-
PDF
- Split View
-
Views
-
Cite
Cite
Juan M Jiménez-Vacas, Antonio J Montero-Hidalgo, Enrique Gómez-Gómez, Antonio C Fuentes-Fayos, Francisco Ruiz-Pino, Ipek Guler, Antonio Camargo, Francisco J Anglada, Julia Carrasco-Valiente, Manuel Tena-Sempere, André Sarmento-Cabral, Justo P Castaño, Manuel D Gahete, Raúl M Luque, In1-Ghrelin Splicing Variant as a Key Element in the Pathophysiological Association Between Obesity and Prostate Cancer, The Journal of Clinical Endocrinology & Metabolism, Volume 106, Issue 12, December 2021, Pages e4956–e4968, https://doi.org/10.1210/clinem/dgab516
- Share Icon Share
Abstract
Recent studies emphasize the importance of considering the metabolic status to develop personalized medicine approaches. This is especially relevant in prostate cancer (PCa), wherein the diagnostic capability of prostate-specific antigen (PSA) dramatically drops when considering patients with PSA levels ranging from 3 to 10 ng/mL, the so-called grey zone. Hence, additional noninvasive diagnostic and/or prognostic PCa biomarkers are urgently needed, especially in the metabolic-status context.
To assess the potential relation of urine In1-ghrelin (a ghrelin-splicing variant) levels with metabolic-related/pathological conditions (eg, obesity, diabetes, body mass index, insulin and glucose levels) and to define its potential clinical value in PCa (diagnostic/prognostic capacity) and relationship with PCa risk in patients with PSA in the grey zone.
Urine In1-ghrelin levels were measured by radioimmunoassay in a clinically, metabolically, pathologically well-characterized cohort of patients without (n = 397) and with (n = 213) PCa with PSA in the grey zone.
Key obesity-related factors associated with PCa risk (BMI, diabetes, glucose and insulin levels) were strongly correlated to In1-ghrelin levels. Importantly, In1-ghrelin levels were higher in PCa patients compared to control patients with suspect of PCa but negative biopsy). Moreover, high In1-ghrelin levels were associated with increased PCa risk and linked to PCa aggressiveness (eg, tumor stage, lymphovascular invasion). In1-ghrelin levels added significant diagnostic value to a clinical model consisting of age, suspicious digital rectal exam, previous biopsy, and PSA levels. Furthermore, a multivariate model consisting of clinical and metabolic variables, including In1-ghrelin levels, showed high specificity and sensitivity to diagnose PCa (area under the receiver operating characteristic curve = 0.740).
Urine In1-ghrelin levels are associated with obesity-related factors and PCa risk and aggressiveness and could represent a novel and valuable noninvasive PCa biomarker, as well as a potential link in the pathophysiological relationship between obesity and PCa.
Ghrelin is a hormone that plays pleiotropic functions throughout the body (1), including the regulation of hormone release (2), glucose homeostasis (3-5), energy homeostasis (6,7), and bone metabolism (8), among others. Moreover, ghrelin has been shown to be dysregulated in numerous pathological conditions, such as heart disease (9,10), muscular atrophy (11), and cancer (12). The ghrelin axis is comprised of different receptors (eg, growth hormone secretagogue receptor types 1a and 1b) and enzymes [eg, ghrelin O-acyl transferase (GOAT)], as well as by additional ligands (1). Specifically, as a result of different biological processes (ie, alternative splicing and proteolytic cleavage), the ghrelin gene can generate different proteins (eg, In1-ghrelin, obestatin) besides canonical ghrelin, which can play distinct roles or even antagonize ghrelin actions, thus increasing the complexity of this hormonal system (1). Among them, In1-ghrelin, an alternative splicing variant originated by the retention of the intron-1 of the preproghrelin messenger RNA, has gained importance during the last years, mainly due to its high expression in different pathologies, especially endocrine-related tumors, and to the plethora of biological processes that it regulates (13-19). Although In1-ghrelin variant shares with ghrelin the first 13 aa, which constitutes the region that is required for GOAT enzyme-mediated acylation and, consequently, for ghrelin to bind and activate growth hormone secretagogue receptor type 1a (20), the actions of In1-ghrelin differ from those of ghrelin, therefore warranting further investigation (1).
In this scenario, although some members of the ghrelin axis are deeply dysregulated in metabolic-related pathologies (eg, obesity, diabetes) and associated to the development of these diseases (1,21,22), the role of In1-ghrelin in metabolic diseases remain poorly known. Initial results demonstrated that In1-ghrelin expression is regulated in the pituitary and hypothalamus of mice in response to metabolic stressors, such as fasting or diet-induced obesity (13). However, the potential dysregulation of In1-ghrelin levels in adverse metabolic conditions in humans has not been addressed so far.
In addition, In1-ghrelin has been found to be upregulated in several endocrine-related cancers, where it associates to tumor development and progression, exerting even more pronounced oncogenic actions than those of native ghrelin in the majority of the pathologies studied (15-19). Among them, prostate cancer (PCa) has been found to be especially sensitive to the oncogenic actions of In1-ghrelin (19). Remarkably, we demonstrated that In1-ghrelin is overexpressed in PCa tissues and cell lines and associated to the aggressiveness of these tumors. Most important, we found that circulating In1-ghrelin levels were significantly elevated in plasma from patients with PCa, pointing out the potential noninvasive diagnostic potential of In1-ghrelin in PCa (19).
In this context, the measurement of circulating prostate-specific antigen (PSA) levels is considered the current gold-standard biomarker for noninvasive PCa diagnosis. Unfortunately, the reliability of PSA remains in question as it is associated with a high number of false positives and false negatives, especially in the 3 to 10 ng/mL range, the so defined grey zone (23). These drawbacks result in unnecessary biopsies and reduced patient quality of life, as well as to overtreatment in a considerable number of patients (24). For that reason, the discovery and establishment of novel and reliable noninvasive biomarkers for PCa detection remains as a clinical unmet need, especially in the context of a personalized medicine approach (ie, considering the metabolic status of the patients), which would improve patient survival and reduce the number of unnecessary biopsies in patients with suspect of PCa.
PCa biology is influenced by several clinical variables, including the metabolic status of the patients (25,26). Particularly, metabolic syndrome (MetS), a clinical condition comprising a combination of pathological alterations (ie, obesity, insulin resistance, elevated blood pressure and triglyceride levels, and decreased levels of cholesterol), has been reported to be associated with increased risk of developing PCa as well as linked to a more aggressive disease (27,28). Indeed, it has been reported that the greater number of MetS criteria met, the greater risk of developing PCa or significant-PCa (defined as PCa with Gleason score ≥ 7) (19,29). Among the MetS criteria, obesity has been especially related to PCa risk and even associated with a more aggressive disease (25,28,30). Moreover, obesity might impact PCa diagnosis since PSA levels have been found to be decreased on patients with high body mass index (BMI) (31). For the same reason, tumor stage could also be underestimated at diagnosis in obese patients (30). Therefore, based on these findings, it could be important to take into consideration potential metabolic and obesity-related factors that are involved in the relationship between PCa and altered metabolic conditions because it could result in the discovery of novel and more effective biomarkers for PCa diagnosis and prognosis in the context of a personalized medicine approach.
However, despite the fact that urine is a noninvasive body sample that is less burdensome and with a more prostate-specific content than plasma (32,33), presumably due to the anatomic proximity of the prostate to the bladder and urethra, which could promote the mobilization of fluids that drain from prostatic ducts into the urethra and then are carried out during micturition, the putative dysregulation of the levels of In1-ghrelin in this body fluid of PCa patients, as well as its potential association with PCa risk and with metabolic-related pathological conditions, has not been explored yet. Therefore, this study was aimed to evaluate, for the first time, urine In1-ghrelin levels in an ample and well-characterized cohort of patients with PSA ranging from 3 to 10ng/mL (n = 610), with PCa, or with suspect of PCa but negative result in the prostate biopsy [control patients (NegBiopsy] to (1) assess the potential relation of urine In1-ghrelin levels with metabolic-related pathological conditions (obesity, diabetes, BMI, insulin and glucose levels, etc) and (2) to define its potential clinical value in PCa (ie, diagnostic and prognostic capacity).
Material and Methods
Patients and Samples
This study was approved by the Ethics Committee of the Reina Sofia University Hospital (Cordoba, Spain), which was conducted in accordance with the Declaration of Helsinki and according to national and international guidelines. Written informed consent from all patients was obtained. All samples were obtained through the Andalusian Biobank (Nodo Córdoba, Servicio Andaluz de Salud, Spain). Urine and plasma samples analyzed herein were taken from a retrospective study wherein patients were recruited between 2013 and 2015 by consecutive recruitment of individuals with suspicion of PCa that underwent a transrectal ultrasound-guided prostate biopsy according to clinical practice in the Urology Department of Reina Sofia Hospital (Córdoba, Spain). Specifically, the cohort is divided in (1) NegBiopsy patients (n = 397; controls) and (2) patients diagnosed with PCa (n = 213). All the patients were selected based on the PSA levels in the grey zone. The grey zone of PSA was defined as PSA levels ranging from 3 to 10ng/mL based on previous studies (34-36).
Urine and blood samples were collected early in the morning after an overnight fast and just before the prostate biopsy. The donated urine samples, taken specifically from the first 100 mL of the urine, were processed at low temperatures (ie, in presence of ice at all times), rapidly frozen, and stored in 1.5 mL aliquots at −80°C. Once aliquoted, a protease cocktail (MiniProtease inhibitor; Roche, Nutley, NJ, USA) was added to the samples. In1-ghrelin levels were analyzed in intact aliquots of urine, which had never been thawed to avoid potential degradation due to freeze-thaw cycles. A blood sample (10 mL) was also collected, and plasma was obtained and stored at −20°C. For transrectal prostate biopsy, 12 biopsy cores were obtained from patients undergoing the first biopsy procedure and a minimum of 16 biopsy cores for those who had a previous biopsy. All biopsy specimens were analyzed by experienced urologic pathologists according to the International Society of Urological Pathology 2005 modified criteria (37).
Determinations of In1-ghrelin and Relevant Parameters Levels in Noninvasive Samples
For the measurement of acylated In1-ghrelin in urine, a competitive radioimmunoassay was used (RK-032–42; Phoenix, Burlingame, CA, USA). The In1-ghrelin radioimmunoassay showed a detection limit lower than 10 pg/mL and a detection range of 1 to 12 800 pg/mL. Urine GOAT levels were previously determined by a commercial enzyme-linked immunosorbent assay (MBS2019923; MyBioSource, San Diego, CA, USA) (35). The GOAT enzyme-linked immunosorbent assay kit shows a detection limit lower than 0.31 ng/mL and a detection range of 0.78 to 50 ng/mL, as well as an intra- and interassay accuracy with a coefficient of variation lower than 10% and 12%, respectively. Measurements of circulating PSA (7k70; Abbott, Madrid, Spain), cholesterol (7D62/20; Abbott), triglycerides (7D74-20; Abbott), glucose (3L82-20; Abbott), glycated hemoglobin (HbA1c; 270-2000; Abbott), C-reactive protein (CRP; 6k26-30/41; Abbott), homocysteine (01L7127-KT, Abbott) and insulin (RE53171; TECAN, Barcelona, Spain) levels were performed in the laboratory service of the Reina Sofia University Hospital of Córdoba following the manufacturer’s instructions.
Statistical Analyses
Statistical differences between 2 groups were calculated by unpaired parametric t test or a nonparametric Mann-Whitney U test, according to normality, assessed by the Kolmogorov-Smirnov test. For differences between the 3 groups, a 1-way analysis of variance analyses was performed. For cohorts’ clinical descriptive characteristics comparison, a chi-square test and an analysis of variance test with Bonferroni post hoc analysis were performed. Spearman’s or Pearson’s bivariate correlations were performed for quantitative variables according to normality. Statistical analyses were assessed using GraphPad Prism 7 (GraphPad Software, La Jolla, CA, USA) or SPSS version 17.0. (IBM, Armonk, NY, USA). Univariate and multivariable logistic regression analyses were performed to assess the risk factors for PCa. The multivariable models are fitted by adjusting the effect of urine In1-ghrelin, with the most common clinical variables, PSA and metabolic-related parameters. Furthermore, the receiver operating characteristic (ROC) curve and the area under the ROC curve (AUC) were analyzed for fitted models using the pROC R package. The logistic regression models and ROC analysis were assessed using R software (version 4.0.3.; R Foundation, Vienna, Austria). Bootstrap CIs were computed with 2000 stratified bootstrap replicates for AUC values. Statistical significance was considered when P < 0.05. A trend was considered when P < 0.1.
Results
Description of the Cohort
Clinical characteristics of the patients included in this study are detailed in Table 1. Specifically, patients with PCa were older than those with negative biopsy result. The percentage of patients with suspicious digital rectal exam (DRE) was higher in PCa group compared with NegBiopsy group. Patients with PCa had higher BMI and waist circumference (WC) compared to NegBiopsy patients. In addition, the proportion of patients with diabetes mellitus 2 (DM2) was higher in the PCa group as compared to the NegBiopsy group. Consequently, patients with PCa exhibited higher levels of glucose and insulin as well as a greater proportion of HbA1c compared to patients with negative biopsy. On the contrary, PCa patients had lower cholesterol levels than NegBiopsy patients. Regarding cholesterol fractions, PCa patients had lower low-density lipoprotein cholesterol levels than NegBiopsy patients while non-statistically significant changes were observed when analyzing high-density lipoprotein cholesterol levels. Patients with PCa had higher circulating CRP and GOAT levels as well as higher urine In1-ghrelin levels as compared with NegBiopsy patients. However, no statistically significant differences in circulating PSA, triglycerides, and testosterone levels were found between groups.
. | Negative biopsy (n = 397) . | PCa (n = 213) . | P-value . | Total (n = 610) . |
---|---|---|---|---|
Age, years | 63.0 (57.0-68.0) | 65.0 (61.0-71.0) | <0.001 | 64.0 (58.0-69.0) |
Suspicious DRE, yes | 37 (9.32%) | 56 (26.30%) | <0.001 | 93 (15.25%) |
PSA, ng/mL | 5.21 (5.35-5.68) | 5.42 (5.54-6.06) | 0.067 | 5.26 (5.47-5.76) |
BMI, kg/m2 | 28.1 (25.9-30.9) | 28.7 (26.7-32.0) | 0.019 | 28.4 (26.1-31.2) |
Waist circumference, cm | 102 (95.0-110) | 105 (99.0-112) | 0.002 | 103 (96.0-110) |
Diabetes mellitus 2, yes | 59 (14.86) | 48 (22.52) | 0.018 | 107 (17.54) |
Glucose, mg/dL | 98.0 (89.0-108) | 101 (91.0-114) | 0.015 | 98.0 (90.0-111) |
Insulin, ng/mL | 7.90 (5.70-10.9) | 8.65 (6.10-12.8) | 0.032 | 8.10 (5.80-11.5) |
HbA1c, % | 5.50 (5.20-5.80) | 5.65 (5.30-6.00) | 0.014 | 5.50 (5.20-5.90) |
Triglycerides, mg/dL | 110 (83.0-145) | 111 (85.0-150) | 0.546 | 110 (83.2-149) |
Cholesterol, ng/mL | 205 (183-228) | 198 (176-225) | 0.040 | 204 (181-226) |
LDL-C, mg/dL | 135 (113-155) | 127 (102.5-149) | 0.010 | 132.5 (109.3-153) |
HDL-C, mg/dL | 46 (39-53) | 45 (39-52.5) | 0.823 | 45 (39-53) |
CRP, mg/L | 1.90 (1.10-3.80) | 2.30 (1.20-4.80) | 0.045 | 2.00 (1.20-4.00) |
Testosterone, ng/mL | 5.04 (3.95-6.42) | 4.74 (3.92-5.98) | 0.231 | 4.99 (3.94-6.16) |
GOAT enzyme, ng/mL | 218 (129-363) | 307 (199-601) | <0.001 | 243 (146-475) |
In1-ghrelin, pg/mL | 0.24 (0.00-0.53) | 0.35 (0.00-0.69) | 0.001 | 0.27 (0.00-0.57) |
. | Negative biopsy (n = 397) . | PCa (n = 213) . | P-value . | Total (n = 610) . |
---|---|---|---|---|
Age, years | 63.0 (57.0-68.0) | 65.0 (61.0-71.0) | <0.001 | 64.0 (58.0-69.0) |
Suspicious DRE, yes | 37 (9.32%) | 56 (26.30%) | <0.001 | 93 (15.25%) |
PSA, ng/mL | 5.21 (5.35-5.68) | 5.42 (5.54-6.06) | 0.067 | 5.26 (5.47-5.76) |
BMI, kg/m2 | 28.1 (25.9-30.9) | 28.7 (26.7-32.0) | 0.019 | 28.4 (26.1-31.2) |
Waist circumference, cm | 102 (95.0-110) | 105 (99.0-112) | 0.002 | 103 (96.0-110) |
Diabetes mellitus 2, yes | 59 (14.86) | 48 (22.52) | 0.018 | 107 (17.54) |
Glucose, mg/dL | 98.0 (89.0-108) | 101 (91.0-114) | 0.015 | 98.0 (90.0-111) |
Insulin, ng/mL | 7.90 (5.70-10.9) | 8.65 (6.10-12.8) | 0.032 | 8.10 (5.80-11.5) |
HbA1c, % | 5.50 (5.20-5.80) | 5.65 (5.30-6.00) | 0.014 | 5.50 (5.20-5.90) |
Triglycerides, mg/dL | 110 (83.0-145) | 111 (85.0-150) | 0.546 | 110 (83.2-149) |
Cholesterol, ng/mL | 205 (183-228) | 198 (176-225) | 0.040 | 204 (181-226) |
LDL-C, mg/dL | 135 (113-155) | 127 (102.5-149) | 0.010 | 132.5 (109.3-153) |
HDL-C, mg/dL | 46 (39-53) | 45 (39-52.5) | 0.823 | 45 (39-53) |
CRP, mg/L | 1.90 (1.10-3.80) | 2.30 (1.20-4.80) | 0.045 | 2.00 (1.20-4.00) |
Testosterone, ng/mL | 5.04 (3.95-6.42) | 4.74 (3.92-5.98) | 0.231 | 4.99 (3.94-6.16) |
GOAT enzyme, ng/mL | 218 (129-363) | 307 (199-601) | <0.001 | 243 (146-475) |
In1-ghrelin, pg/mL | 0.24 (0.00-0.53) | 0.35 (0.00-0.69) | 0.001 | 0.27 (0.00-0.57) |
Values are expressed in median (interquartile range) for quantitative variables and in absolute number (%) for qualitative variables. Statistical tests: Student’s t-test for quantitative variables and chi-squared for qualitative tests.
Abbreviations: BMI, body mass index; CRP, C-reactive protein; DRE, digital rectal exam; HbA1c, glycated hemoglobin; HDL-C, high-density lipoprotein cholesterol; LDL-C, low-density lipoprotein cholesterol; PCa, prostate cancer; PSA, prostate-specific antigen.
. | Negative biopsy (n = 397) . | PCa (n = 213) . | P-value . | Total (n = 610) . |
---|---|---|---|---|
Age, years | 63.0 (57.0-68.0) | 65.0 (61.0-71.0) | <0.001 | 64.0 (58.0-69.0) |
Suspicious DRE, yes | 37 (9.32%) | 56 (26.30%) | <0.001 | 93 (15.25%) |
PSA, ng/mL | 5.21 (5.35-5.68) | 5.42 (5.54-6.06) | 0.067 | 5.26 (5.47-5.76) |
BMI, kg/m2 | 28.1 (25.9-30.9) | 28.7 (26.7-32.0) | 0.019 | 28.4 (26.1-31.2) |
Waist circumference, cm | 102 (95.0-110) | 105 (99.0-112) | 0.002 | 103 (96.0-110) |
Diabetes mellitus 2, yes | 59 (14.86) | 48 (22.52) | 0.018 | 107 (17.54) |
Glucose, mg/dL | 98.0 (89.0-108) | 101 (91.0-114) | 0.015 | 98.0 (90.0-111) |
Insulin, ng/mL | 7.90 (5.70-10.9) | 8.65 (6.10-12.8) | 0.032 | 8.10 (5.80-11.5) |
HbA1c, % | 5.50 (5.20-5.80) | 5.65 (5.30-6.00) | 0.014 | 5.50 (5.20-5.90) |
Triglycerides, mg/dL | 110 (83.0-145) | 111 (85.0-150) | 0.546 | 110 (83.2-149) |
Cholesterol, ng/mL | 205 (183-228) | 198 (176-225) | 0.040 | 204 (181-226) |
LDL-C, mg/dL | 135 (113-155) | 127 (102.5-149) | 0.010 | 132.5 (109.3-153) |
HDL-C, mg/dL | 46 (39-53) | 45 (39-52.5) | 0.823 | 45 (39-53) |
CRP, mg/L | 1.90 (1.10-3.80) | 2.30 (1.20-4.80) | 0.045 | 2.00 (1.20-4.00) |
Testosterone, ng/mL | 5.04 (3.95-6.42) | 4.74 (3.92-5.98) | 0.231 | 4.99 (3.94-6.16) |
GOAT enzyme, ng/mL | 218 (129-363) | 307 (199-601) | <0.001 | 243 (146-475) |
In1-ghrelin, pg/mL | 0.24 (0.00-0.53) | 0.35 (0.00-0.69) | 0.001 | 0.27 (0.00-0.57) |
. | Negative biopsy (n = 397) . | PCa (n = 213) . | P-value . | Total (n = 610) . |
---|---|---|---|---|
Age, years | 63.0 (57.0-68.0) | 65.0 (61.0-71.0) | <0.001 | 64.0 (58.0-69.0) |
Suspicious DRE, yes | 37 (9.32%) | 56 (26.30%) | <0.001 | 93 (15.25%) |
PSA, ng/mL | 5.21 (5.35-5.68) | 5.42 (5.54-6.06) | 0.067 | 5.26 (5.47-5.76) |
BMI, kg/m2 | 28.1 (25.9-30.9) | 28.7 (26.7-32.0) | 0.019 | 28.4 (26.1-31.2) |
Waist circumference, cm | 102 (95.0-110) | 105 (99.0-112) | 0.002 | 103 (96.0-110) |
Diabetes mellitus 2, yes | 59 (14.86) | 48 (22.52) | 0.018 | 107 (17.54) |
Glucose, mg/dL | 98.0 (89.0-108) | 101 (91.0-114) | 0.015 | 98.0 (90.0-111) |
Insulin, ng/mL | 7.90 (5.70-10.9) | 8.65 (6.10-12.8) | 0.032 | 8.10 (5.80-11.5) |
HbA1c, % | 5.50 (5.20-5.80) | 5.65 (5.30-6.00) | 0.014 | 5.50 (5.20-5.90) |
Triglycerides, mg/dL | 110 (83.0-145) | 111 (85.0-150) | 0.546 | 110 (83.2-149) |
Cholesterol, ng/mL | 205 (183-228) | 198 (176-225) | 0.040 | 204 (181-226) |
LDL-C, mg/dL | 135 (113-155) | 127 (102.5-149) | 0.010 | 132.5 (109.3-153) |
HDL-C, mg/dL | 46 (39-53) | 45 (39-52.5) | 0.823 | 45 (39-53) |
CRP, mg/L | 1.90 (1.10-3.80) | 2.30 (1.20-4.80) | 0.045 | 2.00 (1.20-4.00) |
Testosterone, ng/mL | 5.04 (3.95-6.42) | 4.74 (3.92-5.98) | 0.231 | 4.99 (3.94-6.16) |
GOAT enzyme, ng/mL | 218 (129-363) | 307 (199-601) | <0.001 | 243 (146-475) |
In1-ghrelin, pg/mL | 0.24 (0.00-0.53) | 0.35 (0.00-0.69) | 0.001 | 0.27 (0.00-0.57) |
Values are expressed in median (interquartile range) for quantitative variables and in absolute number (%) for qualitative variables. Statistical tests: Student’s t-test for quantitative variables and chi-squared for qualitative tests.
Abbreviations: BMI, body mass index; CRP, C-reactive protein; DRE, digital rectal exam; HbA1c, glycated hemoglobin; HDL-C, high-density lipoprotein cholesterol; LDL-C, low-density lipoprotein cholesterol; PCa, prostate cancer; PSA, prostate-specific antigen.
Urine In1-ghrelin Levels Are Dysregulated in Patients With Adverse Metabolic Conditions
In the cohort of patients examined, obese individuals had higher urine In1-ghrelin levels than overweight and normoweight individuals (Fig. 1A). In addition, overweight patients had higher urine In1-ghrelin levels compared with normoweight patients (Fig. 1A). Moreover, patients with DM2 exhibited higher urine In1-ghrelin than those without DM2 (Fig. 1B). Consistently, urine In1-ghrelin levels were significantly and directly correlated with BMI (Fig. 1C) and WC (Fig. 1D). Consequently, insulin, glucose, triglycerides, and homocysteine levels as well as the percentage of HbA1c were directly and positively correlated with urine In1-ghrelin levels (Fig. 1E-1I, respectively). Interestingly, besides GOAT enzyme, glucose, HbA1c, triglycerides, and cholesterol levels, high urine In1-ghrelin levels were also associated with DM2 risk (Table 2).
Univariate analysis of the association of circulant levels of key molecules, including In1-ghrelin, with diabetes mellitus 2 risk and prostate cancer risk in patients with PSA in the grey zone
. | Odds ratio (95% CI) . | P-value . |
---|---|---|
Diabetes mellitus 2 risk | ||
In1-ghrelin | 2.96 (1.76-4.99) | <0.001 |
GOAT | 1.54 (1.23-1.93) | <0.001 |
Glucose | 32.9 (6.86-157.78) | <0.001 |
Insulin | 1.03 (1.00-1.08) | 0.086 |
HbA1c | 12.1 (7.32-20.0) | <0.001 |
Triglycerides | 2.05 (1.24-3.40) | 0.005 |
Cholesterol | 0.02 (0.00-0.006) | <0.001 |
PSA | 1.08 (0.96-1.21) | 0.333 |
Prostate cancer risk | ||
In1-ghrelin | 2.93 (1.85-4.64) | <0.0001 |
PSA | 1.09 (0.99-1.20) | 0.068 |
. | Odds ratio (95% CI) . | P-value . |
---|---|---|
Diabetes mellitus 2 risk | ||
In1-ghrelin | 2.96 (1.76-4.99) | <0.001 |
GOAT | 1.54 (1.23-1.93) | <0.001 |
Glucose | 32.9 (6.86-157.78) | <0.001 |
Insulin | 1.03 (1.00-1.08) | 0.086 |
HbA1c | 12.1 (7.32-20.0) | <0.001 |
Triglycerides | 2.05 (1.24-3.40) | 0.005 |
Cholesterol | 0.02 (0.00-0.006) | <0.001 |
PSA | 1.08 (0.96-1.21) | 0.333 |
Prostate cancer risk | ||
In1-ghrelin | 2.93 (1.85-4.64) | <0.0001 |
PSA | 1.09 (0.99-1.20) | 0.068 |
Abbreviations: GOAT, ghrelin O-acyl transferase; HbA1c, glycated hemoglobin; PSA, prostate-specific antigen.
Univariate analysis of the association of circulant levels of key molecules, including In1-ghrelin, with diabetes mellitus 2 risk and prostate cancer risk in patients with PSA in the grey zone
. | Odds ratio (95% CI) . | P-value . |
---|---|---|
Diabetes mellitus 2 risk | ||
In1-ghrelin | 2.96 (1.76-4.99) | <0.001 |
GOAT | 1.54 (1.23-1.93) | <0.001 |
Glucose | 32.9 (6.86-157.78) | <0.001 |
Insulin | 1.03 (1.00-1.08) | 0.086 |
HbA1c | 12.1 (7.32-20.0) | <0.001 |
Triglycerides | 2.05 (1.24-3.40) | 0.005 |
Cholesterol | 0.02 (0.00-0.006) | <0.001 |
PSA | 1.08 (0.96-1.21) | 0.333 |
Prostate cancer risk | ||
In1-ghrelin | 2.93 (1.85-4.64) | <0.0001 |
PSA | 1.09 (0.99-1.20) | 0.068 |
. | Odds ratio (95% CI) . | P-value . |
---|---|---|
Diabetes mellitus 2 risk | ||
In1-ghrelin | 2.96 (1.76-4.99) | <0.001 |
GOAT | 1.54 (1.23-1.93) | <0.001 |
Glucose | 32.9 (6.86-157.78) | <0.001 |
Insulin | 1.03 (1.00-1.08) | 0.086 |
HbA1c | 12.1 (7.32-20.0) | <0.001 |
Triglycerides | 2.05 (1.24-3.40) | 0.005 |
Cholesterol | 0.02 (0.00-0.006) | <0.001 |
PSA | 1.08 (0.96-1.21) | 0.333 |
Prostate cancer risk | ||
In1-ghrelin | 2.93 (1.85-4.64) | <0.0001 |
PSA | 1.09 (0.99-1.20) | 0.068 |
Abbreviations: GOAT, ghrelin O-acyl transferase; HbA1c, glycated hemoglobin; PSA, prostate-specific antigen.
![Correlations and associations between urine In1-ghrelin levels and key metabolic parameters. Association of urine In1-ghrelin levels with patients categorized by BMI [normoweight (NW; BMI ≤ 25), overweight (OW; BMI > 25), obesity (OB; BMI ≥ 30)] (A) and the presence of diabetes mellitus 2 (B) in PCa and NegBiopsy patients with PSA in the grey zone (n = 610). Correlation of urine In1-ghrelin levels with BMI (C), waist circumference (WC; D), insulin levels (E), glucose levels (F), triglycerides levels (G), homocysteine levels (H), and HbA1c levels (I) in PCa and NegBiopsy patients with PSA in the grey zone (n = 610). **P < 0.01 and ***P < 0.001 indicate values that significantly differ between groups. Coefficients of correlation (R) were evaluated by Pearson’s test.](https://oup.silverchair-cdn.com/oup/backfile/Content_public/Journal/jcem/106/12/10.1210_clinem_dgab516/1/m_dgab516f0001.jpeg?Expires=1750274351&Signature=Wj2MX9NRuFDkwrJ29eD-jE-sy80t1mgvTFXrg3OC-Pd1SkJhrwOe1NmBXPkPDUT6XkbnixiFVz177bVlyXxqAMUAMpb3-m7mOTCj3RqJkWbpzi2-qepo9lIrxlBTvxUC7jSHu-S~4StJgS7~hnwz55-49asDB6NzrXixUf7TkJ6dxaiRA4YRBCH7aDvb9~xnyHULP4F~MXOAWcw6qVm8T7qO6zNYhAxaezQc-l2zLPvYM-co~0wyxtfgAQJYAh~rvQkaou~hsorHWFlc7IX2u2c04TuGLrdtu~3~lG9J8unXhNNHicAI7cJwYG0VYWjbykkfJ9SEPd~kSV5sexynrg__&Key-Pair-Id=APKAIE5G5CRDK6RD3PGA)
Correlations and associations between urine In1-ghrelin levels and key metabolic parameters. Association of urine In1-ghrelin levels with patients categorized by BMI [normoweight (NW; BMI ≤ 25), overweight (OW; BMI > 25), obesity (OB; BMI ≥ 30)] (A) and the presence of diabetes mellitus 2 (B) in PCa and NegBiopsy patients with PSA in the grey zone (n = 610). Correlation of urine In1-ghrelin levels with BMI (C), waist circumference (WC; D), insulin levels (E), glucose levels (F), triglycerides levels (G), homocysteine levels (H), and HbA1c levels (I) in PCa and NegBiopsy patients with PSA in the grey zone (n = 610). **P < 0.01 and ***P < 0.001 indicate values that significantly differ between groups. Coefficients of correlation (R) were evaluated by Pearson’s test.
Urine In1-ghrelin Levels Are Associated With Relevant Hormones and Inflammatory Proteins
Urine In1-ghrelin levels were directly correlated with plasma levels of PSA, GOAT enzyme, and beta2-microglobulin (Fig. 2A-2C, respectively). On the contrary, urine In1-ghrelin levels were slightly and inversely correlated (r = −0.096) with circulating testosterone levels (38). Furthermore, circulating levels of CRP tended to be weakly and directly correlated (r = 0.074) with urine In1-ghrelin levels (P = 0.076) (38).
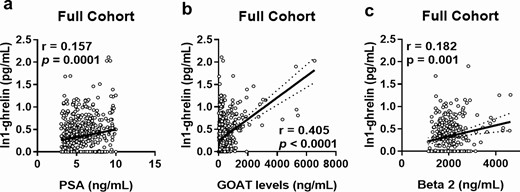
Correlations and associations between urine In1-ghrelin levels and relevant clinical parameters. Correlation of urine In1-ghrelin levels with those of plasma PSA (A), urine GOAT enzyme (B) and plasma beta2-microglobulin (C) in PCa and NegBiopsy patients with PSA in the grey zone (n = 610).
Urine In1-ghrelin Levels Are Elevated in PCa Patients and Linked to PCa Aggressiveness Parameters and PCa Risk
We found that urine In1-ghrelin levels were significantly higher in patients with PCa compared with NegBiopsy patients (Fig. 3A). Likewise, PCa patients tended to have higher plasma PSA levels (P = 0.065) than NegBiopsy patients (Fig. 3B). Remarkably, ROC analyses showed that urine In1-ghrelin levels, but not plasma PSA levels, significantly distinguished between patients with PCa and NegBiopsy patients in this cohort (Fig. 3C). Moreover, univariate analyses showed that high urine In1-ghrelin, but not PSA, levels were associated with an increased PCa risk (Table 2). Furthermore, since In1-ghrelin expression in PCa tissues has been previously reported to be associated with PCa progression (19), we then focused on interrogating the potential relationship between urine In1-ghrelin levels and relevant parameters of PCa aggressiveness. Specifically, we explored the pathological characteristics of the tumors of some patients from our cohort who underwent radical prostatectomy (n = 79). Interestingly, we found that urine In1-ghrelin levels were positively correlated with tumor stage (Fig. 3D). Moreover, high urine In1-ghrelin levels were associated with lymphovascular invasion (Fig. 3E) and tended to be associated with perineural invasion (P = 0.099) (Fig. 3F).
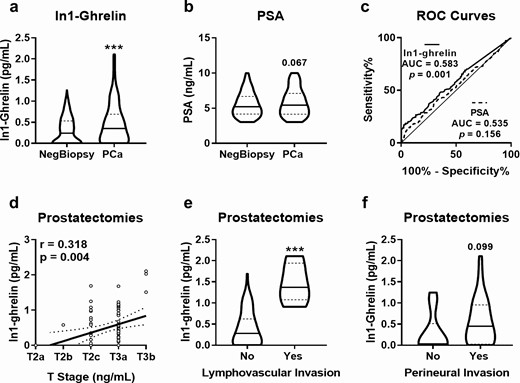
Comparison between urine In1-ghrelin levels and plasma PSA levels. Urine In1-ghrelin levels (A) and plasma PSA levels (B) were determined in patients diagnosed with PCa (n = 213) and patients with negative biopsy result (NegBiopsy; n = 397). (C) AUC of plasma PSA and urine In1-ghrelin to distinguish between PCa and NegBiopsy patients. Association of urine In1-ghrelin levels with tumor stage (T stage; D), lymphovascular invasion (E) and perineural invasion (F) in patients with PCa with PSA in the grey zone who underwent radical prostatectomy (n = 79). ***P < 0.001 indicate values that significantly differ between groups. Coefficients of correlation (R) were evaluated by Pearson’s test.
Diagnostic and Predictive Value of Urine In1-ghrelin Levels in PCa
To further characterize the potential diagnostic value of urine In1-ghrelin levels in PCa, we compared the diagnostic capacity of a clinical model comprised by age, abnormal DRE, previous biopsy, and PSA levels [factors that have been commonly reported to be associated with PCa risk (39-42)], adding or not the urine In1-ghrelin levels variable to the clinical model. Specifically, ROC analyses showed that the accuracy for the diagnosis of PCa patients was significantly higher when urine In1-ghrelin levels were added to the clinical model (AUC: 0.705 with 95% CI bootstrap: 0.6601-0.747 vs 0.682 with 95% CI bootstrap: 0.6364-0.7264; P = 0.024) (Fig. 4). Furthermore, urine In1-ghrelin levels, DRE, age, and prior biopsy were independently associated with an increased risk of PCa, being In1-ghrelin the variable with the most pronounced predictive value (odds ratio = 3.121, P < 0.0001) (Table 3). On the other hand, circulating PSA levels were not associated with PCa risk in our cohort of patients (P = 0.326) (Table 3).
Multivariate analysis of the association of urine In1-ghrelin levels with prostate cancer risk in patients with PSA in the grey zone, adjusting with common clinical variables
. | Odds ratio (95% CI) . | P-value . |
---|---|---|
In1-ghrelin | 3.121 (1.932-5.111) | <0.0001 |
DRE (suspicious) | 2.756 (1.679-4.550) | <0.0001 |
Prior biopsy | 0.446 (0.272-0.713) | <0.0001 |
Age | 1.042 (1.016-1.070) | 0.002 |
PSA | 1.058 (0.945-1.183) | 0.326 |
. | Odds ratio (95% CI) . | P-value . |
---|---|---|
In1-ghrelin | 3.121 (1.932-5.111) | <0.0001 |
DRE (suspicious) | 2.756 (1.679-4.550) | <0.0001 |
Prior biopsy | 0.446 (0.272-0.713) | <0.0001 |
Age | 1.042 (1.016-1.070) | 0.002 |
PSA | 1.058 (0.945-1.183) | 0.326 |
Abbreviations: DRE, digital rectal exam; PSA, prostate-specific antigen.
Multivariate analysis of the association of urine In1-ghrelin levels with prostate cancer risk in patients with PSA in the grey zone, adjusting with common clinical variables
. | Odds ratio (95% CI) . | P-value . |
---|---|---|
In1-ghrelin | 3.121 (1.932-5.111) | <0.0001 |
DRE (suspicious) | 2.756 (1.679-4.550) | <0.0001 |
Prior biopsy | 0.446 (0.272-0.713) | <0.0001 |
Age | 1.042 (1.016-1.070) | 0.002 |
PSA | 1.058 (0.945-1.183) | 0.326 |
. | Odds ratio (95% CI) . | P-value . |
---|---|---|
In1-ghrelin | 3.121 (1.932-5.111) | <0.0001 |
DRE (suspicious) | 2.756 (1.679-4.550) | <0.0001 |
Prior biopsy | 0.446 (0.272-0.713) | <0.0001 |
Age | 1.042 (1.016-1.070) | 0.002 |
PSA | 1.058 (0.945-1.183) | 0.326 |
Abbreviations: DRE, digital rectal exam; PSA, prostate-specific antigen.
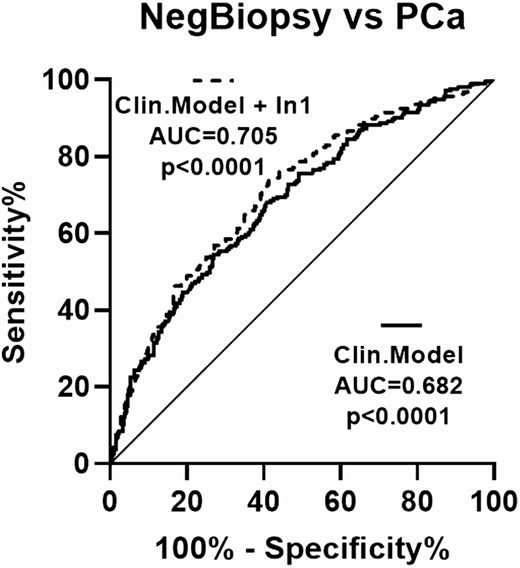
Diagnostic capacity of a clinical model with and without urine In1-ghrelin levels. Comparison between the AUCs of a clinical model consisting of age, suspicious DRE, previous biopsy, and PSA levels including or not urine In1-ghrelin levels to distinguish between PCa patients (n = 213) and NegBiopsy patients (n = 397) with PSA in the grey zone. Differences among AUCs were determined using the De Long test.
The Addition of Metabolic Factors, Including In1-ghrelin, to a Clinical Multivariate Model Improves the Capacity to Diagnose PCa
Obesity and MetS as well as the dysregulation of numerous metabolic factors have been also widely associated to PCa risk (26,43-48). In fact, in the present study we found that high BMI; WC; the presence of DM2; and high glucose, insulin, Hb1Ac, cholesterol, and GOAT levels (but not triglycerides, CRP, and testosterone levels) were associated with an increased risk of PCa in our cohort of patients with PSA ranging from 3 to 10 ng/mL (Table 4). Therefore, we asked whether the addition of these relevant metabolic factors, including urine In1-ghrelin (which was also found to be associated with an increased risk of PCa in the present study) (Table 2) could improve the diagnostic capacity of PCa when they are combined with the clinical multivariate model used before (ie, combining age, abnormal DRE, previous biopsy, and PSA levels).
Univariate analysis of the association of metabolic-related parameters with prostate cancer risk in patients with PSA in the grey zone
. | Odds ratio (95% CI) . | P-value . |
---|---|---|
BMI | 1.05 (1.01-1.09) | 0.027 |
WC | 13.7 (2.39-78.9) | 0.003 |
DM2 | 1.01 (1.00-1.01) | 0.002 |
Glucose | 2.95 (1.36-6.39) | 0.006 |
Insulin | 1.04 (1.00-1.07) | 0.028 |
HbA1c | 1.28 (1.03-1.59) | 0.026 |
Cholesterol | 0.31 (0.12-0.79) | 0.015 |
GOAT | 1.63 (1.34-1.98) | <0.001 |
Triglycerides | 1.01 (0.68-1.52) | 0.946 |
CRP | 1.02 (0.98-1.05) | 0.345 |
Testosterone | 0.93 (0.85-1.02) | 0.142 |
. | Odds ratio (95% CI) . | P-value . |
---|---|---|
BMI | 1.05 (1.01-1.09) | 0.027 |
WC | 13.7 (2.39-78.9) | 0.003 |
DM2 | 1.01 (1.00-1.01) | 0.002 |
Glucose | 2.95 (1.36-6.39) | 0.006 |
Insulin | 1.04 (1.00-1.07) | 0.028 |
HbA1c | 1.28 (1.03-1.59) | 0.026 |
Cholesterol | 0.31 (0.12-0.79) | 0.015 |
GOAT | 1.63 (1.34-1.98) | <0.001 |
Triglycerides | 1.01 (0.68-1.52) | 0.946 |
CRP | 1.02 (0.98-1.05) | 0.345 |
Testosterone | 0.93 (0.85-1.02) | 0.142 |
Abbreviations: BMI, body mass index; CRP, C-reactive protein; DM2, diabetes mellitus 2; GOAT, ghrelin O-acyl transferase; HbA1c, glycated hemoglobin; WC, waist circumference.
Univariate analysis of the association of metabolic-related parameters with prostate cancer risk in patients with PSA in the grey zone
. | Odds ratio (95% CI) . | P-value . |
---|---|---|
BMI | 1.05 (1.01-1.09) | 0.027 |
WC | 13.7 (2.39-78.9) | 0.003 |
DM2 | 1.01 (1.00-1.01) | 0.002 |
Glucose | 2.95 (1.36-6.39) | 0.006 |
Insulin | 1.04 (1.00-1.07) | 0.028 |
HbA1c | 1.28 (1.03-1.59) | 0.026 |
Cholesterol | 0.31 (0.12-0.79) | 0.015 |
GOAT | 1.63 (1.34-1.98) | <0.001 |
Triglycerides | 1.01 (0.68-1.52) | 0.946 |
CRP | 1.02 (0.98-1.05) | 0.345 |
Testosterone | 0.93 (0.85-1.02) | 0.142 |
. | Odds ratio (95% CI) . | P-value . |
---|---|---|
BMI | 1.05 (1.01-1.09) | 0.027 |
WC | 13.7 (2.39-78.9) | 0.003 |
DM2 | 1.01 (1.00-1.01) | 0.002 |
Glucose | 2.95 (1.36-6.39) | 0.006 |
Insulin | 1.04 (1.00-1.07) | 0.028 |
HbA1c | 1.28 (1.03-1.59) | 0.026 |
Cholesterol | 0.31 (0.12-0.79) | 0.015 |
GOAT | 1.63 (1.34-1.98) | <0.001 |
Triglycerides | 1.01 (0.68-1.52) | 0.946 |
CRP | 1.02 (0.98-1.05) | 0.345 |
Testosterone | 0.93 (0.85-1.02) | 0.142 |
Abbreviations: BMI, body mass index; CRP, C-reactive protein; DM2, diabetes mellitus 2; GOAT, ghrelin O-acyl transferase; HbA1c, glycated hemoglobin; WC, waist circumference.
First, given that urine In1-ghrelin levels correlated with the previously mentioned metabolic factors (Fig. 1), we tested whether urine In1-ghrelin levels were able to predict PCa independently of these metabolic factors. Specifically, a multivariate analysis showed that the association between urine In1-ghrelin levels and PCa risk was independent of BMI; WC; DM2; and glucose, insulin, cholesterol, and GOAT levels (Table 5). In addition, the multivariate metabolic model comprised of BMI; WC; DM2; and glucose, insulin, HbA1c, cholesterol, GOAT enzyme, and In1-ghrelin levels was able to distinguish between PCa and NegBiopsy patients with an AUC of 0.636 (95% CI bootstrap: 0.577-0.694) (Fig. 5). Notably, De Long analysis showed that the capacity of a model combining these metabolic factors (ie, BMI, WC, DM2, glucose, insulin, HbA1c, cholesterol, GOAT enzyme, and In1-ghrelin) with previously used key clinical factors (ie, age, abnormal DRE, previous biopsy, and PSA levels) to distinguish PCa and NegBiopsy patients was significantly higher (AUC = 0.740, with 95% CI bootstrap: 0.689-0.785) than that of the metabolic model alone (AUC = 0.636; P < 0.001) and the clinical model alone (AUC = 0.682; P = 0.05).
Multivariate analysis of the association of urine In1-ghrelin levels with prostate cancer risk in patients with PSA in the grey zone adjusting, with metabolic-related parameters
. | Odds ratio (95% CI) . | P-value . |
---|---|---|
BMI | 0.91 (0.81-1.022) | 0.118 |
WC | 1.03 (0.99-1.08) | 0.127 |
DM2 | 1.00 (0.99-1.01) | 0.752 |
Glucose | 1.00 (0.99-1.01) | 0.489 |
Insulin | 1.02 (0.98-1.06) | 0.344 |
Cholesterol | 1.00 (0.99-1.00) | 0.685 |
GOAT | 1.00 (0.99-1.00) | 0.685 |
In1-ghrelin | 2.83 (1.51-5.39) | 0.001 |
. | Odds ratio (95% CI) . | P-value . |
---|---|---|
BMI | 0.91 (0.81-1.022) | 0.118 |
WC | 1.03 (0.99-1.08) | 0.127 |
DM2 | 1.00 (0.99-1.01) | 0.752 |
Glucose | 1.00 (0.99-1.01) | 0.489 |
Insulin | 1.02 (0.98-1.06) | 0.344 |
Cholesterol | 1.00 (0.99-1.00) | 0.685 |
GOAT | 1.00 (0.99-1.00) | 0.685 |
In1-ghrelin | 2.83 (1.51-5.39) | 0.001 |
Abbreviations: BMI, body mass index; DM2, diabetes mellitus 2; GOAT, ghrelin O-acyl transferase; WC, waist circumference.
Multivariate analysis of the association of urine In1-ghrelin levels with prostate cancer risk in patients with PSA in the grey zone adjusting, with metabolic-related parameters
. | Odds ratio (95% CI) . | P-value . |
---|---|---|
BMI | 0.91 (0.81-1.022) | 0.118 |
WC | 1.03 (0.99-1.08) | 0.127 |
DM2 | 1.00 (0.99-1.01) | 0.752 |
Glucose | 1.00 (0.99-1.01) | 0.489 |
Insulin | 1.02 (0.98-1.06) | 0.344 |
Cholesterol | 1.00 (0.99-1.00) | 0.685 |
GOAT | 1.00 (0.99-1.00) | 0.685 |
In1-ghrelin | 2.83 (1.51-5.39) | 0.001 |
. | Odds ratio (95% CI) . | P-value . |
---|---|---|
BMI | 0.91 (0.81-1.022) | 0.118 |
WC | 1.03 (0.99-1.08) | 0.127 |
DM2 | 1.00 (0.99-1.01) | 0.752 |
Glucose | 1.00 (0.99-1.01) | 0.489 |
Insulin | 1.02 (0.98-1.06) | 0.344 |
Cholesterol | 1.00 (0.99-1.00) | 0.685 |
GOAT | 1.00 (0.99-1.00) | 0.685 |
In1-ghrelin | 2.83 (1.51-5.39) | 0.001 |
Abbreviations: BMI, body mass index; DM2, diabetes mellitus 2; GOAT, ghrelin O-acyl transferase; WC, waist circumference.
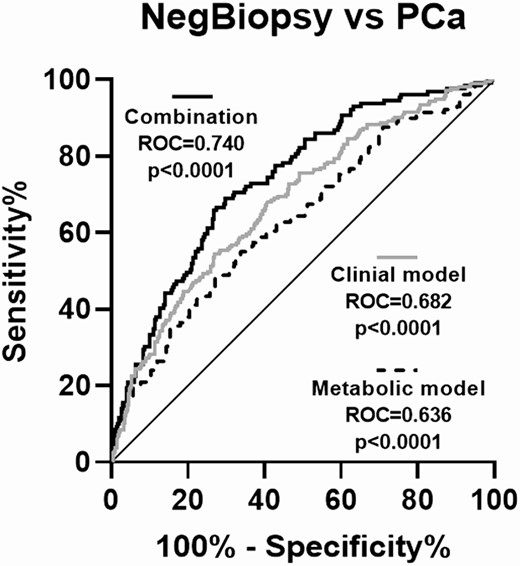
Diagnostic capacity of a clinical model, a metabolic model and a combination of both models. AUCs to distinguish between PCa (n = 213) and NegBiopsy patients (n = 397) in the grey zone of a clinical model (consisting of age, suspicious DRE, previous biopsy, and PSA levels), a metabolic model (consisting of BMI, WC, diabetes mellitus 2, and plasma/urine levels of glucose, insulin, HbA1c, cholesterol, GOAT enzyme, and In1-ghrelin), and a combination of both models. Differences among AUCs were determined using the De Long test.
Discussion
In1-ghrelin is a splicing variant generated from the preproghrelin messenger RNA, specifically by the retention of the intron 1, resulting in a truncated prepropeptide (13,15). In vitro experiments demonstrated that In1-ghrelin is a functionally active molecule that exerts both similar and different functions and regulates both common and distinct signaling pathways than ghrelin (1,19). Interestingly, the levels of both ghrelin and In1-ghrelin have been reported to be influenced by adverse metabolic conditions (13,21,22). Specifically, we demonstrated that the expression levels of In1-ghrelin were significantly higher than those of the native ghrelin in the pituitary and hypothalamus of mice and that the expression of this splicing variant, but not ghrelin, was altered in these metabolic and central tissues in response to fasting and/or obesity conditions (13). However, the relation of In1-ghrelin with metabolic status has not been extensively studied to date in humans. For that reason, herein we analyzed, for the first time, the urine levels of In1-ghrelin in 610 patients metabolically well characterized.
Our results showed that urine In1-ghrelin levels were markedly elevated in obese patients as well as in diabetic patients. Moreover, high urine In1-ghrelin levels were associated with DM2 in our cohort of patients. Notably, we also found that the circulating levels of some key factors characteristically related to obesity/DM2 (ie, glucose, insulin, triglycerides, and HbA1c) were positively correlated with urine In1-ghrelin levels. In this sense, Seim et al showed that in vitro treatment with insulin increased the expression of In1-ghrelin in PCa cells (49), which is consistent with our results. Taken together, our data indicate that In1-ghrelin levels are dysregulated in altered metabolic conditions and associated with relevant obesity-related factors, which pave the way to study this potential association in metabolic-related pathologies.
In this scenario, the development and progression of several endocrine-related cancers are deeply influenced by adverse metabolic conditions, including MetS and obesity (50,51). Among them, PCa, which represents the most common cancer among men in developed countries (52), is especially impacted by the metabolic status of the patient at both molecular and clinical levels (28,53). Herein, we showed that the presence of obesity and/or DM2, as well as elevated levels of glucose, insulin, HbA1c, and cholesterol were associated to a higher PCa risk in our cohort of patients, which is consistent with previous studies (28,54-56). In this sense, MetS, a clinical condition consisting of a combination of pathological alterations (ie, obesity, insulin resistance, elevated blood pressure, and triglyceride levels and decreased cholesterol levels), has been reported to be associated with increased risk of developing PCa and linked to a more aggressive disease (27,28). Indeed, it has been reported that the greater number of MetS criteria meant the greater risk of developing PCa (26,29). Among the MetS criteria, obesity has been especially related to PCa risk and even associated with a more aggressive disease (25,28,30). However, although in our cohort of patients the presence of DM2 was significantly and positively associated with PCa risk, the role of DM2 in PCa biology remains controversial, since both a PCa-protective and PCa-promoting roles have been proposed for this metabolic disease in previous studies (57,58). Therefore, further studies with a prospective design would be essential to validate the potential pathological relation between DM2 and PCa found in our study.
Importantly, urine GOAT enzyme levels were one of the metabolic parameters found to be associated with urine In1-ghrelin levels. Specifically, a positive correlation was found between these 2 components of the ghrelin systems in our cohort of patients. In this sense, GOAT has been previously reported to be dysregulated in response to metabolic stress and to be closely associated with In1-ghrelin levels but not to ghrelin levels in certain cell types (19,22). Thus, our data reinforce the hypothesis that the main substrate of GOAT enzyme in certain conditions, especially in tumor cells, could be In1-ghrelin instead of native ghrelin. Regardless, our data confirm the hypothesis that an adverse metabolic status is related to PCa risk and aggressiveness. Likewise, these findings point to the importance of analyzing the role of metabolic- and obesity-related factors as potential biomarkers for PCa diagnosis and progression.
In this scenario, PCa screening method is mainly based on the measurement of PSA plasma levels (59). Given the low specificity of the PSA test, especially when PSA levels are in the grey zone (ie, 3-10 ng/mL), patients with suspected PCa must undergo prostate biopsies to confirm the presence of the tumor (23). Unfortunately, prostate biopsies are commonly associated with numerous side effects, including bleeding, infections, and pain (24). Therefore, additional noninvasive biomarkers that enhance or outperform the diagnostic value of PSA, especially in its grey zone, are urgently needed to avoid unnecessary biopsies and their associated side effects. In this sense, we have previously reported in a limited cohort of patients that plasma levels of In1-ghrelin, but not those of ghrelin, are elevated in patients with PCa and could distinguish between PCa patients and healthy individuals (19), suggesting that In1-ghrelin could be much more promising than ghrelin as a potential noninvasive diagnostic biomarker for PCa. However, the presence and the potential dysregulation of In1-ghrelin levels in urine, a fluid that is enriched with prostate secretions (32,33,60), had not been studied in patients at risk of PCa or with the disease. Specifically, urine could represent a much more promising source of PCa biomarkers inasmuch as the potential secretions drained into the urethra are diluted into a smaller volume and immediately excreted (ie, urination), while the biomarkers in blood and serum are diluted in the circulation and could be altered by different physiological processes (eg, liver metabolism). In this sense, although we do not have direct evidence showing that ln1-ghrelin measured in urine is more prostate specific than plasma, previous studies in which the levels of some PCa diagnostic biomarkers (eg, GSTP1, RASSF1, HIST1H4K, and TFAP2E) have been measured in plasma and urine demonstrated that these biomarkers showed a higher sensitivity in urine than in plasma (33). For that reason, we herein investigated the levels of In1-ghrelin in urine samples derived from an ample cohort of patients with PSA ranging from 3 to 10ng/mL (n = 610). Our results showed that In1-ghrelin is detected in urine and its levels are higher in PCa patients compared NegBiopsy patients. In fact, urine In1-ghrelin levels were able to distinguish between PCa patients and NegBiopsy patients more effectively than PSA in patients with PSA in the grey zone. Remarkably, we found that urine In1-ghrelin levels were not only associated with PCa risk but also with tumor stage and lymphovascular invasion, thus suggesting a potential relation of urine In1-ghrelin levels with PCa aggressiveness status even in patients with low levels of PSA. Consistently, our group has previously demonstrated that In1-ghrelin plays a relevant oncogenic role in PCa, since the treatment with In1-ghrelin–derived peptides or its overexpression increased while its silencing decreased PCa aggressiveness features in vitro and/or in vivo (19). Although these data do not directly inform us about the main source of urine In1-ghrelin in these patients or whether urine In1-ghrelin levels may correlate with plasma levels, we hypothesize that at least a relevant proportion of the In1-ghrelin found in the urine of PCa patients may derive from PCa cells and that there would not be necessarily a direct relationship between urine and circulating levels of In1-ghrelin. These conclusions are based on previous studies published by our group and other groups demonstrating that (1) urine is a body fluid enriched in prostate-derived compounds (32,33); (2) the expression of In1-ghrelin is markedly pronounced in PCa cells; and (3) different components of the ghrelin system are secreted by PCa cells (19,61). However, further investigation is required to prove this hypothesis, and therefore it should be taken with caution. These results clearly reinforced the hypothesis that the oncogenic effects exerted by In1-ghrelin levels could be mediated, at least in part, by an autocrine/paracrine regulation of PCa cells.
Given that the metabolic status (ie, obesity and diabetes), as well as the circulating levels of several metabolic-related factors (ie, glucose, insulin, HbA1c, and cholesterol), were related to PCa risk and linked to urine In1-ghrelin levels in our cohort of patients, we wondered whether the relation of In1-ghrelin and PCa risk could be dependent or independent of the aforementioned metabolic parameters. Our results demonstrated that high urine In1-ghrelin levels were independently associated with an increased PCa risk, thus indicating a potentially close relation of In1-ghrelin with metabolic disorders as well as PCa risk. These results pave the way to hypothesize that In1-ghrelin dysregulation could represent one of the molecular mechanisms involved in the well-known pathophysiological relation between PCa and metabolic alterations.
Finally, we found that the addition of a metabolic model comprising urine In1-ghrelin levels and other key metabolic variables (ie, glucose, insulin, BMI, WC, HbA1c, and cholesterol) to a clinical model (ie, age, DRE, previous biopsy, and PSA) significantly outperformed the diagnostic capacity of each of these models, exhibiting an AUC of 0.74 to diagnose PCa in our cohort of patients, and further supporting the pathophysiological relationship between metabolism and PCa. These data, in addition to the fact that obesity impacts PCa diagnosis probably by PSA hemodilution (31), reinforce the idea that metabolic status should be considered as a relevant biological and clinical player in patients at risk of PCa, especially in those with low levels of PSA.
The present study has some limitations. First, despite the prospectively collected information, it followed a retrospective design. Second, only 1 spot sample was taken from each patient since 1 of the main aims of this study was to compare the potential diagnostic value of In1-ghrelin with that of PSA. In this sense, we considered that the most appropriate approach to carry out this aim was to mimic as much as possible the methodology followed for the detection of PSA (ie, collection of blood before the prostate biopsy under fasting conditions as spot sample). Therefore, our study does not include additional samples for confirmation of the results, does not address the potential biological variability or day-to-day fluctuations of In1-ghrelin, and does not include the determination of In1-ghrelin levels in 24-h urine as urine spot samples presumable contain a higher and more consistent levels of prostate secretions due to dilution in 24-h urine. For that reason, future studies with a prospective design and that include serial samples should be performed to address the aforementioned limitations.
Taken together, our results demonstrate that urine In1-ghrelin levels are closely associated with the metabolic status of the patients and to critical metabolic-related factors (ie, obesity; DM2; glucose, insulin, HbA1c, and cholesterol levels) as well as PCa risk and aggressiveness parameters, suggesting that the dysregulation of In1-ghrelin levels in PCa might represent one of the molecular mechanisms underlying the pathophysiological relation between obesity/diabetes and PCa. In addition, although prospective validation is required, we have demonstrated that urine In1-ghrelin levels outperform the capacity of plasma PSA to distinguish between PCa patients and non-PCa patients in the grey zone and could represent a novel and valuable noninvasive biomarker for PCa.
Acknowledgments
Financial Support: This work has been supported by Ministry of Science and Innovation (PID2019-105564RB-I00; PID2019-105201RB-I00); Instituto de Salud Carlos III, co-funded by European Union (ERDF/ESF, “Investing in Your Future”; DTS Grant DTS20/00050, FIS Grant PI16/00264 and PI20/01301; University of Córdoba (Programa Operativo FEDER Andalucía: 27416); Spanish Ministry of Universities (Predoctoral contracts FPU16-05059 and FPU18-02485); Junta de Andalucía (BIO-0139, PI-0094-2020); and CIBERobn. CIBER is an initiative of Instituto de Salud Carlos III, Ministerio de Sanidad, Servicios Sociales e Igualdad, Spain. This project has received funding from the European Union’s Horizon 2020 research and innovation programme under the Marie Skłodowska-Curie grant agreement number 847468.
Additional Information
Disclosure Statement: The authors have nothing to disclose.
Data Availability
Some or all data sets generated during and/or analyzed during the current study are not publicly available but are available from corresponding author on reasonable request.
References
Author notes
These authors co-supervised this work.