-
PDF
- Split View
-
Views
-
Cite
Cite
Marcel E. Dinger, Ken C. Pang, Tim R. Mercer, Mark L. Crowe, Sean M. Grimmond, John S. Mattick, NRED: a database of long noncoding RNA expression, Nucleic Acids Research, Volume 37, Issue suppl_1, 1 January 2009, Pages D122–D126, https://doi.org/10.1093/nar/gkn617
- Share Icon Share
Abstract
In mammals, thousands of long non-protein-coding RNAs (ncRNAs) (>200 nt) have recently been described. However, the biological significance and function of the vast majority of these transcripts remain unclear. We have constructed a public repository, the Noncoding RNA Expression Database (NRED), which provides gene expression information for thousands of long ncRNAs in human and mouse. The database contains both microarray and in situ hybridization data, much of which is described here for the first time. NRED also supplies a rich tapestry of ancillary information for featured ncRNAs, including evolutionary conservation, secondary structure evidence, genomic context links and antisense relationships. The database is available at http://jsm-research.imb.uq.edu.au/NRED, and the web interface enables both advanced searches and data downloads. Taken together, NRED should significantly advance the study and understanding of long ncRNAs, and provides a timely and valuable resource to the scientific community.
INTRODUCTION
Non-protein-coding RNAs (ncRNAs) are currently the subject of intense research activity. Just a decade ago, the number of known ncRNAs was restricted to a small number of housekeeping RNAs (including ribosomal RNAs, transfer RNAs and spliceosomal RNAs) and an even more limited collection of regulatory RNAs, such as lin-4 in Caenorhabditis elegans (1) and H19 and Xist in mammals (2,3). Since then, discovery of novel ncRNAs has increased dramatically. Thousands of short ncRNAs have been identified, and various classes—including microRNAs, endogenous short interfering RNAs, PIWI-interacting RNAs and small nucleolar RNAs—can now be readily distinguished on the basis of length, biogenesis, structural/sequence features and function (4,5). Large numbers of long ncRNAs (>200 nt) have also been discovered using full-length cDNA cloning/sequencing and genomic tiling array technologies to comprehensively profile the transcriptome (6–9). In the mouse genome, for instance, long ncRNAs are estimated to number ∼30 000 (7,10), and in the human genome the majority of transcription occurs as long ncRNAs (9).
In recent years, long ncRNAs have been implicated in a variety of regulatory processes, ranging from X chromosome inactivation, genomic imprinting and chromatin modification to transcriptional activation, transcriptional interference and nuclear trafficking (11,12). The exact mechanisms by which these long ncRNAs exert their effects remain unclear. Nevertheless, it has become apparent that long ncRNAs can act both in cis (13) and in trans (14), and that some function as precursors for short ncRNAs (9,15–17), while others act independently as long transcripts.
The function of the vast majority of long ncRNAs is currently a mystery despite this recent progress. Indeed, doubts have been raised as to whether these remaining transcripts are functional at all (18). Certainly, long ncRNAs lack discernable features to facilitate categorization and functional prediction. And yet, there are several reasons to believe that many of these long ncRNAs are likely to be functional. First, their expression is often tissue- and/or cell-specific and localized to specific sub-cellular compartments (19–21), which suggests they are regulated and biologically significant. Second, as mentioned earlier, there are already numerous precedents of long ncRNAs having function, and the number of examples will continue to grow as research in this fledgling area continues. Finally, Willingham and colleagues (22) recently screened several hundred novel long ncRNAs for function in a limited battery of cell-based assays and successfully identified multiple functional ncRNAs, which highlights the untapped functional potential of these transcripts.
To begin to explore the function of the thousands of remaining novel long ncRNAs, we have recently undertaken a range of large-scale expression analyses of long ncRNAs. First, using in situ hybridization (ISH) data from the Allen Brain Atlas (ABA) (23), we identified >800 long ncRNAs that are expressed in the adult mouse brain, the majority of which were associated with specific anatomical regions, cell types or subcellular compartments (20). Second, we found that >900 long ncRNAs were expressed during mouse embryonic stem (ES) cell differentiation using a custom-designed oligonucleotide microarray, and subsequently showed that some of these ncRNAs appear to have a role in the epigenetic regulation of differentiation (21). Using the same custom array platform, we have also profiled the expression of several thousand long mouse ncRNAs during immune cell activation, neural stem cell differentiation, myoblast differentiation and gonadal ridge development. Finally, we have identified organ- and cell-specific expression data for large numbers of long ncRNAs from both human and mouse, using publicly available data from the Genomics Institute of the Novartis Research Foundation (GNF) (24).
In this report, we introduce the Noncoding RNA Expression Database (NRED). The database is available at http://jsm-research.imb.uq.edu.au/NRED, and its primary aim is to provide a specific resource for the expression of long ncRNAs. At this stage, NRED brings together each of the datasets described above, with more expected to follow in the near future. Short RNAs are already well-catered for by a range of other resources (25–27), and are not directly featured in this database. As well as providing detailed expression data, NRED enables researchers to characterize and select long ncRNAs based on various bioinformatic criteria, including predicted secondary structure, evolutionary conservation, and genomic context. In this way, NRED sheds light on a vast and largely unexplored territory of the mammalian transcriptome, and should stimulate and guide future functional studies of long ncRNAs.
DATABASE CONTENT
NRED currently features multiple datasets based on three different experimental platforms (Table 1), each of which is described subsequently.
Dataset . | Organism . | Number of noncoding probesa . |
---|---|---|
Custom noncoding microarray | Mouse | 4926 |
GNF SymAtlas | Human Mouse | 1287 5692 |
Allen Brain Atlas | Mouse | 1308 |
Dataset . | Organism . | Number of noncoding probesa . |
---|---|---|
Custom noncoding microarray | Mouse | 4926 |
GNF SymAtlas | Human Mouse | 1287 5692 |
Allen Brain Atlas | Mouse | 1308 |
aProbes that exclusively target ncRNAs were identified using a previously-described classification pipeline (20) (see Supplementary Materials), and numbers reflect the classification as at 24 September 2008.
Dataset . | Organism . | Number of noncoding probesa . |
---|---|---|
Custom noncoding microarray | Mouse | 4926 |
GNF SymAtlas | Human Mouse | 1287 5692 |
Allen Brain Atlas | Mouse | 1308 |
Dataset . | Organism . | Number of noncoding probesa . |
---|---|---|
Custom noncoding microarray | Mouse | 4926 |
GNF SymAtlas | Human Mouse | 1287 5692 |
Allen Brain Atlas | Mouse | 1308 |
aProbes that exclusively target ncRNAs were identified using a previously-described classification pipeline (20) (see Supplementary Materials), and numbers reflect the classification as at 24 September 2008.
Custom ncRNA microarray
We designed a custom microarray that contained probes uniquely targeting 9225 protein-coding transcripts and 4926 noncoding transcripts from mouse (Supplementary Material 1). The array was interrogated with RNA samples from a range of experimental systems (Supplementary Material 1). These included: (i) ES cell differentiation over a 16-day time course; (ii) macrophage activation in response to lipopolysaccharide; (iii) CD8+ T-cell differentiation and activation; (iv) neural stem cell (NSC) differentiation; (v) C2C12 myoblast differentiation; and (vi) testis and ovary development.
The results of our profiling experiments during ES cell differentiation have been recently reported (21), and demonstrate the utility of our custom microarrays in facilitating in-depth functional study of long ncRNAs. Across the six experimental systems currently featured in NRED, a total of 2913 ncRNAs were expressed above background levels (Supplementary Material 1). Of these, 1475 were differentially expressed in at least one setting (B-statistic >3).
GNF SymAtlas
The GNF previously compiled a large-scale atlas of mammalian gene expression using custom-designed whole-genome gene expression arrays (24). This resource utilized RNAs from 79 human and 61 mouse tissues, and featured the expression of 44 775 human and 36 182 mouse transcripts. We downloaded this publicly available dataset for further analysis (http://symatlas.gnf.org/). Although the probe set used by GNF was originally designed to target the protein-coding transcriptome, we found that 1287 human and 5692 mouse probes uniquely recognized long ncRNAs (Supplementary Material 2). Of these, 733 and 3403 were expressed in human and mouse, respectively.
Allen Brain Atlas
The ABA provides a comprehensive catalogue of gene expression within the adult mouse brain (23). Data were generated using automated high-throughput ISH techniques, and advanced image-based informatics methods enabled automated quantification and mapping of expression information. Through its web interface (http://www.brain-map.org), the atlas permits high-resolution visualization of the expression of ∼20 000 protein-coding transcripts and comprehensive data mining. We downloaded this publicly available dataset for further analysis, and discovered that the ABA also contains ISH data for 1308 ncRNAs (Supplementary Material 2). Of these, 849 are expressed in mouse brain, the majority of which are associated with specific neuroanatomical regions, cell types and/or subcellular compartments (20).
DATABASE ACCESS
Implementation
NRED is available at http://jsm-research.imb.uq.edu.au/NRED. Datasets are stored in relational form in a MySQL database. The web application is implemented in Perl 5, with rich client functionality provided via AJAX and other dynamic HTML procedures. Documentation is provided via jQuery, which allows the user to obtain help on almost any function by simply hovering the mouse on the relevant item on the website. Results tables can be sorted by a field in real-time by clicking on the column headings.
Query interface
NRED can be queried in various ways via the web interface (Figure 1).
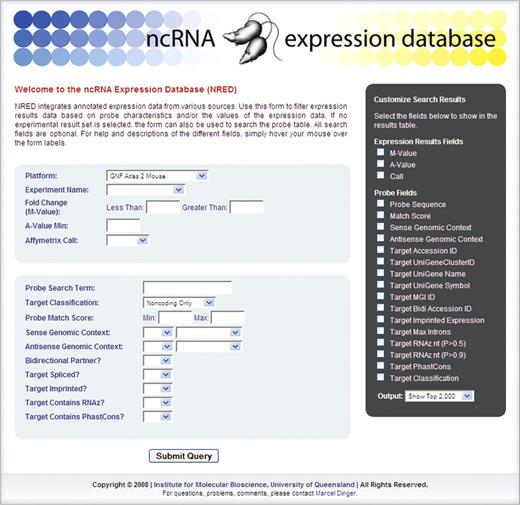
To examine the expression of individual ncRNAs, gene-centric searches can be performed across each of the experimental platforms using the ‘Probe Search Term’ field. For example, queries based on gene name (e.g. ‘Xist’, ‘Air’) or a unique gene identifier (e.g. Genbank accessions, MGI identifiers and UniGene Cluster identifier) can be used to readily display expression data for a given ncRNA of interest.
To identify ncRNAs that are expressed in a particular organ/region/cell type of interest or under particular conditions, an experimental platform must first be selected (e.g. ‘Allen Brain Atlas’). This brings up a series of platform-dependent menus, from which a user can then choose a relevant expression sub-system if desired (e.g. ‘Cerebellum’). Then, to restrict the query to those probes that exclusively recognize ncRNAs, one must specify ‘Noncoding only’ under the Target Classification menu, since the probes contained within the NRED datasets include those that recognize protein-coding transcripts as well.
The two basic query strategies described above—gene- and platform-centric searches —can be refined further by applying various filters. Expression-based filters permit searches to be modified based upon various statistics, such as significance thresholds (e.g. P-values, B-statistics, q-values), fold change (M-values) and expression intensity (e.g. A-values, Affymetrix Present/Absent calls). In this way, users can select their own criteria by which differentially expressed transcripts are identified. A series of other filters can also be applied based on information related to the probe target itself. For example, probes can be selected depending upon whether their targets are spliced or unspliced. Similarly, users can filter search results based on whether target ncRNAs show evidence of evolutionary conservation or predicted secondary structure using the PhastCons and RNAz tools, respectively (28,29) (Supplementary Material 3). In addition, we have previously developed a method for classifying the genomic context of target ncRNAs (20) (Supplementary Material 4). Using this information, probes can also be filtered depending on whether they map in a sense, cis-antisense and/or bi-directional orientation to other transcripts (including protein-coding transcripts, miRNAs, snoRNAs or other ncRNAs).
Data output
Query results are probe-centric, and can be customised to include any number of associated data fields using a simple format output menu (Figure 1). Thus, for any given probe, users can opt to display unique probe target identifiers (e.g. Genbank accession), selected expression data (e.g. B-statistics, M-values, etc.), overlapping sense and antisense transcript information, RNAz predictions and PhastCons data to name just a few.
Results can be displayed in several output formats. The default is to view the results as an online table, but users have the alternative option of obtaining information as a downloadable, tab-delimited text file. Finally, to enable users to use the search results in downstream applications [e.g. via the UCSC Genome Browser (30)], probe data can also be downloaded as individual.bed files.
FUTURE DIRECTIONS
We have recently designed and manufactured second-generation custom ncRNA microarrays. These new arrays will profile 12 000 and 16 000 ncRNAs in mouse and human, respectively. As expression results become available using this new platform, we will update NRED accordingly. Submission of other publicly available expression datasets that might be suitable for NRED is also invited, and should be sent to [email protected].
CITING NRED
To reference NRED, please cite this article. When referring to specific data from the database, the following format is suggested: ‘These data were retrieved from NRED, Institute for Molecular Bioscience, Brisbane, Australia (http://jsm-research.imb.uq.edu.au/NRED) [Date when you retrieved the data.]’.
FUNDING
National Health & Medical Research Council (to K.C.P.); the Foundation for Research, Science and Technology, New Zealand (to M.E.D.); the Australian Research Council, the Queensland State Government and the University of Queensland (to J.S.M.). Funding for open access charge: The University of Queensland.
Conflict of interest statement. None declared.
ACKNOWLEDGEMENTS
We thank Stephen Bruce, Evgeny Glazov, David Hume, Andrew Jackson, Peter Koopmam, Guangyu Li, Mark Mehler, George Muscat, Andrew Perkins and Kate Schroder for providing RNA samples for microarray analyses.
REFERENCES
Author notes
The authors wish it to be known that, in their opinion, the first two authors should be regards as joint First Authors.
Comments