y3I1-1 |
PD | Q9Y6H5 | O60229-2 |
Disease label . | Interactor A . | Interactor B (isoform) . |
---|---|---|
AD | O00213 | P05067-4 |
AD | P49768 | P05067-4 |
AD | P51693 | P05067-4 |
ALS | Q53TS8 | Q96EY1-2 |
ALS | Q9GZT8 | O15197-2 |
ND | P42858 | P54257-2 |
ND | Q8IUH5 | Q96CV9-2 |
ND | Q8IUH5 | P48730-2 |
ND | Q8IUH5 | Q9UQB8-3, Q9UQB8-6 |
ND | Q8IUH5 | Q15047-2 |
ND | Q8IUH5 | P27986-2 |
ND | Q8IUH5 | Q14847-2 |
ND | Q8IUH5 | Q9UI08-2 |
PD | O60260 | Q8IZ52-2 |
PD | P46089 | P05067-4 |
PD | P49841 | P10636-8 |
PD | Q5S007 | P10636-8 |
PD | Q5S007 | Q8N6T3-2 |
PD | Q5S007 | O00429-3 |
PD | Q5S007 | O14640-2 |
PD | Q5S007 | P48729-2 |
PD | Q9BXM7 | Q9Y3I1-1 |
PD | Q9Y6H5 | O60229-2 |
Application in biological research
NDAtlas and the integrated method provided intuitive visualization of neurodegeneration-involved proteins and their interacting partners. NDAtlas can be used for various biological research purposes. For example, to investigate how proteins related to neurodegeneration affect autism spectrum disorder (ASD) subnetworks, the NDAtlas included proteins such as Hepatocyte growth factor receptor (MET). Users can search for MET and get detailed information about it, such as its interaction with DNAJA3 isoform 2 and other proteins. MET also appears in the GO enrichment results. MET is significantly involved in BPs related to oxidative stress. It is part of the same pathway as proteins from AD, PD and ALS, which is supported by recent research [34]. NDAtlas would help researchers explore more functional relations across neurological diseases.
CONCLUSIONS
In summary, NDAtlas possesses the largest integrated dataset, which includes 5325 human proteins (431 proved involved in 27 NDs) and 63 151 related PPIs with high confidence scores. The potential PPI modules participating in neurodegeneration pathogenesis were discovered by graph-theoretic clustering and GO enrichment. The network was enhanced by adding structural affinity information and docking visualization.
We conducted a comprehensive research and annotated the disease categories in the field of NDs with clear diagnostic classification, which is different from previous studies in this field that only focus on individual diseases. The integrated human PPI network was constructed, and the interaction information of protein isoforms was added. Use disease proteins as the core to study the characteristics of its related subnetworks and find disease functional modules, and build a web database with the collated data for queries related to this research field.
Researchers can conveniently discover more proteins and isoforms playing potential roles in NDs with the help of the NDAtlas database. With the accumulation of differential expression profiles and structural information of ND-related interactions, the resolution of the PPI network could get higher so that dynamic and 3D detail could be presented. A disease-involved curated PPI network will have enlightening significance for similar research exploring the crossovers between multiple diseases.
DISCUSSIONS
Because the data size of previous studies was biased due to limited experimental research, previous studies were aimed at elucidating the mechanisms of some prominent diseases [35–39]. The findings were usually influenced by the significant associations between proteins and diseases, which could narrow the view of the extensive group of targets. In this study, we used the most important PPI datasets related to NDs to show how diseases might be connected without enough evidence and to describe how disease modules might be connected. Although many processes behind neurodegeneration are still unclear, more reviewed expression data, like mass spectrometry data, are in demand to enlarge the volume of integrated network details.
Subnetwork and pathogenesis illuminated
NDAtlas provides an intuitive and interactive view that could illuminate a particular subnetwork and its pathological correlations. Researchers can easily check for overlap between diseases through the visualization of the selected interactions. By revealing their hidden mechanisms, this would help extend the knowledge of the NDs.
For the first time, individual isoforms can be separated from the canonical sequence and shown in the disease-related network. It will be helpful to understand the role of alternative splicing in the pathogenesis of NDs. Multiple pathways of NDs were found to have common factors during a systematic investigation. For example, ASD shares involved proteins and PPIs with PD and several types of cerebellar ataxia, supported by the evidence of neurodegeneration in the former research [40]. With an unbiased dataset generated from a larger number of diseases, it would be possible to draw an accurate and complete atlas of the cross-disease PPI network.
Dataset completeness
A pipeline was implemented to acquire datasets from public database sources. The curation and coverage of datasets were guaranteed by the in-house code, which can stably identify the data format generated from multiple sources. The pipeline to construct such a disease-involved curated PPI network will have enlightening significance for similar research on exploring the crossovers between multiple diseases.
Our method could be improved by incorporating additional proteomics data. The availability of such data is essential for the purposes of this investigation, given the extensive network scale. In our future work, further quantitative proteomics data will be investigated.
Structural affinity and visualization
The 3D visualization of protein structures was usually used to present single proteins. The traditional way to visualize PPIs is to consider each protein as a node and to connect the interactors with edges directly. The NGL viewer offers a new way of visualizing the binary interactions. The combination of plugins provides an abundant, high-resolution visualization not only for 2D networks but also for a detailed 3D docking view. We improved the NGL viewer to allow for multiple simultaneous interactions that could illustrate the compatible or competitive interface at an atomic level.
More structural information is required, such as small molecules binding with pathological proteins, which could be potential treatments and help with precision medicine. AlphaFold [41] is the most representative novel method for structure generation at the moment. This approach combines the physical and biological knowledge of protein structure with a deep learning algorithm based on multiple sequence alignment. Its accuracy can compete with the experimental structure, and its performance is also much better than other methods. AlphaFold-Multimer has significant advantages in predicting the complex structures of multiple chains [42], which is promising to identify possible binding pairs in NDAtlas. But AlphaFold-Multimer also has limitations, including a lower speed due to searching for multiple sequence alignment for every unique sequence. Besides, a structural database covering 98.5% of human proteins was generated by AlphaFold that was applied to the human proteome [43]. NDAtlas initially added the function to visit the protein structures in AlphaFold database, and will continue to predict newer and more complete protein binding information from more resources.
MATERIALS AND METHODS
Data collection
DaTo (https://bis.zju.edu.cn/dato/) is a constantly updated online resource of biological web servers and databases [44]. It was used to collect research of PPI databases, integrative neurodegeneration-related databases and human proteome details. The PPI databases were retrieved by searching the term ‘protein–protein interaction’ and setting the filter to ‘database’. The ‘Advanced search’ function was applied to collect ND-related PPI databases by the search terms including abstract: ‘neurodegenerative’, abstract: ‘alzheimer’, category: ‘protein–protein interaction’, filter: ‘database’ or any neurodegeneration-related terms. The candidate databases from search results were then manually curated. We visited the websites of these databases or projects, and downloaded the data tables in text format. The download sources of datasets were indexed in Supplementary Table S1. The PPI data tables are PSI-MITAB formatted [45]. Information on human protein entries, network annotations involving nodes and edges of the PPI network, and annotations involving diseases, BPs, etc. are included in the data tables.
UniProt [17] directly provides a complete map providing protein-disease linkages. We obtained list of disease labels from UniProt disease label data and Human Disease Ontology [46].
Network integration and scoring
We developed in-house Python scripts to process the datasets from the public PPI databases. These scripts ran under a Python environment with the requirement of pandas [47]. They merged PPI entries by same interactor IDs and source publications. The protein IDs were mapped to their UniProt IDs.
Then, the ranking score |$\overline{R}$| of PPI(i) is introduced in a BMA [23] manner. The formula is as follows:
Given a group of PPIs, each PPI is assigned multiple scores (|${O}_i$|) (1 ≤ i ≤ n) by n databases. Average value of all scores is calculated as |$\overline{S}$|. From the top 10% scoring of PPIs, the minimum number of databases was achieved as the weight |$M$|. Finally, a weighted score |$\overline{R}$| was generated.
In addition, a PPI has more relevant publications and more database collections, which can be used as a reference for its real existence to be more credibly verified. |$n$| represents the number of databases that contain this PPI. |$P$| represents the number of PubMed IDs that report the corresponding PPI, that is, the number of published papers related to the experiment. If either of these two values is equal to 0, it is set to 1. The average number of databases included in all PPIs is |$\overline{n}$|. The mean of all PPI publications is denoted as |$\overline{P}$|. The mean value measures the average level of the verification times of the overall PPI in the dataset. The greater the product of the database inclusion value of a single PPI and the value of the published literature, the higher the credibility is. Set the credibility of PPI(i) to |${C}_i$|, when |${C}_i$| >0, it means there are more than average level of evidence supporting this PPI. The formula is as follows:
The completed ranking score and confidence score were assigned to the corresponding PPI, and together with other annotations. To reduce the scale of the network, the cutoffs were set: PPIs with ranking score lower than the average value of all ranking scores were removed. Among 176 549 PPIs passing the ranking score cutoff, we selected those directly connected with the reviewed proteins in neurodegeneration. The overlap of NDs in circular visualization was created by the R package circlize [48].
Ontology enrichment
Import the integrated human PPI network into the network analysis and visualization software Cytoscape [49] to generate the overall network. To generate the disease involved protein subnetwork, we screened the verified protein entries related to disease tags and annotate them with abbreviated tags, put these protein entries into the overall protein interaction integration network and used Cytoscape to automatically find the first adjacent protein nodes.
BiNGO is a tool for analyzing the statistical overrepresentation of GO terms for a small set of genes/proteins in a biological network [50]. It grouped genes/proteins of the same GO protein enrichment. The nodes with higher scores and related interacting proteins were analyzed to obtain the enrichment results of GO BP terms, and to explore the functional connection between these proteins and the ND interaction network.
Taking the interaction subnetwork composed of disease-causing proteins and their first neighbors as the research object, the important nodes and highly dense network modules in each network were counted, respectively. MCODE is a graph-theoretic clustering algorithm that detects densely connected regions in large PPI networks as potential molecular complexes [51]. Using the MCODE plug-in in the Cytoscape software, the network modules with higher output scores were calculated, and the protein interaction complexes with similar network topology attributes of proteins and possibly close functional connections were obtained.
Docking and visualization
The mPPI tool [24] (http://bis.zju.edu.cn/mppi) has been integrated into NDAtlas. After integration, extract the interaction data in the database, process the target, and use the mPPI tool to download the PDB file of the target interaction. The dock module can be used to calculate and store structural docking results of target interacting protein pairs. mPPI will generate a database file, merge and supplement with the original database file of the PPI database, and generate docking data that can be seen by the structural docking visualization script net.php. The net.js script is used to display the one-to-many structural docking of the target interacting protein pair on the docking visualization page.
Database construction
The NDAtlas database was built on a Linux server and powered by Apache2 and PHP5. The MySQL service is used for storing data tables. The table includes information on human protein entries, network annotations related to nodes and edges of the PPI network, and annotations related to diseases, BPs, etc. Utilize the Cytoscape.js plugin to visualize the network interactively. After docking calculation, the one-to-many PPI docking interface structure of some proteins was visualized using mPPI.
A workflow was developed to systematically integrate more neurodegeneration-related interactome data.
Beyond the traditional interaction network, the scoring based on BMA, the protein isoforms and the structural affinity information were combined to the nodes and edges.
Construction of a neurodegenerative disease PPI database NDAtlas, which supports searching for proteins, disease names and functional enrichment.
For the first time, a 3D visualization plug-in of multi-protein docking was imported into the protein interaction database.
ACKNOWLEDGMENTS
Sida Li and Dahui Hu are acknowledged for the web server publishing, and searching for publications. Yincong Zhou is acknowledged for support on materials and methods.
FUNDING
National Key Research and Development Program of China (2016YFA0501704, 2018YFC0310602); the National Natural Sciences Foundation of China (31771477, 32070677); the Science and Technology Innovation Leading Scientist (2022R52035); the 151 talent project of Zhejiang Province (first level), Collaborative Innovation Center for Modern Crop Production co-sponsored by province and ministry.
AUTHOR CONTRIBUTIONS
Conceptualization by H.C. and M.C.; Writing—original draft preparation by H.C.; Methodology by H.C. and Y.Z.; Data curation by H.C.; Software—NDAtlas by H.C.; Software—mPPI by Y.Z.; Writing—review and editing by H.C., Y.Z., Y.L, P.Z. and M.C.; Supervision by M.C.; Project administration by M.C.; Funding acquisition by M.C. All authors have read and agreed to the published version of the manuscript.
DATA AVAILABILITY
The data presented in this study are available at http://bis.zju.edu.cn/ndatlas.
Author Biographies
Hongjun Chen is a PhD graduate at Department of Bioinformatics, College of Life Sciences, Zhejiang University. He focuses on the bioinformatics analysis and data integration of medical proteomics.
Yekai Zhou is a PhD candidate at Department of Computer Science, The University of Hong Kong. His research focuses on medical informatics, with a special interest in artificial intelligence and public health.
Yongjing Liu is a postdoctoral research fellow at Biomedical Big Data Center, the First Affiliated Hospital, Zhejiang University School of Medicine. His research focuses on biomedical data analysis, with a special interest in cancer genomics.
Peijing Zhang is a postdoctoral research fellow at School of Basic Medical Sciences, Zhejiang University School of Medicine. Her research focuses on bioinformatics analysis, with a special interest in deep learning and cross-species single cell landscape.
Ming Chen is a full professor in the Department of Bioinformatics, College of Life Sciences, Zhejiang University. He is the president of Bioinformatics Society of Zhejiang Province, China. His current research focuses on establishing useful bioinformatics tools and platforms to integrate and analyze massive biological datasets.