-
PDF
- Split View
-
Views
-
Cite
Cite
Xichen Lian, Yintao Zhang, Ying Zhou, Xiuna Sun, Shijie Huang, Haibin Dai, Lianyi Han, Feng Zhu, SingPro: a knowledge base providing single-cell proteomic data, Nucleic Acids Research, Volume 52, Issue D1, 5 January 2024, Pages D552–D561, https://doi.org/10.1093/nar/gkad830
- Share Icon Share
Abstract
Single-cell proteomics (SCP) has emerged as a powerful tool for detecting cellular heterogeneity, offering unprecedented insights into biological mechanisms that are masked in bulk cell populations. With the rapid advancements in AI-based time trajectory analysis and cell subpopulation identification, there exists a pressing need for a database that not only provides SCP raw data but also explicitly describes experimental details and protein expression profiles. However, no such database has been available yet. In this study, a database, entitled ‘SingPro’, specializing in single-cell proteomics was thus developed. It was unique in (a) systematically providing the SCP raw data for both mass spectrometry-based and flow cytometry-based studies and (b) explicitly describing experimental detail for SCP study and expression profile of any studied protein. Anticipating a robust interest from the research community, this database is poised to become an invaluable repository for OMICs-based biomedical studies. Access to SingPro is unrestricted and does not mandate a login at: http://idrblab.org/singpro/.
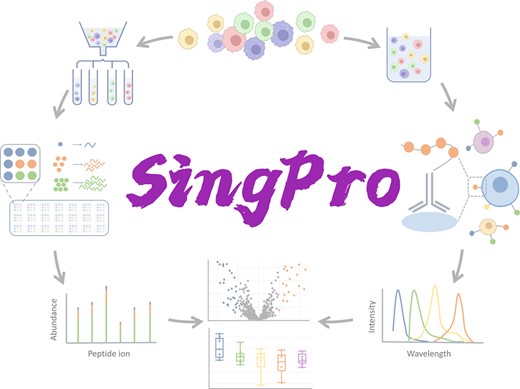
Introduction
Single-cell proteomics (SCP) has emerged as a powerful tool for detecting cellular heterogeneity, offering unprecedented insights into biological mechanisms that are masked in bulk cell populations (1,2). As shown in Figure 1, two techniques are widely adopted in current SCP study (3): flow cytometry-based one (FC-SCP) measuring up to 50 proteins per cell based on the antibodies, which demonstrates remarkable ability to identify disease-specific cell subpopulation and monitor signal transduction (4–7); mass spectrometry-based one (MS-SCP) quantifying over 600 proteins per cell but with limited throughput and relatively lower sensitivity when comparing to FC-SCP, which makes it suitable for identifying new markers and tracking rare cell populations (8–11). Both techniques are powerful and have been frequently adopted to measure the time of delivery (12), uncover the heterogeneity among cells (13,14), realize the high-content drug screening (15), and so on.
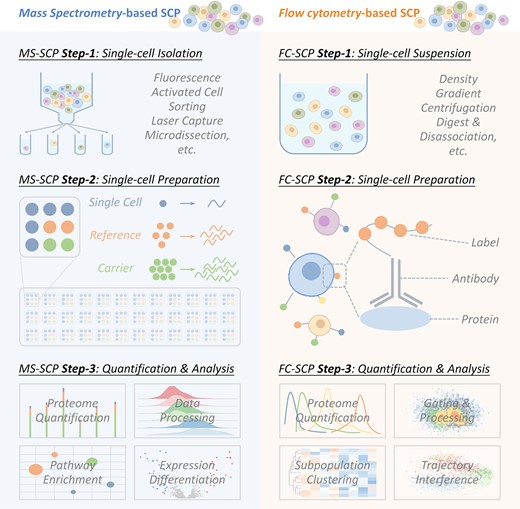
The flowchart of two typical experimental procedures adopted in single-cell proteomic (SCP) analysis, including mass spectrometry-based and flow cytometry-based SCP analyses. For mass spectrometry-based SCP, single cell is (a1) first isolated, (a2) then lysed, digested & labeled and (a3) finally quantified based on MS data & analyzed using pathway enrichments, expression differentiation, and so on. For flow cytometry-based SCP, all cells are (b1) first treated into single-cell suspension, (b2) then stained with antibody, and (b3) finally quantified based on FC data & analyzed using cell subpopulation identification, time trajectory interference, and so on.
However, the extremely-high experimental cost and time-consuming analytical process limit the availability of the publicly accessible SCP data (16–18). An SCP study asks for sophisticated data processing and analysis procedure, and the raw data should be provided to select suitable process (19–21). Meanwhile, it is extremely difficult to conduct SCP-based meta- and multiomic-analysis if the corresponding raw SCP data are unavailable (22–25). For example, the integration of SCP and single-cell transcriptomics (SCT) data is regarded as revolutionary for the understandings of biological characteristics/dynamics (26,27), but it is greatly hampered by the unequal amount of raw data between SCP and SCT (28). With the rapid advancements in AI-based time trajectory analysis and cell subpopulation identification, there exists a pressing need for a database that not only provides SCP raw data but also explicitly describes experimental details and protein expression profiles (29–32).
So far, several proteomics-related databases have been developed (33–42). Some of them provide storage and download of mass spectrometry-based bulk proteomic data, such as ProteomeXchange (33), PRIDE (34), and iProX (36); some others are public repositories providing the experimental data generated using cytometry technique to facilitate cell sorting and immunophenotyping, such as FlowRepository (39) and Immport (40). There are also several R packages that can be used to obtain SCP data, such as scpdata (43). However, none of them are dedicated to provide SCP raw data for either MS-based or FC-based technique. Moreover, the existing databases are specialized in offering the scientific storage of proteomic data, but lack of description on experimental details (such as study procedure, sample label, annotated cell type, and method for single-cell sorting and preparation) and absent of application of data processing and analysis, which makes it difficult for researcher, especially for those without a background in bioinformatics, to intuitively use the provided data and comprehend the protein expression profiles. Thus, a database that is specialized in providing SCP raw data and the explicit description on experimental details and expression profiles is urgently needed.
To address this gap, we developed ‘SingPro’, a database tailored for single-cell proteomics. First, a systematic literature review was conducted, which resulted in a total of 204 studies (129 case-control, 21 multi-class and 54 single-arm studies) containing the SCP raw data of >625 million cells and >16 000 proteins. Second, the experimental details (antibody panel, study procedure, sample label, annotated cell type, method for single-cell sorting and preparation, etc.) were manually retrieved and standardized based on the original publications. Third, all raw data were processed and analyzed using well-established tools to measure the expression profiles among sample groups for each protein. Finally, a user-friendly interface with quick search utility was constructed to facilitate the use of SCP data. All in all, SingPro database is unique in (a) systematically providing the SCP raw data for both mass spectrometry-based and flow cytometry-based studies and (b) explicitly describing the experimental detail of SCP studies and expression profiles of studied proteins. Due to the broad interest from research community, this database is highly expected to be a valuable repository facilitating OMIC-based biomedical studies.
Factual content and data retrieval
Systematic collection of the single-cell proteomic data
The SingPro's single-cell proteomic data were systematically collated as outlined below. First, comprehensive literature review on single-cell proteomic data was conducted by searching PubMed using such keyword combinations as: ‘mass cytometry + proteomics’, ‘flow cytometry + proteomics’, ‘single-cell + proteomics’, ‘single-cell + mass spectrometry’, ‘cytometry time-of-flight’, which resulted in a total of 5780 articles. Second, detailed information of each single-cell proteomic datum (such as studied species, disease indications, clinical status & experimental procedure) was systematically retrieved from original publications, and unified & crosslinked to well-established databases. Finally, a total of 204 studies (129 case-control, 21 multi-class & 54 single-arm studies) containing the SCP raw data of >625 million cells and >16 000 proteins were collected. As a result, SingPro provided SCP data from human and various model organisms (such as Mus musculus, Xenopus laevis and Macaca mulatta) and tissues/organs (such as peripheral blood, kidney and breast). Additionally, the curated data cover an expansive range of diseases, encompassing not just cancer but also conditions like infections, digestive system ailments, and more.
General information of each SCP dataset in SingPro
For each SCP study, its general information was shown in the upper section of the corresponding SCP webpage, such as: project ID, project title, descriptions, research type, reference links to the original publications, data processing, and analytical tool (as illustrated in Figure 2). Two of the commonly adopted research types in SCP included cell subpopulation identification (which were applied to discover new marker protein (44–46)) and time trajectory interference (which had been adopted to reveal signal pathway and the mechanism underlying disease progression (47,48). To make it convenient for users to identify the ideal data for their own research purposes and select suitable analytical algorithm, various research types of the collected SCP study were summarized, which were cell population identification, time trajectory interference (with clarified timepoints), comparative study (with description of different data groups) and novel method (with description of the experimental procedures and equipment innovations). Additionally, SingPro introduces and links to prominent data processing tools like ANPELA (49) and Cytobank (41), streamlining the process for users eager to repurpose the data.
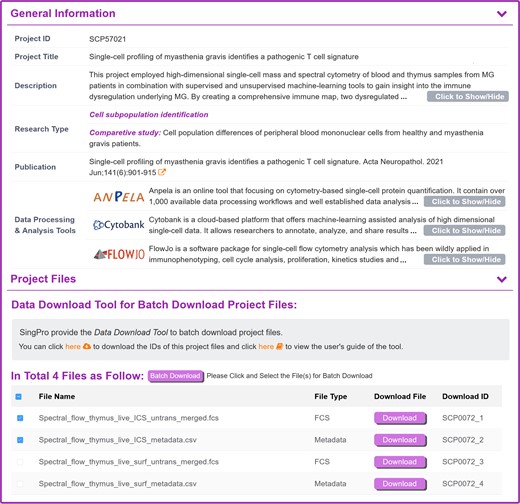
A typical SingPro page describing the general information of a single-cell proteomics study. The information of each study & dataset is explicitly provided in the upper section, which includes: project ID, project title, description, research type, sample type (single-cell/small-cell-population), reference and external linkage of well-established data processing & analysis tools. Project files are established in the following section, including: file type, download linkage (for instant download of individual file), download ID and the corresponding staining panel. The user can select the desired file(s) in the checkbox and click ‘Package Download’ to activate the batch download. For user who want to batch download the files from different studies, a data download tool is also provided for enabling the download of multiple files from various studies.
Describing the quantification process of a SCP dataset
For each flow cytometry-based SCP study, its biological information, such as species, tissue, cell type and condition of the study were provided in SingPro database. According to the type of antibody, FC-SCP studies can be further divided into two quantification methods: fluorescence-based flow cytometry using fluorochrome labels, and cytometry by time of flight (CyTOF) using heavy metal isotopes label. For each method, various data processing and analysis tools were developed, such as CATALYST and CytoSpill were compensation tools specially for CyTOF. To enable users to choose subsequent analysis methods appropriate for that data, SingPro provided quantification process description, such as quantification methods, instrument, data processing method and data analysis method adopted in the original publications. The staining panel was also provided which allowed the researchers to directly determine whether the study contained their preferred proteins or whether the desired clustering could be achieved. The staining panel of each study contains information such as protein name, external link to uniport, fluorochrome/metal isotopes, category (intracellular or surface protein) and clone number (as shown in Figure 3).
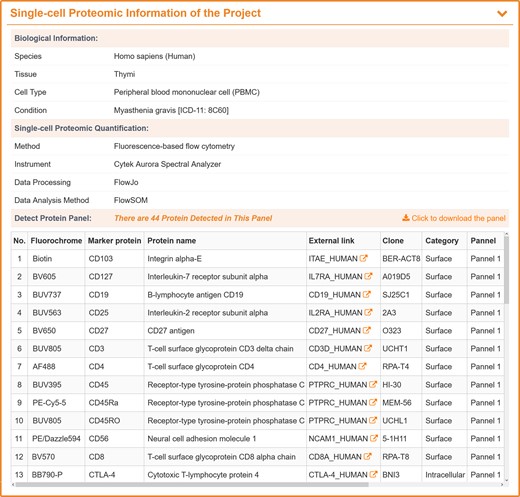
A typical SingPro page describing the quantification process for flow cytometry-based SCP. Each page is carefully organized to three sections: Biological Information (studied species, experiment tissue/organ, analyzed cell type, pathological/physiological conditions, etc.), Single-cell Proteomic Quantification (applied quantification approach, experimental platform, methods for data processing and analysis, etc.), and Protein Panel (fluorochrome, protein marker, external link, clone, category (surface/intracellular) and panel number).
For each mass spectrometry-based SCP study, the cell type information was explicitly described, including cell line name, species, organism, condition (healthy or specific diseases), and external linkage to other well-established database, such as Cellosaurus (50). One of the major difficulties of the MS-SCP was its miniscule amount of proteins in each cell, proper sorting and subsequence preprocessing methods were essential for preserving the protein from digestion loss and surfaces adsorption (51). Therefore, the single-cell sorting and preprocessing method of each dataset were manually collected and explicitly described in SingPro, such as CelleONE (52), nanoPOTS (53) and other popular preprocessing platforms. Furthermore, SingPro also described quantification methods used, such as LC-MS/MS (liquid chromatography-mass spectrometry), HPLC-FAIMS-MS/MS (high performance liquid chromatography-field asymmetric ion mobility spectrometry-MS), and CE-ESI-HRMS (capillary electrophoresis-electrospray ionization-high resolution MS), quantification strategy (dimethyl labeled, TMT labeled, label-free, data acquisition, etc.) and the instrument to facilitate the selection of appropriate analytical algorithms (shown in Figure 4).
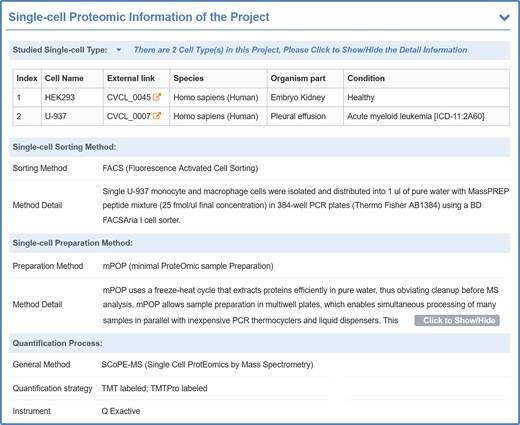
A typical SingPro page describing quantification process of mass spectrometry-based SCP. Each page is carefully organized to four sections: Studied Single-cell Type (studied species, cells, pathological/physiological condition, etc.), Sorting Method (method name & its application detail), Preparation Method (method name & its application detail) and Quantification Process (applied quantification approach, quantification strategy, experimental platform, etc.).
SCP data processing and protein expression profiles
For flow cytometry-based SCP data, all data were imported into FlowJo (54) where the quality control was conducted using FlowAI (55). After removing the anomalies, data were then manually gated for removing dead cells & other atypical events, and scaled with the arcsine transformation (56–59). The data were grouped according to the original publication, the statistical correlations of protein expression difference among groups were determined using two-way student t-test, and P-values <0.05 were considered statistically significant. The analytical result was displayed on the page in the form of box diagrams, user can select all the proteins in the dyeing panel through the drop-down box to view the expression level between groups (as shown in Figure 5).
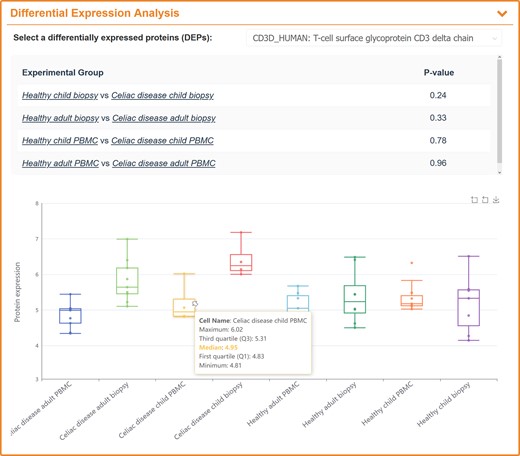
A typical SingPro page describing the expression variations of studied protein among multiple groups using flow cytometry-based SCP data. All proteins in staining panel are included into the drop-down-box where a user can select the protein of interest. The P-value of the selected protein between two groups is calculated and provided.
For mass spectrometry-based SCP data, the raw data were processed using MaxQuant (version 2.4.0.0) (60). TMT channel, digestion enzymes, missed cleavage, variable modifications and many other parameters were set by referring to the original publication. Both peptide and protein were filtered with false discovery rate <1% to ensure the identification confidence. The corrected reporter ion intensities from MaxQuant were imported into Perseus (61). Reverse and contaminant proteins were filtered out and proteins containing over 70% valid values in each sample were considered. All data were then log-transformed and missing values were imputed based on standard distributions by setting width and downshift to 0.3 and 1.8, respectively (62). Fold changes and two-way student t-tests were applied to indicate the significant differences by setting fold change and P-value to >2 and <0.05, respectively). Since MS-SCP quantified much more proteins than FC-SCP, and only few of the thousand's proteins detected by MS-SCP were differentially expressed, SingPro provided the volcano maps to show which protein had differential expressed, and then the expression level of those proteins among multiple groups was shown using box maps (illustrated in Figure 6).
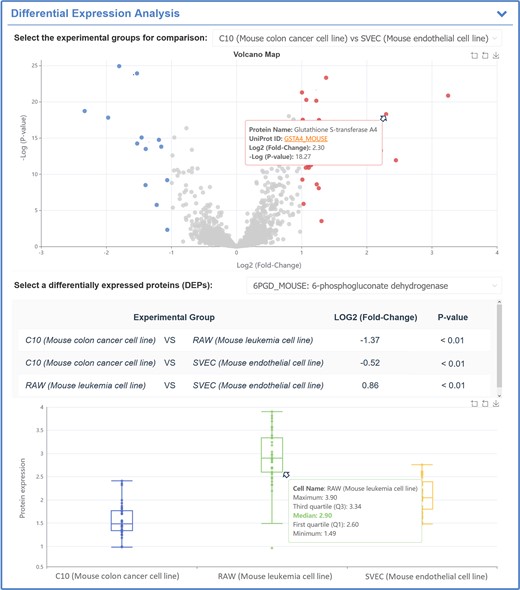
A typical SingPro page describing the expression variations of studied protein among multiple groups using mass spectrometry-based SCP data. Particularly, the volcanic map between two groups is calculated to provide the differential expression profiles for proteins (the horizontal coordinate indicates the log2 fold change (Log2FC) and vertical one denotes log P-value (Log P); the proteins are colored in red and blue based on their Log2FC & P-value (Log2FC > 1 & P-value < 0.05 and Log2FC ←1 & P-value < 0.05, respectively). The differentially expressed proteins can be selected, and the P-value of selected protein between two groups is calculated and provided.
Standardization, access and download of the SCP data
To make the access and analysis of SingPro data convenient for the users, all the collected data were carefully cleaned up and then systematically standardized. These standardizations included: (a) all proteins, cell lines, species, and diseases in SingPro were cross-linked to well-established databases such as uniprot (63), Cellosaurus (50) and NCBI Taxonomy (64); (b) all diseases were standardized using the WHO ICD-11 (65). SingPro provided a user-friendly interface that can conveniently browse and search data, and the quick search utility was provided to allow users to find desired single cell proteomic data in main search frame or in a pull-down menu based on experiment accession numbers and the sample parameters, including quantification method, disease indication, species, tissue, marker proteins, etc. All data could be downloaded (including the MaxQuant analysis results, the raw data, and many other related files, such as the protein sequences in FASTA formats, and compensation matrix). Users can download all these data directly from the corresponding page or download and edit the desired file list then using the batch download tool constructed and provided by SingPro database.
Conclusion and prospectives
In this study, a new database, named SingPro, was introduced to provide comprehensive single-cell proteomic (SCP) data. It was specialized in (a) systematically offering SCP raw data for both mass spectrometry- and flow cytometry-based studies and (b) explicitly describing experimental details of SCP studies and expression profiles of proteins. With the latest breakthrough of high-sensitivity mass spectrometry techniques, there will be an exponentially increasing amount of single-cell proteomic data. Therefore, SingPro will be updated in a timely fashion. Popular analysis and visualization tools, such as cell subpopulation analysis based on different clustering methods, time trajectory inference and pathway enrichment analysis will be added to keep pace with ongoing research. Due to the broad interest from research community, SingPro was highly expected to be a functional and popular complement to the existing molecular biological databases (63,66–75) in facilitating current OMIC-based studies.
Data availability
All single-cell proteomics data can be viewed, accessed, and downloaded from SingPro, which is freely accessible without any login requirement by all users at: http://idrblab.org/singpro/.
Funding
National Natural Science Foundation of China [82373790, 22220102001, U1909208, 81872798]; Natural Science Foundation of Zhejiang Province [LR21H300001]; National Key R&D Program of China [2022YFC3400501]; Leading Talent of the ‘Ten Thousand Plan’ National High-Level Talents Special Support Plan of China; The Double Top-Class Universities [181201*194232101]; Fundamental Research Funds for Central Universities [2018QNA7023]; Key R&D Program of Zhejiang Province [2020C03010]; Westlake Laboratory (Westlake Laboratory of Life Science & Biomedicine); Alibaba Cloud; Information Technology Center of Zhejiang University; Alibaba-Zhejiang University Joint Research Center of Future Digital Healthcare. Funding for open access charge: Natural Science Foundation of Zhejiang Province [LR21H300001].
Conflict of interest statement. None declared.
References
Author notes
The authors wish it to be known that, in their opinion, the first three authors should be regarded as Joint First Authors.
Comments