-
PDF
- Split View
-
Views
-
Cite
Cite
Miriam J Metzger, Christo Wilson, Ben Y Zhao, Benefits of Browsing? The Prevalence, Nature, and Effects of Profile Consumption Behavior in Social Network Sites, Journal of Computer-Mediated Communication, Volume 23, Issue 2, March 2018, Pages 72–89, https://doi.org/10.1093/jcmc/zmx004
- Share Icon Share
Abstract
This study examines the effects of profile browsing on social network sites (SNSs) on social capital via information propagation between users. We analyze data from a study of 42 million users of the Chinese equivalent of Facebook called Renren, with over 1.8 million profile browsing events collected unobtrusively from the network to understand the prevalence and nature of “passive” profile browsing versus more visible forms of social interaction. Results show that profile browsing is more frequent than visible interaction on the SNS and can be modeled on the basis of a user’s network size, account longevity, and production or reception of visible content. Drawing upon scholarship on social capital, we then evaluate the capacity of profile browsing to propagate information within the network and thus to affect bridging social capital. Our results challenge some commonly-held notions about profile consumption behavior on SNSs and its capacity to increase social capital.
Understanding user behavior in social network sites (SNSs) is important because SNSs present both risks and benefits to users. A good deal of the research to date has focussed on the risks associated with SNS use, for example, privacy risks, threats to children, and damage to reputation or relationships (see boyd & Ellison, 2007; Ellison & boyd, 2013), but benefits of SNS use, including opportunities for social support and social capital, well-being, collective action, and learning, have also received attention in the literature. A common assumption across this research is that vulnerability to various risks and opportunity for benefits can be traced to increased SNS use.
Although most research has focussed on connecting general SNS use to these outcomes, studies have begun to recognize that different uses of SNSs may have different consequences for users in terms of social risks and benefits (see Antheunis, Vanden Abeele, & Kanters, 2015; Burke, Kraut, & Marlow, 2011; Ellison, Steinfield, & Lampe, 2011). Regarding types of use, Ellison et al. (2011) found three types of communication behaviors employed by Facebook users: using the SNS to initiate new relationships, to maintain existing relationships, and to seek information about social contacts that users have an offline connection to. Burke et al. (2011) distinguished more active uses of Facebook, including “directed communication” with specific, targeted network members and “broadcast communication” which involves posting content to all linked friends on the network, from mere consumption of others’ broadcasted information (e.g., via profile browsing). Similarly, Jiang et al. (2010) divided SNS use into “visible” interaction (i.e., writing posts and comments on others’ profile wall) and “latent” interaction (i.e., profile browsing without leaving a visible or written trace).
The majority of the literature on the social effects of SNS use focuses on active or “visible” uses of these networks. A much smaller proportion of studies examine “passive” forms of SNS use, including profile browsing.1 Among the earliest, Burke et al. (2011) measured users’ consumption of social news (i.e., reading friends’ status updates or looking at their profile photos) via Facebook server logs. Ellison et al. (2007, 2011) surveyed Facebook users about browsing friends’ and strangers’ profiles. While these studies provide valuable data, Burke et al. were only able to examine profile consumption behavior between linked friends, and self-report data such as that used by Ellison et al. (as well as most others), may underestimate profile browsing due to stigma about being a “Facebook stalker.” Thus, questions remain about the nature of profile consumption behavior and its effects on SNS users. The present study fills this void by investigating the prevalence, nature, and effects of profile browsing. In addition, this study introduces a new method of observing profile browsing and examines unobtrusive behavioral data from millions of user interactions to answer the research questions.
The prevalence and nature of profile consumption behavior in SNSs
The first question this study seeks to answer is the prevalence of profile consumption behavior in SNSs, defined here as profile browsing, compared to other forms of SNS use. Interestingly, different perspectives lead to divergent predictions regarding the prevalence of profile browsing. For example, on one hand, we might expect profile browsing to be less frequent than more active or visible communication behavior (i.e., writing comments on another user’s profile or broadcasting content for others to read) because users might rather take advantage of SNSs’ seemingly primary functionality, which is to visibly communicate with friends rather than to browse other users’ profiles without visibly interacting with them. Supporting this, Ellison et al. (2007, 2011) and others indeed find that people report using Facebook to stay in touch with friends and acquaintances substantially more than using it to meet new people. It may be the case that browsing strangers’ profiles is rare because most users’ privacy settings block strangers from seeing their full profile (Fiesler et al., 2017), which could suppress motivation for random profile browsing since so little information can be obtained.2
Yet other perspectives suggest that reading friends’ or strangers’ profile information and status updates may be more frequent because doing so requires less effort than do more active or visible forms of interaction. Burke et al. (2011), for example, argue that the ease of merely consuming profile content may prompt users to engage in it. They explain that composed (i.e., written) communication, including private messages, wall posts, or comments, require more effort to produce than simpler behaviors such as “one-click” actions (e.g., “Likes”) or reading a status update or viewing a photo. Their over-time data show a higher incidence of consumption of broadcasted information compared to directed communication in Facebook. Moreover, as mentioned earlier, social desirability biases inherent to survey methods may underestimate profile consumption behavior (also called “lurking” or “Facestalking”) and so more research that does not rely on self-reports is needed to understand the frequency of profile browsing versus more active forms of SNS use. Thus RQ1 asks, based on behavioral data:
RQ1: How prevalent is profile browsing compared to visible interaction in a SNS?
Beyond a simple understanding of prevalence are related questions of what predicts profile browsing behavior and who engages in it? Certain features of a SNS profile or user behavior on the network might prompt “indegree” profile browsing by attracting visitors to view a users’ profile.3 For example, users who maintain an active presence on the SNS by adding or updating their profile content frequently, including status updates, diary entries, photos, and shared links to content on the web, may attract more frequent profile visitors because they supply fresh content for people to consume, and thus a recurring reason for others to view their profile. The number of comments that users receive on their profiles from others should indicate a greater number of views as well, as viewing a user’s profile is a prerequisite of leaving comments on it.4 Also, how long a user has been active in the network appears to increase the number of friend linkages they have (Ballings, van den Poel, & Bogaert, 2016; Lewis, Kaufman, Gonzalez, Wimmer, & Christakis, 2008), which could expand his or her opportunities for a greater number of indegree profile views. Thus, H1 examines whether these factors help to predict profile consumption behavior in an SNS in terms of indegree profile views:
H1: (a) The number of linked friends, (b) account longevity, (c) frequency of profile content updates, and (d) amount of comments users receive on their profiles positively predict views of users’ own profiles by others in the network.
The finding reported earlier that people tend to use SNSs to communicate with known others rather than with strangers implies that users extract less utility in casually browsing strangers’ profiles compared to viewing friends’ profile updates and activities. This, coupled with the fact that most SNSs’ privacy preferences limit strangers’ access to their profile information to only the minimal “public” information (e.g., name, gender, username, profile picture), leads to the prediction that profile viewing should be more common among friends than among strangers. Behavioral data on the prevalence of profile browsing between friends versus strangers may also help settle debates about how common interpersonal electronic surveillance and “lurking” by strangers are within SNSs, as estimates vary widely in existing studies, which are usually based on self-reports. The second hypothesis thus predicts that:
H2: Profile browsing is more common among friends than it is among strangers.
Individual differences among users may also influence communication patterns in SNSs (Burke et al., 2011), including profile browsing among friends versus strangers. For the reasons articulated earlier, users with more friends may attract more profile views, and so it is reasonable to predict that as the number of friends users have increases, so might the incidence of indegree profile browsing. But this is particularly likely for profile views coming from friends rather than from strangers, assuming the higher motivation for browsing friends’ rather than strangers’ profiles discussed earlier. Also, because strangers typically do not have established, long-term relationships with profile owners that would provide a motive for repeat viewing, repeat indegree profile viewing should also favor friends over strangers. Thus, it is hypothesized that:
H3: As the number of friends SNS users have increases, they will have more profile views from others, and more of their profile views will come from friends rather than from strangers.
H4: Repeat profile viewing is more common among friends than it is among strangers.
Profile consumption behavior, information propagation, and social capital
Perhaps more important than the question of the prevalence of profile browsing is the impact of this behavior on users. A good deal of research on the benefits of SNS use focuses on the prospects of these applications for increasing users’ social capital. Social capital refers to the actual or potential resources that may be derived from relationships between people who are connected in a social network (see Bourdieu, 1986; Ellison, Steinfield, & Lampe, 2007). Putnam (2000) identified two forms of social capital, one derived from strong or intimate ties, called bonding social capital, and the other originating from weak network ties, called bridging social capital. Closer relationships such as those with friends and family members provide bonding social capital, which enables emotional support, reciprocity, and companionship (Burke et al., 2011). However, weaker ties are theorized to generate bridging social capital, which provides access to novel information because looser ties typically are more heterogenous than close ties and thus offer greater potential for nonredundant information (Granovetter, 1973; see also Ellison, Lampe, Steinfield, & Vitak, 2010).
SNSs are thought to provide social capital benefits by increasing means and opportunities for communication between people, which can serve as a basis of social support and information sharing. SNSs are theorized to increase bonding social capital by helping users identify common ground with others that may then deepen relationships and facilitate access to sources of social support. SNSs are also believed to increase bridging social capital by lowering the costs of communication with weaker ties or enabling connections to form between strangers, thus allowing individuals to maintain a larger and more diverse network of ties, through which novel information can flow (Ellison et al., 2007, 2010).
Studies have found empirical evidence that SNS use increases users’ perceptions of both bridging and bonding social capital (e.g., Ellison et al., 2007; Steinfield, Ellison, & Lampe, 2008). As mentioned earlier, recent work has begun to examine the link between specific uses of SNSs and social capital, finding that different uses lead to different social capital benefits (for a review, see Antheunis et al., 2015). For example, Papacharissi and Mendelson (2011) found that using Facebook for sharing personal information and for relaxation were more conducive to social capital than other types of uses. Similarly, studies have found that people who used SNSs actively for socially directed communication reported higher social capital, relational closeness, or access to resources (Brandtzaeg, 2012; Brooks, Hogan, Ellison, Lampe, & Vitak, 2014; Burke et al., 2011; Koroleva, Krasnova, Veltri, & Günther, 2011; Vitak, 2012).
While it makes sense that visible interaction between SNS users (communicating with others via wall comments, tagging photos, expressing approval via clicking “Like,” etc.) would establish connections to others that could increase users’ feelings of social capital, including getting social support, obtaining information, etc., it is less clear why mere profile browsing would affect social capital. Burke et al. (2011) argue that undirected communication within SNSs, including profile browsing, “provides content for conversational grounding and reveals users’ similarities” (p. 3) and thus can promote relationship development and social capital. That said, profile consumption behavior may be more likely to influence bridging rather than bonding social capital because, despite no purposive communication between the two users, it affords opportunities for information propagation, as users may glean new information by simply viewing others’ profiles (Cerni & Talmud, 2015; Metzger, Wilson, Pure, & Zhao, 2012; Vitak, 2012). Moreover, the relatively low effort of profile browsing may increase its frequency compared to visible interaction, thus creating greater opportunities for information dissemination (or “propagation”) through the network as users consume content posted by others.
It is also easy to understand why profile viewing between linked friends within an SNS would increase social capital, as friends are likely more able and motivated to provide social support to friends than are strangers, and friends will probably pay greater attention to information on friends’ versus information on strangers’ profiles. Ellison et al. (2011) argue that interaction with strangers is unlikely to generate bonding social capital benefits because SNS users with no shared history will have less inclination to answer information requests, and that “provisions of emotional support will be less meaningful when coming from strangers with little personal knowledge of the recipient” (p. 878). On the other hand, however, the theories that have been invoked to explain the link between SNS use and bridging social capital support the idea that strangers may also offer some social capital benefits.
For example, Granovetter’s (1973) strength of weak ties argument says that novel information and opportunities tend to come from weak rather than strong ties because strong ties are more homogeneous, and thus weak ties can provide more benefit because the chance of receiving nonredundant information is greater. Ellison et al. (2007, 2011) say weaker ties are also more likely to serve as “bridging ties,” which are especially important for information dissemination in networks because they connect components of the network (e.g., groups of individuals) that might not connect otherwise, thus allowing novel information to spread across the network. Latent Tie theory (Haythornthwaite, 2002, 2005) says communication media enable individuals to open new paths for information flows by providing an infrastructure of latent ties (i.e., ties that are technically possible but have not been activated). Based on this, Ellison et al. (2007) argue that by including identifying information that users can use to forge connections, SNSs may encourage people to convert latent ties into weak ties that can lead to social capital benefits, such as access to new information and opportunities.
Yet very little is known about the relationship between profile browsing among friends or strangers and social capital. Only a small handful of studies have attempted to look at profile consumption behavior and social capital online, and they reached mixed conclusions (Burke et al., 2011; Ellison et al., 2011; Koroleva et al., 2011). For example, Ellison et al. (2011) found that using a SNS to learn about people (e.g., via profile browsing) that one has an offline connection to, such as a classmate or neighbor, resulted in higher perceptions of bridging and bonding social capital, whereas using a SNS to meet strangers or communicate with close friends does not. And Burke et al. (2011) found that only directed communication from linked friends was associated with bridging social capital, whereas mere consumption of friends’ profile information and broadcasting information such as status updates to one’s entire network were not predictive of either bridging or bonding social capital. These two studies reach conflicting conclusions about the impact of profile browsing on social capital. Moreover, only one of these studies considered profile browsing among strangers, finding that using Facebook to learn about strangers may not be linked to social capital outcomes. Conflicting results across prior studies of profile browsing and the theoretical reasons articulated earlier that profile browsing among friends and strangers should be an efficient means of obtaining novel information upon which social capital can be obtained warrant further research on this topic.
The idea that information propagation across network ties can increase social capital stems from the earliest conceptualizations of social capital that define it as the ability for people to access resources in their social network (e.g., Bourdieu, 1986). Theoretically, then, information flowing between (any) nodes in a network has the capacity to increase social capital because it would help those nodes become aware of, locate, and thus potentially access available resources. While it is obvious that active communication between users propels information from one node to another, merely reading other users’ profiles likely also allows information to flow between network members. Evidence that profile consumption transmits information from one user to another is shown by studies that find, for example, that profile browsing can lead to “ambient awareness” of what others in the network are doing (see also Levordashka & Utz, 2016; Utz & Levordashka, 2017), and can “broaden horizons” resulting in users feeling “informed” and “learning new things” (e.g., Krasnova, Wenninger, Widjaja, & Buxmann, 2013). Other studies find that SNS lurking results in information gain (e.g., Arnold & Paulus, 2010).
In sum then, this study adds to the literature on social capital by taking Ellison et al.’s (2007) thesis that SNSs affect social capital by facilitating the conversion of latent to weak ties one step further to theorize that obtaining social capital benefits is possible even without any directed or visible communication between users. In other words, we propose that mere profile browsing can itself impart informational resources between users, and thus social capital benefits. Specifically, in our study we investigate how relatively passive profile browsing behavior impacts the rate of information propagation in an SNS that could underlie the formation of bridging social capital. To do this, the capacity of profile browsing among both linked friends and strangers to spread information across a social network is measured and compared to more active forms of social interaction in a SNS in order to answer the following research question:
RQ2: How do visible interaction and profile browsing among friends and strangers compare in terms of their ability to propagate information across an SNS?
Method
Gathering data on profile browsing is a significant challenge because most SNSs, including Facebook, do not make these data available to external researchers or to web crawlers. Thus, the data for this study come from Renren (www.renren.com), which is the largest SNS in China with over 200 million users (www.statista.com). Renren is a clone of Facebook, with very similar structure, user interface, and features (see Figure 1) and, like Facebook, Renren evolved from a university-based social network called Xiaonei. As in Facebook, Renren users maintain personal profiles, upload photos, write status updates and diary entries (wall posts), and establish bidirectional social links with their friends. Similar to the Facebook news feed, all user-generated updates and comments are tagged with the sender’s name and a time stamp.
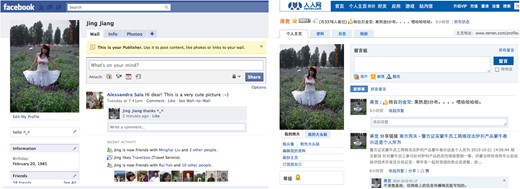
Unlike Facebook, however, Renren has two unique features that make it a particularly attractive platform to study user behavior. First, although Renren users have privacy control over their personal profiles, their “Friend lists” are public, which means that they can be crawled to produce Renren’s entire “social graph,” which shows who is officially “Friends” with (linked to) whom in the network. Indeed, the data for this study are based on a social graph with 42 million users and 1.66 billion explicit Friend links. Second, each Renren user profile shows the total number of visitors to that profile, along with names and links to the last nine visitors ordered from most to least recent, which is updated in real time and visible to profile owners. In addition, each photo and diary entry has its own page that includes a count of visits by users other than the profile owner. These records expose profile browsing events (i.e., profile visits) to crawlers. With this information, we were able to capture users’ profile browsing behavior, granting us a unique opportunity to gather and analyze large-scale naturalistic data on profile consumption behavior within the Renren social network.
Two methods were applied to the Renren social graph data to investigate the hypotheses and research questions advanced in this study. The first method involved a large-scale crawl of the Renren network to measure properties of profile browsing, including its prevalence as compared to visible interactions. The second method employed a simulation using cascade models to measure and compare information propagation via visible interaction versus profile browsing across the network. Each method is explained below.
Crawling the Renren social network to analyze the nature and prevalence of profile browsing
Data captured from crawling the entire Renren network over a three-month period produced an exhaustive snapshot that included 42,115,509 users and 1,657,273,875 Friendship links. In addition to this, we also performed smaller, more detailed crawls of the Peking University (PKU) network over the same time period to collect information about users’ interaction patterns. Because we collected the network memberships of all users during our complete crawl, we were able to isolate the 100,973 members of the PKU network to seed our detailed crawl. Of these users, the majority (60.8% or 61,405 users) had the default, permissive privacy policy, enabling us to collect their detailed information. During the PKU crawls, we gathered all comments generated by users in message board posts, diary entries, photos, and status updates. These data are the basis of our analysis of visible social interactions and include the complete record of public visible interactions between users in the PKU network. In total, 19,782,140 comments were collected, with 1,218,911 of them originating during the 90-day data collection timeframe.
In addition to measuring visible interactions in the PKU network, we also constructed profile browsing histories by recording the recent visitor records displayed on each PKU user’s profile via successive crawls. This information forms the basis of our study of profile consumption behavior. Across the 61,405 user profiles crawled, we extracted 1,863,168 unique profile visit events. Although Renren does not show individual recent visitors of user diaries and photos, it does display the total number of visits, which we crawled as well. Our data set shares similar properties with social network data sets collected in prior studies, including from Facebook, which reflect a good deal of homogeneity in terms of basic network structural properties across different SNSs (e.g., Chun, Kwak, Eom, Ahn, Moon, & Jeong, 2008; Wilson, Boe, Sala, Puttaswamy, & Zhao, 2009).
To address RQ1 and H1–H4, the frequency of visible interaction and profile consumption behavior (i.e., profile browsing) between both linked friends and strangers were measured. The measurement of visible social interaction consisted of counting the number of comments on message board posts, diary entries, photos, and status updates. Profile consumption behavior was measured by counting the number of views each profile received, and the number of others’ profiles that a user viewed was also counted. Whether the profile view came from a linked friend in the network or from a stranger, and the frequency of both unique profile viewing and repeat viewing were measured as well. We also captured each user’s total number of linked friends and number of comments received from other users in the network from information displayed publicly on each user’s profile. The frequency of profile content updates was measured by calculating the total number of new status updates, diary entries, photos, and shared links to web content made by the profile owner. Account longevity was estimated by calculating the number of days between when a user received their first comment and their most recent comment in the Renren network. The timestamp of the first comment a user received is often indicative of their network join date because the comment is likely to be a welcome message from a friend greeting the new user.
Because our study focuses on the structure of social graphs and communication (or profile viewing) events between users, we do not need to examine any actual content of comments, photos, or user profiles. To further protect user privacy, all userIDs were anonymized and stripped of any private data. Additionally, all user IDs were changed to random IDs and timestamps were replaced with relative sequence numbers.
Analyzing information propagation in SNSs
Understanding information dissemination within a SNS platform is typically done in computer science via social influence maximization methods. These methods seek to determine the most influential individuals who will maximize the spread of information within a social network and to study how influence spreads or cascades from those individuals (see Chen, Wang, & Yang, 2009; Kempe, Kleinberg, & Tardos, 2003). Statistical cascade models based on raw social network data can be built to show connections between participants, and algorithms can be designed to study social influence maximization problems within these models from which information dissemination in the network may be analyzed.
Because cascade models are built atop network graphs (i.e., mathematical structures used to model pairwise relations between nodes in a network), to compare the information dissemination capabilities of visible interaction versus profile browsing within a social network, and thus to address RQ2, we first constructed three different types of Renren social network graphs: the social graph, the visible interaction graph, and the profile consumption graph. The social graph is a pictorial representation of who is connected to whom via explicit and confirmed “Friend” links within the network. The visible interaction graph is constructed by connecting users from the social graph who have visibly interacted with one another (i.e., left comments) one or more times. In other words, this graph represents only the nodes that have visibly communicated with each other on the network, rather than all nodes that are linked friends with each other, as in the social graph. The profile consumption graph is constructed as the set of users that are connected via profile views with no visible interaction between them. In this article we restrict our social, visible, and profile consumption graphs to only contain users from the PKU network (N = 61,405) because these are the only users for whom we have complete interaction records. Because strangers can view fellow network members’ profiles, the profile consumption graph contains links between users who are not linked friends in the PKU social graph, which is helpful in answering RQ3.
We next performed a simulation to compare information dissemination in these graphs by implementing three types of corresponding influence/cascade models on the network graphs. First is the weighted cascade model, which is based on the social graph and relies on explicit “Friend” linkages plus an activation probability to simulate the information dissemination process. After receiving information, a user has a single chance of activating each currently inactive linked friend. In this model, the activation probability is related to the number of Friends a user has in the network such that if person w has dw Friends, that person may be activated by his or her Friends with a probability of 1/dw. Of course, information may not be disseminated equally through all social links. User profiles are likely only viewed by a portion of one’s friends, and thus not all social links may be active in information propagation. Moreover, some portion of the visits to profiles can be attributed to non-Friends (i.e., strangers). As discussed earlier, even when a user merely browses a profile, information on the web page is naturally propagated to that user. We suggest that it is thus more accurate to represent information dissemination capabilities by links in profile consumption graphs than by Friendship links in the social graph.
To measure information propagation within the profile consumption graph we created the consumption cascade model. When a person receives information from browsing a user’s profile, they have a chance to activate inactive neighbors, who have directed links pointing to that person in the profile consumption graph. To transform the visitor counts from our PKU crawl data into activation probabilities, we calculated the number of unique profile visitors for every user and determined the most popular user. We then computed the activation probability of every user by normalizing each user’s number of unique visitors by the maximum value. This approximates an activation probability using the “visit rate” normalized to the most popular profile. We use visits from unique users and exclude repeated visits here because the first visit contributes the most to information propagation, and repeated follow-up visits are less likely to propagate novel information. Finally, we built the visible cascade model atop the visible interaction graph in a similar fashion to the consumption cascade model. The information dissemination possibilities here are determined by visible interaction events between nodes, and the per-user activation probability is then determined using the same method as in the consumption cascade model.
Using these models we perform a simulation to analyze information propagation within the social, visible interaction, and profile consumption graphs. Our simulation uses the MixedGreedyWC algorithm as recommended by Chen et al. (2009) to find the most influential individuals in each of our three cascade models, and then we compute the number of people influenced. We varied the set of seed users to the algorithm from 1 to 100 in our tests and observe the effects on influence spread within the networks. Seeds were selected randomly each time, and each seed was run 10 times. Our simulation thus allows us to compare the structural properties of the consumption graph (i.e., profile views) against those of both the visible interaction graph (i.e., written posts and comments) and the social graph (i.e., confirmed “Friend” linkages) in terms of each graph’s potential for information propagation. For more detail about our methods, see our earlier work reported in Jiang et al. (2010).
Results
The first research question asked how prevalent profile browsing is compared to more visible forms of SNS interaction. To understand the frequency of profile consumption (i.e., viewing others’ profiles) and visible interaction (i.e., leaving posts and comments on others’ profiles) in Renren, we examined how often users engaged in both kinds of activities. The data show that profile consumption behavior is very prevalent across all users, with more than 93% of users engaging in profile browsing of friends or strangers. In contrast, the majority of visible interactions were produced by a small but highly interactive portion of the user base: 28% of users in the network account for nearly all visible interactions (see Figure 2). The findings show that most Renren users are more active in viewing profiles than they are in writing visible comments and, thus, profile consumption is more prevalent than visible social interaction.
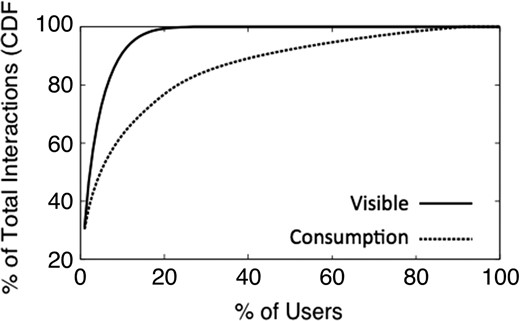
Distribution of visible interaction and profile consumption behavior.
The data also show that profile browsing covers a wider range of friends than does visible interaction. For each user, we computed a distribution of their profile consumption behaviors and their visible interactions across all of their social links and plotted the percentage of linked friends involved in each type of interaction. As can be seen in Figure 3, around 80% of users visibly interacted with only about 5% of their friends, and no users interacted with more than 40% of their friends. In contrast, roughly 80% of users viewed 20% or more of their friends’ profiles, and a small portion of the population viewed all of their friends’ profiles regularly. This further shows that profile browsing is more prevalent than visible interaction (see also Jiang et al., 2010 for greater detail on these findings).
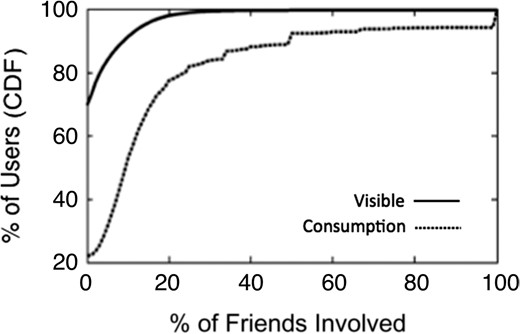
H1a–d predicted that four factors, including the more linked friends, account longevity, frequency of user-generated profile content updates, and number of comments that a user profile received from others, will correspond to a greater number of views of that user’s profile. To analyze this question we divided users into four groups based on their profile “popularity” (i.e., number of profile views received), and calculated both the average value of the four factors in each group and the correlation between each factor and user profile popularity. Users in the low viewership popularity group (57% of the population studied) received between 0–100 profile views, whereas users in the two medium viewership popularity categories (42%) received between 100–1,000 and 1,000–10,000 profile views. Users whose profiles exceeded 10,000 views (by far the smallest group at 1%) were categorized as the most popular.
Table 1 shows the correlations. Although all factors except number of friends exhibited substantial correlations within the low popularity group (those whose profiles were rarely viewed by others), this is an artifact of the tied ranks among the many low popularity users. Unsurprisingly, these users exhibit very low interactivity and number of friends, which leads to the high levels of correlation (similar statistical artifacts have been observed when analyzing all users in a large SNS data set, see Cha, Mislove, & Gummadi, 2009). Low popularity users likely use the network infrequently. By contrast, users in the two medium popularity groups (100–1,000 and 1,000–10,000 profile views) represent more “normal” social network users in the sense that they use the SNS somewhat regularly for its intended communication purposes. Users in these categories show relatively higher correlations across all of the user-generated content factors compared to users in the highest popularity category. Also, in both medium popularity groups, the number of friends was most strongly correlated with profile views. Account lifetime is a less important factor for users in the 1,000–10,000 popularity range than for the other medium popularity group, which likely reflects the fact that users can amass hundreds of friends quickly on SNSs. Generating more user-generated content and receiving comments also appear to be less important impetuses for users with higher popularity to attract profile views (i.e., correlations were stronger for the lower medium popularity group than for the higher medium popularity group).
Average Value of Factors Affecting User Popularity and Correlations of Factors With Each User Popularity Category
User Popularity (# of profile views) . | Friends . | Lifetime . | Diary . | Photo . | Status . | Share . | Comment . |
---|---|---|---|---|---|---|---|
0–100 | 16 (.15) | 35 (.55) | 1 (.51) | 3 (.47) | 1 (.50) | 1 (.50) | 2 (.54) |
100–1,000 | 131 (.56) | 423 (.41) | 11 (.33) | 41 (.24) | 27 (.36) | 43 (.41) | 96 (.45) |
1,000–10,000 | 401 (.43) | 792 (.24) | 50 (.18) | 125 (.10) | 115 (.18) | 155 (.23) | 596 (.28) |
>10,000 | 708 (.02) | 869 (.02) | 117 (.02) | 251 (−.03) | 236 (.01) | 273 (−.05) | 1,581 (.01) |
all users | 112 (.73) | 263 (.75) | 12 (.70) | 34 (.61) | 28 (.69) | 39 (.72) | 134 (.76) |
User Popularity (# of profile views) . | Friends . | Lifetime . | Diary . | Photo . | Status . | Share . | Comment . |
---|---|---|---|---|---|---|---|
0–100 | 16 (.15) | 35 (.55) | 1 (.51) | 3 (.47) | 1 (.50) | 1 (.50) | 2 (.54) |
100–1,000 | 131 (.56) | 423 (.41) | 11 (.33) | 41 (.24) | 27 (.36) | 43 (.41) | 96 (.45) |
1,000–10,000 | 401 (.43) | 792 (.24) | 50 (.18) | 125 (.10) | 115 (.18) | 155 (.23) | 596 (.28) |
>10,000 | 708 (.02) | 869 (.02) | 117 (.02) | 251 (−.03) | 236 (.01) | 273 (−.05) | 1,581 (.01) |
all users | 112 (.73) | 263 (.75) | 12 (.70) | 34 (.61) | 28 (.69) | 39 (.72) | 134 (.76) |
Note: Average values are shown in the table cells; Spearman rank correlation coefficients for each average value are shown in parentheses. All correlations are significant at p < .001, as would be expected in a data set this large. Pearson r correlations were not used due to drastic differences in the size of each popularity group.
Average Value of Factors Affecting User Popularity and Correlations of Factors With Each User Popularity Category
User Popularity (# of profile views) . | Friends . | Lifetime . | Diary . | Photo . | Status . | Share . | Comment . |
---|---|---|---|---|---|---|---|
0–100 | 16 (.15) | 35 (.55) | 1 (.51) | 3 (.47) | 1 (.50) | 1 (.50) | 2 (.54) |
100–1,000 | 131 (.56) | 423 (.41) | 11 (.33) | 41 (.24) | 27 (.36) | 43 (.41) | 96 (.45) |
1,000–10,000 | 401 (.43) | 792 (.24) | 50 (.18) | 125 (.10) | 115 (.18) | 155 (.23) | 596 (.28) |
>10,000 | 708 (.02) | 869 (.02) | 117 (.02) | 251 (−.03) | 236 (.01) | 273 (−.05) | 1,581 (.01) |
all users | 112 (.73) | 263 (.75) | 12 (.70) | 34 (.61) | 28 (.69) | 39 (.72) | 134 (.76) |
User Popularity (# of profile views) . | Friends . | Lifetime . | Diary . | Photo . | Status . | Share . | Comment . |
---|---|---|---|---|---|---|---|
0–100 | 16 (.15) | 35 (.55) | 1 (.51) | 3 (.47) | 1 (.50) | 1 (.50) | 2 (.54) |
100–1,000 | 131 (.56) | 423 (.41) | 11 (.33) | 41 (.24) | 27 (.36) | 43 (.41) | 96 (.45) |
1,000–10,000 | 401 (.43) | 792 (.24) | 50 (.18) | 125 (.10) | 115 (.18) | 155 (.23) | 596 (.28) |
>10,000 | 708 (.02) | 869 (.02) | 117 (.02) | 251 (−.03) | 236 (.01) | 273 (−.05) | 1,581 (.01) |
all users | 112 (.73) | 263 (.75) | 12 (.70) | 34 (.61) | 28 (.69) | 39 (.72) | 134 (.76) |
Note: Average values are shown in the table cells; Spearman rank correlation coefficients for each average value are shown in parentheses. All correlations are significant at p < .001, as would be expected in a data set this large. Pearson r correlations were not used due to drastic differences in the size of each popularity group.
Interestingly, no factor was correlated with profile views for users in the highest popularity group (>10,000 profile views). This finding is important in that it shows profile popularity is not gained simply by having lots of friends or posting great amounts of user-generated content. This also suggests that there are other factors not measured in our study that determine users’ profile popularity, for example, it may be that quality rather than quantity of user-generated content is more important for attracting profile visitors, or that real-world celebrity status may be the most important factor in profile viewership. Overall, the data in Table 1 show that all factors generally increase with popularity such that users with more profile views also have more friends, older accounts, and generate the largest amounts of content. These results support H1a–d among more typical SNS users (i.e., the medium popularity groups).
The second hypothesis predicted that profile consumption behavior would be more common among friends than among strangers. We tested this expectation by analyzing the composition of visitors to user profiles to determine whether profile visitors were mostly friends or strangers (i.e., network members who are not liked “Friends”). Results show that profile views are fairly evenly divided between friends and strangers. That said, whereas about 45% of users receive less than half of their profile visits from strangers, a small majority (about 55%) receives most of their profile views from strangers (see Figure 4). These data do not support H2.
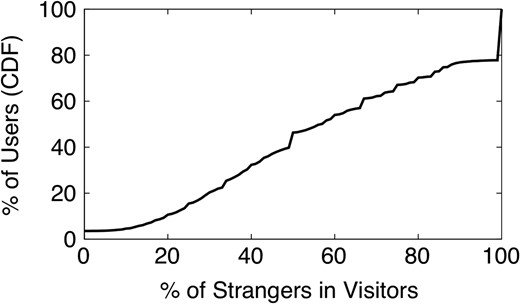
The results vary, however, by how connected users are in the network. H3 predicted that as the number of friends that SNS users have increases, they will receive more profile views, and more of the profile views will come from friends rather than from strangers. We grouped profile owners by the number of linked friends they had (<100, 100–1,000, and >1,000) and computed the average number of profile visitors who were linked friends, friends-of-friends, or “other” visitors. We found that for less connected users (<100 friends), a large majority of visitors are strangers and very few visitors are friends-of-friends. For more connected users (100–1,000 friends), however, the majority of visitors are linked friends with a substantial number of visits by friends-of-friends as well. For the most connected users (>1,000 friends), the pattern shifts again toward strangers, such that about half of the visitors are linked friends, with a quarter of profile views coming from friends-of-friends and a quarter from strangers. Figure 5 illustrates these results. Overall, the results provide partial support for H3. Although the expected patterns emerged across the low to medium groups, highly popular users draw more strangers to visit their profiles. In any case, the data reveal quite a lot of profile browsing that happens between strangers, particularly among the low and high popular user groups.
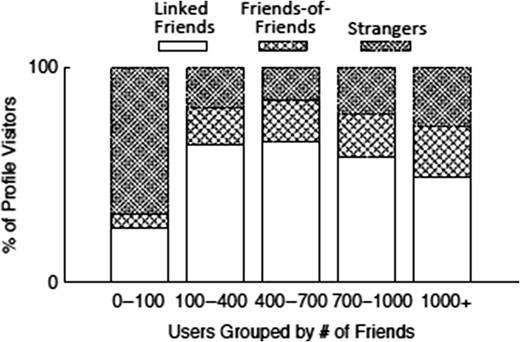
Breakdown of profile visitors by profile owner’s connectedness.
H4 expected repeat profile viewing would be more common among friends than among strangers. To investigate this we first examined repeat profile viewing generally by calculating the percentage of repeat visitors for each profile and plotting the data in a distribution. The data show that about 70% of users have less than 50% repeat visitors, which indicates that most profile visitors do not repeat-browse the same profile. This result makes sense because most users only need to view a profile once to consume its information. This finding further indicates that most profile browsing occurs by users randomly browsing others in the network. Turning now to H4 specifically, we expected that repeat profile viewing behavior should happen more often between friends than strangers because, unlike friends, strangers do not tend to build long-term relationships with profile owners. Surprisingly, however, as seen in Figure 6 when we computed the average number of visits from strangers and friends for each profile, we found that the repeat profile viewing behavior for friends and strangers is quite similar, with friends edging out strangers by only a tiny margin (see also Jiang et al., 2010).
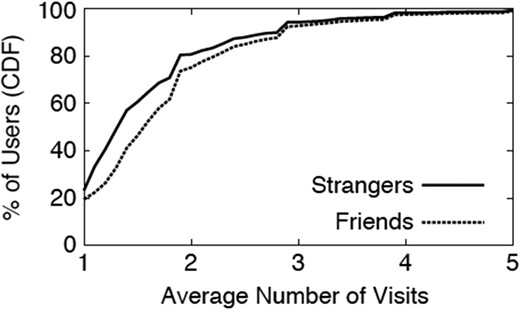
The final research question focussed on the ability of profile browsing to propagate information. RQ2 asked how profile browsing compares to visible interaction in their ability to propagate information within an SNS. As mentioned earlier, we performed simulations using cascade models to understand information propagation within the social, visible, and profile consumption graphs. Figure 7 shows influence spread (i.e., number of people influenced) versus seed set size for our three cascade models. Influence in the social and consumption cascade models both increase quickly at first. As more seeds are selected, the number of people influenced in the profile consumption cascade model surpasses the social cascade model. This can be explained by the fact that, although social graphs have a large number of links, most links in the network are inactive because most users are only linked friends with a small subset of others in the network. This inherently limits the spread of information to well connected components of the graph. By contrast, consumption graphs are constructed by mere profile views, which have a higher activation probability because they likely require less time to perform than do visible interactions (which require both profile viewing and writing content). So, while people necessarily receive information when they merely browse profiles, information cannot be propagated through inactive social (“Friend”) links. Visible interaction graphs have the least number of links, and although activation probabilities are relatively high, the visible cascade model has the smallest influence spread due to its relative dearth of links (see our prior work in Jiang et al., 2010 for more detail). To summarize, the profile consumption graph was most efficient for propagating information between users because profile viewing happens more frequently than visible interaction, and because social graphs can only spread information between linked friends and there are far fewer formal connections (i.e., Friend linkages) than there are informal profile browsing events between users in the network.
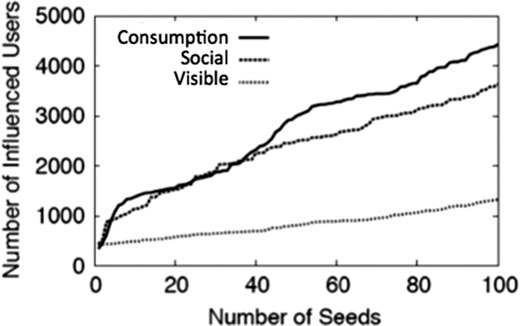
Influence spread using the MixedGreedyWC algorithm on the three graphs.
Discussion
Findings from this study provide new insight into the nature and frequency of profile consumption behavior in SNSs and the role that it plays in information propagation that is relevant to social capital formation. Our data indicate that profile browsing events on SNSs are more numerous, often connect strangers, and thus may be more efficient for information dissemination in a network compared to visible interaction. Moreover, our findings speak to some debates and mixed findings in the literature about such things as the prevalence of interpersonal electronic surveillance or lurking that takes place in SNSs, and the means through which SNS users may be able to extract social capital benefits from their network connections.
For example, past estimates of the prevalence of relatively passive types of SNS uses (e.g., profile browsing and lurking) vary widely across studies. While some have argued that social media are fundamentally about interactivity rather than mere consumption or passivity, some studies of online communities find a great deal of lurking (Pempek, Yermolayeya, & Calvert, 2009; Preece, Nonnecke, & Andrews, 2004). Moreover, estimates based on self-reports may underestimate the amount of such behavior—and particularly the amount of viewing others’ profiles in SNSs—because people may be reluctant to admit to engaging in any “cyberstalking” behavior. Our data confirm that SNS users frequently engage in profile browsing. We find that many more Renren users engage in this behavior than in visible social interaction, and while most users communicated with only a small percentage of their network, they browsed content from a much larger proportion of the network. These results are similar to findings for Facebook (Facebook Data Team, 2009). We further find that a fair proportion of repeat profile viewing comes from people randomly browsing the network.
Our data also speak to assumptions concerning who tends to engage in profile consumption behavior. As discussed earlier, some feel SNS users would have little motivation to look at strangers’ profiles. Our analyses of H2 through H4 reveal that profile viewing between friends and strangers is about equal, with only a slight edge to strangers. Even repeat viewing shows nearly equal distribution between friends and strangers, with repeat viewing of friends’ profiles only slightly outpacing viewing strangers’ profiles. Together, these results reveal the assumption that friends are the dominant source of profile browsing events to be somewhat false.
Although early concerns of SNS use focussed on the dangers of social surveillance and cyberstalking by strangers, most user surveys find that the majority of SNS interactions are between acquaintances and friends rather than strangers. However, as mentioned above, our data show that a good portion of social interactions on SNSs occur between strangers and that profile browsing is an important component of SNS use. Our data also add nuance by showing that profile views of users who possess a middle range of friends on the network come mostly from friends, whereas much of the profile views of users with either very few or very many linked friends come from strangers. This indicates that some users may be more susceptible to so-called “stranger danger” in SNSs than are others.
The analysis of H1a–d offers some of the only available empirical insight into what drives profile browsing behavior. Owning an account for a longer period of time, regularly posting new profile content, and having a higher number of linked friends were all predictive of profile views. Again, though, significant differences emerged in the patterns observed among low-, medium-, and highly-viewed users. This suggests that there are different motivations underlying profile browsing for different types of users, and that future studies should take such user differences (e.g., user popularity) into account. As discussed earlier, the results of H1a–d also suggest that factors beyond those studied here may better explain motivations for viewing very low and very high popular users, which future research could examine.
A recent review of the literature on social capital concluded that while active and directed communication behavior on SNSs increase social capital, passive uses of SNSs (including profile consumption) do not (Antheunis et al., 2015). This conclusion is based on research such as that by Burke et al. (2011), described earlier, which found little evidence that profile consumption increases social capital. Yet, the data from our simulations clearly show that profile browsing is superior in terms of its potential for spreading information in the network compared to visible interaction. While information propagation is not equivalent to realizing social capital benefits in SNSs, it is a necessary first step. Profile consumption behavior may be particularly important in that profile browsing has potential to activate latent or non-existent ties between users, which the user could then derive social capital benefits from (Ellison et al., 2007). For example, via profile browsing, users could learn about common concerns, efforts, or interests of fellow SNS members, which could cause them to activate a relationship or, more simply, users could learn about available resources from information they encounter in other users’ profiles. Profile browsing may thus have a “mere exposure” effect on social capital formation.
Results from RQ2 also show that social capital, when defined in terms of the potential for information propagation in a network, is perhaps best increased through the actions of strangers rather than friends. This contradicts a basic tenet of social capital theory, which emphasizes that social capital flows between connected nodes who are both motivated and able to provide social support or answer queries for assistance or information from linked friends. At least for one form social capital—learning of resources that may be available—our finding that information is propagated most effectively via profile browsing shows that strangers can be equally important as are linked friends as a source of social capital. Not only do we find that strangers initiate a large portion of profile browsing events, but that there are more profile consumption events than visible interaction events between both linked friends and between strangers. We also see clear evidence of strangers randomly browsing the network. Taken together, this indicates that researchers should not overlook the significance of non-linked strangers when considering information dissemination in SNSs via profile browsing.
Conclusion
In sum, the results of our study challenge existing claims in the literature that profile consumption behavior: (a) is infrequent, (b) occurs more between friends than strangers, and (c) does not contribute to social capital outcomes. Our results also alert us to the fact that different types of SNS users (e.g., those with greater or fewer linked friends or profile views) might experience profile viewing differently and for different reasons, which may have important implications for realizing social capital benefits in SNSs.
Despite having a much larger amount of data compared to most other studies of social capital and data that do not rely on self-reports, one limitation of our study concerns its generalizability to other SNS platforms and to SNS users in other parts of the world. Regarding the first point, different SNS systems have different interfaces and features, which might influence user behavior in unique ways. The most pressing example relevant to our study is that profile browsing in Renren is displayed publicly and is thus visible to profile owners, whereas this is not the case in Facebook. This is important because the ability to browse profiles anonymously might result in increased profile viewing since users can do so without fear of being exposed as a “Facestalker.” This suggests that profile browsing would be more prevalent in Facebook than in Renren. On the other hand, compared to Renren, profile browsing in Facebook may be depressed by the fact that users know most people have privacy settings that prevent strangers from seeing much information. Also, seeing who has viewed your profile in Renren may prompt reciprocal profile views, as people might be curious to learn more about users who have viewed their profile. This suggests that perhaps profile browsing occurs more frequently in Renren than in Facebook. It is thus unclear whether profile browsing is more prevalent in Facebook or Renren, or if it is about even. As noted earlier, our findings on the relative frequency of profile browsing are similar to data provided from Facebook itself (Facebook Data Team, 2009).
Regarding the second point, there is nothing about the insights gained from our Renren data on profile browsing or its psychological motivations that is so culturally-specific as to cast doubt on their applicability to users of other SNSs, including those who live in the United States. In addition, our study provides a rare look at large-scale data from a non-U.S. population. In any event, further research on a wider array of SNSs and with a broader population of users is needed to fully understand the generalizability of our findings. This is a challenging task, however, because such data are not available from many SNS platforms, including Facebook.
Another limitation is that we are only able to analyze data from users with public profiles (which luckily is the default in Renren and most other SNSs), and it is possible that users with public profiles behave differently than users with private profiles. Ideally, to circumvent this limitation all user profiles would be subject to analysis, but this is difficult because most SNSs are unwilling to release visitor log data, even to researchers. That said, having a private profile does not preclude a user from engaging in profile browsing, and because it was the default setting at the time of data collection, a large majority of Renren users had public profiles.
Finally, studying how visible interaction and profile consumption behavior are distributed among both linked friends and strangers in SNSs and identifying popular or influential users within the network can assist in understanding information propagation in social networks, which is important to many social applications and to social science alike. Lessons learned from research on how users behave in SNSs can guide software developers toward more engaging mechanisms for social interaction and relationship management, as well as tools for privacy protection. At the same time, such research can help scholars better understand and model the variety of ways that people form social connections in SNSs that may help them realize the benefits that such connections can afford.
Notes
“Passive” uses of SNSs that have been studied in the literature include actions such as reading or looking at other users’ profile information or photos without commenting or otherwise visibly communicating on the SNS. Because any action within SNSs requires some effort, and because browsing other users’ profiles may be done either randomly or in a targeted manner (e.g., to seek information about someone who attends the same school), “passive” is used in this article in relative rather than absolute terms. Thus, consuming other users’ profile information is only “passive” in the sense that it is less “active” than posting a comment, clicking like, or any other communication behaviors that leave a visible trace because those actions involve both reading/looking at the profile information and then taking further action to write comments, etc.
For the purposes of this research, both friends and strangers are defined in network terms such that nodes (network members) with an existing edge (official “Friend” link) between them are designated as “friends” and nodes with no edge between them are designated as “strangers.” As such, in this study browsing a “stranger’s” profile simply refers looking at someone’s profile whom you do not share a Friend linkage with.
Borrowing terminology from social network analysis, we define indegree profile browsing as the number of views directed to a node’s profile by other users, whereas outdegree profile browsing is the number of others’ profiles that the node views.
Although social capital is typically measured by self-reports, a few past studies have measured aspects of social capital in terms of network properties, including network density and information propagation (e.g., Brooks et al., 2014). Moreover, Soroka and Rafaeli (2006) take a similar approach as our study while examining the relationship between social capital and lurking behavior, which is a similar form of SNS use as what we study in this article.
References
Author notes
Accepted by Nicole Ellison