-
PDF
- Split View
-
Views
-
Cite
Cite
Tilman Brück, Michele Di Maio, Sami H Miaari, Learning The Hard Way: The Effect of Violent Conflict on Student Academic Achievement, Journal of the European Economic Association, Volume 17, Issue 5, October 2019, Pages 1502–1537, https://doi.org/10.1093/jeea/jvy051
- Share Icon Share
Abstract
We study the effect of the Israeli–Palestinian conflict on various education outcomes for Palestinian high school students in the West Bank during the Second Intifada (2000–2006). Exploiting within-school variation in the number of conflict-related Palestinian fatalities during the academic year, we show that the conflict reduces the probability of passing the final exam, the total test score, and the probability of being admitted to university. The effect of conflict varies with the type and the timing of the violent events the student is exposed to and it is not significant for students in the upper tail of the test score distribution. We discuss various possible transmission mechanisms explaining our main result. Evidence suggests a role for both the conflict-induced deterioration of school infrastructures and the worsening in the student's psychological well-being due to direct exposure to violent events.
1. Introduction
Differences in academic achievement play a crucial role in explaining individual and cross-country differences in earnings and economic well-being (Card 1999; Hanushek and Woessmann 2011, Chap. 2). Individual academic achievement can be influenced by several factors such as personal, household, and school characteristics, as well as local and national socioeconomic conditions (Glewwe and Kremer 2006, Chap. 16). In this paper, we investigate one potentially important but little studied determinant of academic achievement, namely, living in and attending school in a locality affected by violent conflict.
Recent research on the microlevel effects of violent conflict provides evidence of the negative impact of the latter on education as measured by different outcomes, such as school enrolment, school attendance, and school attainment (UNESCO 2011a; Justino 2012, Chap. 13; Leon 2012; Buvinić et al. 2014). Yet, there are still significant knowledge gaps concerning both the effects of conflict on academic achievement and the mechanisms underlying such possible effects, in particular for high school students. Answering these questions is important because academic achievement is a predictor of future earnings, especially when admission to university is determined by high school final exam performance. Moreover, learning about the effects of conflict on high school students is crucial for a better understanding of the impact of violent conflict on the development prospects of an economy, given that these students will make up a large part of the country's high-skilled workforce in the future.
Despite the relevance of these questions, there are no studies on the effects of violent conflict on academic achievement of high school students. The main reason for that is a dearth of data. First, violent conflicts often occur in developing countries where collecting data on education and, in particular, on academic achievement is very difficult. The conflict situation usually exacerbates these difficulties. Second, the identification of the effect of conflict on academic achievement requires detailed data to measure conflict intensity. These are rarely available.
The characteristics of our two main data sets allow us to overcome the limitations of the literature. The first data set contains unique administrative data on individual test scores for the final high-school exam (Tawjihi General Examination) of the whole population of students enrolled in the Literary (Adabi) and the Scientific (Elmi) stream in high schools in the West Bank for all academic years of the Second Intifada (2000–2006). The Tawjihi General Examination is a crucial exam for young Palestinians. Completing high school education and getting a high test score at the final exam are expected to have a positive impact on the future earnings of the student, because these determine eligibility for admission to university in the West Bank and abroad, as well as for applying for well-paid public sector jobs. The second data set provides detailed information on all conflict-related Palestinian fatalities during the Second Intifada. These include the age, gender, and nationality of the victims, the date and locality of the events, as well as a brief description of the circumstances of the events. This comprehensive conflict data set allows us to construct an accurate measure of conflict intensity at the locality level for each academic year, as well as for different time periods, types of victims and type of conflict events.
Taking advantage of these unique data, this paper provides the first empirical analysis of the effects of a violent conflict on academic achievement for high school students. Specifically, we study the effect of the Israeli–Palestinian conflict on individual results at the Tawjihi General Examination for the population of high school students in the West Bank during the Second Intifada, a period of intense violence between Israelis and Palestinians.1 The West Bank during the Second Intifada provides a very suitable context for the analysis of the effects of violent conflict on academic achievement of high school students for various reasons. First, high school enrolment in the West Bank has always been high by international standards (UNESCO 2007). This provides us with a large population to analyze. Second, the functioning of the Palestinian education system was never interrupted during the Second Intifada, in contrast with many countries affected by extremely violent conflict. Third, the Second Intifada is a conflict characterized by meaningful temporal and geographical variation in violence intensity.
Our empirical analysis proceeds in two steps. First, we estimate the effect of conflict on individual academic achievement. We use spatial and time variation in conflict intensity—as measured by the locality-level number of all Palestinians killed by Israeli Defense Forces (IDF) during the academic year—to identify the effect of the Israeli–Palestinian conflict on individual exam results. By exploiting the within-school variation in the number of fatalities over time, we find that conflict reduces the probability of passing the exam, the total test score at the exam and the probability of achieving the minimum test score needed for admission to university. We provide evidence that these results are not due to sample selection, omitted variable bias, reverse causality, or migration choices. Moreover, we show that these results are robust to a time placebo test, to different samples and econometric models, and to the inclusion of controls for the level of internal mobility restrictions imposed by the IDF. We also document that the effect of conflict intensity is not significant for students in the upper tail of the test score distribution.
Second, we look for possible transmission mechanisms explaining why the conflict has a negative effect on academic achievement. As a first mechanism, we show that conflict negatively affects the quality of the learning environment at school. In particular, conflict increases student density in the classroom and this in turn correlates with a lower probability of passing the final exam. The second mechanism we explore is the worsening in student’s psychological well-being due to exposure to conflict-related violence. Direct exposure to conflict—as proxied by the per-capita number of fatalities in the locality—has a large negative impact on the probability of passing the exam, which effect is largest for conflict events occurring shortly before the exam date. Finally, we look at alternative possible mechanisms such as conflict-induced reduction in the number of school days, student and teacher absenteeism, reduction in quality of teaching and parental distress. Results indicate that conflict intensity seems to be associated with more school closures and more student and teacher absenteeism. Yet, due to severe data limitations on these variables, we cautiously interpret the overall empirical evidence on these mechanisms as being inconclusive.
Our paper builds upon the literature on the microeconomic effects of violent conflicts on education (see Blattman and Miguel 2010; Justino 2012, Chap. 13 (Verwimp et al. 2018) for reviews of the literature). The existing empirical evidence indicates that the effects of violent conflict on education are highly heterogeneous. The sign and magnitude of the effects depend, among other elements, on the characteristics of the country and of the violent events, on the age and gender of the student, as well as on the education outcome considered (see Grogger 1997; Blattman and Annan 2010; Chamarbagwala and Morán 2010; de Groot and Goksel 2011; Shemyakina 2011; Leon 2012; Rodriguez and Sanchez 2012; Di Maio and Nandi 2013; Valente 2013; Akbulut-Yuksel 2014; Justino et al. 2014; Swee 2015; Gershenson and Tekin 2018; Monterio and Rocha 2017; Michaelsen and Salardi 2018; Bertoni et al. forthcoming).
Our paper contributes to the existing literature in three ways. First, while the majority of previous studies look at the effect of conflict on the quantity of education (as measured by education enrolment, attendance or attainment), we estimate the impact of conflict on the quality of education (as measured by test score results at the school-leaving examination). Second, we provide novel evidence of the heterogeneous effect of conflict at the individual level. Our unique data allow us to explore aspects that the previous studies, using school-level observations as the unit of analysis, could not consider. In particular, we document that conflict has a heterogeneous effect along the test score distribution and on the type and timing of the conflict events the student is exposed to. Finally, we are able to explore various possible mechanisms. In particular, the finding that conflict reduces academic achievement, because it worsens the learning and teaching environment conditions by damaging school and classroom infrastructures, is new to the literature, and has important policy implications, especially in post-conflict situations. Moreover, to the best of our knowledge, our analysis of the psychological mechanism, and the suggestive evidence we provide supporting it, is also new to the literature.
The paper proceeds as follows. The next section provides some background on the Israeli–Palestinian conflict and the Second Intifada, as well as on the main characteristics of the Palestinian education system and of the Tawjihi General Examination. Section 3 describes the data. Section 4 discusses the econometric model and the identification strategy. Section 5 presents the regression analysis and the results. Section 6 discusses the possible transmission mechanisms. Section 7 concludes.
2. Background
As a result of the Six-Day War in 1967, the West Bank and the Gaza Strip were occupied by Israel. In 1993, the Oslo Accords created the Palestinian National Authority giving Palestinians control over some administrative functions (e.g., education, health, and taxation) in both the West Bank and the Gaza Strip (henceforth the Occupied Palestinian Territories (OPT)) for the first time. Israel retained control over the strategic issues of security, foreign trade, and border controls.
The Second Intifada (also known as the Al-Aqsa Intifada) started in September 2000, ending the relatively peaceful period that followed the Oslo Accords. The Second Intifada was a violent revolt against the Israeli occupation of the OPT characterized by frequent clashes between Palestinians and the IDF.2 During this period, numerous violent acts were perpetrated by both the Palestinians and the IDF, including the killing of civilians and Palestinian militants in the OPT, Palestinian suicide attacks in Israel, assassinations of Palestinians leaders in the OPT, and the demolition of Palestinian houses by the IDF.
In terms of number of fatalities, the conflict was highly asymmetric. Between 2000 and 2006, Palestinians killed 703 Israeli civilians and 316 IDF soldiers, whereas the IDF killed more than four thousand Palestinians (B’Tselem 2007). Although the intensity of violence varied over time and space, the conflict persisted throughout the entire period. While there is no official end date for the Second Intifada, violence decreased substantially after 2006. The results of the 2006 Palestinian legislative elections caused a de facto division of the OPT into a Fatah-controlled West Bank and a Hamas-controlled Gaza Strip. Starting from January 2007, Israel imposed a complete blockade on the Gaza Strip as a sanction against Hamas. After that, the West Bank and the Gaza Strip started to diverge in economic and political terms (Etkes and Zimring 2015).
Since the Oslo Accords, the education system in the OPT has been managed by the Palestinian Ministry of Education and Higher Education (MOEHE). The Palestinian education system includes government schools, private schools, and UNRWA (United Nations Relief and Works Agency for Palestine Refugees in the Near East) schools. In the West Bank, education is compulsory for ten years starting from age six.3
The secondary education cycle consists of either a vocational or an academic track and includes grades 11 and 12. High school students in the academic track can choose between two streams: Adabi (arts) and Elmi (scientific).4 Government schools and private schools offer high school grades for both streams whereas UNRWA schools do not provide services beyond grade 10. There are no differences in the requirements for entering the two streams. The geographical distribution of the schools offering each stream is homogenous (World Bank 2011) and the fee to be paid is the same.5
At the end of these two years, all students take a final exam called the Tawjihi General Examination. The exam takes place on the same day (at the end of the academic year around the end of June) in all schools in the West Bank under exactly the same conditions. An external commission nominated by the MOEHE grades the exam. The exam is different for the Adabi and Elmi streams in terms of both subjects and for the weight given to each subject in the total test score (see Online Appendix B for details). The maximum test score in both streams is 1000. To pass the final exam, the student has to get more than 50% of the score in all subjects included in the test. Students who do not pass the exam can retake it the next year.
The Tawjihi Certificate is equivalent to a high school completion certificate and is required for students who wish to pursue undergraduate studies. The exam test score determines the likelihood of being accepted by a university in the OPT or abroad and which majors the student can enter. In the West Bank, the minimum score at the Tawjihi General Examination required for admission to university is 650, although some specific majors have higher minimum test scores for admission. For instance, the minimum test score for admission to engineering is 850. Similarly, the minimum test score required for the admission as a foreign student to most universities in other countries in the Middle East is 850.
Education is given much importance in the West Bank (Nicolai 2007). The number of enrolled students in all grades has been constantly increasing in the OPT, at least since the creation of the national curriculum following the 1994 Oslo Accords. This resulted in enrolment and attendance rates, and number of years of schooling, that are high by regional standards (UNESCO 2007). According to data by the Palestinian Central Bureau of Statistics (PCBS various years), the percentage of students enrolled in high school during the Second Intifada was between 72% and 83%,6 a very high level for a developing country.7 The high value placed on schooling also extends to the education of girls. In fact, the female participation rate in secondary education is slightly higher than male one (Di Maio and Nisticò 2018).
High school education has several perceived benefits in Palestinian society (World Bank 2006). Completing high school education and receiving a high test score at the final exam are important for at least three reasons. First, completing high school is the necessary step to access university. This is an attractive choice since university education is practically free of charge (EACEA 2012) and private rates of return for university graduates during the Second Intifada were higher than for any other education level (Al-Kafri 2004; World Bank 2006; Tansel and Daoud 2011). Second, higher education is a requirement to access most of the public sector jobs in the OPT. Wages in the public sector are higher than in the private sector and public sector workers receive important work-related benefits and privileges (ETF 2014; Miaari 2018). Moreover, while the private sector has been dramatically affected by the conflict (Amodio and Di Maio forthcoming), this was much less so for the employment and wages in the public sector (Miaari 2009; Cali and Miaari 2018). Third, obtaining the Tawjihi Certificate with a high total test score is a necessary condition to access any university abroad. This opportunity, although being an important career opportunity for many students, is particularly attractive to those living in a conflict-affected country because it would allow moving to a safer environment. In summary, students completing high school education and receiving high test scores are expected to have higher future earnings.
3. Data
Our analysis combines four data sets. The first data set includes unique information on individual test scores in all subjects at the high-school final exam (Tawjihi General Examination) for the whole population of Palestinian students in the West Bank for all the academic years 2000–2006. These data are provided by MOEHE. There are 138,135 students included in our analysis. Our main variable of interest is Pass exam, a dummy that takes value 1 if the student passes the final exam and 0 otherwise. As shown in Table 1, 74% of the students have passed the final exam. The average total test score at the exam is 640. The data set also includes information on age, gender, religion, and stream of study (Adabi or Elmi) of the student as well as the identification number and locality8 of the school the student is enrolled in. Summary statistics are reported in Table 1. The average age is 18.3 with 95% of the students being between 17 and 19 years old. The majority of the student population is female (55%). More than 97% of the students are Muslims and 2% are Christians. Students enrolled in the Adabi stream comprise 73% of the total.
. | Observations . | Mean . | Std. Dev. . | Min . | Max . |
---|---|---|---|---|---|
Pass exam | 138,135 | 0.74 | 0.4 | 0 | 1 |
Total test score | 138,135 | 640.51 | 213.2 | 0 | 997 |
Age | 138,135 | 18.32 | 0.6 | 17 | 28 |
Male | 138,135 | 0.45 | 0.9 | 0 | 1 |
Adabi | 138,135 | 0.73 | 0.4 | 0 | 1 |
Christian | 138,135 | 0.02 | 0.1 | 0 | 1 |
Number of Fatalities (locality-year level) | 1398 | 7.71 | 17.1 | 0 | 105 |
. | Observations . | Mean . | Std. Dev. . | Min . | Max . |
---|---|---|---|---|---|
Pass exam | 138,135 | 0.74 | 0.4 | 0 | 1 |
Total test score | 138,135 | 640.51 | 213.2 | 0 | 997 |
Age | 138,135 | 18.32 | 0.6 | 17 | 28 |
Male | 138,135 | 0.45 | 0.9 | 0 | 1 |
Adabi | 138,135 | 0.73 | 0.4 | 0 | 1 |
Christian | 138,135 | 0.02 | 0.1 | 0 | 1 |
Number of Fatalities (locality-year level) | 1398 | 7.71 | 17.1 | 0 | 105 |
Notes: Pass exam is a dummy that takes value 1 if the student has passed the final exam (i.e., the student gets more than 50% of the scores in each subject at the exam) and 0 otherwise. Total test score is the standardized total test score at the exam. Number of fatalities is the locality-level number of conflict-related Palestinian fatalities caused by the IDF during the academic year.
. | Observations . | Mean . | Std. Dev. . | Min . | Max . |
---|---|---|---|---|---|
Pass exam | 138,135 | 0.74 | 0.4 | 0 | 1 |
Total test score | 138,135 | 640.51 | 213.2 | 0 | 997 |
Age | 138,135 | 18.32 | 0.6 | 17 | 28 |
Male | 138,135 | 0.45 | 0.9 | 0 | 1 |
Adabi | 138,135 | 0.73 | 0.4 | 0 | 1 |
Christian | 138,135 | 0.02 | 0.1 | 0 | 1 |
Number of Fatalities (locality-year level) | 1398 | 7.71 | 17.1 | 0 | 105 |
. | Observations . | Mean . | Std. Dev. . | Min . | Max . |
---|---|---|---|---|---|
Pass exam | 138,135 | 0.74 | 0.4 | 0 | 1 |
Total test score | 138,135 | 640.51 | 213.2 | 0 | 997 |
Age | 138,135 | 18.32 | 0.6 | 17 | 28 |
Male | 138,135 | 0.45 | 0.9 | 0 | 1 |
Adabi | 138,135 | 0.73 | 0.4 | 0 | 1 |
Christian | 138,135 | 0.02 | 0.1 | 0 | 1 |
Number of Fatalities (locality-year level) | 1398 | 7.71 | 17.1 | 0 | 105 |
Notes: Pass exam is a dummy that takes value 1 if the student has passed the final exam (i.e., the student gets more than 50% of the scores in each subject at the exam) and 0 otherwise. Total test score is the standardized total test score at the exam. Number of fatalities is the locality-level number of conflict-related Palestinian fatalities caused by the IDF during the academic year.
The second data set—also provided by the MOEHE—includes information on the characteristics of the 555 high schools in the West Bank, including the locality of the school, the type of stream offered (Adabi/Elmi) and the supervising authority (government/private). As for the stream of study, 274 schools offer both the Adabi and Elmi streams, 260 only the Adabi stream, and 21 only the Elmi one. 9% of schools are private. The data set also reports the following characteristics for all schools: Student/Teacher Ratio, Average Size of the Classrooms, Average Density in the Classroom, Average (years of) Teaching Experience, Percentage of Christians in the School, and Percentage of Dropout at the school level.9 Table A.1 in Online Appendix A reports the descriptive statistics for these variables for the whole Second Intifada period (2000–2006). We merge this data set on school characteristics with the data set containing the individual test scores by the identification number of the school.
The third data set contains information on all Palestinian fatalities during the Second Intifada. Data on fatalities related to the Israeli–Palestinian conflict are collected and distributed by the Israeli NGO B’Tselem (Israeli Information Center for Human Rights in the Occupied Territories).10 For each fatality, the B’Tselem data set reports name, age, and gender of the victim, location of the event, and a description of the circumstances of the event. The information on each fatality is based on B’Tselem's investigation into the circumstances of each death occurred during a conflict event.11 B’Tselem crosschecks its information with official IDF announcements, information gathered by Palestinian and international human rights organizations and media reports. B’Tselem data on fatalities are considered to be accurate and reliable by both Israelis and Palestinians (Mansour and Rees 2012) and have previously been used by other scholars studying the Israeli–Palestinian conflict (e.g., Jaeger and Paserman 2008; Jaeger et al. 2012).
Using the available information for each fatality, we construct our main variable to measure conflict intensity as the total number of all Palestinians killed by the IDF in each locality during each academic year.12 We merge our conflict intensity variable with the other two data sets using the information on the locality of the event. Table A.2 in Online Appendix A reports the descriptive statistics for Palestinian fatalities between the beginning of the Second Intifada in September 2000 and June 2006.13 After the rapid increase in the number of fatalities after the start of the Second Intifada, the conflict reaches a peak in 2002 and then decreases in intensity until 2006. The total number of Palestinian fatalities during the period under consideration is 1495. As reported in Table 1, the yearly average number at the locality level is 7.7, its standard deviation is 17.2 and the maximum is 105. This indicates a large variation in students' exposure to conflict across localities and within localities over time.
The fourth data source is the Palestinian Labor Forces Surveys (PLFS) for the period 2000–2006, as provided by the PCBS. The PLFS is a representative household survey of Palestinians living in the OPT. The PLFS is a rotating quarterly panel where households are surveyed four times over six quarters. They are surveyed for two consecutive quarters, not surveyed in the next two quarters, and then surveyed again for two consecutive quarters. The survey collects quarterly data on employment and socioeconomic characteristics of individual household members aged 15 or older. The survey also reports school attendance status and the number of completed years of education for each individual. We use PLFS data to discuss the possible issue of sample selection in our main data set and to compute various economic variables (e.g., district-level wage, low-skilled wage, and unemployment rate) to explore the relationship between conflict intensity and local-level economic conditions.
4. Econometric Model and Identification Strategy
The sign of the relationship between conflict intensity and student academic achievement is not obvious. On the one hand, conflict may negatively affect the performance of the student at the final exam. For instance, the conflict may make the learning process more difficult (by deteriorating the quality of the school environment or by causing the student to suffer psychological distress), or it could reduce the incentives to attend school (by reducing the value of education or increasing the value of the student's outside option), thus lowering the probability of passing the exam. On the other hand, the conflict may provide a strong incentive to increase the learning effort and to perform well at the final exam, because this could offer the opportunity to access well-paid jobs after school completion or to escape the conflict situation by studying abroad. Moreover, it is also possible that the effect of the conflict is heterogeneous across students and that the sign and magnitude of its impact depend on individual characteristics such as academic ability or gender. Only a rigorous analysis can discriminate between these theoretical predictions.
5. Results
5.1. The Impact of Violent Conflict on the Final Exam Outcome
In our baseline specification of model (1), we use as dependent variable Pass exam, a dummy that takes value 1 if the student passes the final exam and 0 otherwise. Table 2 reports the within-school estimates of the effect of conflict intensity on the individual probability of passing the exam. Column (1) shows the result for a specification that includes only birth-cohort, school-, and year-fixed effects. The estimated coefficient for Number of fatalities captures the difference in percentage points in the probability of passing the final exam for students enrolled in the same school in different years that is only due to the difference in the number of Palestinian fatalities occurring in the school locality during the academic year. The results shown in the table indicate that the impact of conflict on the probability of passing the exam is negative and highly significant.
Effect of conflict intensity on the probability of passing the high-school final exam for Palestinian students in the West Bank during the Second Intifada.
. | Pass exam . | |||
---|---|---|---|---|
. | Full sample . | First-time takers . | ||
. | (1) . | (2) . | (3) . | (4) . |
Number of fatalities | |$-$|0.43*** | |$-$|0.49*** | |$-$|0.52*** | |$-$|0.45*** |
(|$-$|0.14) | (|$-$|0.15) | (|$-$|0.15) | (|$-$|0.14) | |
Male | |$-$|0.06*** | |$-$|0.06*** | |$-$|0.07*** | |
(|$-$|0.01) | (|$-$|0.01) | (|$-$|0.01) | ||
Christian | |$-$|0.01 | |$-$|0.01 | |$-$|0.01 | |
(|$-$|0.02) | (|$-$|0.02) | (|$-$|0.02) | ||
Adabi | |$-$|0.19*** | |$-$|0.19*** | |$-$|0.18*** | |
(|$-$|0.01) | (|$-$|0.01) | (|$-$|0.01) | ||
District-level economic controls | No | No | Yes | Yes |
Birth-cohort fixed effects | Yes | Yes | Yes | Yes |
School-fixed effects | Yes | Yes | Yes | Yes |
Academic-year fixed effects | Yes | Yes | Yes | Yes |
Number of observations | 138,135 | 138,135 | 138,135 | 103,756 |
Mean of the dependent variable | 0.74 | 0.74 | 0.74 | 0.78 |
. | Pass exam . | |||
---|---|---|---|---|
. | Full sample . | First-time takers . | ||
. | (1) . | (2) . | (3) . | (4) . |
Number of fatalities | |$-$|0.43*** | |$-$|0.49*** | |$-$|0.52*** | |$-$|0.45*** |
(|$-$|0.14) | (|$-$|0.15) | (|$-$|0.15) | (|$-$|0.14) | |
Male | |$-$|0.06*** | |$-$|0.06*** | |$-$|0.07*** | |
(|$-$|0.01) | (|$-$|0.01) | (|$-$|0.01) | ||
Christian | |$-$|0.01 | |$-$|0.01 | |$-$|0.01 | |
(|$-$|0.02) | (|$-$|0.02) | (|$-$|0.02) | ||
Adabi | |$-$|0.19*** | |$-$|0.19*** | |$-$|0.18*** | |
(|$-$|0.01) | (|$-$|0.01) | (|$-$|0.01) | ||
District-level economic controls | No | No | Yes | Yes |
Birth-cohort fixed effects | Yes | Yes | Yes | Yes |
School-fixed effects | Yes | Yes | Yes | Yes |
Academic-year fixed effects | Yes | Yes | Yes | Yes |
Number of observations | 138,135 | 138,135 | 138,135 | 103,756 |
Mean of the dependent variable | 0.74 | 0.74 | 0.74 | 0.78 |
Notes: OLS regression results for equation (1). For all regressions, the dependent variable is Pass exam, a dummy that takes value 1 if the student has passed the final exam and 0 otherwise. Number of fatalities is the locality-level number of conflict-related Palestinian fatalities caused by the IDF during the academic year divided 1000. Male is a dummy that takes value 1 if the student is male and 0 otherwise. Christian is a dummy that takes value 1 if the student is Christian and 0 otherwise. Adabi is a dummy that takes value 1 if the student is enrolled in the Adabi stream and 0 if enrolled in the Elmi stream. District-level economic controls include district-level average wage, district-level low-skilled wage and district-level unemployment rate. Column (4) includes only first-time exam takers, that is, students who are 17 or 18 at the exam. In each regression, a constant is included but not shown. Robust standard errors in parenthesis are clustered at the locality level. ***Significant at 1%.
Effect of conflict intensity on the probability of passing the high-school final exam for Palestinian students in the West Bank during the Second Intifada.
. | Pass exam . | |||
---|---|---|---|---|
. | Full sample . | First-time takers . | ||
. | (1) . | (2) . | (3) . | (4) . |
Number of fatalities | |$-$|0.43*** | |$-$|0.49*** | |$-$|0.52*** | |$-$|0.45*** |
(|$-$|0.14) | (|$-$|0.15) | (|$-$|0.15) | (|$-$|0.14) | |
Male | |$-$|0.06*** | |$-$|0.06*** | |$-$|0.07*** | |
(|$-$|0.01) | (|$-$|0.01) | (|$-$|0.01) | ||
Christian | |$-$|0.01 | |$-$|0.01 | |$-$|0.01 | |
(|$-$|0.02) | (|$-$|0.02) | (|$-$|0.02) | ||
Adabi | |$-$|0.19*** | |$-$|0.19*** | |$-$|0.18*** | |
(|$-$|0.01) | (|$-$|0.01) | (|$-$|0.01) | ||
District-level economic controls | No | No | Yes | Yes |
Birth-cohort fixed effects | Yes | Yes | Yes | Yes |
School-fixed effects | Yes | Yes | Yes | Yes |
Academic-year fixed effects | Yes | Yes | Yes | Yes |
Number of observations | 138,135 | 138,135 | 138,135 | 103,756 |
Mean of the dependent variable | 0.74 | 0.74 | 0.74 | 0.78 |
. | Pass exam . | |||
---|---|---|---|---|
. | Full sample . | First-time takers . | ||
. | (1) . | (2) . | (3) . | (4) . |
Number of fatalities | |$-$|0.43*** | |$-$|0.49*** | |$-$|0.52*** | |$-$|0.45*** |
(|$-$|0.14) | (|$-$|0.15) | (|$-$|0.15) | (|$-$|0.14) | |
Male | |$-$|0.06*** | |$-$|0.06*** | |$-$|0.07*** | |
(|$-$|0.01) | (|$-$|0.01) | (|$-$|0.01) | ||
Christian | |$-$|0.01 | |$-$|0.01 | |$-$|0.01 | |
(|$-$|0.02) | (|$-$|0.02) | (|$-$|0.02) | ||
Adabi | |$-$|0.19*** | |$-$|0.19*** | |$-$|0.18*** | |
(|$-$|0.01) | (|$-$|0.01) | (|$-$|0.01) | ||
District-level economic controls | No | No | Yes | Yes |
Birth-cohort fixed effects | Yes | Yes | Yes | Yes |
School-fixed effects | Yes | Yes | Yes | Yes |
Academic-year fixed effects | Yes | Yes | Yes | Yes |
Number of observations | 138,135 | 138,135 | 138,135 | 103,756 |
Mean of the dependent variable | 0.74 | 0.74 | 0.74 | 0.78 |
Notes: OLS regression results for equation (1). For all regressions, the dependent variable is Pass exam, a dummy that takes value 1 if the student has passed the final exam and 0 otherwise. Number of fatalities is the locality-level number of conflict-related Palestinian fatalities caused by the IDF during the academic year divided 1000. Male is a dummy that takes value 1 if the student is male and 0 otherwise. Christian is a dummy that takes value 1 if the student is Christian and 0 otherwise. Adabi is a dummy that takes value 1 if the student is enrolled in the Adabi stream and 0 if enrolled in the Elmi stream. District-level economic controls include district-level average wage, district-level low-skilled wage and district-level unemployment rate. Column (4) includes only first-time exam takers, that is, students who are 17 or 18 at the exam. In each regression, a constant is included but not shown. Robust standard errors in parenthesis are clustered at the locality level. ***Significant at 1%.
The negative effect of conflict on final exam result is robust to the inclusion of several control variables. In column (2), we add a set of student characteristics.14 Results indicate that the probability of passing the exam is lower for male students and for those enrolled in the Adami stream. In column (3), we include a set of district-level economic variables, such as the average wage, low-skilled wage and unemployment rate, to control for time-varying local economic conditions and the student's outside options. The magnitude and significance of the effect of conflict on the probability of passing the exam remains highly significant and slightly increases.
Computing the average magnitude of the within-school estimates of the effect of conflict, we find that one standard deviation increase in the number of fatalities occurring during the academic year at the locality level reduces the probability of passing the exam by 1 percentage point [(−0.52/1000) × (17.2) = −0.01]. As the mean of Pass exam is 0.74, this implies that a one standard deviation increase in the Number of fatalities reduces the probability of passing the exam by 1.2% of the mean.15
Finally, in column (4), we report the results when we restrict the sample to students taking the exam for the first time.16 The impact of conflict on the probability of passing the exam remains negative and highly significant whereas its magnitude is slightly reduced.
5.1.1. Threats to Identification
Our identifying strategy is valid under the assumption that, conditional on school- and time-fixed effects and controlling for observable individual characteristics, the within-school variation in conflict intensity over time is orthogonal to any other determinant of student results at the exam. There are four possible reasons why this assumption may not hold.
First, it is possible that our analysis suffers from a sample selection bias due to conflict affecting the characteristics of the pool of students taking the final exam. There are at least two aspects to consider. The conflict could affect the characteristics of students who enroll in high school. Furthermore, conflict may select the students who are actually taking the exam at the end of the academic year. As for the first aspect, the concern is that low-ability students who in the absence of the conflict would have abandoned school after the compulsory years may instead decide to continue schooling because the conflict reduces out-of-school occupational opportunities. In this case, the finding of a lower academic performance in localities with higher conflict intensity would just be the result of the conflict lowering the average ability of students enrolling in high school in those localities.17
Unfortunately, the MOEHE data set includes information only on students who actually took the exam. This implies that we cannot compare the ability level of enrolled individuals versus those who are not. Thus, to explore how conflict affects the probability of continuing to high school, we use PLFS data to estimate the determinants of the transition from compulsory to noncompulsory education. We take advantage of the fact that the PLFS is a rotating quarterly panel and define a variable capturing the change in schooling status from one year to the other. To identify those individuals who are in their last year of compulsory education, we focus on students aged 15 and 16 with at least 9 years of completed education at the first interview. We measure conflict intensity as the cumulative number of fatalities occurred during the twelve months before the last interview in the district of residence of the student.
Results reported in Table A.3 of Online Appendix A, column (1) indicate that the probability of continuing to high school after compulsory education is uncorrelated with conflict intensity, whereas it is positively correlated with the level of household head's education. In column (2), we include the interactions of conflict intensity with the level of household head's education level and with the district-level economic conditions, our proxies for the value of the student's outside options. Results are unchanged with respect to column (1) and indicate none of the interaction terms is significant. We interpret this evidence as indicating that students continuing to high school are those from higher-educated and wealthier households. Moreover, these results do not support the possibility that conflict induces lower-ability or disadvantaged students to stay in school by changing the out-of-school occupational opportunities.18
As noted previously, conflict may also select the sample of students who actually take the exam at the end of the academic year. In this case, the concern is that, if conflict makes higher-ability students to drop out during the academic year, our finding of a negative effect of conflict on the probability of passing the exam could just be the consequence of the lower average ability of the students taking the exam.
To test for this possibility, we perform two exercises. First, we estimate the determinants of school attendance for students in high school using the PLFS data set. To this end, we consider individuals aged 16, 17, and 18 living in the West Bank who have completed 10 years of education and are attending school. Results shown in Table A.4 of Online Appendix A indicate that high school attendance is uncorrelated with conflict intensity, whereas it is positively correlated with the household head having secondary or higher education.
Second, we look at the effect of conflict on the number of students actually taking the final exam at the end of grade 12 using the information included in the MOEHE data set. To test for this, we use the school-level Number of exam takers and Percentage of dropouts, that is, percentage of individuals who are enrolled but abandon school during the academic year. We regress these measures on the locality number of fatalities controlling for local economic conditions and school- and academic-year fixed effects. Results reported in Table A.5 of Online Appendix A show that the within-school number of exam takers and the dropout rate do not change with conflict intensity. These results indicate that—among the enrolled students—those taking the exam at the end of grade 12 are not selected by the conflict.
In summary, we do not find evidence that higher conflict intensity induces lower-ability students to continue to high school, to stay in school or to take the final exam, compared to other students. Taken together, these findings suggest that there is no evidence of a conflict-induced sample selection invalidating our analysis.
A third possible threat to our identification strategy is that of reverse causality, that is, student's academic achievement determines conflict intensity at the locality level, with localities with more dropouts or low-performing students having a higher number of fatalities.21 In this case, the estimated coefficient for the number of fatalities would be upward biased to the extent that the error term is capturing the fact that students not passing the final exam would be those fighting against the IDF, thus creating the conditions for more fatalities. Although we cannot completely rule out this possibility, we argue that the effect is unlikely to be large. As discussed previously, our data show no correlation between conflict intensity and dropout rate at the locality or school levels. Moreover, our measure of conflict intensity is the total number of all fatalities occurring during the academic year up to the date of the exam, thus before the exam actually takes place.
A final threat to our identification strategy would result from students responding to an increase in conflict intensity by changing residential location. If migrating students were the higher-ability ones, the negative effect of the conflict on exam results would just be due to the decision of these students to move away from schools in high-conflict localities. The available empirical evidence suggests that this is not the case. In fact, internal and external mobility was severely restricted in the West Bank during the Second Intifada (World Bank 2007a; CIA 2008).22 Although we cannot directly test the determinants of student migration decisions, there is indirect empirical evidence showing that Palestinian mobility was extremely low during the period under consideration. PCBS (2009) documents that internal migration was “very negligible” (PCBS 2009, p. 93) after the outbreak of the Second Intifada. Between 1997 and 2007, the percentage of Palestinians who migrated internally decreased by 16%. Only 7.1% of the total population in the West Bank moved within the same district in this ten-year period. Looking at individuals aged 19 or younger, there has been a reduction by 8% in the number of movers during this period. Interestingly, the main motivations for internal migration during the period under analysis is marriage. Based on these results, we argue that, in the context of the Second Intifada, internal and external migration of students should not be a threat to our identification strategy.
A related concern is that students may decide to move to a different school in the same locality to avoid higher conflict intensity. Although this may be a common behavior in other conflict settings, there are two elements suggesting that this is less likely in the case of the West Bank during the Second Intifada. First, as we have seen, there is no evidence that schools more exposed to conflict suffer a larger reduction in the number of exam takers (see Table A.6 in Online Appendix A). Second, this strategy is unlikely to reduce the risk of being exposed to violence. In the case of the West Bank during the Second Intifada, this risk increases with traveling time (see Save the Children 2003; World Bank 2007a). Since moving to a different school is likely to significantly increase the latter,23 it is doubtful that this would decrease the risk of being victim of a violent event (Akesson 2014).
5.1.2. Robustness Checks
Our results are robust to several checks. To begin, we include as an additional control in regression (1) the number of fatalities during the previous academic year. Results shown in Table A.7 of Online Appendix A (column (1)) indicate that the lagged conflict-intensity variable is not significant whereas the magnitude and significance of Number of fatalities is unchanged with respect to our main specification.24 This suggests that the number of fatalities occurring during the academic year of the exam is the relevant variable to include.
Next, we conduct a placebo test on the timing of the conflict events. We estimate regression (1) including the locality-level number of Palestinian fatalities in the next academic year. Results shown in Table A.7 of Online Appendix A (column (2)) indicate that the effect of the number of fatalities in the year after the one in which the student takes the exam is not significant whereas the coefficient for Number of fatalities is only slightly reduced.
Third, we check that our results are robust to possible spillover effects. To this end, we include as an additional control in regression (1) the total number of Palestinian fatalities in the district the school belongs to minus the number of fatalities in the locality of the school (to avoid double counting). This variable allows us to capture in a simple way the effects of conflict events taking place in localities that are near the school from a geographical and an administrative perspective. Results shown in Table A.7 of Online Appendix A (column (3)) indicate that including this additional control slightly increases the magnitude of the effect of the number of Palestinian fatalities at the locality level and its effect remains highly significant. The coefficient of the proxy for the spillover effect has the expected negative sign. This suggests that higher conflict intensity in neighboring localities has a potential negative impact on students’ academic performance. Yet, the effect is not significant at conventional levels. We interpret this result as suggesting that the locality level is the relevant dimension to look at to capture the effects of conflict intensity on academic outcomes.
Finally, we check that our results are robust to the inclusion of controls for the intensity of the internal mobility restrictions imposed by the IDF.25 To this end, we use the index presented in Cali and Miaari (2018). The index is a time-varying, locality-level variable that for each locality and each year counts the number of all mobility obstacles (i.e., physical barriers and checkpoints) within thirty minutes of travel time (by existing roads) from the locality's centroid, weighted by the inverse of their travel time.26 Results shown in Table A.8 of Online Appendix A, column (2) indicate that the effect of conflict intensity on the probability of passing the exam does not change with respect to our main specification (see Table A.8 in Online Appendix A, column (1)), whereas the effect of the mobility restrictions (as measured by the coefficient Mobility Restriction Index) is not significant at conventional levels. Next, to account for possible differences in the effect of mobility restrictions depending on the locality-level population size, we compute the Congestion Mobility Restriction Index as the product between the Mobility Restriction Index and the locality-level population.27 Results shown in Table A.8 of Online Appendix A, column (3), confirm that our main result is unchanged.
5.2. Additional Results
5.2.2. Alternative Measures of Academic Achievement
To test for the effect of conflict on the total test score as an alternative measure of academic achievement, we estimate again regression (1) using for Resultislt the individual Total test score at the exam.28 The effect of conflict intensity is significant and negative (Table 3, column (1)). The size of the estimated coefficient indicates that a one standard deviation increase in the number of fatalities decreases Total test score by 4 percentage points [(−218.4/1000) × 17.2], which, given that the mean value of the dependent variable is 640, is equivalent to a 1% reduction. This translates into a 2 points reduction in the Total test score for a student enrolled in a school in a locality characterized by the average level of conflict intensity [(−218.4/1000) × 7.7] relative to a student enrolled in a school in a locality that experienced no conflict. The reduction can be as large as 22 points for students in schools situated in the most conflict-exposed localities.
Effect of conflict intensity on alternative measures of academic achievement.
. | Total test score . | Math test score . | Arabic test score . | Access University . | Access University Abroad . |
---|---|---|---|---|---|
. | (1) . | (2) . | (3) . | (4) . | (5) . |
Number of fatalities | |$-$|218.43** | |$-$|50.44*** | |$-$|22.99*** | |$-$|0.53*** | |$-$|0.12 |
(93.24) | (15.10) | (7.43) | (0.18) | (0.18) | |
Individual characteristics | Yes | Yes | Yes | Yes | Yes |
District-level economic controls | Yes | Yes | Yes | Yes | Yes |
Birth-cohort fixed effects | Yes | Yes | Yes | Yes | Yes |
School-fixed effects | Yes | Yes | Yes | Yes | Yes |
Academic-year fixed effects | Yes | Yes | Yes | Yes | Yes |
Number of observations | 138,135 | 138,060 | 138,135 | 138,135 | 138,135 |
Mean of the dependent variable | 640.5 | 64.3 | 66.8 | 0.53 | 0.17 |
. | Total test score . | Math test score . | Arabic test score . | Access University . | Access University Abroad . |
---|---|---|---|---|---|
. | (1) . | (2) . | (3) . | (4) . | (5) . |
Number of fatalities | |$-$|218.43** | |$-$|50.44*** | |$-$|22.99*** | |$-$|0.53*** | |$-$|0.12 |
(93.24) | (15.10) | (7.43) | (0.18) | (0.18) | |
Individual characteristics | Yes | Yes | Yes | Yes | Yes |
District-level economic controls | Yes | Yes | Yes | Yes | Yes |
Birth-cohort fixed effects | Yes | Yes | Yes | Yes | Yes |
School-fixed effects | Yes | Yes | Yes | Yes | Yes |
Academic-year fixed effects | Yes | Yes | Yes | Yes | Yes |
Number of observations | 138,135 | 138,060 | 138,135 | 138,135 | 138,135 |
Mean of the dependent variable | 640.5 | 64.3 | 66.8 | 0.53 | 0.17 |
Notes: OLS regression results for equation (1). Total test score is the standardized total test score at the exam (see Online Appendix B for details). Math test score is the standardized test score for the math module of the exam. Arabic test score is the standardized test score for the Arabic module of the exam. For details on how Access University is a dummy variable that takes value 1 is the student total score at the exam is higher than the minimum test score necessary for admission to university (650) and 0 otherwise. Access University Abroad is a dummy variable that takes value 1 if the student total score at the exam is higher than the minimum test score necessary for admission to universities in other Middle Eastern countries (850) and 0 otherwise. Number of fatalities is the locality-level number of conflict-related Palestinian fatalities caused by the IDF during the academic year divided by 1000. Individual characteristics include: Male (a dummy that takes value 1 if the student is male and 0 otherwise); Christian (a dummy that takes value 1 if the student is Christian and 0 otherwise); Adabi (a dummy that takes value 1 if the student is enrolled in the Adabi stream and 0 if enrolled in the Elmi stream). District-level economic controls are the same as in Table 2. In each regression, a constant is included but not shown. Robust standard errors in parenthesis are clustered at the locality level. **Significant at 5%; ***significant at 1%.
Effect of conflict intensity on alternative measures of academic achievement.
. | Total test score . | Math test score . | Arabic test score . | Access University . | Access University Abroad . |
---|---|---|---|---|---|
. | (1) . | (2) . | (3) . | (4) . | (5) . |
Number of fatalities | |$-$|218.43** | |$-$|50.44*** | |$-$|22.99*** | |$-$|0.53*** | |$-$|0.12 |
(93.24) | (15.10) | (7.43) | (0.18) | (0.18) | |
Individual characteristics | Yes | Yes | Yes | Yes | Yes |
District-level economic controls | Yes | Yes | Yes | Yes | Yes |
Birth-cohort fixed effects | Yes | Yes | Yes | Yes | Yes |
School-fixed effects | Yes | Yes | Yes | Yes | Yes |
Academic-year fixed effects | Yes | Yes | Yes | Yes | Yes |
Number of observations | 138,135 | 138,060 | 138,135 | 138,135 | 138,135 |
Mean of the dependent variable | 640.5 | 64.3 | 66.8 | 0.53 | 0.17 |
. | Total test score . | Math test score . | Arabic test score . | Access University . | Access University Abroad . |
---|---|---|---|---|---|
. | (1) . | (2) . | (3) . | (4) . | (5) . |
Number of fatalities | |$-$|218.43** | |$-$|50.44*** | |$-$|22.99*** | |$-$|0.53*** | |$-$|0.12 |
(93.24) | (15.10) | (7.43) | (0.18) | (0.18) | |
Individual characteristics | Yes | Yes | Yes | Yes | Yes |
District-level economic controls | Yes | Yes | Yes | Yes | Yes |
Birth-cohort fixed effects | Yes | Yes | Yes | Yes | Yes |
School-fixed effects | Yes | Yes | Yes | Yes | Yes |
Academic-year fixed effects | Yes | Yes | Yes | Yes | Yes |
Number of observations | 138,135 | 138,060 | 138,135 | 138,135 | 138,135 |
Mean of the dependent variable | 640.5 | 64.3 | 66.8 | 0.53 | 0.17 |
Notes: OLS regression results for equation (1). Total test score is the standardized total test score at the exam (see Online Appendix B for details). Math test score is the standardized test score for the math module of the exam. Arabic test score is the standardized test score for the Arabic module of the exam. For details on how Access University is a dummy variable that takes value 1 is the student total score at the exam is higher than the minimum test score necessary for admission to university (650) and 0 otherwise. Access University Abroad is a dummy variable that takes value 1 if the student total score at the exam is higher than the minimum test score necessary for admission to universities in other Middle Eastern countries (850) and 0 otherwise. Number of fatalities is the locality-level number of conflict-related Palestinian fatalities caused by the IDF during the academic year divided by 1000. Individual characteristics include: Male (a dummy that takes value 1 if the student is male and 0 otherwise); Christian (a dummy that takes value 1 if the student is Christian and 0 otherwise); Adabi (a dummy that takes value 1 if the student is enrolled in the Adabi stream and 0 if enrolled in the Elmi stream). District-level economic controls are the same as in Table 2. In each regression, a constant is included but not shown. Robust standard errors in parenthesis are clustered at the locality level. **Significant at 5%; ***significant at 1%.
In columns (2) and (3), we report the results for the two most important subjects that are present in the exam for both streams, namely mathematics and Arabic. The results indicate that the effect of conflict intensity is larger for Math test score than for Arabic test score, with a one standard deviation increase in the number of fatalities decreasing the former by 0.03 standard deviations and the latter by 0.02. The larger effect for Math test score is in line with previous studies showing that math test scores are more sensitive to conditions in the learning environment (e.g., Hanushek and Rivkin 2010; Monteiro and Rocha 2017; Gershenson and Tekin 2018).29
Finally, we consider two other measures of academic achievement. We estimate model (1) using for Resultislt, respectively: Access University, a dummy variable that takes value 1 if the student obtains at least the minimum test score for admission to university in the OPT (i.e., 650 over 1000) and 0 otherwise; and Access University Abroad, a dummy variable that takes value 1 if the student gets at least the minimum test score required for admission to university in other Middle Eastern countries and the engineering major in Palestinian universities (i.e., 850 over 1000) and 0 otherwise. Results reported in column (4) indicate that the conflict reduces students’ probability of achieving the minimum test score required for admission to university. Interestingly, results in column (5) instead indicate that conflict does not reduce the probability of achieving the minimum test score necessary for admission to universities abroad. We interpret these results as suggesting the possibility that the effect of conflict is heterogeneous across levels of academic ability, with high-ability students possibly being less affected by the conflict. We explore this issue in more detail in what follows.
5.2.3. Heterogeneity
Performance at the Exam: Conflict intensity reduces the Total test score at the exam. Yet, it is possible that the magnitude of the effect is heterogeneous along the Total test score distribution. To test for this, we employ a quantile regression model (Koenker and Hallock 2001). Results are shown in Table 4, columns (2)–(6). Column (1) reports for comparison the OLS estimate of the effect of conflict intensity on Total test score (the same as in Table 2, column (1)). The quantile regression results indicate some interesting differences in the effect of conflict across the various parts of the conditional distribution of the Total test score. The conflict lowers all the quantiles of the distribution but the 95th, for which the effect of conflict on Total test score is instead very small and not significant.30 Under the assumption that conflict does not affect the rank of students in the test score distribution, these results indicate that higher conflict intensity, whereas it reduces both the probability of passing the exam (see Table 2) and of being admitted to university (see Table 3), has no effect on students in the upper tail of the distribution.
Effect of conflict intensity on total test score: OLS and quantile regression results.
. | Total test score . | |||||||
---|---|---|---|---|---|---|---|---|
. | OLS . | Quantiles . | ||||||
. | . | 5th . | 10th . | 25th . | 50th . | 75th . | 90th . | 95th . |
. | (1) . | (2) . | (3) . | (4) . | (5) . | (6) . | (7) . | (8) . |
Number of fatalities | |$-$|218.43*** | |$-$|200.60*** | |$-$|239.98** | |$-$|204.93** | |$-$|188.93** | |$-$|240.57*** | |$-$|178.43** | |$-$|46.68 |
(93.24) | (83.77) | (90.47) | (65.42) | (58.15) | (55.47) | (56.59) | (49.35) | |
All controls | Yes | Yes | Yes | Yes | Yes | Yes | Yes | Yes |
Birth-cohort fixed effects | Yes | Yes | Yes | Yes | Yes | Yes | Yes | Yes |
School-fixed effects | Yes | Yes | Yes | Yes | Yes | Yes | Yes | Yes |
Academic-year fixed effects | Yes | Yes | Yes | Yes | Yes | Yes | Yes | Yes |
No. observations | 138,135 | 138,135 | 138,135 | 138,135 | 138,135 | 138,135 | 138,135 | 138,135 |
Mean of dep. variable | 640 | 220 | 330 | 522 | 670 | 807 | 890 | 927 |
. | Total test score . | |||||||
---|---|---|---|---|---|---|---|---|
. | OLS . | Quantiles . | ||||||
. | . | 5th . | 10th . | 25th . | 50th . | 75th . | 90th . | 95th . |
. | (1) . | (2) . | (3) . | (4) . | (5) . | (6) . | (7) . | (8) . |
Number of fatalities | |$-$|218.43*** | |$-$|200.60*** | |$-$|239.98** | |$-$|204.93** | |$-$|188.93** | |$-$|240.57*** | |$-$|178.43** | |$-$|46.68 |
(93.24) | (83.77) | (90.47) | (65.42) | (58.15) | (55.47) | (56.59) | (49.35) | |
All controls | Yes | Yes | Yes | Yes | Yes | Yes | Yes | Yes |
Birth-cohort fixed effects | Yes | Yes | Yes | Yes | Yes | Yes | Yes | Yes |
School-fixed effects | Yes | Yes | Yes | Yes | Yes | Yes | Yes | Yes |
Academic-year fixed effects | Yes | Yes | Yes | Yes | Yes | Yes | Yes | Yes |
No. observations | 138,135 | 138,135 | 138,135 | 138,135 | 138,135 | 138,135 | 138,135 | 138,135 |
Mean of dep. variable | 640 | 220 | 330 | 522 | 670 | 807 | 890 | 927 |
Notes: Column (1) reports OLS regression results. Columns (2)–(6) report quantile regression results. The dependent variable for all regressions is Total test score. Number of fatalities is the number of all conflict-related Palestinian fatalities caused by IDF at the locality level during the academic year divided by 1000. All controls include Individual characteristics and District-level economic controls as in Table 3. In each regression, a constant is included but not shown. For column (1), robust standard errors in parenthesis are clustered at the locality level. For columns (2)–(6), bootstrap standard errors in parenthesis. **Significant at 5%; ***significant at 1%.
Effect of conflict intensity on total test score: OLS and quantile regression results.
. | Total test score . | |||||||
---|---|---|---|---|---|---|---|---|
. | OLS . | Quantiles . | ||||||
. | . | 5th . | 10th . | 25th . | 50th . | 75th . | 90th . | 95th . |
. | (1) . | (2) . | (3) . | (4) . | (5) . | (6) . | (7) . | (8) . |
Number of fatalities | |$-$|218.43*** | |$-$|200.60*** | |$-$|239.98** | |$-$|204.93** | |$-$|188.93** | |$-$|240.57*** | |$-$|178.43** | |$-$|46.68 |
(93.24) | (83.77) | (90.47) | (65.42) | (58.15) | (55.47) | (56.59) | (49.35) | |
All controls | Yes | Yes | Yes | Yes | Yes | Yes | Yes | Yes |
Birth-cohort fixed effects | Yes | Yes | Yes | Yes | Yes | Yes | Yes | Yes |
School-fixed effects | Yes | Yes | Yes | Yes | Yes | Yes | Yes | Yes |
Academic-year fixed effects | Yes | Yes | Yes | Yes | Yes | Yes | Yes | Yes |
No. observations | 138,135 | 138,135 | 138,135 | 138,135 | 138,135 | 138,135 | 138,135 | 138,135 |
Mean of dep. variable | 640 | 220 | 330 | 522 | 670 | 807 | 890 | 927 |
. | Total test score . | |||||||
---|---|---|---|---|---|---|---|---|
. | OLS . | Quantiles . | ||||||
. | . | 5th . | 10th . | 25th . | 50th . | 75th . | 90th . | 95th . |
. | (1) . | (2) . | (3) . | (4) . | (5) . | (6) . | (7) . | (8) . |
Number of fatalities | |$-$|218.43*** | |$-$|200.60*** | |$-$|239.98** | |$-$|204.93** | |$-$|188.93** | |$-$|240.57*** | |$-$|178.43** | |$-$|46.68 |
(93.24) | (83.77) | (90.47) | (65.42) | (58.15) | (55.47) | (56.59) | (49.35) | |
All controls | Yes | Yes | Yes | Yes | Yes | Yes | Yes | Yes |
Birth-cohort fixed effects | Yes | Yes | Yes | Yes | Yes | Yes | Yes | Yes |
School-fixed effects | Yes | Yes | Yes | Yes | Yes | Yes | Yes | Yes |
Academic-year fixed effects | Yes | Yes | Yes | Yes | Yes | Yes | Yes | Yes |
No. observations | 138,135 | 138,135 | 138,135 | 138,135 | 138,135 | 138,135 | 138,135 | 138,135 |
Mean of dep. variable | 640 | 220 | 330 | 522 | 670 | 807 | 890 | 927 |
Notes: Column (1) reports OLS regression results. Columns (2)–(6) report quantile regression results. The dependent variable for all regressions is Total test score. Number of fatalities is the number of all conflict-related Palestinian fatalities caused by IDF at the locality level during the academic year divided by 1000. All controls include Individual characteristics and District-level economic controls as in Table 3. In each regression, a constant is included but not shown. For column (1), robust standard errors in parenthesis are clustered at the locality level. For columns (2)–(6), bootstrap standard errors in parenthesis. **Significant at 5%; ***significant at 1%.
Gender of the Student Previous research has documented that exposure to conflict can result in significant gender differentials in individual educational outcomes (Chamarbagwala and Morán 2010; Shemyakina 2011; Buvinić et al. 2014; Valente 2013). To check for this possibility in the context of the Second Intifada, we include the interaction between the number of fatalities and the dummy for the gender of the student (Male). Results reported in Table A.9 of Online Appendix A indicate that there is no evidence of a statistically significant differential effect of conflict intensity between boys and girls on the probability of passing the exam or on the total test score. This suggests that the mechanisms behind the impact of conflict on academic achievement are gender neutral.
Stream of Study, Age Cohort, and Private versus Government Schools In unreported regressions (available upon request), we also look at the possible differential effects of conflict by stream of study, by age cohorts, and by school supervising authority (government/private).31 These additional checks do not alter our conclusions in any meaningful way.
6. Possible Transmission Mechanisms
6.1. Changes in the Quality of the School-Level Learning Conditions
The first mechanism we consider is that of the conflict-induced change in the quality of the learning environment at school. To show that this may contribute to explain the negative effect of conflict on academic achievement, we first provide evidence of the effect of conflict on a set of school characteristics that determine the quality of the learning environment. Next, we show how those characteristics are correlated with exam performance.
We proxy school characteristics using a large set of variables provided by the MOEHE. These are: Student/Teacher Ratio, Average Size of the Classroom, Average Density in the Classroom, and Teachers’ Average (years of) Experience. The Student/Teacher Ratio is the school-level ratio between the number of students and the number of teachers. The Average Size of the Classroom is the school-level average size of the classroom measured in square meters, that is, it is a measure of the physical size of the classroom. Teachers’ Average Experience is the average number of teaching years of the teachers in the school. The Average Density in the Classroom is the average number of students per square meter in the classroom. To these variables commonly used in the literature to proxy for the quality of school supply, we add the Percentage of Christians in the School, to proxy for religious and cultural heterogeneity at the school level.
To estimate the effect of conflict intensity on the quality of the school-learning environment, we regress each school characteristic on the locality-level number of Palestinian fatalities caused by the IDF during the academic year, controlling for school- and academic-year fixed effects.
Results are reported in Table 5. Conflict intensity increases Average Density in the Classroom (column (1)). There are two main reasons for this effect. First, military attacks and school occupations by IDF damaged and—in some cases—destroyed premises and properties of Palestinian schools (Palestinian Ministry of Education and Higher Education (MOEHE) 2005). Several classrooms became unavailable due to the destruction of walls, doors, windows and furniture (Giacaman et al. 2002). Military operations during the Second Intifada indeed caused a chronic shortage of classrooms (UN-OCHA-OPT Humanitarian Monitoring, various issues).32 Second, the conflict made it more difficult for the Palestinian Authority to increase the supply of schools in the OPT to meet the growing number of school-aged Palestinians (World Bank 2002).33 The need to rebuild infrastructure damaged by the conflict reduced the resources available to expand existing schools or to construct new ones. In fact, during the Second Intifada, the development or upgrading of Palestinian schools was very difficult, even when financial resources were available. Since permits for public infrastructure projects in the West Bank were managed by the Israeli Government, investment projects were delayed or even refused during the conflict period (World Bank 2008). All these factors contributed to overcrowded classrooms, creating a serious challenge to the Palestinian education system and to students’ learning (World Bank 2002; UNESCO 2011b).
Effect of conflict intensity on the quality of the school learning conditions.
. | Density in the classroom . | Students/teacher ratio . | Teachers’ average experience . | Average size of the classroom . | Percentage of Christians . |
---|---|---|---|---|---|
. | (1) . | (2) . | (3) . | (4) . | (5) . |
Number of fatalities | 2.66*** | |$-$|25.88*** | 12.14*** | 10.64 | 0.13 |
(0.50) | (6.77) | (3.77) | (8.15) | (0.13) | |
School-fixed effects | Yes | Yes | Yes | Yes | Yes |
Academic-year fixed effects | Yes | Yes | Yes | Yes | Yes |
Number of observations | 2651 | 2643 | 2649 | 2651 | 2651 |
Mean value of the dependent | 1.5 | 18.1 | 9.6 | 39.5 | 0.1 |
. | Density in the classroom . | Students/teacher ratio . | Teachers’ average experience . | Average size of the classroom . | Percentage of Christians . |
---|---|---|---|---|---|
. | (1) . | (2) . | (3) . | (4) . | (5) . |
Number of fatalities | 2.66*** | |$-$|25.88*** | 12.14*** | 10.64 | 0.13 |
(0.50) | (6.77) | (3.77) | (8.15) | (0.13) | |
School-fixed effects | Yes | Yes | Yes | Yes | Yes |
Academic-year fixed effects | Yes | Yes | Yes | Yes | Yes |
Number of observations | 2651 | 2643 | 2649 | 2651 | 2651 |
Mean value of the dependent | 1.5 | 18.1 | 9.6 | 39.5 | 0.1 |
Notes: OLS regression results. The dependent variable for each regression is reported in the first row of the table. Number of fatalities is the locality-level number of conflict-related Palestinian fatalities caused by the IDF during the academic year divided by 1000. Average Size of the Classroom is the average number of students per square meters in the classroom. Students/Teacher Ratio is the school-level ratio between the number of students and the number of teachers. Teachers’ Average Experience is the average number of teaching years of the teachers in the school. Average Density in the Classroom is the school-level average size of the classroom measured in square meters. Percentage of Christians is the school-level percentage of Christian students. In each regression, a constant is included but not shown. Robust standard errors in parenthesis are clustered at the locality level. ***Significant at 1%.
Effect of conflict intensity on the quality of the school learning conditions.
. | Density in the classroom . | Students/teacher ratio . | Teachers’ average experience . | Average size of the classroom . | Percentage of Christians . |
---|---|---|---|---|---|
. | (1) . | (2) . | (3) . | (4) . | (5) . |
Number of fatalities | 2.66*** | |$-$|25.88*** | 12.14*** | 10.64 | 0.13 |
(0.50) | (6.77) | (3.77) | (8.15) | (0.13) | |
School-fixed effects | Yes | Yes | Yes | Yes | Yes |
Academic-year fixed effects | Yes | Yes | Yes | Yes | Yes |
Number of observations | 2651 | 2643 | 2649 | 2651 | 2651 |
Mean value of the dependent | 1.5 | 18.1 | 9.6 | 39.5 | 0.1 |
. | Density in the classroom . | Students/teacher ratio . | Teachers’ average experience . | Average size of the classroom . | Percentage of Christians . |
---|---|---|---|---|---|
. | (1) . | (2) . | (3) . | (4) . | (5) . |
Number of fatalities | 2.66*** | |$-$|25.88*** | 12.14*** | 10.64 | 0.13 |
(0.50) | (6.77) | (3.77) | (8.15) | (0.13) | |
School-fixed effects | Yes | Yes | Yes | Yes | Yes |
Academic-year fixed effects | Yes | Yes | Yes | Yes | Yes |
Number of observations | 2651 | 2643 | 2649 | 2651 | 2651 |
Mean value of the dependent | 1.5 | 18.1 | 9.6 | 39.5 | 0.1 |
Notes: OLS regression results. The dependent variable for each regression is reported in the first row of the table. Number of fatalities is the locality-level number of conflict-related Palestinian fatalities caused by the IDF during the academic year divided by 1000. Average Size of the Classroom is the average number of students per square meters in the classroom. Students/Teacher Ratio is the school-level ratio between the number of students and the number of teachers. Teachers’ Average Experience is the average number of teaching years of the teachers in the school. Average Density in the Classroom is the school-level average size of the classroom measured in square meters. Percentage of Christians is the school-level percentage of Christian students. In each regression, a constant is included but not shown. Robust standard errors in parenthesis are clustered at the locality level. ***Significant at 1%.
Results shown in Table 5 also indicate that conflict intensity reduces Student/Teacher Ratio (column (2)) and increases Teachers’ Average Experience (column (3)). These results suggest that not all aspects of the school learning conditions worsen with an increase in conflict intensity. In fact, a lower student/teacher ratio is usually believed to improve academic achievement (Alderman et al. 2001). The same positive impact is expected from longer teacher experience, although typically studies have found that there is a positive effect for the first three years of experience and any additional year has little effect (Rivkin et al. 2005). Although there may be different explanations for these results, one possibility is that MOEHE compensated schools hit by violence by sending more teachers34 and teachers with more experience.35 Finally, conflict intensity does not affect the Average Size of the Classroom36 (column (4)) nor the composition of the student pool (at least in terms of religion), as measured by the Percentage of Christians in the School (column (5)).
Results shown in Table A.10 of Online Appendix A indicate that the probability of passing the exam is negatively correlated with Average Density in the Classroom (our proxy for difficulties in teaching and learning conditions in the classroom),37 whereas it is positively correlated with the Average Size of the Classroom (our proxy for quality of school infrastructures).38 Interestingly, the other two school characteristics that are affected by conflict (see Table 5), namely Student/Teacher Ratio and Teachers’ Average Experience, are not correlated with the probability of passing the exam. Finally, results show that the proxy for religious heterogeneity, that is, Percentage of Christians in the school, is not relevant for explaining academic achievement for students in the West Bank.
Reading these results together with those from Table 5 provides two interesting insights. First, the effect of conflict on exam results is attenuated when school learning conditions are controlled for. Indeed, when we include the full set of school characteristics in regression model (1), the coefficient for Number of fatalities becomes 0.45, which is 14% smaller than in the main specification (results available on request). Second, they indicate that, among all school characteristics, Average Density in the Classroom is the only one affected by conflict that is also correlated with the probability of passing the exam. We interpret this as suggestive evidence that the conflict-induced increase in classroom density is a possible transmission mechanism explaining the negative impact of the conflict on students’ results at the final exam. Our computations indicate the deterioration in school learning conditions can indeed account for 23% of the total effect of conflict intensity on the probability of passing the exam. Interestingly, this result is in line with the findings of reports and case studies showing that one of the major barriers to the provision of quality education in the OPT is the low quality of school infrastructures and classrooms (UNDP 2015).
This conclusion is reinforced by the results emerging from the analysis of the effect of school characteristics along the test score distribution. Quantile regression analysis (results available on request) reveals that the effects of Average Density in the Classroom and Average Size of the Classroom are significant only for students in the lower tail of the test score distribution. This suggests that those are the students for whom the quality of the learning environment at school is likely to be more important.
6.2. Changes in Students’ Psychological Well-Being
There is abundant evidence suggesting that the student’s psychological well-being is an important determinant of academic achievement (Roeser et al. 1998). In particular, previous research has documented the negative relationship between cognitive performance and exposure to violence (Wilson and Rosenthal 2003; Sharkey 2010). Youth directly or indirectly exposed to different forms of violence show reduced sleep, increased anxiety and difficulties concentrating, possibly leading to reduced academic performance (Pynoos et al. 1987; McGill et al. 2014; Gershenson and Tekin 2018). In fact, the psychological impact of the security conditions is an important element to be considered to understand the determinants of Palestinian students’ academic performance (World Bank 2006).
Although our data set does not allow for a direct test of these effects,39 in the following we provide indirect evidence for the possible role of the psychological mechanism in explaining our main result. As a first piece of evidence, we estimate model (1) using as a measure of conflict intensity the per-capita number of Palestinian fatalities at the locality level during the academic year.40 We use this measure to proxy for the intensity of the direct exposure to violence experienced by the student: the higher the value of the per-capita number of fatalities, the higher the probability that the student has been (physically) close to a violent event.
Results are shown in Table 6, column (1). Direct exposure to fatalities has a negative and highly significant effect on academic achievement. This can be interpreted as indicating that the more student is (or believes she is) likely to be the victim of a conflict event, the lower her academic performance is. Next, we consider the possibility that a process of self-identification by the student with young fatalities contributes to the effect of conflict intensity on academic performance. In column (2), we use as a measure of conflict intensity the per-capita number of young (less than 18-year-old) Palestinian fatalities in the locality during the academic year. Results show that the effect is highly significant and larger in magnitude than the effect of per-capita number of non-young Palestinians fatalities, with the difference between the two coefficients being statistically significant at 1%. Interestingly, these findings are in line with the psychological literature showing that the type of conflict-related violence to which the individual is exposed to is a key factor driving the incidence of both mental health disorders and violence-related trauma symptoms (RAND 2015).
Effect of direct exposure to conflict on the probability of passing the final exam.
. | Pass exam . | ||
---|---|---|---|
. | (1) . | (2) . | (3) . |
Per-capita number of fatalities | |$-$|12.44*** | ||
(4.51) | |||
Per-capita number of young fatalities | |$-$|88.22*** | ||
(25.56) | |||
Per-capita number of non-young fatalities | |$-$|1.73 | ||
(5.94) | |||
Per-capita number of Palestinians killed while not fighting | |$-$|16.37*** | ||
(6.19) | |||
Per-capita number of Palestinians killed (other) | |$-$|1.42 | ||
(11.69) | |||
Individual-level characteristics | Yes | Yes | Yes |
District-level economic conditions | Yes | Yes | Yes |
Birth-cohort fixed effects | Yes | Yes | Yes |
School-fixed effects | Yes | Yes | Yes |
Academic-year fixed effects | Yes | Yes | Yes |
Number of observations | 135,090 | 135,090 | 135,090 |
Mean of the dependent variable | 0.74 | 0.74 | 0.74 |
. | Pass exam . | ||
---|---|---|---|
. | (1) . | (2) . | (3) . |
Per-capita number of fatalities | |$-$|12.44*** | ||
(4.51) | |||
Per-capita number of young fatalities | |$-$|88.22*** | ||
(25.56) | |||
Per-capita number of non-young fatalities | |$-$|1.73 | ||
(5.94) | |||
Per-capita number of Palestinians killed while not fighting | |$-$|16.37*** | ||
(6.19) | |||
Per-capita number of Palestinians killed (other) | |$-$|1.42 | ||
(11.69) | |||
Individual-level characteristics | Yes | Yes | Yes |
District-level economic conditions | Yes | Yes | Yes |
Birth-cohort fixed effects | Yes | Yes | Yes |
School-fixed effects | Yes | Yes | Yes |
Academic-year fixed effects | Yes | Yes | Yes |
Number of observations | 135,090 | 135,090 | 135,090 |
Mean of the dependent variable | 0.74 | 0.74 | 0.74 |
Notes: OLS regression results for equation (1). The dependent variable is Pass exam, a dummy that takes value 1 if the student has passed the final exam and 0 otherwise. Per-capita number of fatalities is the per-capita number of Palestinian fatalities caused by IDF at the locality level computed by dividing the number of fatalities at the locality level occurred during the academic year by the locality-level population in 1997. Per-capita number of Palestinians killed (other) include all those killed excluding those that were not fighting, that is, those fighting and those for which the status is unknown. See the text for additional details on the variables construction. Individual characteristics and District-level economic controls are the same as in Table 3. In each regression, a constant is included but not shown. Sample is smaller than in Table 2 because of missing values for the variable Population at the locality level in 1997. Robust standard errors in parenthesis are clustered at the locality level. ***Significant at 1%.
Effect of direct exposure to conflict on the probability of passing the final exam.
. | Pass exam . | ||
---|---|---|---|
. | (1) . | (2) . | (3) . |
Per-capita number of fatalities | |$-$|12.44*** | ||
(4.51) | |||
Per-capita number of young fatalities | |$-$|88.22*** | ||
(25.56) | |||
Per-capita number of non-young fatalities | |$-$|1.73 | ||
(5.94) | |||
Per-capita number of Palestinians killed while not fighting | |$-$|16.37*** | ||
(6.19) | |||
Per-capita number of Palestinians killed (other) | |$-$|1.42 | ||
(11.69) | |||
Individual-level characteristics | Yes | Yes | Yes |
District-level economic conditions | Yes | Yes | Yes |
Birth-cohort fixed effects | Yes | Yes | Yes |
School-fixed effects | Yes | Yes | Yes |
Academic-year fixed effects | Yes | Yes | Yes |
Number of observations | 135,090 | 135,090 | 135,090 |
Mean of the dependent variable | 0.74 | 0.74 | 0.74 |
. | Pass exam . | ||
---|---|---|---|
. | (1) . | (2) . | (3) . |
Per-capita number of fatalities | |$-$|12.44*** | ||
(4.51) | |||
Per-capita number of young fatalities | |$-$|88.22*** | ||
(25.56) | |||
Per-capita number of non-young fatalities | |$-$|1.73 | ||
(5.94) | |||
Per-capita number of Palestinians killed while not fighting | |$-$|16.37*** | ||
(6.19) | |||
Per-capita number of Palestinians killed (other) | |$-$|1.42 | ||
(11.69) | |||
Individual-level characteristics | Yes | Yes | Yes |
District-level economic conditions | Yes | Yes | Yes |
Birth-cohort fixed effects | Yes | Yes | Yes |
School-fixed effects | Yes | Yes | Yes |
Academic-year fixed effects | Yes | Yes | Yes |
Number of observations | 135,090 | 135,090 | 135,090 |
Mean of the dependent variable | 0.74 | 0.74 | 0.74 |
Notes: OLS regression results for equation (1). The dependent variable is Pass exam, a dummy that takes value 1 if the student has passed the final exam and 0 otherwise. Per-capita number of fatalities is the per-capita number of Palestinian fatalities caused by IDF at the locality level computed by dividing the number of fatalities at the locality level occurred during the academic year by the locality-level population in 1997. Per-capita number of Palestinians killed (other) include all those killed excluding those that were not fighting, that is, those fighting and those for which the status is unknown. See the text for additional details on the variables construction. Individual characteristics and District-level economic controls are the same as in Table 3. In each regression, a constant is included but not shown. Sample is smaller than in Table 2 because of missing values for the variable Population at the locality level in 1997. Robust standard errors in parenthesis are clustered at the locality level. ***Significant at 1%.
Finally, we construct a measure of conflict intensity looking at the characteristics of the fighting status of the killed. In column (3), we proxy conflict intensity using the per-capita number of Palestinians killed by the IDF while not taking part in the hostilities.41 The negative impact of conflict intensity as measured by those killed while not fighting is negative, highly significant and larger than the effect of other fatalities (those killed while fighting or unknown status). Yet, we cannot reject the null hypothesis that the two coefficients are equal.
To provide additional evidence supporting the psychological mechanism, we look at the impact of the direct exposure to conflict at various points in time during the academic year. In particular, we are interested in the effect of conflict events occurring very close in time to the date of the exam. The basic idea is that—if significant—the negative effect of these events on student’s exam result would be difficult to explain by a worsening in the quality of the school learning environment.42 Instead, the effect would be consistent with the direct exposure to conflict negatively affecting the student’s psychological well-being and—in turn—the performance at the final exam.
To this end, we take advantage of the information on the exact date of each fatality included in the B’Tselem data set and compute the per-capita number of fatalities occurred in each locality 1 month before the exam, 2 months before the exam and so on, up to 9 months before the exam. Then, we re-estimate our main regression model (1) including all these measures of direct exposure to conflict at the same time. This allows us to provide a detailed characterization of how the effect of direct exposure to conflict varies depending on the specific moment at which it occurs with respect to the date of the exam.
Figure 1 depicts the estimated coefficients and the corresponding confidence intervals for the per-capita number of fatalities at the locality level in each month. Results indicate that direct exposure to conflict events in any of the months of the academic year has a negative effect on the probability of passing the final exam. Yet, the magnitude of the effect is small and not precisely estimated in most cases. Interestingly, the effect is significant and the largest for the conflict events occurring 1 month before the exam. Although events occurring earlier also seem to matter (see for instance the coefficient of the lag at 6 months), we interpret the large negative effect associated with being exposed to conflict-related violence shortly before the exam as providing support for the psychological mechanism.
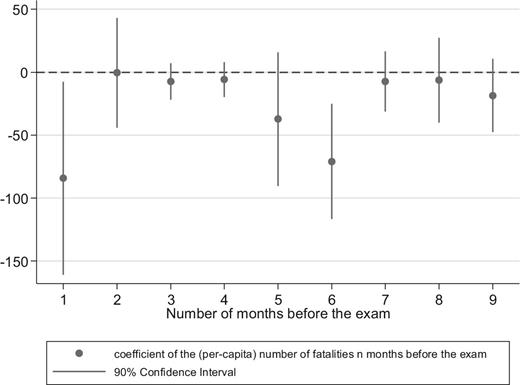
Effect of direct exposure to conflict on the probability of passing the final exam by months before the exam. Dependent variable is the probability of passing the final exam (Pass exam). The Figure plots the estimated coefficients for direct exposure to conflict in the nth month before the exam, as measured by the per-capita number of fatalities caused by IDF n (with n = 1, …, 9) months before the exam at the locality level. The solid vertical lines show the 90% confidence interval of each estimate, whereas the dashed horizontal line indicates zero.
These results are in line with psychological research that has documented conflict exposure as the origin of high levels of emotional and behavioral problems and of psychological disorders in adolescents in the OPT (Dubow et al. 2009; Mataria et al. 2009; Thabet et al. 2002). Palestinian adolescents exposed to conflict-related violence report higher levels of depression and mental disorders, Post-Traumatic Stress Disorders (PTSD) symptoms (Al-Krenawi et al. 2007; Abdeen et al. 2008; RAND 2015) and an increase in symptoms like restlessness, fear, anxiety, communication difficulties and a reduced ability to concentrate on their studies (UNRWA 2003; Arafat and Boothby 2003; PCBS 2004; Save the Children 2004). Pedersen et al. (2001) report that heavy shelling and attacks directly affect students’ concentration on schoolwork and makes final exam preparation more difficult. Giacaman et al. (2007) find that the experience of conflict-related violence is a strong predictor of depression-like states that in turn may affect academic performance.43 Taken together, this evidence supports the view that the psychological channel plays a role in explaining the negative effect of the conflict on the probability of passing the final exam.
One difference between the psychological mechanism and the school-quality mechanism is that, although the latter is geographically localized, the former is much less so. Young Palestinians are exposed to conflict-related violence not only through direct or indirect experience but also through intense media coverage. As reported by Save the Children (2003), children often talk about conflict-related incidents seen on television. In addition, it is common for images of Palestinians killed to be displayed in public places, including school walls (Sultana 2006). Since the West Bank is a small geographical area and there are more than 48 private radio and television stations, all the information related to the conflict and the political situation rapidly and fully spreads among the population (European Union 2006). The intense media coverage of the conflict is likely to impact on students’ psychological well-being. Gvirsman et al. (2014) show that higher levels of exposure to media covering violent events related to the conflict are associated with higher levels of PTSD symptoms in young Palestinians. The larger this media effect is, the more likely our results are to represent a lower bound of the effect of conflict on academic achievement.
6.3. Other Possible Mechanisms
6.3.1. Days of School Closure
Another possible mechanism explaining our main result could be the negative effect of conflict intensity on the quantity of learning time at school, the latter being an important determinant of students’ academic performance. Anecdotal evidence indicates that during the Second Intifada the Palestinian Authority closed schools and interrupted the normal schooling activities for security reasons.44 Often, these decisions were the response to episodes of occupation of schools by the IDF or to situations of conflict-related violence around the schools (MOEHE 2005; UN-OCHA-OPT various issues).
To test the effect of the reduction in learning days due to conflict on students’ performance at the final exam, we use confidential data provided by the MOEHE on the percentage of school days lost due to school closures for security or military reasons. Unfortunately, these data suffer from two limitations. First, data are available only at the district level. Second, data are not available for the whole period under analysis. In particular, there are no data for the 2000–2001 and the 2001–2002 academic years. We estimate the impact of conflict intensity on students’ learning time by regressing Percentage of days lost due to closure of school (i.e., the district-level percentage of days lost due to closure of schools for security/military measure) on district-level conflict intensity, controlling for district-level economic conditions and district- and year-fixed effects. Table A.11 of Online Appendix A shows that conflict intensity increases the percentage of school closure days, that is, it reduces the number of learning days at school.
Next, we check the possible role of Percentage of days lost due to closure of school as a mechanism behind our main result. To this end, we include it in regression (3) together with the school characteristics discussed in Section 6.1. Results shown in Table A.12 of Online Appendix A report that its coefficient is negative, suggesting that a reduced number of days of school is associated with a lower probability of passing the exam. Yet, the effect is not significant at conventional levels.45 Interestingly, the negative effect of Average Density in the Classroom on Pass exam remains highly significant and its magnitude does not change with respect to that reported in Table A.10 of Online Appendix A.
6.3.2. Student Absenteeism
Conflict may reduce academic achievement by increasing student absenteeism (World Bank 2006; Giacaman et al. 2007). Unfortunately, the possibility of testing this mechanism in our setting is limited by the absence of either individual-level or school-level information on student absenteeism. To gain some insights into the possible role of student absenteeism in explaining our main result, we use confidential data provided by the MOEHE on the district-level percentage of students who lost days of school due to the conflict. Yet, these data cover only two academic years (2000–2001 and 2001–2002). Results indicate a positive correlation (0.4, significant at 1%) between district-level conflict intensity and percentage of students who lose days of schools, that is, suffering a reduction in learning days. However, the number of observations is very small and one should thus be cautious in interpreting this evidence. Finally, we note that according to MOEHE high school regulations, students enrolled in high school who have been absent for more than 30 days during the academic year without justification are not allowed to take the final exam (Nicolai 2007). This implies that students with high absenteeism are not included in the MOEHE data set we use in our main analysis.
6.3.4. Quality of Teaching and Teacher Absenteeism
Another channel through which conflict may reduce students’ academic performance is via its effect on teachers (Hanushek and Woessmann 2011, Chap. 2). There is abundant anecdotal evidence that the conflict affected teachers and teaching activities in the West Bank. Teachers had to cope with repeated rights violations and the negative psychological impact of pervasive violence in their lives (Nicolai 2007). As reported by a school principal in Pedersen et al. (2001, p. 18): “several of our teachers have martyrs and injured in their families”. Giacaman et al. (2002) note that teachers face problems related to frequent class interruptions, cancellation of school days, increases in workload and management of traumatized children. Although there are few doubts that conflict affects daily teacher activities, Giacaman et al. (2002) argue that the effect of conflict on teaching quality is not obvious in the context of the Second Intifada. There is some evidence showing that the difficult situations experienced by the teachers had both positive and negative effects on the quality of their teaching. In fact, although some teachers reported being chronically exhausted with a decline in their performance, others experienced a sense of increased commitment, covering other delayed or absent teachers and providing special care to the students (Nicolai 2007).
Previous research has shown that teacher absenteeism—by reducing the quantity of learning time—is another possible mechanism behind the negative effect of conflict and violence on education (Justino, Marinella and Salardi 2014; Monteiro and Rocha 2017). Unfortunately, MOEHE does not collect individual or school-level data on teacher absenteeism. The only existing data are the district-level percentage of teachers losing at least one day of school for military reasons. These data are available only for two years (2000–2001 and 2001–2002). Results indicate a positive correlation (0.3, significant at 10%) between district-level conflict intensity and the percentage of teachers missing days of school. However, since we have observations only for two years, we cannot include this variable as an additional control in our regression analysis of the impact of school-level characteristics on academic achievement (equation (3)).
Although not conclusive, our evidence is line with the case studies reported in Save the Children (2003) showing that teachers faced severe difficulties in accessing schools during the Second Intifada. In fact, the type and range of problems teachers experience because of the conflict are not different from those reported by other Palestinian workers: delays in reaching the workplace because of checkpoints, risk of being exposed to shootings, and so forth. Yet, the education sector is reported to have encountered the fewest problems with absenteeism among all public sectors (World Bank 2007a).
Taken together, these results seem to indicate that there is some evidence that conflict intensity is associated with more school closure days and more student and teacher absenteeism. Yet, severe limitations on data availability do not to allow us to establish robust empirical relationships between these variables and student performance at the exam. Therefore, we cautiously interpret the overall evidence on these mechanisms as inconclusive.
6.3.5. Parental Distress
Finally, we consider as a potential mechanism the fact that conflict may negatively affect the well-being of parents, causing them—in turn—to have a negative influence on their children's academic performance. Several psychological studies suggest the possibility that adults experiencing conflict-induced stress modify their behavior toward their own children. Ayer et al. (2017) argue that nearly all psychological studies looking at the Israeli–Palestinian conflict find that individuals more exposed to violence are more likely to be unable to carry out normal functions related to personal relationships. Thabet et al. (2008) and Srour (2005) document that exposure to war trauma impacts on both parents’ and children’ mental health and that their emotional responses are inter-related.
Although these studies suggest the possible existence of a parental distress channel affecting student academic performance, we cannot test this hypothesis with our data. Yet, as long as parental exposure to conflict can be proxied by direct exposure to conflict experienced by the student, we argue that this mechanism is captured by the effect of the per-capita number of fatalities on the probability of passing the final exam, as discussed in Section 6.2.
7. Concluding Remarks
In this paper, we analyze the impact of violent conflict on the academic achievement of high school students. In particular, we study the effect of the Second Intifada on the individual results of the high school leaving exam (Tawjihi General Examination) for the whole population of Palestinian students enrolled in the Adabi (Literary) and Elmi (Scientific) streams in the West Bank during the period 2000–2006.
Our results show that conflict reduces the probability of passing the final exam, the total test score and the probability of being admitted to university. We also document that the magnitude of the effect of conflict varies along the test score distribution and with the timing and the type of violent events a student is exposed to.
Several transmission mechanisms could explain our findings. First, we show that the conflict negatively affects the quality of the school learning environment and that this in turn negatively correlates with results on the final exam. Second, we provide suggestive evidence consistent with a psychological mechanism contributing to the explanation of our main result. In particular, we show that direct exposure to conflict has a large negative impact on the probability of passing the exam and that the effect is the largest for conflict events occurring shortly before the exam date. Together, these results indicate that the conflict has a negative impact on exam results by affecting both the learning process and performance at the exam. The fact that the two mechanisms we identify have very distinct characteristics implies that effective education policies to mitigate the negative effects of conflict on academic achievement need to account for each of them.
Finally, we note that our findings indicate that the negative effect of conflict on academic achievement may also have long-lasting consequences. By reducing the probability of students successfully completing their high school studies and of being admitted to university, conflict may significantly hinder human capital accumulation and economic development. This is a potential long-term cost to be added to the several other already documented negative consequences of the Israeli–Palestinian conflict.
Acknowledgments
We thank the Editor (M. Daniele Paserman) and three anonymous referees for their constructive comments and suggestions. We thank the Palestinian Ministry of Education and Higher Education (MOEHE) for providing us with the data on exam results. We thank Applied Research Institute of Jerusalem for the superb help with the barriers’ data. We thank Francesco Amodio, Muhammad Asali, Sonia Bhalotra, Massimiliano Cali, Luca de Benedictis, Francesco Drago, Belal Fallah, Nathan Fiala, Roberto Galbiati, Olaf de Groot, Kati Krähnert, Vincenzo Lombardo, Paolo Naticchioni, Roberto Nisticò, Giovanni Prarolo, Paola Salardi, Olga Shemyakina, Eik Swee, Chiara Toglia, Asaf Zussman, Noam Zussman, the participants at the XII Jan Tinbergen Conference, the 8th HiCN Conference, and seminar participants at GIGA (Hamburg) and at Humboldt University (Berlin). Sami Miaari thanks the European Union Seventh Framework Programme under grant agreement no. 218105 (EUSECON) for funding his stay at DIW Berlin while he was working on this project. Sami Miaari also acknowledges that this project has received funding from the Horizon 2020 research and innovation programme under the Marie Sklodowska-Curie grant agreement No. 752410. All errors are our own.
Notes
The editor in charge of this paper was M. Daniele Paserman.
Footnotes
The First Intifada—the name given to the first Palestinian uprising against the Israeli occupation of the Palestinian Territories—lasted from December 1987 until 1993.
For a description of the different periods of violence during the Second Intifada, see Jaeger and Paserman (2008).
The education system is divided into three levels: elementary school (grades 1–6), middle school (grades 7–10), and high school (grades 11 and 12). For a detailed analysis of the Palestinian education system, see Nicolai (2007).
One difference between the two streams of study is that graduates holding the Elmi Tawjihi Certificate may apply for all majors at the university, whereas graduates with the Adabi Tawjihi Certificate are limited in their choice to majors taught in the faculties of arts, economics, business administration, and law.
The upward trend in enrolment that characterizes the period 2000–2006 mirrors the increase in population growth in the decade before the Second Intifada (Nicolai 2007; World Bank 2007b; UNICEF 2010). World Bank (2002) reports that the average population growth rate before the Second Intifada was about 4% per year, one of the highest in the world, with about half of the population being under 15 years old. We discuss this issue in detail in Section 4.
As for comparison, the percentage of secondary enrolment in 2006 for Egypt is 81 and for Lebanon 77, whereas the average for Arab countries is 67 (UNESCO 2007).
The locality is the smallest administrative unit used by the PCBS. There are 610 localities in the West Bank.
See Section 6.1 for the definitions of these variables.
Data on fatalities for the Israeli–Palestinian conflict are available at this link: http://www.btselem.org/statistics.
Previous studies on the Israeli–Palestinian conflict used a variety of variables to proxy conflict intensity: number of closure days, number of Palestinian suicide bombings in Israel, and so forth. With respect to those, the locality-level number of Palestinian fatalities provides a significantly more accurate measure of individual exposure to conflict intensity (see Brück et al. 2016).
The Second Intifada began on September 29, 2000 but there is no official end to it. Although some indicate 2005, the violence continued on both sides throughout 2006. In fact, the number of Palestinian fatalities in 2006 was three times that of 2005 (B’Tselem 2007). Since our results are not affected by this choice (results available upon request from the authors), we also include year 2006 in our analysis.
The MOEHE data set does not include information on the socioeconomic condition of students. Although this is a limitation of the data set, we argue that since our analysis focuses on students enrolled in nonmandatory high school, this information is somehow less relevant than for other studies. In fact, the variance in household income for these students is likely to be much smaller than is the case for, for example, primary school students.
To the best of our knowledge, the only other study looking at the effect of conflict on high school completion is Swee (2015). In the case of the Bosnia–Herzegovina War, a one standard deviation increase in the casualty rate reduces the probability of completing high school by 3.8 percentage points. This larger effect is due to the fact that those who do not enroll or dropout during the academic year are also included among noncompleting individuals.
First-time takers should be 18 years old at the exam. In our sample, 3% of students are 17 years old at the exam. We define first-time exam takers as the students that are 17 or 18 years old at the day of the exam. Data show that, although 25% of students take the exam at least one year later than expected, only 4% of students take the exam more than two years later than expected.
Another reason why conflict may increase the number of low-ability students in high school is that it may induce parents to keep them in school as a way of protecting them from violence. Although this may be a common strategy in other conflict-affected environments, this is not the case for the West Bank. In fact, the conflict situation made travelling between home and school risky and difficult. For example, MOEHE reports 132 students killed and 2,500 injured while traveling to or from school in 2003. Schools were also not safe places: during the Second Intifada, schools suffered attacks and were occupied by the military. Akesson (2014) reports that students felt unsafe at school and parents were often reluctant to send children to school because commuting was considered dangerous.
These results are consistent with the evidence reported in UNICEF (2010) that the primary reasons for dropping out after compulsory schooling years are the need to work to help the family and for early marriage.
Economic variables are computed at the district level because the PLFS does not report the locality of the individual.
We interpret this variable as a proxy for students’ opportunity costs of being in school.
As suggested by Rodriguez and Sanchez (2012), being a dropout or failing the final exam may increase rebellion in adolescents in a context of violent conflict. In turn, this may contribute to increase the number of demonstrations, the level of violence and, possibly, the number of the consequent conflict-related fatalities.
World Bank (2007a, p. 1) reports that “administrative restrictions, rooted in military orders associated with the occupation of the West Bank […] are used to restrict Palestinian access to large segments of the territories […]. Permit policies limit the freedom of Palestinians to […] move about outside of their municipal jurisdiction”.
The possibility of school choice within localities is limited. MOEHE data indicate than 76% of localities have less than four high schools with only very large localities having a larger number of schools.
Since we use school-fixed effects and are clustering errors at the locality level, the fact that including previous-year conflict intensity does not affect the magnitude and significance of Number of fatalities suggests that our main result is robust to the threat of serial correlation.
One of the distinctive features of the Israeli–Palestinian conflict is the presence of restrictions imposed by the IDF on the mobility of goods and people within the OPT as well as across the border with Israel. As for internal mobility restrictions, these include checkpoints, roadblocks, and physical barriers between villages within the OPT. Cali and Miaari (2018) present suggestive evidence that the placement of internal mobility restrictions is exogenous to the evolution of the Palestinian labour market and motivated by military-security consideration by the IDF.
The weight captures the idea that the more distant a mobility obstacle is (taking into account the road system) the less it affects mobility to and from the locality. For additional detail on the index, see Cali and Miaari (2018).
The basic idea here is that for a given number of barriers, the larger the population of a locality the more time-consuming it would be to pass the barriers due to the larger number of people to be checked by the IDF.
See Online Appendix B for details on how the Total test score is computed for the Adabi and the Elmi streams, respectively.
These results are of comparable magnitude to those from previous studies looking at the impact of conflict and violence on academic achievement, even if referred to samples and school grades different from ours. Monteiro and Rocha (2017) look at elementary schools in Brazil and report a 0.010 standard deviation reduction in math test scores in schools exposed to drug-related violence. Instead, the effect of violence on reading test scores is never significant. Kibris (2015) looks at the university admission test in Turkey in 2005 and finds that each casualty is associated with a 0.014 and 0.013 points decrease in the math and Turkish test scores, respectively. In our case, each fatality reduces the math test and the Arabic test score by 0.05 and 0.02 points, respectively. Finally, Gershenson and Tekin (2018) show that primary schools within a five miles radius of a sniper attack have a reduction between 2% and 4% in the school-level pass rate in math. Computing a similar measure for our analysis, we find that a one standard deviation increase in the number of fatalities leads to a 2% reduction in the probability of passing the math part of the exam.
In fact, quantile coefficients are not significantly different at conventional levels among them but for the 95th quantile.
Conflict intensity does not influence the stream (Adabi vs. Elmi) or the type of school (government vs. private) the student enrols in. There is no evidence that the conflict has a differential effect on the probability of passing the exam in either of the two streams, whereas its effect is larger and it is significant only for students in government schools.
Additional regressions using the MOEHE data set (available upon request) show that the conflict reduces the Number of classrooms and the Size of the school flat, even if both effects are not significant at conventional levels.
The number of students in the OPT has been constantly increasing since the creation of the national curriculum with the 1994 Oslo Agreements. In fact, overcrowding was also a problem before the Second Intifada due to school enrolment growing at the rate of 2% annually and serious difficulties in obtaining construction permits in the OPT from Israel (Sultana 2003). The conflict, by damaging existing schools and reducing the number of permits to expand them, has probably exacerbated an already existing problem.
The conflict-induced decrease in the Student/Teacher Ratio is indeed the result of the combination of the (nonsignificant) effect of conflict on the number of high school students (negative, see Section 4) and on the number of teachers (positive, results available upon request).
An alternative interpretation for the increase in the Teachers’ Average (years of) Experience are the difficulties faced by the MOEHE in recruiting fully qualified staff during the Second Intifada (Save the Children 2003). In this case, a higher Teachers’ Average (years of) Experience would not proxy for higher teaching staff capabilities to prepare the students for the exams, but it would more likely capture an increasingly older teaching staff.
Note that the result that conflict intensity does not affect the Average Size of the Classroom does not contradict our explanation of the effect of conflict on Average Density in the Classroom. The fact that the conflict destroys some classrooms (or makes them unusable due to damage) does not imply that the average size of the existing classrooms needs to change as well. Moreover, the two measures capture different aspects of the quality of the school: whereas Average Size of the Classroom can be interpreted as a proxy for the quality of school-level infrastructures, Average Density in the Classroom is instead a proxy for the quality of the teaching and learning conditions in the classroom.
Although the literature on the relationship between overcrowding and student learning is quite limited (McMullen and Rouseb 2012), there is some evidence that overcrowding can have an adverse impact on learning. Rivera-Batiz and Marti (1995) show that in New York public schools between 2% and 9% fewer students pass reading and mathematics proficiency tests in overcrowded schools compared to students in nonovercrowded schools.
The per-capita number of fatalities at the locality level is computed dividing the number of fatalities at the locality level occurred during the academic year divided by the locality-level population in 1997, which is the only pre-Second Intifada year for which population data at the locality level are available.
B’Tselem defines Palestinians killed by the IDF who was not taking part in the hostilities as people not participating directly in hostilities at the time they were killed and were not fulfilling a continuous combat function. Examples taken from the B’Tselem data set are: [name omitted] 32 years old, killed near Ramallah by gunfire when hiking with relatives on hills. Without warning, soldiers opened fire at them from about 500 m away. [name omitted] 15 years old, killed near Kfar Dan by gunfire. Shot in the back by Border Police who passed by in a jeep. Testimony given to B’Tselem indicates the street was quiet and the officers were not in danger. [name omitted] 25 years old, killed near Nablus by gunfire while serving customers in his butchery.
Conflict-induced changes in the quality of the school learning environment take time to materialize. Moreover, it is unlikely that those school-level changes would immediately affect students’ academic performance.
Pedersen et al. (2001) report the interview of a school principal saying: “When the students come to school they are very often afraid, sometimes they have not slept because there has been shooting or bombing during the night […]. Sometimes the Israeli soldiers shoot after the students when they go to school […].”
When we control for Percentage of days lost due to closure of school in our main regression (1), the effect of the Number of fatalities is reduced by 4%. The coefficient for Percentage of days lost due to closure of school is negative but not significant (results available upon request). Note that when we control for Percentage of days lost due to closure of school we lose 31% of our observations because of missing values. In this restricted sample, the coefficient for the Number of fatalities is twice as large as in our baseline regression (−1.15, significant at 1%).