-
PDF
- Split View
-
Views
-
Cite
Cite
Xuhua Xia, Huaichun Wang, Zheng Xie, Malisa Carullo, Huang Huang, Donal Hickey, Cytosine Usage Modulates the Correlation between CDS Length and CG Content in Prokaryotic Genomes, Molecular Biology and Evolution, Volume 23, Issue 7, July 2006, Pages 1450–1454, https://doi.org/10.1093/molbev/msl012
- Share Icon Share
Abstract
Previous studies have argued that, given the AT-rich nature of stop codons, the length and CG% of coding sequences (CDSs) should be positively correlated. This prediction is generally supported empirically by prokaryotic genomes. However, the correlation is weak for a number of species, with 4 species showing a negative correlation. Here we formulate a more general hypothesis incorporating selection against cytosine (C) usage to explain the lack of strong positive correlation between the length and GC% of CDSs. Two factors contribute to the selection against C usage in long CDSs. First, C is the least abundant nucleotide in the cell, and a long CDS should have fewer Cs to increase transcription efficiency. Second, C is prone to mutation to U/T and selection for increased reliability should reduce C usage in long CDSs. Empirical data from prokaryotic genomes lend strong support for this new hypothesis.
Introduction
The length and GC% of coding sequences (CDSs) are in most cases positively correlated in prokaryotic genomes (Oliver and Marin 1996; Xia et al. 2003). This positive correlation is attributed to the effect of mutation on the distribution and frequency of stop codons that are relatively AT-rich and should be encountered less frequently in GC-rich genomes. However, there are at least 4 bacterial genomes, Treponema pallidum subsp. pallidum str. Nichols (NC_000919), Tropheryma whipplei TW08/27 (NC_004551), T. whipplei str. Twist (NC_004572), and Ureaplasma parvum serovar 3 str. ATCC 700970 (NC_002162) that, instead of exhibiting the predicted positive correlation between the length and GC% of CDSs, have a negative correlation between the two (Xia et al. 2003). There are also many other species exhibiting a positive correlation that is not significantly different from zero. In addition, the positive correlation is mostly absent in eukaryotic genomes (Duret et al. 1995; Carels and Bernardi 2000; Xia et al. 2003; Wang et al. 2004). In short, although the effect of mutation on the distribution and frequency of stop codons can account for a significant proportion of variation in prokaryotic CDS length, the proportion is small and other explanatory factors need to be sought. In this paper, we propose a more general hypothesis on factors contributing to the variation of CDS lengths and provide empirical substantiation of the hypothesis.
Two factors may modulate the correlation between the length and the GC% of the CDSs, both invoking selection against cytosine (C) usage. The first is based on the observation of generally low intracellular C availability in both eukaryotes and prokaryotes. For example, in the exponentially proliferating chick embryo fibroblasts in culture, the concentrations of adenosine triphosphate, cytidine triphosphate (CTP), guanosine triphosphate, and uridine triphosphate, in the unit of (moles × 10−12 per 106 cells), are 1890, 53, 190, and 130, respectively, in 2-hour culture and 2390, 73, 220, and 180, respectively, in 12-hour culture (Colby and Edlin 1970). The protozoan parasite, Trypanosoma brucei, exemplifies C-limitation in mammalian blood. The parasite maintains its de novo synthesis pathway for CTP and inhibiting its CTP synthetase effectively eradicates the parasite population in the host (Hofer et al. 2001). This suggests that little CTP can be salvaged from the host. In contrast, the parasite does not have de novo synthesis pathways for purines, suggesting that the parasite can obtain the purines by its salvage pathway. C-limitation appears to be a general feature in bacterial species, and a biochemical explanation has been offered to explain the general C-limitation in bacterial species (Rocha and Danchin 2002). Thus, a long CDS with many Cs may take inordinately long to be transcribed and should therefore be selected against. The selection against C usage (and consequently against GC%) in long CDSs naturally will result in long CDSs to become less C-rich (and consequently less CG-rich), leading to a decrease in the correlation between the length and GC% of CDSs.
Designate AC as an index measuring the intensity of selection against C usage in a bacterial genome (where the letter “A” confer the meaning of “against”) and RL,GC as the correlation between the length and GC% of CDSs within a genome. The reasoning in the previous paragraph involving selection against C usage would lead to the prediction that a genome with a large AC should have a small RL,GC (or a genome with a small AC should have a large RL,GC). In short, AC and RL,GC should be negatively correlated across genomes. This can explain why the 4 genomes listed in the previous paragraph do not have a positive RL,GC—they may have a large AC.
The second factor that may contribute to a negative RL,GC is derived from the observation of a much higher spontaneous deamination rate of C to U or methylated C to T than other chemical processes leading to the decay of DNA and RNA (Sancar A and Sancar GB 1988; Frederico et al. 1990; Frederico et al. 1993; Lindahl 1993). The single-stranded mRNA is particularly prone to mutations caused by the spontaneous deamination because the deamination rate is about 100 times higher in single-stranded nucleotide sequences than in double-stranded ones (Frederico et al. 1990). We here present a simple model to link the high deamination rate to the correlation between the length and GC% of the CDSs, by following the same logic as the late Maynard Smith (1998) in linking the mutation rate and the genome length.
The 2 factors above, that is, the limited availability of C and the high deamination rate involving C may both contribute to selection against C usage in long CDSs and consequently decrease RL,GC. We may therefore hypothesize that, if we can devise an index, say AC, measuring the selection against C usage, we expect this index to be negatively correlated with RL,GC. This hypothesis will henceforth be referred to as the hypothesis of C-minimization in long CDSs, or C-minimization hypothesis for short.
We can now predict a negative correlation between AC (i.e., selection against C usage) and RL,GC. In other words, a genome with a small AC should have a large and positive RL,GC, but a genome with a large AC should have a small RL,GC. The prediction gained a hint of truth when our preliminary study showed that the 4 genomes mentioned above with a negative RL,GC happen to all have positive AC values. It is interesting to examine the generality of the prediction by studying all complete prokaryotic genomic sequences.
Materials and Methods
All 281 prokaryotic genomes as of 16 Novemeber 2005 were retrieved from http://www.ncbi.nlm.nih.gov/genomes/lproks.cgi. CDSs were extracted by using DAMBE (Xia 2001; Xia and Xie 2001). AC and RL,GC were computed for each genome to test the prediction that genomes with a small AC should have a large RL,GC and vice versa.
We examine the relationship between RL,GC and AC in 2 ways. First, all 281 pairs of (RL,GC, AC) values from the 281 complete prokaryotic genomes were studied for a negative correlation without reference to their phylogenetic relationships, that is, there were treated as independent data points. This is not a statistically rigorous approach because of the shared ancestry among prokaryotic lineages that violates the assumption of data independence (Felsenstein 1985, 1988). A conceptually more appropriate approach is to construct a phylogenetic tree of all these genomes and perform a phylogeny-based comparative analysis (Felsenstein 1985, 1988) that we have used in analyzing the relationship between genome size and temperature (Xia 1995), in tracing transitions and transversions along branches in a phylogenetic tree (Xia et al. 1996), and in relating the length and GC% of CDSs in a small set of species (Xia et al. 2003). However, there are practical difficulties in using the method for analyzing the diverse array of prokaryotic species in this study. The rRNA sequences do poorly as universal evolutionary yardsticks, often with sequence differences between strains of the same species much greater than that between different species. Take the 16S rRNA sequences from the 2 Thermus thermophilus strains (HB27 and HB8) for example. The p-distance and the genetic distance based on the TN93 model (Tamura and Nei 1993) between the 2 T. thermophilus strains are 0.523 and 0.921, respectively, whereas the corresponding distances between T. thermophilus HB27 and the phylogenetic more remote Thermotoga maritime are only 0.178 and 0.205, respectively. This is not due to sequencing error. The 2 T. thermophilus strains each contain 2 copies of 16S rRNA, and the 2 copies are identical within each strain. No phylogenetic methods could possibly group the 2 Thermus thermophilus strains together as a monophyletic group. There are other less extreme but also troubling cases, making the phylogeny-based comparative method difficult to apply. An alternative is to use universally shared protein-coding sequences such as RNA polymerase or concatenated ribosomal proteins (Wolf et al. 2001), but similar problems exist. A third alternative is to Blast (Altschul et al. 1990, 1997) genes in each genome against all other genomes and use the gene sharing as an index to build a tree (Wolf et al. 2001; Henz et al. 2005). However, gene sharing changes substantially with the Blast cutoff E value, for example, changing the E value from 0.01 to 0.001 leads to substantially different phylogenies. In addition, this approach would incorporate the information from genes that must have undergone extensive horizontal gene transfer, for example, only one of the 7 genes in the urease gene cluster in Helicobacter pylori is shared with its close relatives such as Campylobacter jejuni, but all 7 are shared with several remotely related gram-positive bacterial species. Such results weaken our confidence in taking this Blast approach in building genomic trees. For these reasons, we limit phylogeny-based comparisons within species known to be closely related.
The large number of genomes available, however, does allow a statistically sound alternative method. The 281 genomes span many major taxonomic groups (table 1) whose phylogenetic relationships are poor predictors of AC, GC%, or CDS length. Thus, these 3 variables may be considered independent of their phylogenetic affiliation at this particular taxonomic level. We can study the correlation between RL,GC and AC within each taxonomic group. A negative correlation between RL,GC and AC in one taxonomic group contributes one point in favor of the C-minimization hypothesis, and a positive correlation between the 2 from a taxonomic group contribute one data point against the C-minimization hypothesis. When RL,GC and AC are not related (which is the null hypothesis), then the number of positive and negative correlations between the 2 from those major taxonomic groups should be equal to each other. Our alternative hypothesis is that there should be significantly more negative correlations than positive correlations.
Genomes with Strong Selection against C Usage (a large AC) Are Associated with a Low Correlation between the Length and GC% of CDSs (RL,GC), Leading to a Consistently Negative Correlation between the 2 (third column)
Taxon . | N . | r(RL,GC, Ac) . | Pa . |
---|---|---|---|
Archaea | 24 | −0.3501 | 0.0468 |
Actinobacteria | 20 | −0.7182 | 0.0002 |
Alphaproteobacteria | 29 | −0.4424 | 0.0082 |
Betaproteobacteria | 17 | −0.0212 | 0.4678 |
Chlamydiae/Verrucomicrobia | 10 | −0.9288 | 0.0001 |
Cyanobacteria | 15 | −0.5440 | 0.0180 |
FirmicutesAb | 14 | −0.1994 | 0.2472 |
FirmicutesBc | 53 | −0.4171 | 0.0010 |
Gammaproteobacteria | 63 | −0.2259 | 0.0376 |
Delta/Epsilonproteobacteria | 14 | −0.6359 | 0.0073 |
Mixedd | 22 | −0.2050 | 0.1801 |
Taxon . | N . | r(RL,GC, Ac) . | Pa . |
---|---|---|---|
Archaea | 24 | −0.3501 | 0.0468 |
Actinobacteria | 20 | −0.7182 | 0.0002 |
Alphaproteobacteria | 29 | −0.4424 | 0.0082 |
Betaproteobacteria | 17 | −0.0212 | 0.4678 |
Chlamydiae/Verrucomicrobia | 10 | −0.9288 | 0.0001 |
Cyanobacteria | 15 | −0.5440 | 0.0180 |
FirmicutesAb | 14 | −0.1994 | 0.2472 |
FirmicutesBc | 53 | −0.4171 | 0.0010 |
Gammaproteobacteria | 63 | −0.2259 | 0.0376 |
Delta/Epsilonproteobacteria | 14 | −0.6359 | 0.0073 |
Mixedd | 22 | −0.2050 | 0.1801 |
One-tailed test.
Wall-less Mollicutes.
All Firmicutes excluding wall-less Mollicutes.
Including Bacteroidetes (7), Spirochaetes (6), Thermotogae (1), Aquificae (1), Chloroflexi (2), Planctomycetes (1), Deinococcus–Thermus (3), and Fusobacteria (1), where the number within parenthesis indicates the number of genomes.
Genomes with Strong Selection against C Usage (a large AC) Are Associated with a Low Correlation between the Length and GC% of CDSs (RL,GC), Leading to a Consistently Negative Correlation between the 2 (third column)
Taxon . | N . | r(RL,GC, Ac) . | Pa . |
---|---|---|---|
Archaea | 24 | −0.3501 | 0.0468 |
Actinobacteria | 20 | −0.7182 | 0.0002 |
Alphaproteobacteria | 29 | −0.4424 | 0.0082 |
Betaproteobacteria | 17 | −0.0212 | 0.4678 |
Chlamydiae/Verrucomicrobia | 10 | −0.9288 | 0.0001 |
Cyanobacteria | 15 | −0.5440 | 0.0180 |
FirmicutesAb | 14 | −0.1994 | 0.2472 |
FirmicutesBc | 53 | −0.4171 | 0.0010 |
Gammaproteobacteria | 63 | −0.2259 | 0.0376 |
Delta/Epsilonproteobacteria | 14 | −0.6359 | 0.0073 |
Mixedd | 22 | −0.2050 | 0.1801 |
Taxon . | N . | r(RL,GC, Ac) . | Pa . |
---|---|---|---|
Archaea | 24 | −0.3501 | 0.0468 |
Actinobacteria | 20 | −0.7182 | 0.0002 |
Alphaproteobacteria | 29 | −0.4424 | 0.0082 |
Betaproteobacteria | 17 | −0.0212 | 0.4678 |
Chlamydiae/Verrucomicrobia | 10 | −0.9288 | 0.0001 |
Cyanobacteria | 15 | −0.5440 | 0.0180 |
FirmicutesAb | 14 | −0.1994 | 0.2472 |
FirmicutesBc | 53 | −0.4171 | 0.0010 |
Gammaproteobacteria | 63 | −0.2259 | 0.0376 |
Delta/Epsilonproteobacteria | 14 | −0.6359 | 0.0073 |
Mixedd | 22 | −0.2050 | 0.1801 |
One-tailed test.
Wall-less Mollicutes.
All Firmicutes excluding wall-less Mollicutes.
Including Bacteroidetes (7), Spirochaetes (6), Thermotogae (1), Aquificae (1), Chloroflexi (2), Planctomycetes (1), Deinococcus–Thermus (3), and Fusobacteria (1), where the number within parenthesis indicates the number of genomes.
Results and Discussion
The Pearson correlation between AC and RL,GC is −0.21143 for all 281 genomes (P = 0.0004). This is consistent with our prediction that genomes with a large AC should have a small RL,GC, that is, a negative correlation between the 2. However, as we have mentioned earlier in the Materials and Methods section, such a correlation, computed from all 281 pairs of AC and RL,GC values, suffers from the problem of nonindependence, and the P value consequently cannot be interpreted in a strict probabilistic sense.
Given the difficulty in performing a phylogeny-based comparative analysis (see the Materials and Methods section for details), we have adopted a conservative alternative by computing the correlation between AC and RL,GC separately for each of the taxonomic groups in table 1. The grouping follows that of National Center for Biotechnology Information (NCBI)'s microbial genome page at http://www.ncbi.nlm.nih.gov/genomes/lproks.cgi, except for the following 2 treatments. First, the wall-less Mollicutes within Firmicutes are analyzed separately from the rest of Firmicutes. Second, the deltaproteobacteria and epsilonproteobacteria each contain only 7 genomes and are merged because they belong to the same delta/epsilon division according to NCBI's Taxonomic browser at http://www.ncbi.nlm.nih.gov/Taxonomy. All other taxonomic groups with 7 or fewer genomes were all lumped into the “mixed” group that is taxonomically heterogeneous (table 1).
The 10 genomes in the Chlamydiae/Verrucomicrobia group exhibit the highest negative correlation between AC and RL,GC, with the Pearson r being −0.9288 (P = 0.0001, fig. 1 and table 1). Other taxonomic groups (table 1) also provide consistent support for the C-minimization hypothesis, with the correlation between AC and RL,GC being consistently negative.
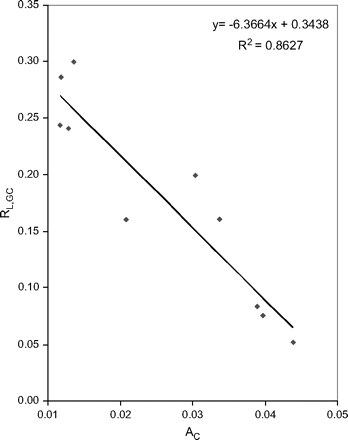
Negative relationship between AC (selection against C usage) and RL,GC (correlation between the length and GC% of CDSs) in 10 Chlamydiae/Verrucomicrobia genomes.
As a more conservative test, we take one negative correlation between RL,GC and AC in one taxonomic group as only one data point in favor of the C-minimization hypothesis and one positive correlation between RL,GC and AC in one taxonomic group as one data point against the C-minimization hypothesis. The null hypothesis of r between RL,GC and AC equal to 0 can be conclusively rejected by the 11 consistently negative r values (mean r = −0.4262, standard error = 0.08014, t = 5.3183, df = 10, P = 0.00034, 2-tailed test). One can also express the expectation of the null hypothesis of r equal to 0 as having equal number of r(RL,GC, AC) values above or below zero, that is, the probability of having an r(RL,GC, AC) value above zero is 0.5. This expectation can also be conclusively rejected because P = 0.511 = 0.00049.
It may also be worth noting that, if we separate the last mixed group (table 1) into 1) the Bacteroidetes with 7 genomes, 2) Spirochaetes with 6 genomes, and 3) a mixed group with 3 Deinococcus–Thermus genomes, 2 Chloroflexi genomes, one Thermotogae genome, one Aquificae genome, one Planctomycetes genome and one Fusobacteria genome, then the correlation between AC and RL,GC is negative in each of the 3 groups, therefore, providing 3 data points in favor of the C-minimization hypothesis.
A more critical test of the C-minimization can be carried out with reference to the 4 bacterial genomes with a negative RL,GC: T. pallidum (NC_000919), T. whipplei (NC_004551), T. whipplei (NC_004572), and U. parvum (NC_002162). If the C-minimization hypothesis is correct and powerful, then we should expect these species to have relatively high AC values. Treponema pallidum has its RL,GC = −0.0976 and AC = 0.0898. Its sister species, Treponema denticola (NC_002967) has its RL,GC = 0.2350 and AC = 0.0766. This is consistent with the C-minimization hypothesis. Tropheryma whipplei (NC_004551) and T. whipplei (NC_004572) are the only 2 genomes within Actinobacteria that exhibit a negative RL,GC (equal to −0.0927 and −0.0745, respectively). They also have the largest AC values within Actinobacteria (equal to 0.0740 and 0.0781, respectively). The mean AC value within Actinobacteria is only 0.0029. Ureaplasma parvum belongs to the wall-less Mollicutes (labeled as FirmicutesA in table 1) and is the only species within the group that has a negative RL,GC (= −0.0355), and it also has the largest AC value (=0.1027) within the group. Such comparisons with local phylogenetic information nicely illustrate the excellent predictive power of the C-minimization hypothesis.
In short, our comparative genomic analysis substantiates the C-minimization hypothesis that explains why some bacterial genomes do not show a positive correlation between the length and GC% of CDSs. We have provided 2 plausible factors that would contribute to selection against C usage in long CDSs, that is, the limited availability of C in cells and the high C → U mutations mediated by spontaneous deamination. In general, when there are such factors contributing to selection against C usage in long CDSs, the originally predicted positive correlation between the length and CG% of CDSs (Oliver and Marin 1996; Xia et al. 2003) is reduced and may even become negative.
Takashi Gojobori, Associate Editor
This study is supported by grants from the Natural Sciences and Engineering Research Council's Discovery, Research Tools and Instruments, and Strategic Grants to X.X. We thank S. Aris-Brousou, E. Betran, P. Higgs, A. Morin, and E. Rocha for their insightful comments and suggestions.
References
Altschul SF, Gish W, Miller W, Myers EW, Lipman DJ.
Altschul SF, Madden TL, Schaffer AA, Zhang J, Zhang Z, Miller W, Lipman DJ.
Colby C, Edlin G.
Duret L, Mouchiroud D, Gautier C.
Frederico LA, Kunkel TA, Shaw BR.
Frederico LA, Kunkel TA, Shaw BR.
Henz SR, Huson DH, Auch AF, Nieselt-Struwe K, Schuster SC.
Hofer A, Steverding D, Chabes A, Brun R, Thelander L.
Lobry JR.
Oliver JL, Marin A.
Rocha EP, Danchin A.
Tamura K, Nei M.
Wang HC, Singer GA, Hickey DA.
Wolf YI, Rogozin IB, Grishin NV, Tatusov RL, Koonin EV.
Xia X.
Xia X.
Xia X, Hafner MS, Sudman PD.
Xia X, Xie Z.
Author notes
*Department of Biology, University of Ottawa, Ottawa, Ontario, Canada; †Center for Advanced Research in Environmental Genomics, University of Ottawa, Ottawa, Ontario, Canada; ‡Department of Mathematics and Statistics, Dalhousie University, Halifax, Nova Scotia; and §Biology Department, Concordia University, Montreal, Quebec, Canada