-
PDF
- Split View
-
Views
-
Cite
Cite
David R Greig, Vivienne Do Nascimento, Israel Olonade, Craig Swift, Satheesh Nair, Claire Jenkins, Surveillance of antimicrobial resistant Shiga toxin-producing E. coli O157:H7 in England, 2016–2020, Journal of Antimicrobial Chemotherapy, Volume 78, Issue 9, September 2023, Pages 2263–2273, https://doi.org/10.1093/jac/dkad231
- Share Icon Share
Abstract
Shiga toxin-producing Escherichia coli (STEC) O157:H7 are zoonotic pathogens and transmission to humans occurs via contaminated food or contact with infected animals. The aim of this study was to describe the frequency, and distribution across the phylogeny, of antimicrobial resistance (AMR) determinants in STEC O157:H7 isolated from human cases in England.
Short-read whole-genome sequencing data from 1473 isolates of STEC O157:H7 from all seven sub-lineages (Ia-Ic, IIa-IIc and I/II) were mapped to genes known to confer phenotypic resistance to 10 different classes of antibiotic. Long-read sequencing was used to determine the location and genomic architecture of the AMR determinants within phylogenetic clusters exhibiting multidrug resistance.
Overall, 216/1473 (14.7%) isolates had at least one AMR determinant, although the proportion of isolates exhibiting AMR varied by sub-lineage. The highest proportion of AMR determinants were detected in sub-lineages Ib (28/64, 43.7%), I/II (18/51, 35.3%) and IIc (122/440, 27.7%). In all sub-lineages, the most commonly detected AMR determinants conferred resistance to the aminoglycosides, tetracyclines and sulphonamides, while AMR determinants conferring resistance to fluroquinolones, macrolides and third-generation cephalosporins were rarely detected. Long-read sequencing analysis showed that the AMR determinants were co-located on the chromosome in sub-lineages Ib and lineage I/II, whereas those associated with sub-lineage IIc were encoded on the chromosome and/or large plasmids.
AMR genes were unevenly distributed across the different sub-lineages of STEC O157:H7 and between different clades within the same sub-lineage. Long-read sequencing facilitates tracking the transmission of AMR at the pathogen and mobile genetic element level.
Introduction
Surveillance of antimicrobial resistance (AMR) detected in gastrointestinal (GI) pathogens is important from both the clinical and public health perspective. Data on the prevalence of resistance to the different classes of antimicrobials informs guidance on the clinical management and empirical treatment of patients presenting with GI symptoms (https://www.bmj.com/content/372/bmj.n437, https://bestpractice.bmj.com/topics/en-gb/1174). Monitoring of AMR contributes to the tracking of the global spread of multidrug resistant (MDR) GI pathogens isolated from patients reporting traveller’s diarrhoea and provides insight in to emerging MDR, and novel exposures and routes of transmission.1–6 Surveillance of zoonotic, foodborne GI pathogens such as Shiga toxin-producing Escherichia coli (STEC), facilitates monitoring the transmission of AMR from the animal reservoir to humans via the food chain.7,8 The analysis of short and long-read genome sequencing data for routine surveillance of GI pathogens enables us to track the acquisition and intra- and inter-species dissemination of AMR determinates on mobile genetic elements (MGE).9–15
STEC is known to colonize the gastrointestinal tract of healthy ruminants, and cattle studies have shown that approximately 20% of cattle herds are colonized with STEC O157:H7, the most frequently detected STEC serotype in the UK.16 Transmission to humans occurs via the faecal–oral route, either through consumption of contaminated food or through contact with animals or their environments.
There are three main lineages of STEC O157:H7 (I, II and I/II) and seven sub-lineages, Ia-Ic, IIa-IIc and I/II.17,18 Historically, the early outbreaks of STEC O157:H7 in the 1980s were caused by isolates belonging to lineage I/II,17,18 however, over the last 10 years the dominant sub-lineages have been Ic, IIa, IIb and IIc. By contrast, strains belonging to Ia, Ib and I/II have been rarely detected.17,19 In the UK, sub-lineages Ic and IIb are almost entirely linked to domestic acquisition, whereas sub-lineages IIa and IIc are characterized by both domestic and travel-acquired isolates.19
Prior to the implementation of WGS at UK Health Securities Agency (UKHSA), monitoring AMR in STEC was limited, partly because antibiotic treatment of STEC is contraindicated and partly because of the laboratory safety implications of performing extra testing on Hazard Group 3 pathogens. Since 2015, genome derived AMR profiles of all STEC submitted to UKHSA have been available in real-time.7,8 The aim of this study was to describe the occurrence and frequency of AMR determinants in STEC O157:H7 isolates linked to cases resident in England and determine the distribution of AMR determinants across the STEC O157:H7 phylogeny.
Methods
Bacterial strains
In England, faecal specimens from patients with suspected gastrointestinal infection are tested for a range of gastrointestinal pathogens, including STEC O157:H7 [UK SMI S 7: gastroenteritis - GOV.UK (www.gov.uk)]. Isolates are submitted to UKHSA for WGS.17,19
Whole-genome sequencing on the Illumina platform
Genomic DNA was extracted and sequenced on an Illumina HiSeq platform to produce 100 bp short-read sequence fragments (Illumina, Cambridge, UK). AMR determinants were sought using ‘Gene-Finder’, a customized algorithm that uses Bowtie2 (v.2.3.5.1)20 to map reads to a set of reference sequences and Samtools (v.1.8)21 to generate an mpileup file, as previously described.7,8 The presence of resistance genes was defined based on 100% read coverage and >90% nucleotide identity relative to the reference sequence, with the exception of β-lactamase variants that were determined with 100% identity using the reference sequences downloaded from the Lahey (www.lahey.org) and National Center for Biotechnology Information (NCBI) β-lactamase data resources (https://www.ncbi.nlm.nih.gov/pathogens/beta-lactamase-data-resources). Known acquired-resistance genes and resistance-conferring mutations relevant to β-lactams (including carbapenems), fluoroquinolones, aminoglycosides, chloramphenicol, macrolides, sulphonamides, tetracyclines, trimethoprim, rifamycins and fosfomycin were included in the analysis. Chromosomal mutations focused on variations in the quinolone resistant determining regions (QRDR)s of gyrA, gyrB, parC and parE. Isolates that had AMR determinants known to confer resistance to three or more classes of antimicrobial were defined as MDR.
Illumina reads were mapped to the STEC O157:H7 reference genome Sakai (GenBank accession BA000007) using BWA-MEM v.0.7.13.22 SNPs were identified using GATK v.2.6 in unified genotyper mode.23 Core-genome positions that had a high-quality SNP (>90% consensus, minimum depth 10× MQ ≥30) in at least one isolate were extracted for further analysis. Genomes were compared to the sequences held in the UKHSA STEC O157:H7 WGS database, using SnapperDB v.0.2.5.24 The maximum-likelihood phylogenetic tree was constructed by RAxML v.8.1.1725 using an alignment generated from SnapperDB v.0.2.524 in which recombination had been masked by Gubbins v.2.00.26 Visualization/annotation of the phylogenetic tree was performed using FigTree v.1.4.4.
Nanopore-based whole-genome sequencing
Genomic DNA was extracted and purified using the Revolugen Fire Monkey DNA extraction kit (Revolugen, Glossop, UK). Library preparation was performed using the rapid barcoding kit (SQK-RBK004) (Oxford Nanopore Technologies, Oxford, UK). The prepared libraries were loaded onto FLO-MIN106 R9.4.1 flow cells (Oxford Nanopore Technologies, Oxford, UK) and sequenced using the MinION (Oxford Nanopore Technologies, Oxford, UK) for 48 h.
Data produced in a raw FAST5 format were base-called and de-multiplexed using the Guppy v.4.3.4 FAST model (Oxford Nanopore Technologies, Oxford, UK) into FASTQ format and grouped in each sample’s respective barcode. Demultiplexing was performed using Deepbinner v.0.2.0.27 Sequencing run metrics were generated using Nanoplot v.1.8.1.28 The barcode and y-adapter from each sample’s reads were trimmed, and chimeric reads split using Porechop v.0.2.4 (Wick RR, https://github.com/rrwick/Porechop). Finally, the trimmed reads were filtered using Filtlong v.0.2.0 (Wick RR, https://github.com/rrwick/Filtlong) with the following parameters, min length = 1000 bp, keep percentage = 90 and target bases = 275 Mbp, to generate approximately 50× coverage of the STEC genome (5.5 Mbp) to generate two FASTQ files one for the longest (−length_weight = 10) and one for the highest-quality (−mean-q-weight = 10) reads.
The filtered nanopore FASTQ file with the 50 × coverage of longest reads was assembled using Flye v.2.829 with the minimum overlap length (-m) set to 10 000 and the –meta component enabled. Assembly correction (or polishing) was performed in a three-step process. First, correction was initiated using Nanopolish v.0.11.330 using both the highest-quality nanopore FASTQ and the FAST5 files for each respective sample accounting for methylation using the—methylation-aware = dcm and—min-candidate-frequency = 0.1. The alignment of reads to draft assembly was generated using Minimap2 v.2.17.31 Secondly, the correction was continued with Pilon v.1.2232 using Illumina FASTQ reads as the query dataset with the use of BWA v.0.7.17 and Samtools v.1.7. Finally, Racon v.1.3.3, also using BWA v.0.7.1722 and Samtools v.1.7,21 was used again with the Illumina FASTQ reads. As the chromosome from each assembly was circularized and closed, they were re-orientated to start at the dnaA gene (GenBank accession no. NC_000913) from E. coli K-12, using the –fixstart parameter in Circlator v.1.5.533 and Prokka v.1.1334 with default parameters.
In silico plasmid replicon typing and detection of and characterization of AMR determinants
The plasmid replicon for each non-chromosomal contig within the final assembly of each sample was performed using PlasmidFinder v.2.135 with the Enterobacteriaceae, minimum identity = 90% and minimum coverage = 90% parameters set. The MGE harbouring AMR determinants where annotated using PGAP build 2022-12-13.36 Finally, alignments were generated using Clinker v.0.0.27.37
Data deposition
llumina FASTQ files are available from NCBI BioProject PRJNA315192. Nanopore FASTQ and finalized assembly files are also available from BioProject PRJNA315192. Short-read archive accession numbers for each isolate are listed in the Supplementary Table S1 (available as Supplementary data at JAC Online).
Results
Overall, 217/1473 (14.7%) isolates in this study had at least one AMR determinant in the Gene-Finder database (Table 1 and Supplementary Table S1). However, the proportion of isolates exhibiting full susceptibility varied by sub-lineage, with the highest proportion of AMR determinants detected in sub-lineages Ib (28/64, 43.7%), I/II (18/51, 35.3%) and IIc (122/440, 27.7%), and the lowest proportion detected in sub-lineages Ic (7/342, 2.0%), IIb (7/208, 3.3%), Ia (3/38, 7.9%) and IIa (31/323, 9.6%) (Table 1 and Figures 1–3). The most commonly detected AMR determinants across all seven sub-lineages were to the aminoglycosides, tetracyclines and sulphonamides, while AMR to fluroquinolones, macrolides and third-generation cephalosporins was rare (Table 1 and Supplementary Figure S1).
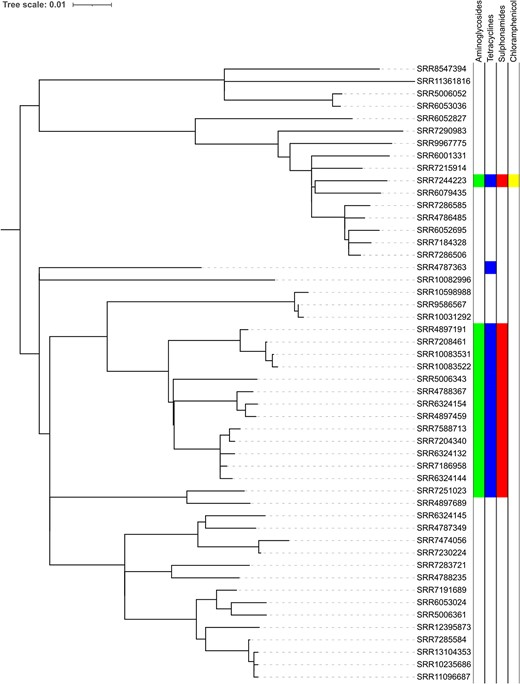
Phylogeny of STEC O157:H7 lineage I/II showing the distribution of AMR determinants.
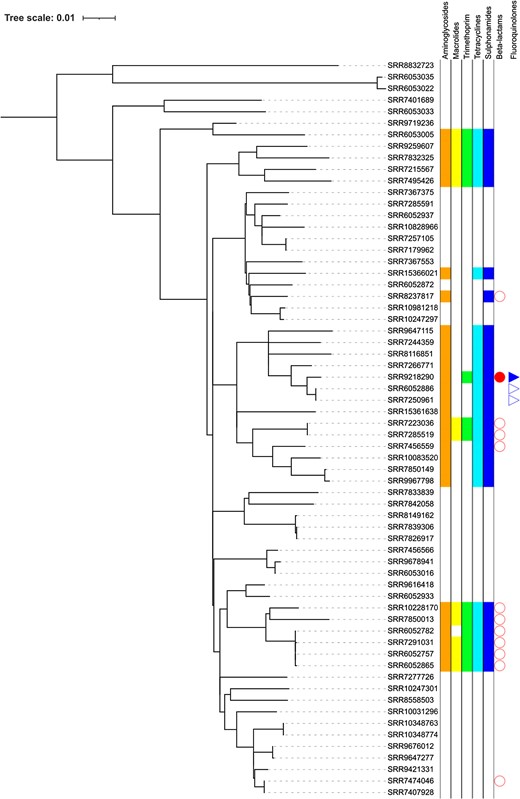
Phylogeny of STEC O157:H7 lineage Ib showing the distribution of AMR determinants.
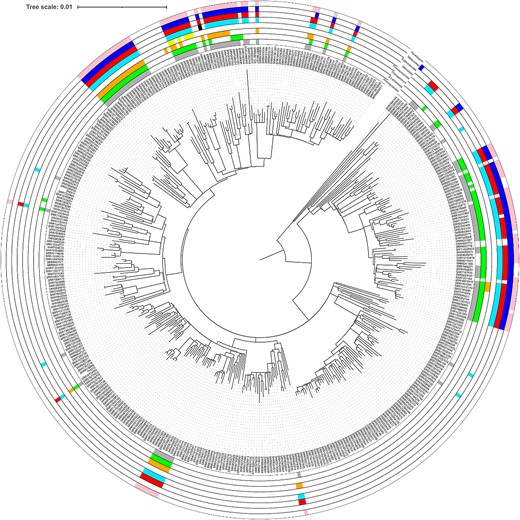
Phylogeny of STEC O157:H7 lineage IIc showing the distribution of AMR determinants.
Proportion of isolates within each lineage and sub-lineage predicted to confer resistance to a range of classes of antimicrobials, and the proportion of cases reporting recent travel (within 7 days of onset of symptoms) outside the UK
Lineage . | Ia . | . | Ib . | . | Ic . | . | IIa . | . | IIb . | . | IIc . | . | I/II . | . |
---|---|---|---|---|---|---|---|---|---|---|---|---|---|---|
. | n . | % . | n . | % . | n . | % . | n . | % . | n . | % . | n . | % . | n . | % . |
Total | 38 | 64 | 342 | 323 | 215 | 440 | 51 | 51 | ||||||
AMR | ||||||||||||||
Fully susceptible | 35 | 92.1 | 36 | 56.3 | 335 | 98 | 292 | 90.4 | 208 | 96.7 | 318 | 72.3 | 33 | 64.7 |
B-lactams | 0 | 0 | 12 | 18.8 | 5 | 1.5 | 13 | 4 | 4 | 1.9 | 95 | 21.5 | 0 | 0 |
Aminoglyosides | 3 | 7.9 | 27 | 42.2 | 0 | 0 | 17 | 5.3 | 2 | 0.9 | 108 | 24.5 | 15 | 29.4 |
Fluroquinolones | 1 | 2.6 | 1 | 1.6 | 0 | 0 | 7 | 2.2 | 0 | 0 | 32 | 7.3 | 2 | 2 |
Macrolides | 3 | 7.9 | 0 | 0 | 0 | 0 | 1 | 0.3 | 0 | 0 | 7 | 1.6 | 0 | 0 |
Trimethoprim | 1 | 2.6 | 14 | 21.9 | 0 | 0 | 6 | 1.9 | 0 | 0 | 96 | 21.8 | 0 | 0 |
Sulphonamides | 2 | 5.3 | 27 | 42.2 | 5 | 1.5 | 17 | 5.3 | 0 | 0 | 107 | 24.3 | 15 | 29.4 |
Tetracycline | 3 | 7.9 | 26 | 40.6 | 4 | 1.2 | 17 | 5.3 | 1 | 0.5 | 99 | 22.5 | 16 | 31.4 |
Choramphenicol | 0 | 0 | 0 | 0 | 0 | 0 | 9 | 2.8 | 0 | 0 | 48 | 10.9 | 1 | 1 |
Recent ravel outside UK | 11 | 28.9 | 18 | 28.1 | 15 | 4.4 | 86 | 26.6 | 9 | 4.2 | 93 | 21.1 | 8 | 15.7 |
Lineage . | Ia . | . | Ib . | . | Ic . | . | IIa . | . | IIb . | . | IIc . | . | I/II . | . |
---|---|---|---|---|---|---|---|---|---|---|---|---|---|---|
. | n . | % . | n . | % . | n . | % . | n . | % . | n . | % . | n . | % . | n . | % . |
Total | 38 | 64 | 342 | 323 | 215 | 440 | 51 | 51 | ||||||
AMR | ||||||||||||||
Fully susceptible | 35 | 92.1 | 36 | 56.3 | 335 | 98 | 292 | 90.4 | 208 | 96.7 | 318 | 72.3 | 33 | 64.7 |
B-lactams | 0 | 0 | 12 | 18.8 | 5 | 1.5 | 13 | 4 | 4 | 1.9 | 95 | 21.5 | 0 | 0 |
Aminoglyosides | 3 | 7.9 | 27 | 42.2 | 0 | 0 | 17 | 5.3 | 2 | 0.9 | 108 | 24.5 | 15 | 29.4 |
Fluroquinolones | 1 | 2.6 | 1 | 1.6 | 0 | 0 | 7 | 2.2 | 0 | 0 | 32 | 7.3 | 2 | 2 |
Macrolides | 3 | 7.9 | 0 | 0 | 0 | 0 | 1 | 0.3 | 0 | 0 | 7 | 1.6 | 0 | 0 |
Trimethoprim | 1 | 2.6 | 14 | 21.9 | 0 | 0 | 6 | 1.9 | 0 | 0 | 96 | 21.8 | 0 | 0 |
Sulphonamides | 2 | 5.3 | 27 | 42.2 | 5 | 1.5 | 17 | 5.3 | 0 | 0 | 107 | 24.3 | 15 | 29.4 |
Tetracycline | 3 | 7.9 | 26 | 40.6 | 4 | 1.2 | 17 | 5.3 | 1 | 0.5 | 99 | 22.5 | 16 | 31.4 |
Choramphenicol | 0 | 0 | 0 | 0 | 0 | 0 | 9 | 2.8 | 0 | 0 | 48 | 10.9 | 1 | 1 |
Recent ravel outside UK | 11 | 28.9 | 18 | 28.1 | 15 | 4.4 | 86 | 26.6 | 9 | 4.2 | 93 | 21.1 | 8 | 15.7 |
Travel history was captured from information on the laboratory submission form; if no travel history was reported, it cannot be inferred that the case did not travel.
Proportion of isolates within each lineage and sub-lineage predicted to confer resistance to a range of classes of antimicrobials, and the proportion of cases reporting recent travel (within 7 days of onset of symptoms) outside the UK
Lineage . | Ia . | . | Ib . | . | Ic . | . | IIa . | . | IIb . | . | IIc . | . | I/II . | . |
---|---|---|---|---|---|---|---|---|---|---|---|---|---|---|
. | n . | % . | n . | % . | n . | % . | n . | % . | n . | % . | n . | % . | n . | % . |
Total | 38 | 64 | 342 | 323 | 215 | 440 | 51 | 51 | ||||||
AMR | ||||||||||||||
Fully susceptible | 35 | 92.1 | 36 | 56.3 | 335 | 98 | 292 | 90.4 | 208 | 96.7 | 318 | 72.3 | 33 | 64.7 |
B-lactams | 0 | 0 | 12 | 18.8 | 5 | 1.5 | 13 | 4 | 4 | 1.9 | 95 | 21.5 | 0 | 0 |
Aminoglyosides | 3 | 7.9 | 27 | 42.2 | 0 | 0 | 17 | 5.3 | 2 | 0.9 | 108 | 24.5 | 15 | 29.4 |
Fluroquinolones | 1 | 2.6 | 1 | 1.6 | 0 | 0 | 7 | 2.2 | 0 | 0 | 32 | 7.3 | 2 | 2 |
Macrolides | 3 | 7.9 | 0 | 0 | 0 | 0 | 1 | 0.3 | 0 | 0 | 7 | 1.6 | 0 | 0 |
Trimethoprim | 1 | 2.6 | 14 | 21.9 | 0 | 0 | 6 | 1.9 | 0 | 0 | 96 | 21.8 | 0 | 0 |
Sulphonamides | 2 | 5.3 | 27 | 42.2 | 5 | 1.5 | 17 | 5.3 | 0 | 0 | 107 | 24.3 | 15 | 29.4 |
Tetracycline | 3 | 7.9 | 26 | 40.6 | 4 | 1.2 | 17 | 5.3 | 1 | 0.5 | 99 | 22.5 | 16 | 31.4 |
Choramphenicol | 0 | 0 | 0 | 0 | 0 | 0 | 9 | 2.8 | 0 | 0 | 48 | 10.9 | 1 | 1 |
Recent ravel outside UK | 11 | 28.9 | 18 | 28.1 | 15 | 4.4 | 86 | 26.6 | 9 | 4.2 | 93 | 21.1 | 8 | 15.7 |
Lineage . | Ia . | . | Ib . | . | Ic . | . | IIa . | . | IIb . | . | IIc . | . | I/II . | . |
---|---|---|---|---|---|---|---|---|---|---|---|---|---|---|
. | n . | % . | n . | % . | n . | % . | n . | % . | n . | % . | n . | % . | n . | % . |
Total | 38 | 64 | 342 | 323 | 215 | 440 | 51 | 51 | ||||||
AMR | ||||||||||||||
Fully susceptible | 35 | 92.1 | 36 | 56.3 | 335 | 98 | 292 | 90.4 | 208 | 96.7 | 318 | 72.3 | 33 | 64.7 |
B-lactams | 0 | 0 | 12 | 18.8 | 5 | 1.5 | 13 | 4 | 4 | 1.9 | 95 | 21.5 | 0 | 0 |
Aminoglyosides | 3 | 7.9 | 27 | 42.2 | 0 | 0 | 17 | 5.3 | 2 | 0.9 | 108 | 24.5 | 15 | 29.4 |
Fluroquinolones | 1 | 2.6 | 1 | 1.6 | 0 | 0 | 7 | 2.2 | 0 | 0 | 32 | 7.3 | 2 | 2 |
Macrolides | 3 | 7.9 | 0 | 0 | 0 | 0 | 1 | 0.3 | 0 | 0 | 7 | 1.6 | 0 | 0 |
Trimethoprim | 1 | 2.6 | 14 | 21.9 | 0 | 0 | 6 | 1.9 | 0 | 0 | 96 | 21.8 | 0 | 0 |
Sulphonamides | 2 | 5.3 | 27 | 42.2 | 5 | 1.5 | 17 | 5.3 | 0 | 0 | 107 | 24.3 | 15 | 29.4 |
Tetracycline | 3 | 7.9 | 26 | 40.6 | 4 | 1.2 | 17 | 5.3 | 1 | 0.5 | 99 | 22.5 | 16 | 31.4 |
Choramphenicol | 0 | 0 | 0 | 0 | 0 | 0 | 9 | 2.8 | 0 | 0 | 48 | 10.9 | 1 | 1 |
Recent ravel outside UK | 11 | 28.9 | 18 | 28.1 | 15 | 4.4 | 86 | 26.6 | 9 | 4.2 | 93 | 21.1 | 8 | 15.7 |
Travel history was captured from information on the laboratory submission form; if no travel history was reported, it cannot be inferred that the case did not travel.
Resistance to β-lactams
Of the 1473 isolates in this study, 129 (8.8%) had genes predicted to confer resistance to β-lactams. Including blaTEM-1 (n = 116), blaTEM-190 (n = 1), blaTEM-191 (n = 3), blaTEM-117 (n = 2) and the extended-spectrum β-lactamases (ESBLs) blaCTX-M-15 (n = 7) (Table 2). Carbapenemase genes were not detected in any of the isolates. The highest proportion of STEC O157:H7 isolates resistant to β-lactamases belonged to either sub-lineage Ib (12/64, 18.8%) or IIc (95/441, 21.5%) (Table 2 and Figures 2 and 3). The seven isolates harbouring blaCTX-M-15 were distributed across three sub-lineages, Ib (n = 1), IIa (n = 2) and IIc (n = 4); three cases reported travelling outside the UK within 7 days of onset of symptoms to the Middle East, Egypt and Spain (Supplementary Table S1).
Number of isolates within each lineage and sub-lineage harbouring AMR determinants included in the UKHSA Gene-Finder reference database, predicted to confer resistance to a range of classes of antimicrobials
AMR determinant . | Ia . | . | Ib . | . | Ic . | . | IIa . | . | IIb . | . | IIc . | . | I/II . | . |
---|---|---|---|---|---|---|---|---|---|---|---|---|---|---|
. | n . | % . | n . | % . | n . | % . | n . | % . | n . | % . | n . | % . | n . | % . |
B-lactams | ||||||||||||||
TEM-1 | 0 | 11 | 17.2 | 5 | 1.5 | 9 | 2.7 | 3 | 1.4 | 90 | 20.5 | 0 | ||
CTX-M-15 | 0 | 1 | 1.6 | 0 | 2 | 0.6 | 0 | 4 | 0.9 | 0 | ||||
TEM-117 | 0 | 0 | 0 | 1 | 0.3 | 1 | 0.5 | 0 | 0 | |||||
TEM-190 | 0 | 0 | 0 | 1 | 0.3 | 0 | 0 | 0 | ||||||
TEM-191 | 0 | 0 | 0 | 0 | 0 | 6 | 1.4 | 0 | ||||||
DHA-21 | 0 | 0 | 0 | 0 | 0 | 1 | 0.2 | 0 | ||||||
Aminoglycosides | ||||||||||||||
strA-strB | 3 | 7.9 | 27 | 42.2 | 6 | 1.8 | 17 | 5.3 | 1 | 0.5 | 105 | 23.9 | 15 | 29.4 |
aac(3)-IId | 0 | 1 | 1.6 | 0 | 0 | 0 | 7 | 1.6 | 0 | |||||
aadA-1b | 0 | 0 | 0 | 2 | 0.6 | 0 | 5 | 1.1 | 0 | |||||
aadA-2 | 0 | 0 | 0 | 2 | 0.6 | 0 | 12 | 2.7 | 0 | |||||
aadA-17 | 0 | 0 | 0 | 1 | 0.3 | 0 | 0 | 0 | ||||||
aac(6’)-Iy | 0 | 0 | 0 | 0 | 1 | 0.5 | 0 | 0 | ||||||
aac(3)-Iva | 0 | 0 | 0 | 0 | 1 | 0.5 | 23 | 5.2 | 0 | |||||
aadD | 0 | 0 | 0 | 0 | 1 | 0.5 | 23 | 5.2 | 0 | |||||
aph(4)-Ia | 0 | 0 | 0 | 0 | 1 | 0.5 | 22 | 5 | 0 | |||||
aadA-8b | 0 | 0 | 0 | 0 | 0 | 9 | 2 | 0 | ||||||
aadA-3 | 0 | 0 | 0 | 0 | 0 | 2 | 0.5 | 0 | ||||||
aac(3)-IIa | 0 | 0 | 0 | 0 | 0 | 2 | 0.5 | 0 | ||||||
aadA-1 | 0 | 0 | 0 | 0 | 0 | 2 | 0.5 | 0 | ||||||
Fluoroquinolones | ||||||||||||||
gyrA83:S-L | 1 | 2.6 | 0 | 0 | 3 | 0.9 | 0 | 26 | 5.9 | 2 | 3.9 | |||
gyrA83:S-A | 0 | 0 | 0 | 1 | 0.3 | 0 | 0 | 0 | ||||||
qnrS-1 | 0 | 1 | 1.6 | 0 | 4 | 1.2 | 0 | 6 | 1.4 | 0 | ||||
qnrB-19 | 0 | 3 | 4.7 | 0 | 2 | 0.6 | 0 | 0 | 0 | |||||
gyrA83:S-L;parC80:S-I | 0 | 0 | 0 | 0 | 0 | 4 | 0.9 | 0 | ||||||
Macrolides | ||||||||||||||
mphA | 0 | 0 | 0 | 1 | 0.3 | 0 | 2 | 0.5 | 0 | |||||
mphB | 0 | 12 | 18.8 | 0 | 0 | 0 | 34 | 7.7 | 0 | |||||
ermB | 0 | 0 | 0 | 0 | 0 | 3 | 0.7 | 0 | ||||||
erm42 | 0 | 0 | 0 | 0 | 0 | 2 | 0.5 | 0 | ||||||
Trimethoprim | ||||||||||||||
dfrA-8 | 1 | 2.6 | 0 | 0 | 0 | 0 | 40 | 9.1 | 0 | |||||
dfrA-1 | 0 | 14 | 21.9 | 0 | 3 | 0.9 | 0 | 49 | 11.2 | 0 | ||||
dfrA-5 | 0 | 0 | 0 | 1 | 0.3 | 0 | 0 | 0 | ||||||
dfrA-14 | 0 | 0 | 0 | 3 | 0.9 | 0 | 0 | 0 | ||||||
dfrA-12 | 0 | 0 | 0 | 0 | 0 | 10 | 2.3 | 0 | ||||||
Tetracycline | ||||||||||||||
tetA | 3 | 7.9 | 26 | 40.6 | 4 | 1.2 | 17 | 5.3 | 1 | 0.5 | 99 | 22.5 | 16 | 31.3 |
Sulphonamide | ||||||||||||||
sul1 | 0 | 13 | 20.3 | 0 | 0 | 0 | 48 | 10.9 | 0 | |||||
sul2 | 2 | 5.2 | 27 | 42.2 | 5 | 1.5 | 16 | 5 | 0 | 101 | 23 | 15 | 29.4 | |
sul3 | 0 | 0 | 0 | 2 | 6.2 | 0 | 8 | 1.8 | 0 | |||||
Chloramphenicol | ||||||||||||||
catA | 0 | 0 | 0 | 0 | 0 | 15 | 3.4 | 0 | ||||||
floR | 0 | 0 | 0 | 8 | 2.5 | 0 | 43 | 9.8 | 1 | 2 | ||||
cml-1 | 0 | 0 | 0 | 1 | 0.3 | 0 | 7 | 1.6 | 0 | |||||
Fully susceptible | 35 | 92.1 | 36 | 56.3 | 335 | 98 | 292 | 90.4 | 208 | 96.7 | 318 | 72.3 | 33 | 64.7 |
At least 1 AMR determinant | 3 | 7.9 | 28 | 43.7 | 7 | 2 | 31 | 9.6 | 7 | 3.3 | 122 | 27.7 | 18 | 35.3 |
Total | 38 | 64 | 342 | 323 | 215 | 440 | 51 |
AMR determinant . | Ia . | . | Ib . | . | Ic . | . | IIa . | . | IIb . | . | IIc . | . | I/II . | . |
---|---|---|---|---|---|---|---|---|---|---|---|---|---|---|
. | n . | % . | n . | % . | n . | % . | n . | % . | n . | % . | n . | % . | n . | % . |
B-lactams | ||||||||||||||
TEM-1 | 0 | 11 | 17.2 | 5 | 1.5 | 9 | 2.7 | 3 | 1.4 | 90 | 20.5 | 0 | ||
CTX-M-15 | 0 | 1 | 1.6 | 0 | 2 | 0.6 | 0 | 4 | 0.9 | 0 | ||||
TEM-117 | 0 | 0 | 0 | 1 | 0.3 | 1 | 0.5 | 0 | 0 | |||||
TEM-190 | 0 | 0 | 0 | 1 | 0.3 | 0 | 0 | 0 | ||||||
TEM-191 | 0 | 0 | 0 | 0 | 0 | 6 | 1.4 | 0 | ||||||
DHA-21 | 0 | 0 | 0 | 0 | 0 | 1 | 0.2 | 0 | ||||||
Aminoglycosides | ||||||||||||||
strA-strB | 3 | 7.9 | 27 | 42.2 | 6 | 1.8 | 17 | 5.3 | 1 | 0.5 | 105 | 23.9 | 15 | 29.4 |
aac(3)-IId | 0 | 1 | 1.6 | 0 | 0 | 0 | 7 | 1.6 | 0 | |||||
aadA-1b | 0 | 0 | 0 | 2 | 0.6 | 0 | 5 | 1.1 | 0 | |||||
aadA-2 | 0 | 0 | 0 | 2 | 0.6 | 0 | 12 | 2.7 | 0 | |||||
aadA-17 | 0 | 0 | 0 | 1 | 0.3 | 0 | 0 | 0 | ||||||
aac(6’)-Iy | 0 | 0 | 0 | 0 | 1 | 0.5 | 0 | 0 | ||||||
aac(3)-Iva | 0 | 0 | 0 | 0 | 1 | 0.5 | 23 | 5.2 | 0 | |||||
aadD | 0 | 0 | 0 | 0 | 1 | 0.5 | 23 | 5.2 | 0 | |||||
aph(4)-Ia | 0 | 0 | 0 | 0 | 1 | 0.5 | 22 | 5 | 0 | |||||
aadA-8b | 0 | 0 | 0 | 0 | 0 | 9 | 2 | 0 | ||||||
aadA-3 | 0 | 0 | 0 | 0 | 0 | 2 | 0.5 | 0 | ||||||
aac(3)-IIa | 0 | 0 | 0 | 0 | 0 | 2 | 0.5 | 0 | ||||||
aadA-1 | 0 | 0 | 0 | 0 | 0 | 2 | 0.5 | 0 | ||||||
Fluoroquinolones | ||||||||||||||
gyrA83:S-L | 1 | 2.6 | 0 | 0 | 3 | 0.9 | 0 | 26 | 5.9 | 2 | 3.9 | |||
gyrA83:S-A | 0 | 0 | 0 | 1 | 0.3 | 0 | 0 | 0 | ||||||
qnrS-1 | 0 | 1 | 1.6 | 0 | 4 | 1.2 | 0 | 6 | 1.4 | 0 | ||||
qnrB-19 | 0 | 3 | 4.7 | 0 | 2 | 0.6 | 0 | 0 | 0 | |||||
gyrA83:S-L;parC80:S-I | 0 | 0 | 0 | 0 | 0 | 4 | 0.9 | 0 | ||||||
Macrolides | ||||||||||||||
mphA | 0 | 0 | 0 | 1 | 0.3 | 0 | 2 | 0.5 | 0 | |||||
mphB | 0 | 12 | 18.8 | 0 | 0 | 0 | 34 | 7.7 | 0 | |||||
ermB | 0 | 0 | 0 | 0 | 0 | 3 | 0.7 | 0 | ||||||
erm42 | 0 | 0 | 0 | 0 | 0 | 2 | 0.5 | 0 | ||||||
Trimethoprim | ||||||||||||||
dfrA-8 | 1 | 2.6 | 0 | 0 | 0 | 0 | 40 | 9.1 | 0 | |||||
dfrA-1 | 0 | 14 | 21.9 | 0 | 3 | 0.9 | 0 | 49 | 11.2 | 0 | ||||
dfrA-5 | 0 | 0 | 0 | 1 | 0.3 | 0 | 0 | 0 | ||||||
dfrA-14 | 0 | 0 | 0 | 3 | 0.9 | 0 | 0 | 0 | ||||||
dfrA-12 | 0 | 0 | 0 | 0 | 0 | 10 | 2.3 | 0 | ||||||
Tetracycline | ||||||||||||||
tetA | 3 | 7.9 | 26 | 40.6 | 4 | 1.2 | 17 | 5.3 | 1 | 0.5 | 99 | 22.5 | 16 | 31.3 |
Sulphonamide | ||||||||||||||
sul1 | 0 | 13 | 20.3 | 0 | 0 | 0 | 48 | 10.9 | 0 | |||||
sul2 | 2 | 5.2 | 27 | 42.2 | 5 | 1.5 | 16 | 5 | 0 | 101 | 23 | 15 | 29.4 | |
sul3 | 0 | 0 | 0 | 2 | 6.2 | 0 | 8 | 1.8 | 0 | |||||
Chloramphenicol | ||||||||||||||
catA | 0 | 0 | 0 | 0 | 0 | 15 | 3.4 | 0 | ||||||
floR | 0 | 0 | 0 | 8 | 2.5 | 0 | 43 | 9.8 | 1 | 2 | ||||
cml-1 | 0 | 0 | 0 | 1 | 0.3 | 0 | 7 | 1.6 | 0 | |||||
Fully susceptible | 35 | 92.1 | 36 | 56.3 | 335 | 98 | 292 | 90.4 | 208 | 96.7 | 318 | 72.3 | 33 | 64.7 |
At least 1 AMR determinant | 3 | 7.9 | 28 | 43.7 | 7 | 2 | 31 | 9.6 | 7 | 3.3 | 122 | 27.7 | 18 | 35.3 |
Total | 38 | 64 | 342 | 323 | 215 | 440 | 51 |
Number of isolates within each lineage and sub-lineage harbouring AMR determinants included in the UKHSA Gene-Finder reference database, predicted to confer resistance to a range of classes of antimicrobials
AMR determinant . | Ia . | . | Ib . | . | Ic . | . | IIa . | . | IIb . | . | IIc . | . | I/II . | . |
---|---|---|---|---|---|---|---|---|---|---|---|---|---|---|
. | n . | % . | n . | % . | n . | % . | n . | % . | n . | % . | n . | % . | n . | % . |
B-lactams | ||||||||||||||
TEM-1 | 0 | 11 | 17.2 | 5 | 1.5 | 9 | 2.7 | 3 | 1.4 | 90 | 20.5 | 0 | ||
CTX-M-15 | 0 | 1 | 1.6 | 0 | 2 | 0.6 | 0 | 4 | 0.9 | 0 | ||||
TEM-117 | 0 | 0 | 0 | 1 | 0.3 | 1 | 0.5 | 0 | 0 | |||||
TEM-190 | 0 | 0 | 0 | 1 | 0.3 | 0 | 0 | 0 | ||||||
TEM-191 | 0 | 0 | 0 | 0 | 0 | 6 | 1.4 | 0 | ||||||
DHA-21 | 0 | 0 | 0 | 0 | 0 | 1 | 0.2 | 0 | ||||||
Aminoglycosides | ||||||||||||||
strA-strB | 3 | 7.9 | 27 | 42.2 | 6 | 1.8 | 17 | 5.3 | 1 | 0.5 | 105 | 23.9 | 15 | 29.4 |
aac(3)-IId | 0 | 1 | 1.6 | 0 | 0 | 0 | 7 | 1.6 | 0 | |||||
aadA-1b | 0 | 0 | 0 | 2 | 0.6 | 0 | 5 | 1.1 | 0 | |||||
aadA-2 | 0 | 0 | 0 | 2 | 0.6 | 0 | 12 | 2.7 | 0 | |||||
aadA-17 | 0 | 0 | 0 | 1 | 0.3 | 0 | 0 | 0 | ||||||
aac(6’)-Iy | 0 | 0 | 0 | 0 | 1 | 0.5 | 0 | 0 | ||||||
aac(3)-Iva | 0 | 0 | 0 | 0 | 1 | 0.5 | 23 | 5.2 | 0 | |||||
aadD | 0 | 0 | 0 | 0 | 1 | 0.5 | 23 | 5.2 | 0 | |||||
aph(4)-Ia | 0 | 0 | 0 | 0 | 1 | 0.5 | 22 | 5 | 0 | |||||
aadA-8b | 0 | 0 | 0 | 0 | 0 | 9 | 2 | 0 | ||||||
aadA-3 | 0 | 0 | 0 | 0 | 0 | 2 | 0.5 | 0 | ||||||
aac(3)-IIa | 0 | 0 | 0 | 0 | 0 | 2 | 0.5 | 0 | ||||||
aadA-1 | 0 | 0 | 0 | 0 | 0 | 2 | 0.5 | 0 | ||||||
Fluoroquinolones | ||||||||||||||
gyrA83:S-L | 1 | 2.6 | 0 | 0 | 3 | 0.9 | 0 | 26 | 5.9 | 2 | 3.9 | |||
gyrA83:S-A | 0 | 0 | 0 | 1 | 0.3 | 0 | 0 | 0 | ||||||
qnrS-1 | 0 | 1 | 1.6 | 0 | 4 | 1.2 | 0 | 6 | 1.4 | 0 | ||||
qnrB-19 | 0 | 3 | 4.7 | 0 | 2 | 0.6 | 0 | 0 | 0 | |||||
gyrA83:S-L;parC80:S-I | 0 | 0 | 0 | 0 | 0 | 4 | 0.9 | 0 | ||||||
Macrolides | ||||||||||||||
mphA | 0 | 0 | 0 | 1 | 0.3 | 0 | 2 | 0.5 | 0 | |||||
mphB | 0 | 12 | 18.8 | 0 | 0 | 0 | 34 | 7.7 | 0 | |||||
ermB | 0 | 0 | 0 | 0 | 0 | 3 | 0.7 | 0 | ||||||
erm42 | 0 | 0 | 0 | 0 | 0 | 2 | 0.5 | 0 | ||||||
Trimethoprim | ||||||||||||||
dfrA-8 | 1 | 2.6 | 0 | 0 | 0 | 0 | 40 | 9.1 | 0 | |||||
dfrA-1 | 0 | 14 | 21.9 | 0 | 3 | 0.9 | 0 | 49 | 11.2 | 0 | ||||
dfrA-5 | 0 | 0 | 0 | 1 | 0.3 | 0 | 0 | 0 | ||||||
dfrA-14 | 0 | 0 | 0 | 3 | 0.9 | 0 | 0 | 0 | ||||||
dfrA-12 | 0 | 0 | 0 | 0 | 0 | 10 | 2.3 | 0 | ||||||
Tetracycline | ||||||||||||||
tetA | 3 | 7.9 | 26 | 40.6 | 4 | 1.2 | 17 | 5.3 | 1 | 0.5 | 99 | 22.5 | 16 | 31.3 |
Sulphonamide | ||||||||||||||
sul1 | 0 | 13 | 20.3 | 0 | 0 | 0 | 48 | 10.9 | 0 | |||||
sul2 | 2 | 5.2 | 27 | 42.2 | 5 | 1.5 | 16 | 5 | 0 | 101 | 23 | 15 | 29.4 | |
sul3 | 0 | 0 | 0 | 2 | 6.2 | 0 | 8 | 1.8 | 0 | |||||
Chloramphenicol | ||||||||||||||
catA | 0 | 0 | 0 | 0 | 0 | 15 | 3.4 | 0 | ||||||
floR | 0 | 0 | 0 | 8 | 2.5 | 0 | 43 | 9.8 | 1 | 2 | ||||
cml-1 | 0 | 0 | 0 | 1 | 0.3 | 0 | 7 | 1.6 | 0 | |||||
Fully susceptible | 35 | 92.1 | 36 | 56.3 | 335 | 98 | 292 | 90.4 | 208 | 96.7 | 318 | 72.3 | 33 | 64.7 |
At least 1 AMR determinant | 3 | 7.9 | 28 | 43.7 | 7 | 2 | 31 | 9.6 | 7 | 3.3 | 122 | 27.7 | 18 | 35.3 |
Total | 38 | 64 | 342 | 323 | 215 | 440 | 51 |
AMR determinant . | Ia . | . | Ib . | . | Ic . | . | IIa . | . | IIb . | . | IIc . | . | I/II . | . |
---|---|---|---|---|---|---|---|---|---|---|---|---|---|---|
. | n . | % . | n . | % . | n . | % . | n . | % . | n . | % . | n . | % . | n . | % . |
B-lactams | ||||||||||||||
TEM-1 | 0 | 11 | 17.2 | 5 | 1.5 | 9 | 2.7 | 3 | 1.4 | 90 | 20.5 | 0 | ||
CTX-M-15 | 0 | 1 | 1.6 | 0 | 2 | 0.6 | 0 | 4 | 0.9 | 0 | ||||
TEM-117 | 0 | 0 | 0 | 1 | 0.3 | 1 | 0.5 | 0 | 0 | |||||
TEM-190 | 0 | 0 | 0 | 1 | 0.3 | 0 | 0 | 0 | ||||||
TEM-191 | 0 | 0 | 0 | 0 | 0 | 6 | 1.4 | 0 | ||||||
DHA-21 | 0 | 0 | 0 | 0 | 0 | 1 | 0.2 | 0 | ||||||
Aminoglycosides | ||||||||||||||
strA-strB | 3 | 7.9 | 27 | 42.2 | 6 | 1.8 | 17 | 5.3 | 1 | 0.5 | 105 | 23.9 | 15 | 29.4 |
aac(3)-IId | 0 | 1 | 1.6 | 0 | 0 | 0 | 7 | 1.6 | 0 | |||||
aadA-1b | 0 | 0 | 0 | 2 | 0.6 | 0 | 5 | 1.1 | 0 | |||||
aadA-2 | 0 | 0 | 0 | 2 | 0.6 | 0 | 12 | 2.7 | 0 | |||||
aadA-17 | 0 | 0 | 0 | 1 | 0.3 | 0 | 0 | 0 | ||||||
aac(6’)-Iy | 0 | 0 | 0 | 0 | 1 | 0.5 | 0 | 0 | ||||||
aac(3)-Iva | 0 | 0 | 0 | 0 | 1 | 0.5 | 23 | 5.2 | 0 | |||||
aadD | 0 | 0 | 0 | 0 | 1 | 0.5 | 23 | 5.2 | 0 | |||||
aph(4)-Ia | 0 | 0 | 0 | 0 | 1 | 0.5 | 22 | 5 | 0 | |||||
aadA-8b | 0 | 0 | 0 | 0 | 0 | 9 | 2 | 0 | ||||||
aadA-3 | 0 | 0 | 0 | 0 | 0 | 2 | 0.5 | 0 | ||||||
aac(3)-IIa | 0 | 0 | 0 | 0 | 0 | 2 | 0.5 | 0 | ||||||
aadA-1 | 0 | 0 | 0 | 0 | 0 | 2 | 0.5 | 0 | ||||||
Fluoroquinolones | ||||||||||||||
gyrA83:S-L | 1 | 2.6 | 0 | 0 | 3 | 0.9 | 0 | 26 | 5.9 | 2 | 3.9 | |||
gyrA83:S-A | 0 | 0 | 0 | 1 | 0.3 | 0 | 0 | 0 | ||||||
qnrS-1 | 0 | 1 | 1.6 | 0 | 4 | 1.2 | 0 | 6 | 1.4 | 0 | ||||
qnrB-19 | 0 | 3 | 4.7 | 0 | 2 | 0.6 | 0 | 0 | 0 | |||||
gyrA83:S-L;parC80:S-I | 0 | 0 | 0 | 0 | 0 | 4 | 0.9 | 0 | ||||||
Macrolides | ||||||||||||||
mphA | 0 | 0 | 0 | 1 | 0.3 | 0 | 2 | 0.5 | 0 | |||||
mphB | 0 | 12 | 18.8 | 0 | 0 | 0 | 34 | 7.7 | 0 | |||||
ermB | 0 | 0 | 0 | 0 | 0 | 3 | 0.7 | 0 | ||||||
erm42 | 0 | 0 | 0 | 0 | 0 | 2 | 0.5 | 0 | ||||||
Trimethoprim | ||||||||||||||
dfrA-8 | 1 | 2.6 | 0 | 0 | 0 | 0 | 40 | 9.1 | 0 | |||||
dfrA-1 | 0 | 14 | 21.9 | 0 | 3 | 0.9 | 0 | 49 | 11.2 | 0 | ||||
dfrA-5 | 0 | 0 | 0 | 1 | 0.3 | 0 | 0 | 0 | ||||||
dfrA-14 | 0 | 0 | 0 | 3 | 0.9 | 0 | 0 | 0 | ||||||
dfrA-12 | 0 | 0 | 0 | 0 | 0 | 10 | 2.3 | 0 | ||||||
Tetracycline | ||||||||||||||
tetA | 3 | 7.9 | 26 | 40.6 | 4 | 1.2 | 17 | 5.3 | 1 | 0.5 | 99 | 22.5 | 16 | 31.3 |
Sulphonamide | ||||||||||||||
sul1 | 0 | 13 | 20.3 | 0 | 0 | 0 | 48 | 10.9 | 0 | |||||
sul2 | 2 | 5.2 | 27 | 42.2 | 5 | 1.5 | 16 | 5 | 0 | 101 | 23 | 15 | 29.4 | |
sul3 | 0 | 0 | 0 | 2 | 6.2 | 0 | 8 | 1.8 | 0 | |||||
Chloramphenicol | ||||||||||||||
catA | 0 | 0 | 0 | 0 | 0 | 15 | 3.4 | 0 | ||||||
floR | 0 | 0 | 0 | 8 | 2.5 | 0 | 43 | 9.8 | 1 | 2 | ||||
cml-1 | 0 | 0 | 0 | 1 | 0.3 | 0 | 7 | 1.6 | 0 | |||||
Fully susceptible | 35 | 92.1 | 36 | 56.3 | 335 | 98 | 292 | 90.4 | 208 | 96.7 | 318 | 72.3 | 33 | 64.7 |
At least 1 AMR determinant | 3 | 7.9 | 28 | 43.7 | 7 | 2 | 31 | 9.6 | 7 | 3.3 | 122 | 27.7 | 18 | 35.3 |
Total | 38 | 64 | 342 | 323 | 215 | 440 | 51 |
Resistance to tetracyclines, sulphonamides and trimethoprim
There were 166/1473 (11.3%) isolates dispersed across all seven sub-lineages that had tetA, predicted to confer tetracycline resistance. The sub-lineages with the highest proportions of isolates exhibiting predicted resistance to tetracycline were sub-lineages Ib (26/64, 40.6%), I/II (16/50, 32.0%) and IIc (99/441, 22.5%) (Tables 1 and 2).
One hundred and seventy-three (11.7%) isolates had genes expected to confer sulphonamide resistance, found in all but one of the sub-lineages. Of these, one had sul-1, 111 had sul-2, 53 had both sul-1 and sul-2, and nine had sul3 either alone (n = 1), or in combination with sul2 (n = 6) or sul1 and sul2 (n = 2) (Table 2). As with tetracycline, the sub-lineages with the highest proportions of isolates exhibiting predicted resistance to the sulphonamides were sub-lineages Ib (14/64, 21.8), I/II (15/51, 29.4%) and IIc (59/440, 13.4%) (Table 1 and Figures 1–3). All isolates harbouring sul-3 belonged to sub-lineage IIc.
One hundred and seventeen (7.9%) isolates had dfrA genes (dfrA-1 = 66; dfrA-5 = 1; dfrA-8 = 41; dfrA-12 = 10, dfrA-14 = 1), conferring resistance to trimethoprim, either alone or in combination (Table 1). The highest proportions of isolates exhibiting predicted resistance to trimethoprim were sub-lineages Ib (14/64, 21.9%) and IIc (59/440, 13.4%), with all trimethoprim resistant isolates in Ib harbouring dfrA-1, whereas those in IIc had dfrA-1, dfrA-8 and dfrA-12 (Table 2).
Resistance to aminoglycosides
There were 172/1472 (11.7%) isolates that had genes expected to confer streptomycin resistance, of which 167/172 (70.9%) had strA, strB only or in combination with another gene known to confer resistance to one of the aminoglycosides, and four had aadA variants (aadA-1, n = 2; aadA-1b, aadA-2) in the absence of strA, strB, and one had aac6'-Iy only (Table 2). Most isolates harbouring aminoglycoside resistance genes in sub-lineages Ia, Ib, Ic, IIa and I/II had strA, strB alone (62/67, 92.5%), compared to sub-lineage IIc where 65/108 (60.2%) isolates had strA, strB alone. A wide variety of genes predicted to confer resistance to gentamicin, kanamycin, tobramycin, neomycin and apramycin, were detected in sub-lineage IIc, and these are listed in Table 2.
Resistance to quinolones, macrolides and the phenicols
Forty-three STEC O157 isolates (43/1473,2.9%), distributed across sub-lineages IIa (11/232), Ib (3/64) and IIc (32/441), contained mutations in gyrA/parC (predominantly gyrA83:S-L) and/or harboured a plasmid-mediated quinolone resistance genes. There were 25 isolates that had gyrA83:S-L only, four that had gyrA83:S-L; parC80:S-I or gyrA83:S-L plus qnrS1 and five had qnrS1 only (Table 2).
Resistance determinants predicted to confer to the macrolides were detected in eight isolates (8/1472). Five had ermB and all belonged to sub-lineage IIc and three had mphA (sub-lineages IIa n = 2 and IIc n = 1) (Table 2).
Fifty-one isolates had floR, catA or cml1 either alone or in combination (floR, cat-A or cml-1). All but one of these isolates belonged to either sub-lineage IIa or IIc (Table 2).
Phylogenetic analysis of AMR isolates and analysis of ONT data
The sub-lineages exhibiting the highest proportion of MDR isolates (lineage I/II and sub-lineages Ib and IIc) were analysed further (Figure 1). Most MDR isolates in lineage I/II were restricted to a 50 SNP single linkage cluster and had strA, strB/tetA/sul2 (Figure 1). Long-read sequencing revealed that these three AMR determinants were co-located on a 9kbp Tn10 intergron integrated into the chromosome at 2 080 998–2 089 926 within a prophage at clpA (Figure 4). Two isolates each had a single mutation in gyrA83:S-L, both were from cases reporting recent travel to Mexico (Supplementary Table S1).

Long-read sequencing analysis of the region encoding the AMR determinants in lineage I/II showed that strA, strB/tetA/sul2 were co-located on a 9kbp Tn10 intergron integrated into the chromosome at 2 080 998–2 089 926 within a prophage at clpA.
Most MDR isolates that fell within sub-lineage Ib had either strA-strB/tetA/sul2 or blaTEM-1/strA-strB/dfrA-1/tetA/sul1/sul2 (Figure 2). Acquisition of dfrA-1 correlated with acquisition of sul-1 and, in some isolates, blaTEM-1 (Figure 2). Again, all the AMR determinants were co-located on the chromosome on a 39 466 bp fragment of DNA inserted within the LEE at 5 376 390–5 415 856 (Figure 5).

Long-read sequencing analysis of the region encoding the AMR determinants in sub-lineage Ib showed that all the AMR determinants (either strA-strB/tetA/sul2, or blaTEM-1/strA-strB/dfrA-1/tetA/sul1/sul2) were co-located on the chromosome on a 39 466 bp fragment of DNA inserted within the Locus of Enterocyte Effacement at 5 376 390–5 415 856.
The AMR profiles in sub-lineage IIc were the most variable. Loss and acquisition of a wide range of different AMR determinants was observed across the phylogeny, although most of the MDR isolates belonged to one of four 50 SNP single linkage clusters and one 100 SNP single linkage cluster, each with its own characteristic AMR profile (Figure 3).
MDR isolates belonging to Cluster1 had the AMR profile blaTEM-1/strA, strB/aadA-8b/aadA-2/aac(3)-IId/dfrA-12/sul-2/floR/catA-1, predicted to confer resistance to aminoglycosides, ampicillin, trimethoprim, sulphonamides and chloramphenicol. All these AMR determinants were co-located on the chromosome on a 30 071 bp fragment of DNA that also encodes the mercury resistance cassette, inserted into the genome at position 1 555 382–1 585 453.
The AMR determinants harboured by the MDR isolates belonging to Cluster 2 were also co-located and conferred resistance to aminoglycosides, ampicillin, trimethoprim, sulphonamides and chloramphenicol. However, the specific AMR genes were different blaTEM-1 /strA, strB/aadA-1b aac(3)-Iva/aph(4)-Ia, aadD/dfrA-1/sul-1/sul-2/tetA/floR, and, in contrast to MDR isolates belonging to 2.8.351.%, they were located on a IncHI2 plasmid.
Long-read sequencing data from isolates belonging to the clade designated Cluster 3 identified two groups of AMR determinants, both located on the same IncHI2 plasmid. Group 1 comprised blaTEM-1/strA, strB/aadA-1/dfrA-1/sul-1/sul-2/tetA, and Group 2 had strA, strB/sul-2/floR and blaCTX-M-15/qnrS-1.
Most MDR isolates in the clade designated Cluster 4 had the AMR profile blaTEM-1/strA, strB/dfrA-8/sul-2/tetA. All AMR genes were co-located on a 26 887 bp fragment of DNA integrated into the Locus of Enterocyte Effacement on the chromosome. A small subset of isolates also had an IncHI2 plasmid encoding ermB/mph-B/strA, strB/aadA-1/aadA-2/aadA-8b/aac(3)-Iva/aph(4)-Ia, aadD/dfrA-1/dfrA-12/sul1/sul2/tetA.
Travel history and association with sub-lineages
As shown in previous studies17,19, the analysis of the isolates in this study indicated that the UK sub-lineages Ic and IIb were mostly associated with domestically acquired infection with only 15/342 (4.4%) and 9/215 (4.2%) of cases, respectively, reporting travel outside the UK before onset of symptoms (Table 1). By contrast, a higher proportion of cases infected with isolates each belonging to sub-lineages Ia (11/38, 28.9%), Ib (18/64, 28.1%), IIa (86/323, 26.6%) and IIc (93/440, 21.1%) reported travelling outside the UK within 7 days of onset of symptoms, and were designated travel associated cases (Table 1, Supplementary Table S1).
Discussion
Since the 1990s, surveillance of STEC O157:H7 in England has included monitoring AMR profiles to assess the risk of transmission of MDR pathogens from animals to humans via the food chain.7,38,39 In this study, we reviewed the AMR gene profiles of STEC O157:H7 by sub-lineage and found that AMR genes were unevenly distributed across the phylogeny. AMR determinants were identified in less frequently in sub-lineages Ia, Ic, IIa and IIb, whereas sub-lineages Ib, IIa and IIc, and lineage I/ll comprised a higher proportion of isolates that were predicted to be resistant to at least one class of antimicrobial based on the AMR gene content.
Over the last two decades, the proportion of isolates of STEC O157:H7 harbouring resistance to at least one class of antibiotic has decreased from 20% to 14.7%.7,38 Our previous analysis of the evolutionary history of STEC O157:H7 in the UK showed that during the 1980s and 1990s lineage I/ll was the dominant UK lineage.17,18 Lineage I/II comprises a higher proportion of isolates harbouring AMR determinants than sub-linages Ic or IIb. The decrease in the number of cases infected with STEC O157:H7 belonging to I/II, and the emergence of sub-lineage Ic, and more recently IIb, may explain the decreasing proportion of strains exhibiting AMR. Historically, and in this study, most lineage I/ll isolates that had AMR determinants were predicted to be resistant to streptomycin, tetracycline and the sulphonamides (STR/TET/SUL).38,39 STEC O157:H7 is zoonotic and endemic in the UK cattle and sheep population, and the prevalence of genes encoding resistance to STR/TET/SUL in lineage I/ll is consistent with these antimicrobials being used as therapeutic options in veterinary practice.7 Improvements in the animal husbandry practices in the UK and more regulated use of these antimicrobials since the 1990s resulting in the reduction of selective pressure, may explain why strains belonging to the more recently emerged sub-lineages Ic and IIb have remained, for the most part, fully susceptible to the classes of antibiotics included in this study.
In contrast to sub-lineages Ic and IIb, there is little or no evidence that sub-lineages Ia and Ib are endemic in the UK ruminant population, and infection is most likely to occur either following travel outside the UK or via the consumption of imported contaminated food. Sub-lineage Ib has a higher proportion of isolates encoding AMR genes than sub-lineage Ia, even though both sub-lineages appear to be widely distributed across all regions of the globe, and the reasons for this difference remain unclear.
We have previously presented evidence that, within sub-lineages IIa and IIc isolated from human cases in the UK, there are clades that are endemic in the UK cattle population and those that are imported into the UK, either by travellers returning to the UK from abroad or via non-domestically produced food items.19,40 Sub-lineage IIc has a higher proportion of isolates encoding AMR genes than sub-lineage IIa, even though both sub-lineages comprise a similar proportion of travel related cases. As before, the reasons for the different proportions of AMR isolates between the two sub-lineages are unclear. It is possible that strains from certain sub-lineages originate from regions where unregulated antibiotic use in animals and/or treatment of human infection is contributing to the persistence and transmission of AMR in the local STEC population. Alternatively, certain sub-lineages may be better adapted to acquiring and maintaining MGE encoding AMR.
As well as being unevenly distributed across the different sub-lineages of STEC O157:H7, AMR genes are unevenly distributed between different clades within the same sub-lineage.40 Most AMR determinants were co-located either on the chromosome and/or on large plasmids. Dallman et al.40 concluded that domestic clades of sub-lineage IIc were largely populated with susceptible isolates, whereas non-domestic clades contained a higher proportion of MDR isolates. Once stabilized in the population, these clades may persist in certain environments where antibiotic use is high. AMR determinants located on MGE may transfer from pathogen to commensal bacteria in the gut, increasing the pool of MDR bacteria that may cause life-threatening extraintestinal infections. Monitoring AMR in gastrointestinal pathogens may provide an early warning of emerging risks to public health regarding the clinical management and empirical treatment of infectious diseases.
Resistance mechanisms not detected in our previous study in 20167 were identified, although the numbers of isolates that had these resistance mechanisms were low. We identified mutations in gyrA and parC predicted to exhibit an increase in MIC to fluroquinolones, blaCTX-M-15 predicted to confer resistance to the third-generation cephalosporins, and mphA and ermB predicted to confer resistance to azithromycin. The genes conferring resistance to the third-generation cephalosporins and azithromycin were plasmid-encoded, and therefore had the potential to be transferred to other bacteria in the gut.9,41,42
It has been suggested that antimicrobial use in food-producing animals may be a risk factor in the transmission of MDR strains of E. coli from animals to humans, via the food chain. Analysis of data from outbreak investigations has provided evidence of this transmission route,43 however, it has been challenging to identify associations between consumption of contaminated food and infection with MDR STEC O157:H7 in sporadic cases. Moving forward, real-time WGS analysis of genome derived AMR determinants, including characterization of drug determinant regions, will enable us to monitor the persistence of MDR strains in the animal reservoir and assess the risk factors associated with transmission to humans. Wider application of long-read sequencing for public health surveillance will enable us to observe the loss and acquisition of MGE encoding AMR determinants across the STEC O157:H7 phylogeny and, potentially, transmission to other bacteria in the gut of both humans and animals, and in the environment.
In summary, the majority of STEC O157:H7 causing domestically acquired infection in the UK are susceptible to most classes of antimicrobials, whereas strains associated with travellers’ diarrhoea are more likely to be multidrug resistant and may exhibit resistance to ampicillin, trimethoprim and chloramphenicol. Historically, in the UK the unregulated use of antibiotics in veterinary practice may have been a selective pressure for the acquisition of resistance genes, however, in this dataset most MDR isolates causing human infection on the UK were acquired abroad. The implementation of long-read sequencing for routine surveillance will enable us to monitor the emergence and spread of both MDR enteric pathogens and the AMR genes, thus enabling us to track the transmission of AMR at the pathogen and mobile genetic element level.
Funding
David Greig and Claire Jenkins are affiliated to the National Institute for Health and Care Research Health Protection Research Unit (NIHR HPRU) in Gastrointestinal Infections at University of Liverpool in partnership with the United Kingdom Health Securities Agency (UKHSA), and the University of Warwick. The views expressed are those of the author(s) and not necessarily those of the NHS, the NIHR, the Department of Health and Social Care, or UKHSA.
Transparency declarations
Nothing to declare.
Supplementary data
Figure S1 and Table S1 are available as Supplementary data at JAC Online.
References
- antibiotics
- phenotype
- plasmids
- cephalosporins
- chromosomes
- drug resistance, microbial
- drug resistance, multiple
- food contamination
- genes
- genome
- phylogeny
- toxins
- travel
- genetics
- macrolides
- sulfonamides
- tetracyclines
- pathogenic organism
- aminoglycosides
- antimicrobials
- surveillance, medical
- zoonotic pathogens
- shiga-toxigenic escherichia coli
- whole genome sequencing