-
PDF
- Split View
-
Views
-
Cite
Cite
Wang Liao, Natalya N Bazarova, Y Connie Yuan, Unpacking Medium Effects on Social Psychological Processes in Computer-mediated Communication Using the Social Relations Model, Journal of Computer-Mediated Communication, Volume 23, Issue 2, March 2018, Pages 90–106, https://doi.org/10.1093/jcmc/zmy002
- Share Icon Share
Abstrat
This article introduces the social relations model (SRM) to unpack medium effects on social psychological processes. Although such effects have been theorized at group, dyadic, and individual levels separately, some of them can be concurrently analyzed as medium moderation on SRM components. Drawing on existing theories of computer-mediated communication (CMC), we map SRM components onto social psychological processes susceptible to medium effects: (a) “group mean” and “consensus” onto group social integration, (b) “uniqueness” and “dyadic reciprocity” onto relationship development, and (c) “assimilation” and relations between ingroup and outgroup SRM components onto social identity processes. We also summarize the SRM analytics and scenarios of medium moderation, and illustrate the key analytical procedures for adopting the SRM for CMC research.
Research in computer-mediated communication (CMC) has identified various medium effects of information and communication technologies (ICTs) on common social psychological processes in social interactions (e.g., Siegel, Dubrovsky, Kiesler, & McGuire, 1986; Spears, Lea, & Lee, 1990; Walther, 1992, 1996). Such effects are typically conceptualized at the level of individual, dyad, or group, separately; and there is limited understanding of how they coexist in CMC. Such separate treatments have, to some extent, contributed to the “gross theoretical pluralism” of competing theories that explain similar outcomes with discordant mechanisms (Walther, 2009, p. 749). In addition to the theoretical discord, the CMC field faces ecological validity challenges, as daily interactions collide multiple social processes and blur boundaries between different ICTs, along with face-to-face (FtF) interactions. By and large, there is a need to examine how the medium, or tool(s), “with which we can shape our interactions” (Sundar, Jia, Waddell, & Huang, 2015, p. 49), interplays with multilevel processes jointly in CMC.
Consider, for example, how strangers interact in a text-based chatroom. Research has identified two distinct mechanisms through which this CMC environment can moderate their social dynamics: an individual-level, social identity-based process of group conformity and polarization (Spears et al., 1990); or a dyadic-level, relationship-based process looping between selective self-presentation and heuristic impression formation (Walther, 1992, 1996). Despite abundant support (see Walther, 2011) and some side-by-side comparisons (e.g., Postmes, Spears, Lee, & Novak, 2005), little research has studied the two mechanisms jointly.
To further complicate the dynamics, the strangers may belong to a team with various emergent properties (e.g., common fate, solidarity, and hierarchy [Moreland, 1987]) that are also likely to be susceptible to medium effects. This raises questions about how medium can affect group processes together with individual and dyadic processes in CMC.
To bridge disparate theoretical accounts at multiple levels of analysis, we propose an analytical approach based on the social relations model (SRM) (Kenny, 1994; Kenny & La Voie, 1984), a multilevel model with built-in theoretical interpretations for several common social psychological processes underlying interpersonal behaviors or perceptions. On par with ideas about interdependences of social processes and technologies (e.g., Fulk, Steinfield, Schmitz, & Power, 1987; Poole & DeSanctis, 1992; Walther, 1992), the proposed approach treats medium as a moderator of social psychological processes underlying interpersonal perceptions or behaviors.
In particular, the SRM can advance CMC research through mapping of its components onto existing conceptions of CMC grounded in social identity (Spears et al., 1990), relationship development (Walther, 1992), and group processes (Siegel et al., 1986). Moreover, although the original SRM assumes a single communication environment, it can incorporate different media as a categorical moderator. Medium effects can then be identified through contrasting medium uses (e.g., channels, technological features, ways of using ICTs, as well as FtF communication) on the SRM components that reflect interpersonal behaviors or perceptions.
The rest of this article aims to explicate connections between the SRM and previous conceptions of CMC (also illustrated in Figure 1). Brief introductions to the machinery of SRM and its intergroup variation (the ISRM) are offered (see for more detailed treatments, Kenny, Gomes, & Kowal, 2015; Kenny, Kashy, & Cook, 2006; Kenny & La Voie, 1984), along with potential scenarios of medium moderation. We summarize the key analytical procedures of using the SRM for CMC research with an empirical example (data and codes can be accessed online1).
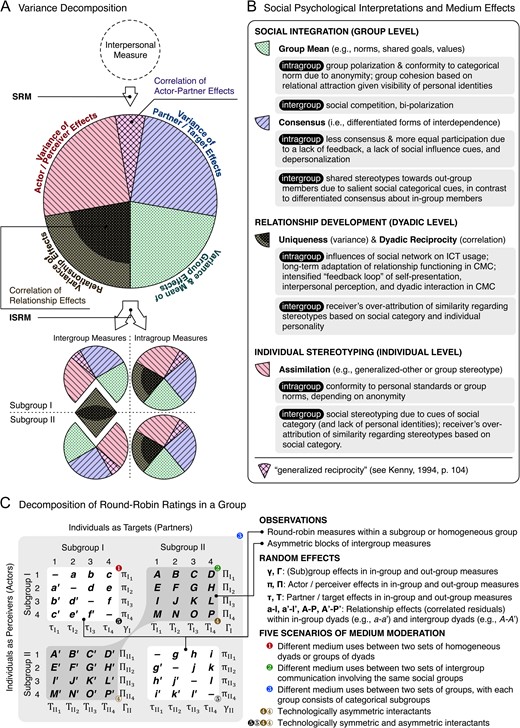
Conceptual illustrations of the SRM variance decomposition. A illustrates variance decomposition by SRM and ISRM. B summarizes the corresponding social psychological interpretations and related medium effects (in gray blocks). C illustrates the decomposition of interpersonal (round-robin) ratings in a 8-person group with two nominal subgroups, as well as the loci of potential medium moderation.
Social psychological conceptions of CMC through the lens of the SRM
Social interactions present a complex interplay of situational and dispositional influences. The SRM decomposes their effects on interpersonal behaviors or perceptions in at least five ways2 (referred to as SRM components per Kenny, 1994): At the group level, there are: (a) shared “group-mean,” and (b) “consensus,” likely emergent from social integration via group processes (Moreland, 1987). At the dyadic level, there are: (c) “uniqueness,” and (d) “dyadic reciprocity” of distinct relationships, induced by relationship development. At the individual level, there is: (e) “assimilation” that reflects stereotyping in social behavior and cognition (Kenny, 1994, p. 40). Another layer of complexity is added by distinguishing these components between ingroup and outgroup (Kenny et al., 2015) in intergroup situations. The next sections explicate these components in relation to CMC theorizations at the corresponding levels of analysis.
Teamwork in CMC and social integration
Early CMC research set out to discover the effects of technological characteristics in teamwork (see for review, Culnan & Markus, 1987). Collectively known as the “cues-filtered-out” approach (Culnan & Markus, 1987; Walther, 2011), these studies examined how cues (e.g., non-verbal behaviors) filtered out in CMC interactions could reduce the sense of social presence and awareness of social context. These early theories received mixed support (see for review, Walther, 1996), but there were consistent findings about medium effects at the group level: Text-based CMC enhanced equality of participation across group members but suppressed reaching of agreement, compared to FtF interactions (Kiesler, Siegel, & McGuire, 1984; Siegel et al., 1986). These two effects can be conceptualized as group properties emergent from social integration (Moreland, 1987).
Social integration refers to the process of developing “groupness” or “strengthening of the bonds among persons” (Moreland, 1987, p. 81) along environmental (i.e., being physically or socially close), behavioral, cognitive, and affective dimensions. Such integration takes at least two forms: similarity and differentiated interdependence. The SRM component, “group mean” (Kenny et al., 2006, p. 227), characterizes similarity among group members’ interpersonal behaviors and perceptions. For example, when group members converge in interpersonal liking, the group mean reflects unique affective characteristics of the group, along with a small within-group variation. As within-group variation reduces, differences in interpersonal liking across multiple groups are increasingly attributed to characteristics of each group, that is, as a between-group variation, which hence indicates the extent of such affective integration (sometimes referred to as “cohesiveness” by Kenny et al., 2015, p. 153). This between-group variation may also reveal group-level medium effects, for example, when some groups make decisions in FtF situations, while others use group decision support systems (e.g., Poole & DeSanctis, 1992).
Social integration also entails differentiated interdependence among group members (e.g., a consensus about differentiated roles in a group, Moreland, 1987). More equal participation in text-based CMC compared to FtF (Siegel et al., 1986) is an example of medium effects on such differentiated interdependence, that is, medium use altering status differentiation—a typical form of differentiated interdependence in groups (Ridgeway, 2001). In interacting groups, the SRM can capture such forms of interdependence, reflecting a “shared reality or understanding” (Ervin & Bonito, 2014, p. 607). Kenny (1994, p. 50) uses the terms, “consensus,” to refer such differentiated interdependence, as elaborated later.
Thus, by capturing similarity and differentiated interdependence, the SRM can reveal related medium effects on social integration. Furthermore, the SRM may also help to explain the mixed support for the cues-filtered-out approach by separating group-level medium effects from dyadic- and individual-level effects.
Relationships in CMC and dyadic processes
CMC research has a rich repertoire of relational perspectives. One of the most well-known perspectives is Walther’s (1992) social information processing (SIP) theory, which conceptualizes text-based CMC as relationally constraining, but only in the short-term. In the long-term, people adapt to constraints of a medium, guided by uncertainty reduction and relationship development motivations (Berger & Calabrese, 1975). They can strategically exploit CMC affordances by selectively presenting themselves and stereotypically construing others, thus engaging in an intensifying “loop” of relationship development that can even alter the nature of a relationship (i.e., to make it “hyperpersonal,” see Walther, 1996). Beyond a single medium, the use of multiple media also impacts relationship development and maintenance (i.e., media multiplexity, Haythornthwaite, 2005).
At the dyadic level, we propose that medium effects on relational processes correspond to changes in “uniqueness” and “dyadic reciprocity” components in the SRM (Kenny, 1994, pp. 82, 109). Uniqueness refers to the extent to which individuals interact differently across social contacts (e.g., lover vs. coworker, or friend vs. acquaintance)—a basic condition to distinguish relationship-based processes from general dispositions towards others. Uniqueness itself, however, does not warrant reciprocity, as in the case of unrequited love (Kenny, 1994). Dyadic reciprocity, on the other hand, is critical for relationship development (Berger & Calabrese, 1975). A hyperpersonal “loop” between a sender’s self-presentation and a receiver’s perception of him or her (Walther, 1996) exemplifies such reciprocity reshaped by CMC. Similar effects may be extended to other forms of reciprocity: conversational reciprocity (Berger & Calabrese, 1975) and mimicry or complementarity (see Sadler, Ethier, & Woody, 2011), to name a few.
In short, if indeed medium affects relationship-based processes, as theories predict (Walther, 1992, 1996), such effects will be revealed in changes to uniqueness or dyadic reciprocity. Moreover, the SRM also reveals such dyadic-level effects in relation to medium effects at other levels, including not only those related to group social integration as described before, but also medium effects on individual-level processes, such as social identity processes.
Self in CMC and social identity
Another departure from the cues-filtered-out approach is the social identity model of deindividuation effects (SIDE) (Reicher, Spears, & Postmes, 1995) based on social identity theory and self-categorization theory (Tajfel & Turner, 1986; Turner, Hogg, Oakes, Reicher, & Wetherell, 1987). According to SIDE, anonymous CMC triggers depersonalization and in turn evokes social self-categorization: a shift in self-perception from “I” to “we,” contingent on the presence of a salient social identity. This shift is responsible for group conformity and polarization in single-group anonymous CMC (Spears et al., 1990), and for categorical stereotyping and bi-polarization in intergroup CMC (Lea, Spears, & de Groot, 2001).
On a more general level, social identity processes often entail three intergroup schemas—intergroup accentuation (cognitive assimilation per social category), ingroup favoritism, and social competition (Brewer, 2010). Conceptually, the most relevant SRM component to intergroup accentuation is “assimilation,” which refers to the extent to which a person tends to treat all others similarly based on his or her idiosyncrasies, resembling stereotyping or categorization. Thus, it is tempting to use assimilation as an indicator of intergroup accentuation, one of the intergroup schemas mentioned above. However, assimilation in the basic form of the SRM can draw from either general perceptual tendencies of other people (“generalized-other”) or from group stereotypes (Kenny, 1994, p. 45), making this SRM component conceptually ambiguous.
A cleaner way to study intergroup accentuation, ingroup favoritism, and social competition would be through an ingroup and outgroup comparison (i.e., with the ISRM, Kenny et al., 2015). Then assimilation, along with other SRM components, could be broken down by the ingroup/outgroup situation to: (a) draw a comparison, or (b) estimate an association between intragroup and intergroup perceptions and behaviors. For example, the comparison between ingroup and outgroup assimilation would capture the extent to which outgroup members are treated more homogeneously compared to ingroup members (Boldry & Kashy, 1999; i.e., “outgroup homogeneity,” see Kenny et al., 2015). Meanwhile, ingroup favoritism and social competition can be studied as the association between ingroup SRM components (not limited to assimilation) and its outgroup counterparts for perceptions or behaviors that carry emotional significance or value (e.g., liking or gift-giving). For example, a negative association between ingroup and outgroup assimilation in liking of other members suggests ingroup favoritism.
By distinguishing between ingroup and outgroup components, the SRM allows examination of SIDE predictions about the interplay of medium and social identity. Researchers, however, must be guided by analytical assumptions, because SRM components may be tied to multiple theoretical mechanisms and needs to be interpreted in light of a study’s research design. The next section outlines the SRM’s analytics in homogeneous and heterogeneous dyads and groups (i.e., distinguished by social categories), and how medium effects can be incorporated.
Statistical realization of the SRM and the moderating effects of medium
The SRM decomposes a single variable (or multi-item construct) of reciprocal measures into a set of variance components. Rather than their realized effects, the variance–covariance parameters of these components are the analytical focus (Kenny, 1994). Among SRM designs (see Kenny & La Voie, 1984, p. 150), we introduce the “round-robin design,” which covers all SRM components; and the “block-round-robin design,” which distinguishes between ingroup and outgroup situations for these components in groups consisting of two nominal subgroups.
Round-robin SRM for homogeneous groups
The parameters of these effects have theoretical interpretations. First, among a population of groups, variance of group effects (group mean), i.e., , indicates the extent to which non-independence is due to shared characteristics of each group (e.g., “norms, shared goals, and values,” Kenny et al., 2006, p. 228). Second, the population mean describes the nature (e.g., valence) of such characteristics (e.g., liking). The interpretation of these two parameters as indicating social integration assumes a group with a local history or interaction opportunities, instead of a nominal group with non-interacting similar members (Kenny & Judd, 1986).
Variance of target effects (, i.e., consensus) taps into the second aspect of social integration—differentiated interdependence—as it jointly depends on: (a) agreement among perceivers regarding every target, and (b) differentiation across targets. To interpret it as an indicator of differentiated interdependence also requires the assumption of a group’s local history or interaction, and may only be meaningful with measures that are typically differentiated as a result of group processes (e.g., status). Without interaction, target variance may merely reflect perceivers’ accurate judgements about the targets. However, Kenny (1994, p. 63; Kenny & La Voie, 1984, p. 177) and others (Ervin & Bonito, 2014) argue that such an asocial explanation is inadequate in interacting groups because interaction can bring about emergent properties that render individuals’ cognitions and behaviors interdependent. In this case, targets are the “person-as-situation,” meaning that interacting individuals become a situational force of their own that reshapes one another’s interpersonal behaviors or perceptions (Kenny & La Voie, 1984, p. 177). If differentiated interdependence emerges in this way, the target variance will be altered.
Next, variance of the perceiver effect captures assimilation based on the extent to which: (a) each perceiver rates all targets similarly, and (b) different perceivers have different perceptual tendencies towards the same target. As discussed earlier, there is an interpretative ambiguity regarding the perceiver variance. When a group is homogeneous, perceiver variance indicates the “generalized-other” assimilation, defined as “how a person sees other people in general” (Kenny, 1994, p. 40). A high level of such assimilation is what the cues-filtered out perspective would predict in a CMC environment, that is, interpersonal perceptions and behaviors become idiosyncratic because a perceiver would treat all CMC partners similarly based on his/her idiosyncrasies (see for review, Walther, 2011). However, such assimilation would decrease with a salient local group identity in CMC, as members’ perceptions of one another start to conform to shared group stereotypes, as SIDE predicts (Lea et al., 2001). Kenny (1994) also points out, “if all perceivers share the same stereotype, then the perceiver effect does not reflect a person’s stereotype of the group; the stereotype is reflected only in the group mean.” (p. 45). The above-mentioned ambiguity can be reduced when distinguishing assimilation towards ingroup versus outgroup, as discussed in the next section.
Finally, variance of the relationship effects and their within-dyad correlations correspond to uniqueness and dyadic reciprocity, as also discussed above. Relationship variance captures perception differences across dyads, revealing the extent to which people distinguish between their personal contacts and relationships. The magnitude of the correlation coefficient reflects the degree to which a given measure is positively or negatively reciprocated in dyads, thus suggesting relational coordination and interdependence.
Block-round-robin SRM for categorically heterogeneous groups
In a block-round-robin design (e.g., the ISRM), people interact with not only ingroup peers, but also those from another subgroup, yielding four blocks of measures, as illustrated in Figure 1c: The two diagonal “symmetric blocks” resemble two round-robin SRMs for ratings within Subgroup I and II (in lowercase), while the two off-diagonal “asymmetric blocks” (Kenny et al., 2006, p. 205) contain ratings across subgroups (in uppercase). Thus, the variance components quadruple, and covariation is also allowed across blocks (see the formulas in note3, also Kenny et al., 2015).
The increased complexity comes with benefits. As mentioned before, the difference and correlation between an ingroup SRM component and its outgroup counterpart indicate intergroup accentuation, and ingroup favoritism or intergroup competition. This approach considers intergroup relation as moderating SRM components. For example, a difference in “group mean” between perceptions towards ingroup and outgroup members suggests intergroup accentuation with potential social integration among ingroup members. Dyadic idiosyncrasy and reciprocity can also be compared, such that individuals may treat their relationships within their subgroups differently from their personal relationships across subgroups. This treatment of intergroup relation is hence critical for integrating some of the CMC theories (e.g., SIP and SIDE) because social identity processes do not necessarily compete with group and dyadic processes.
Medium moderation
Although the SRM was designed for a single communication environment, it can be extended to study medium effects as a categorical moderator between two media (more than two media can be studied through pairwise comparisons). There are two classes of medium moderation. The first moderates at the dyadic level, when two interactants use the same medium within encounters. Medium moderation can thereby occur either between different dyads that use different media, or within the same dyad that uses different media across encounters as in mixed-media relationships (Parks, 2017) or modality switching (Ramirez, Sumner, Fleuriet, & Cole, 2015). The second class consists of medium moderation at the level of conversational turn-taking, when two or more people use different media within encounters (i.e., as discontinuity in “cross-turn coherence,” Herring, 1999). The temporal and dyadic boundaries of encounters in this case also blur, especially when asynchronous media are involved.
These two classes can be further broken down into at least five scenarios, depending on how medium moderation is applied to the round-robin or block-round-robin design (also see Figure 1c). There are three scenarios at the dyadic level. First, based on the round-robin SRM, different media are used across dyads consisting of otherwise homogeneous interactants, or by same dyads across different episodes of interaction (e.g., FtF interactions in the office and CMC for remote collaboration). In these situations, medium effects are captured as differences in SRM components between two sets of interactants or as longitudinal changes in these components. Second, moderating the asymmetric blocks in the block-round-robin design, different media are involved in two sets of intergroup behaviors or perceptions. For example, CMC between students of two social groups can be compared with FtF communication between either the same students or students from the same populations, while intragroup measures are not analyzed. Third, when both intra- and intergroup measures in the block-round-robin design (i.e., the ISRM) are considered (e.g., combining the above two scenarios in a social group by medium factorial design), both in- and outgroup components of the ISRM can be moderated by medium, yielding more comprehensive medium effects in intergroup situations.
There are two scenarios of medium moderation at the level of conversational turn-taking. The fourth scenario captures the use of two media by the same pair of people within episodes of interaction. This is possible when two people interact on the same digital platform via different terminals. For example, messages sent from the Facebook mobile app are read and replied to from the desktop website or the virtual reality environment of Facebook. Such a medium asymmetry can be represented as the two asymmetric blocks in a block-round-robin design, similar to how the ISRM treats intragroup measures. Finally, the fifth scenario extends the fourth by adding two symmetric blocks, thus intersecting multiple people and multiple media. For example, Facebook users’ mobile interactions with each other (i.e., symmetric blocks) can be juxtaposed with their interactions with other users from the virtual reality environment of Facebook (i.e., asymmetric blocks). It is noteworthy that the fifth scenario also relaxes the need for medium asymmetry, as it only requires individuals interacting with two sets of partners, each via a different medium (e.g., teammates in a local office interacting with each other via FtF, and with remote partners via videoconference). Put simply, the last two scenarios resemble the second and third scenarios based on the ISRM. However, unlike the ISRM, the “subgroups” here are distinguished not by social identity (e.g., classes of cadets or fandoms of football teams, as studied by Boldry & Kashy, 1999; Kenny et al., 2015), but by their medium use.4
These five scenarios of medium moderation on different SRM components can instigate many research possibilities. There are a few common analytical procedures needed for a successful adoption of the SRM for CMC research, as we illustrate below.
Key analytical procedures and an empirical example
Step 1: Conceptualize reciprocal measures and social context
As so far discussed, a theme in CMC research is the interplay between technology and social structures or processes that are jointly embodied in interactions. It is important that a researcher is well-versed in, and ideally controlling, the nature of social processes implied in the measures and social context when investigating medium effects.
To illustrate how the SRM can be applied to analyze the medium effects, we used a dataset collected from 51 four-person groups (n = 204). In each group, two Chinese students (one male and one female) and two American students (one male and one female) worked on a group task to solve several intellectual problems together. All American students were non-Asian (87% self-identified as Caucasian, 6% African American, 4% Hispanic, 1% Native American, and 2% “other”). Participants were randomly assigned to FtF or CMC (a text-based chatroom) groups.
We decomposed medium effects on interpersonal perceptions, particularly ratings of warmth and competence across the two nationality subgroups, resembling the second scenario of medium moderation as described before. Both perceptions have multilevel implications. At the individual level, people are likely to stereotype other social groups based on warmth and competence (Cuddy, Fiske, & Glick, 2007). At the group level, the similarity of perceived warmth can emerge as a result of affective integration (Moreland, 1987), whereas status hierarchy in a group relies on differentiated interdependence in terms of competence perceptions (Ridgeway, 2001). Finally, at the dyadic level, perceived warmth and competence may indicate the development of bonding and hierarchical relationships (Sadler et al., 2011).
Several procedures were undertaken to encourage multilevel processes as described above. To encourage group processes, participants were asked to discuss and collectively solve several analytical problems, following the collective decision-making paradigm in previous CMC studies (e.g., Siegel et al., 1986). There was also a collective reward, a $30 gift certificate, awarded to each member of the five top-performing groups, with the aim of incentivizing teamwork. Having different nationalities (American and Chinese members) in each group presented participants with salient social categories that could trigger social identification and categorization processes. CMC participants placed in different rooms were instructed to take photos of their faces, which remained visible in their chatroom accounts throughout the interaction, thus making nationality salient. Finally, to help participants interpersonally relate to each other, they were asked to share their real names with one another; in CMC interactions participants’ names were visible in their chatroom accounts throughout the discussion.
Step 2: Assess measurement validity and reliability of SRM variance components
There are two potential validity issues of the SRM critical to CMC research. First, in the SRM with a single-item construct, relationship variance is confounded with measurement error, which is problematic for analyzing relational processes in CMC. Second, the ISRM of categorically heterogeneous groups may face a lack of measurement invariance between them, which is problematic for testing SIDE predictions. The ISRM compares latent variables (i.e., SRM components), but they can be incomparable if their measurement structures differ between the given social groups. For example, intergroup difference in assimilation between Chinese and Americans could be an artifact of cultural differences in understanding measurement instruments between the two nationality groups rather than a product of social group stereotypes. The solution to both issues is measuring a construct with multiple items, which allows for: (a) separation of measurement errors from the relationship component (Kenny, 1994, p. 241), and (b) assessment of measurement invariance across groups, without which (at least metric invariance, i.e., identical factor structure and loadings, see Vandenberg & Lance, 2000) an intergroup difference in variance between latent variables is not meaningful.
Using a multi-item construct also makes it possible to separate between stable and unstable portions for each of the four SRM variances (Kenny, 1994, p. 241)—a method to improve measurement reliability of the SRM variance components.5 For example, the stable portion of perceiver variance refers to the commonality of the assimilative tendency across all measured items, whereas its unstable portion absorbs the remaining inconsistency across these items in measuring each perceiver’s assimilative tendency. The comparisons for medium effects should be only performed on the stable portions of all SRM components because an unstable portion represents a measurement error for the corresponding SRM component.
Thus, the analysis of medium moderation via the SRM ideally requires multi-item constructs. In our example, participants were asked to rate each other’s warmth using three items: “unpleasant–pleasant,” “unlikable–likable,” and “unfriendly–friendly.” Competence was rated using “uninformed–informed,” “unintelligent–intelligent,” and “incompetent–competent.” Only intergroup ratings (i.e., asymmetric blocks) were analyzed, because participants made two intergroup ratings (for the two members of a different nationality) but only one intragroup rating (for one other member of the same nationality). This fits the second scenario described earlier.
An SRM analysis can be overcomplicated when incorporating multi-item construct, subgroup, and medium moderation in a single statistical model. Thus, we recommend a two-step approach to separate the assessment of measurement quality (Step 2) from the test of hypotheses (Step 3). For Step 2 in our example, we ran a multigroup confirmatory factor analysis (CFA) to test measurement invariance between American members’ ratings and Chinese members’ ratings across all groups. The CFA was specified with a factor structure adapted from Kenny’s parameterization that distinguishes between stable and unstable SRM components for a multi-item construct (1994, p. 241). The only adaptation is that factor loadings were not assumed to be equal in order to make it possible to assess metric invariance between the two subgroups. This type of CFA can be estimated as a structural equation model (SEM), as per Olsen and Kenny (2006) (see our R[lavaan] or MPlus codes for the model specification and testing).
For both warmth and competence, we first estimated a configural invariance model, which freed all parameters across the nationality subgroups (see Vandenberg & Lance, 2000). The models fitted data well: For warmth, χ2(40) = 44.95 (p = .272); and for competence, χ2(40) = 36.08 (p = .647). Then we estimated metric invariance models by constraining factor loadings of the stable SRM components to be equal across the subgroups. For warmth, the model fitted data as well as the configural invariance model: χ2(42) = 45.59 (p = .325) and Δχ2(2) = .65 (p = .729). For competence, the model fitted data well, χ2(42) = 44.87 (p = .353), but worse than the configural invariance model, Δχ2(2) = 8.79 (p = .012), suggesting that the two subgroups interpreted the construct differently. Chinese participants’ conception of competence loaded heavier on the “unintelligent–intelligent” item compared to Americans, Δχ2(1) = 8.65, p = .003. We thus estimated a partial metric invariance model to allow for this variation. This model fitted data as well as the configural invariance model, χ2(41) = 36.22 (p = .683) and Δχ2(1) = 1.31 (p = .252). In sum, it is safe to interpret intergroup differences in SRM components for warmth given the metric invariance between the two subgroups; but for competence, such differences should be interpreted with caution. Factor scores, which were predicted from the stable SRM components of the metric invariance model for warmth and the partial metric invariance model for competence, are ready to be used to in the next step.
Step 3: Estimate SRM components and test medium effects
SRM can be estimated using method of moments (e.g., SOREMO and BLOCK by Kenny, 1998; and TripleR by Schönbrodt, Back, & Schmukle, 2012), maximum likelihood methods (SEM by Olsen & Kenny, 2006; multilevel model by Snijders & Kenny, 1999), and Bayesian methods (Lüdtke, Robitzsch, Kenny, & Trautwein, 2013). Currently, we consider the maximum likelihood methods better suited for studying CMC because they are more flexible than the method of moments for concurrent analysis of categorical moderation and block designs (e.g., the ISRM); they are also more familiar to social scientists compared to Bayesian methods.
In the example, we modified Kenny et al.’s (2015) multilevel model (implemented via the MIXED procedure in Statistical Analysis System, SAS) to estimate the asymmetric blocks of the ISRM with a CMC moderation, for both warmth and competence factor scores from the CFA models described above (see our SAS codes for model specification and testing; these may also be done via other packages for multilevel modeling or SEM). The model estimates SRM components for the intergroup ratings, along with asymptotic standard errors for testing variance estimates against zero—typically required for an SRM analysis. Medium effects can be tested by constraining parameters of an SRM component in CMC and FtF groups to be equal, and then testing changes in deviance scores (ΔG2, Kenny et al., 2015). Bootstrapped confidence intervals (CIs) can also be obtained for differences between CMC and FtF groups, offering another way to test medium effects, especially for parameters that may not be easily constrained, such as correlation (e.g., dyadic reciprocity).
Once estimated, SRM components are often interpreted in terms of both raw variance and relative variance (i.e., effect size), as summarized in Figure 2. First of all, there is more between-group variation (subgroup variance) of Americans’ ratings of Chinese members’ perceived warmth in CMC than FtF groups, with ∆G2(1) = 5.88, p = .015. Correspondingly, the within-group variation (perceiver, target, and relationship variance together) in CMC groups are reduced among Americans (see their relative portions in Figure 2b), suggesting similarity of intergroup perception. In particular, Chinese members were rated as less warm by Americans (subgroup mean) in CMC (5.34, SE = .14) compared to FtF (5.99, SE = .13), p < .001. Such a more homogeneous view towards outgroup members in CMC compared to FtF (in our data towards Chinese members by Americans) is consistent with SIDE’s predictions.
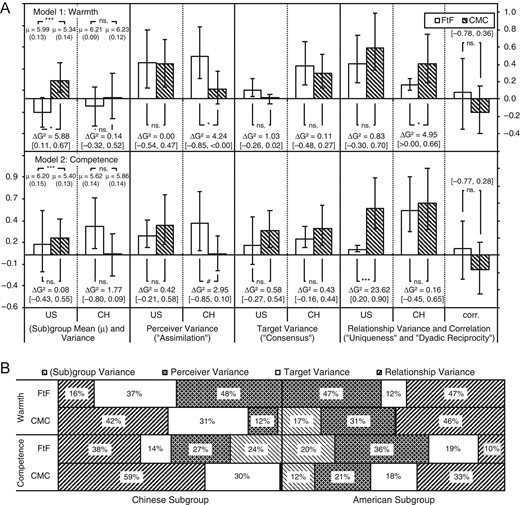
Variance estimates. In Panel A, CH and US indicate Chinese and American participants, respectively. Error bars cover 90% CIs for variance (ɑone-tailed = .05) and 95% CIs for correlation. Data labels include (sub)group means (i.e., μ; SE in parenthesis) and tests of medium effect (i.e., ΔG2 of equality constraint with df = 1 and 95% CIs of the difference). All CIs are bootstrapped (bias-corrected and accelerated, n = 5,000). ***p < .001, **p < .01, *p < .05, #p < .1. In Panel B, relative variance is calculated against total variance in asymmetric blocks.
Second, contradicting standard SIDE predictions, Chinese participants in text CMC were less assimilative (perceiver variance) in their perceptions towards the Americans, particularly in perceived warmth, ∆G2(1) = 4.24, p = .039. This reduction in assimilation appears to be counterbalanced by an increment in the Chinese participants’ dyadic uniqueness (relationship variance) in warmth perception towards Americans in CMC compared to FtF, ∆G2(1) = 4.95, p = .026. A similar increment in dyadic uniqueness is also observed in Americans’ competence perceptions towards Chinese participants in CMC compared to FtF, ∆G2(1) = 23.62, p < .001. The increased dyadic uniqueness is consistent with SIP such that the participants in CMC tend to form interpersonal perceptions per unique dyads, instead of social categories. However, we did not observe dyadic reciprocity in warmth and competence perceptions, as their CIs cover zero (see Figure 2a).
Overall, this example illustrates the key procedures needed for adopting the SRM for CMC research. As summarized in Figure 2b, this analysis has allowed us to decompose variances of warmth and competence into relative portions attributable to subgroup, perceiver, target, and relationship effects. Their distributions are not equal across FtF and text CMC, revealing joint medium effects on relational and social identity-based processes.
Discussion
The unique contribution of the SRM lies in its potential to probe interpersonal behaviors and cognitions as a synthesis of individual, relational, and group influences (Kenny & La Voie, 1984). Despite its prior recognition as a promising tool for studying interpersonal phenomena in CMC (e.g., Walther, 2011, p. 460), and some infrequent applications to online interactions (e.g., Markey & Wells, 2002), there has been no account of how the SRM can be conceptually aligned with CMC processes and effects. This article attempts to fill this gap. The proposed approach offers a way to bridge CMC theories and enables a holistic understanding of how medium can affect individual, relational, and group mechanisms concurrently, but to different degrees. Furthermore, the SRM can help articulate conceptual boundaries of existing CMC theories when applied to more naturalistic settings where such boundaries often overlap. By revealing common mechanisms in applied research, the SRM could also offer better theoretical guidance for technology design and understanding user practices.
Although we see much potential in applying the SRM to CMC, it is not intended in any way to be a standalone, meta-theoretical framework. Instead, we hope our introduction of the SRM will stimulate creative adaptations along with dedicated conceptualization and research designs by CMC scholars. We have a few general suggestions to this end. One research direction could investigate how competing mechanisms for the same social outcome may coexist under certain medium uses or ICTs. For example, the SRM can give a more comprehensive understanding of group cohesion in CMC by revealing how social identity and relational processes may coexist and complement one another, in contrast to research that highlights distinct conditions for each mechanism (cf., Postmes et al., 2005). Medium effects on more complicated social identity processes can also be examined; for example, how medium use alters outgroup homogeneity (Boldry & Kashy, 1999) or the differentiation between “ingroup love” and “outgroup hate” (Brewer, 2010). In a nutshell, previous CMC conceptions can be used as building blocks for more complicated explanations of CMC through the lens of the SRM.
Second, with a careful understanding of assumptions, the SRM can be flexibly used to inspect more naturalistic data. Such data can include observable social or organizational behaviors in CMC (e.g., social media data streams), which are increasingly accessible to researchers. Situational factors (or social context effects, Fulk et al., 1987), including but not limited to intergroup relations, can be incorporated as moderators in the SRM. Furthermore, repeated decomposition of interpersonal measures with the SRM across time can foster understanding of relational and group properties emergent from social interaction; joining multiple SRMs of different interpersonal measures (e.g., via SEM) can address more complicated research questions involving multivariate relations.
Third, medium moderation deserves special attention. Such moderation consists of ideally randomized (or quasi-randomized) contrasts across media, which take on new meanings in today’s blurred boundaries between media modalities (Baym, 2009) and a surge of mixed-media relationships (Parks, 2017). Medium moderation in media-rich environments can be identified: (a) as the discontinuity in interactional coherence (Herring, 1999), (b) at the moment of modality switching (Ramirez et al., 2015), (c) within the media multiplexity effects on relationship (Haythornthwaite, 2005; Ledbetter, 2010), and (d) at the intersections of multiple media and multiple relationships in social networks (Parks, 2017). These different types of medium moderation are currently open questions, and the SRM can help to answer some of them via several scenarios described before, combined with longitudinal interpersonal data.
Although we have limited the treatment of CMC processes to the levels of individual, dyad, and group, higher-level medium effects could also be investigated through the SRM. For example, medium effects in relation to culture or institution could be explored by comparing people from different cultural or institutional backgrounds, or having them interact in different ICTs. The SRM may also be applied to interactions between corporate actors (e.g., firms), although theoretical re-interpretations of SRM components are needed.
The unique potential of the SRM can also be revealed when compared to other analytical approaches to studying social processes in CMC and ICTs. First, CMC and ICTs can be studied as various types of covariate effects in a generic multilevel model (e.g., within-/between-group effect, contextual effect, or cross-level interaction, see Snijders & Bosker, 2011). However, a multilevel model requires a dedicated conceptualization of these effects and is often used to only adjust for, not to interpret, non-independence in observations. In contrast, the SRM builds on social psychological interpretations of non-independence and thus brings theoretical insights into what often remains hidden in the practices of multilevel modeling. We believe modeling socially-induced non-independence is a key to comprehending socio-technological interdependencies (Rice, 2009), or how medium effects intertwine with social processes in CMC.
Medium effects can also be studied as pseudo-common method factors in multitrait-multimethod (MTMM) models of multimodal communication (see Ledbetter, 2010). Like multilevel modeling, this approach demands dedicated conceptualization of research questions. It also explicitly treats non-independence, although such a treatment is typically applied to individual measures, in contrast to the SRM’s focus on interpersonal measures. Thus, the MTMM approach may work better for studying medium effects on individual traits, including perceptions of relationship, as well as their within-individual changes across time.
Finally, CMC and ICTs can also be studied as edges and nodes in multidimensional networks mixing human and nonhuman agents (Contractor, 2009), modeled via statistical network analyses (see Snijders, 2011). Similar to the SRM, such models address non-independence in dyadic data, and some of the basic models “can be viewed as logistic versions of the SRM” (see Kenny et al., 2006, p. 314). However, network models often focus on structures and processes beyond interpersonal dynamics (Snijders, 2011). Some scholars suggest the multidimensional networks and related analytics may be suitable for studying CMC and ICTs as “organizing forces related to, or forms of, the organizing processes” (Rice, 2009, p. 718), which typically operate at levels higher than the SRM’s focus.
Conclusion
Despite its promise, it is important to recognize some limitations of the proposed applications of the SRM to CMC (for general limitations of the SRM see Kenny & La Voie, 1984, pp. 173–175 and Kenny, 1994, p. 213). One obvious limitation has to do with conceptual and practical difficulties of obtaining meaningful dyadic data and applying the SRM analytics. This requires a researcher to: (a) focus on common social psychological processes underlying CMC, (b) conceptualize them in the language of non-independence via SRM components, and (c) find the right places to identify medium effects from observed interpersonal behaviors or perceptions. Thus, although the SRM can help advance CMC research, it requires non-trivial efforts in conceptualization, research design, and data analysis. Finally, the SRM is not suitable or optimal for the analyses of individual behaviors and higher-level social processes, for which we recommend the aforementioned alternative approaches.
To conclude, the SRM is a useful method for analyzing medium effects in CMC. It has the potential to reveal concurrent multilevel medium effects on social psychological processes and connect different CMC theorizations. We hope scholars will adopt and make creative use of it for understanding communication in contemporary media-rich environments.
Acknowledgment
This research was supported in part by the National Science Foundation (IIS-1405634). We appreciate the constructive feedback and insightful comments from the three anonymous reviewers and the associate editor. We would also like to thank Jessie Taft for her careful editorial assistance.
Notes
Another component, “generalized reciprocity,” which technically refers to the correlation between perceiver (actor) effects and target (partner) effects, is not the focus of ours as it can be induced by different mechanisms at multiple levels (see Kenny, 1994, pp. 104–109).
The block-round-robin SRM (e.g., ISRM) can be formulated as follows where in group k, subject i from either subgroup I or II rates ingroup subject j and outgroup subject j:
It is possible that social identification occurs as individuals interacting with two sets of partners, each residing in a different medium environment (e.g., local office vs. remote teamwork). In this case, medium effects and social identity-based effects can confound each other in a block-round-robin design. Additional research designs are needed to address this issue.
This is different from the reliability of random effects separately estimated or predicted from each of the random variables behind SRM components. See Bonito and Kenny (2010) for details about this kind of reliability, which is important for studies that use effect estimates of the SRM.
References
Author notes
Accepted by Joseph Walther