-
PDF
- Split View
-
Views
-
Cite
Cite
Romain Bricca, Sylvain Goutelle, Sandrine Roux, Marie-Claude Gagnieu, Agathe Becker, Anne Conrad, Florent Valour, Frederic Laurent, Claire Triffault-Fillit, Christian Chidiac, Tristan Ferry, Genetic polymorphisms of ABCB1 (P-glycoprotein) as a covariate influencing daptomycin pharmacokinetics: a population analysis in patients with bone and joint infection, Journal of Antimicrobial Chemotherapy, Volume 74, Issue 4, April 2019, Pages 1012–1020, https://doi.org/10.1093/jac/dky541
- Share Icon Share
Abstract
Daptomycin has been recognized as a therapeutic option for the treatment of bone and joint infection (BJI). Gene polymorphism of ABCB1, the gene encoding P-glycoprotein (P-gp), may influence daptomycin pharmacokinetics (PK).
We aimed to examine population PK of daptomycin and its determinants, including genetic factors, in patients with BJI.
We analysed data from patients who received daptomycin for BJI between 2012 and 2016 in our regional reference centre and who had measured daptomycin concentrations and P-gp genotyping. A population approach was used to analyse PK data. In covariate analysis, we examined the influence of three single nucleotide variations (SNVs) of ABCB1 (3435C > T, 2677G > T/A and 1236C > T) and that of the corresponding haplotype on daptomycin PK parameters. Simulations performed with the final model examined the influence of covariates on the probability to achieve pharmacodynamic (PD) targets.
Data from 81 patients were analysed. Daptomycin body CL (CLDAP) correlated with CLCR and was 23% greater in males than in females. Daptomycin central V (V1) was allometrically scaled to body weight and was 25% lower in patients with homozygous CGC ABCB1 haplotype than in patients with any other genotype. Simulations performed with the model showed that sex and P-gp haplotype may influence the PTA for high MIC values and that a dosage of 10 mg/kg/24 h would optimize efficacy.
Daptomycin dosages higher than currently recommended should be evaluated in patients with BJI. Gender and P-gp gene polymorphism should be further examined as determinants of dosage requirements.
Introduction
Daptomycin is a lipopeptide antibacterial agent that is active against Gram-positive bacteria. Currently daptomycin is approved for the treatment of complicated skin and soft tissue infections, and bacteraemia and endocarditis caused by Staphylococcus aureus. Although still off-label usage, daptomycin is recognized as a therapeutic option for staphylococcal bone and joint infection (BJI) by the IDSA, with a recommended dosage of 6 mg/kg.1
Experimental studies have demonstrated that the antimicrobial effect of daptomycin is concentration dependent, with both Cmax/MIC and AUC/MIC being correlated with the killing effect.2–4 In addition, it has been shown that serum creatine phosphokinase (CK) elevation, a marker of muscular toxicity of daptomycin, was linked with the trough concentration of daptomycin (Cmin), with a greater risk for Cmin ≥ 24.3 mg/L.5 These pharmacokinetic (PK)/pharmacodynamic (PD) relationships are arguments supporting therapeutic drug monitoring (TDM) of daptomycin, although this practice is not officially recommended.6
It has been suggested that higher doses of daptomycin (> 6 mg/kg and even > 9 mg/kg) may be more effective,7–10 but concerns have been raised about a potentially greater risk of adverse drug reactions.8,11,12
Previous population PK studies on daptomycin have shown that renal function, body weight and acute infection were the most significant covariates influencing daptomycin PK.13–15 It is uncertain how these results can be extrapolated to patients with BJI because these patients usually receive prolonged treatment. To our knowledge, our group has performed the only daptomycin population PK study in patients with BJI. This study showed substantial inter-individual variability in daptomycin PK, but also significant intra-individual variability of daptomycin body CL (CLDAP), the latter being uncorrelated with changes in renal function.16
P-glycoprotein (P-gp) is a transmembrane efflux transport protein encoded by the ATP-binding cassette B1 (ABCB1) gene, ABCB1 [also known as the MDR 1 gene (MDR1)]. P-gp is expressed in many cells and tissues (e.g. enterocytes, hepatocytes and renal tubular cells) and has been shown to influence the disposition of many drugs.17 Gene polymorphisms of ABCB1 have been associated with changes in drug PK and effects.18
It has been shown that P-gp influences the intracellular concentration and activity of daptomycin in macrophages and kidney cells.19 A recent study in 23 patients has shown an association between gene polymorphisms of ABCB1 and CLDAP.20
Our aims were to study the population PK of daptomycin and its determinants, including ABCB1 genotype, in patients with BJI, and to assess the potential influence of those determinants on daptomycin efficacy and toxicity.
Patients and methods
Patient population and data collection
This was a single-centre retrospective study in our regional reference centre for the management of complex BJI (CRIOAc Lyon; http://www.crioac-lyon.fr). We analysed data from all patients who received daptomycin for BJI between 2012 and 2016 as salvage therapy in usual care and practice and had measured daptomycin concentrations as well as determination of ABCB1 genotype.
Patients’ written informed consent was obtained before P-gp genotyping, in accordance with French regulation. Patients were informed orally and by letter that data were being collected in an officially declared national database. This study was subject to a declaration to the local Commission for Data Protection and Liberties (number 17-057) and is registered on ClinicalTrial.gov (number NCT03134521).
Daptomycin TDM has been performed in our centre since 2012 to ensure patient safety, as doses of daptomycin greater than those recommended for approved indications are often used for BJI and the muscular toxicity of daptomycin has been related to drug concentration. TDM can also permit avoidance of underexposure that may compromise the efficacy of this concentration-dependent agent. Daptomycin concentrations were measured using an HPLC assay with a photodiode array detector. The method was adapted from an earlier publication.21 The lower limit of quantification was 2 mg/L. The interday precision was less than 11% with a bias lower than 8%. TDM of daptomycin typically included three timepoints: pre-dose (Cmin); 30 min after the end of the 30 min infusion (Cmax); and a last sample obtained 5 to 6 h post-infusion. TDM could be performed on several occasions (on average every month) in each patient. Data collected on all occasions in all patients were analysed simultaneously.
The three most common single-nucleotide variations (SNVs) of ABCB1 (3435C > T rs1045642, p.I1145I; 2677G > T/A rs2032582, p.A893S/T; and 1236C > T rs1128503, p.G412G) were detected using a TaqmanTM real-time PCR assay. These variants are characterized by strong linkage disequilibrium and the haplotypes CGC/CGC and TTT/TTT have been described in most ethnic groups.18 In the present study, Hardy–Weinberg and linkage disequilibrium analyses were performed by using the Genepop and SNPStats web tools.22,23
Other variables were collected from the computerized patient files, including age, sex, weight, serum creatinine, estimated glomerular filtration rate (eGFR) estimated by the four-variable modification of diet in renal disease (MDRD) equation, CLCR estimated by the Cockcroft–Gault equation, serum CK, dosing history of daptomycin and concomitant use of rifampicin, which is a known inducer of P-gp that may be used in combination with daptomycin in patients with BJI.
Population PK analysis
A population approach was used to analyse PK data. Non-linear mixed-effects modelling was performed using the Stochastic Approximation Expectation Maximization (SAEM) algorithm implemented in the Monolix software (version 4.2.2, Lixoft, Antony, France). Various structural, residual error, covariance and covariate models were assessed using a stepwise approach.
In covariate modelling, the following variables were examined: sex, age, body weight, serum creatinine, CLCR estimated with the Cockcroft–Gault equation, eGFR estimated with the MDRD and with the Chronic Kidney Disease Epidemiology study (CKD-EPI) equations, and the co-administration of rifampicin. Regarding the genetic variables, we first considered each individual ABCB1 SNV as a binary variable for the status WT or double mutation. For example, for the 3435C > T SNV, we coded patients as carriers of the WT (CC, yes or no) and double-mutated (TT, yes or no) variant. We also examined the influence of the haplotype based on the three SNVs (3435C > T, 2677G > T/A and 1236C > T) and used the status CGC/CGC and TTT/TTT as binary variables.
The change in the objective function value ( − 2 log-likelihood) was used to assess the influence of covariates, assuming a χ2 distribution with appropriate degrees of freedom. Statistical significance was set at a P value of 0.05 for forward selection and 0.01 for backward deletion of a covariate in the model.
The best final model was based on the classical criteria including objective function, parameter values along with their standard errors, plots of observed versus predicted concentrations, plots of residuals and visual predictive checks (simulation-based diagnostic based on 1000 replicates of each patient dataset).24,25
PK/PD simulations
PK/PD simulations were performed with the final model to investigate the influence of daptomycin dose and that of categorical covariates included in the final model (i.e. sex and ABCB1 haplotype; see below) on the probability to achieve predefined target exposure. All simulations were performed with the Pmetrics R package.26 Final estimates of the mean and variance of PK parameters obtained with Monolix were imported into Pmetrics.
We simulated patients with variable CLCR. A truncated (minimum 31 mL/min; maximum 200 mL/min) normal distribution was used, with a mean of 108 mL/min and SD of 47 mL/min, in accordance with data from the study population. Because we simulated weight-based dosages, a fixed weight of 70 kg was used for all simulated patients. Three dosages were examined: 420 mg/24 h (6 mg/kg), 560 mg/24 h (8 mg/kg) and 700 mg/24 h (10 mg/kg). Results were analysed after the fifth dose to reflect the steady-state. We considered four groups of sex and ABCB1 haplotypes: females without CGC/CGC haplotype, males without CGC/CGC haplotype, females with CGC/CGC haplotype and males with CGC/CGC haplotype. We simulated 1000 daptomycin PK profiles for each dosage and patient group.
We then evaluated the probability of achieving predefined targets of efficacy for daptomycin into bone. First, we assumed that only the free fraction of daptomycin (i.e. unbound to plasma protein) can penetrate into bone tissue. This has been suggested by a PK study from Traunmuller et al.,27 who reported a mean fAUC24 ratio (tissue/plasma, over 24 h) of 1.17 into metatarsal bone. We then derived AUC24/MIC and Cmax/MIC targets for free plasma concentrations based on the PD study performed by Safdar et al.2 in mice. This group reported that an AUC24/MIC ratio ≥ 666 (total concentration) and a Cmax/MIC ratio ≥ 129 (also in total concentrations) were associated with bactericidal effect (1 log10 killing) against S. aureus. As the rate of protein binding reported by Traunmuller et al.27 in humans (91%) was similar to that reported in mice (90%),2 we can assume that the exposure targets identified in the mouse model are valid in humans. After correction for 91% protein binding, this led to the following free plasma concentration targets: fAUC24/MIC ≥ 66 and fCmax/MIC ≥ 12.
For each simulation, we evaluated the probability of achieving these efficacy targets for the following MIC values: 0.064, 0.125, 0.25, 0.5, 1, 2 and 4 mg/L. These are representative of MICs reported by EUCAST for S. aureus, for which the daptomycin epidemiological cut-off (ECOFF) is 1 mg/L (https://mic.eucast.org/Eucast2/). We considered a PTA ≥ 90% as optimal. Cumulative fraction of response was also calculated, by using the MIC distribution reported by EUCAST for S. aureus.
For each simulation, we also calculated the probability of achieving a safety target of Cmin ≥ 24.3 mg/L, which was the threshold associated with increased risk of CK elevation identified by Bhavnani et al.5
Results
Population data
Data from 81 patients collected between March 2012 and December 2016 were analysed. A total of 577 daptomycin concentrations were collected, with 2.5±7.9 TDM occasions per patient. Patient characteristics are shown in Table 1.
Characteristic . | Value . |
---|---|
Number of patients (female/male) | 81 (34/47) |
Age (years) | 60±18 |
Weight (kg) | 79±20 |
eGFR (mL/min/1.73 m2) | 100±41 |
Measured daptomycin concentrations (n) | 577 |
TDM occasions per patient (n) | 2.5±7.9 |
Daptomycin initial dose (mg/kg per 24 h) | 8.0±1.9 |
Characteristic . | Value . |
---|---|
Number of patients (female/male) | 81 (34/47) |
Age (years) | 60±18 |
Weight (kg) | 79±20 |
eGFR (mL/min/1.73 m2) | 100±41 |
Measured daptomycin concentrations (n) | 577 |
TDM occasions per patient (n) | 2.5±7.9 |
Daptomycin initial dose (mg/kg per 24 h) | 8.0±1.9 |
Data are given as mean±SD, unless otherwise stated.
Characteristic . | Value . |
---|---|
Number of patients (female/male) | 81 (34/47) |
Age (years) | 60±18 |
Weight (kg) | 79±20 |
eGFR (mL/min/1.73 m2) | 100±41 |
Measured daptomycin concentrations (n) | 577 |
TDM occasions per patient (n) | 2.5±7.9 |
Daptomycin initial dose (mg/kg per 24 h) | 8.0±1.9 |
Characteristic . | Value . |
---|---|
Number of patients (female/male) | 81 (34/47) |
Age (years) | 60±18 |
Weight (kg) | 79±20 |
eGFR (mL/min/1.73 m2) | 100±41 |
Measured daptomycin concentrations (n) | 577 |
TDM occasions per patient (n) | 2.5±7.9 |
Daptomycin initial dose (mg/kg per 24 h) | 8.0±1.9 |
Data are given as mean±SD, unless otherwise stated.
Results of Hardy–Weinberg and linkage disequilibrium analyses are shown in Table S1 (available as Supplementary data at JAC Online). Allele frequencies for the three loci were in accordance with Hardy–Weinberg equilibrium. Results also showed linkage disequilibrium, which confirmed haplotype association of the three SNVs. The distributions of ABCB1 genotypes are shown in Figure 1. The haplotype CGC/CGC was found in 18 patients (22%).
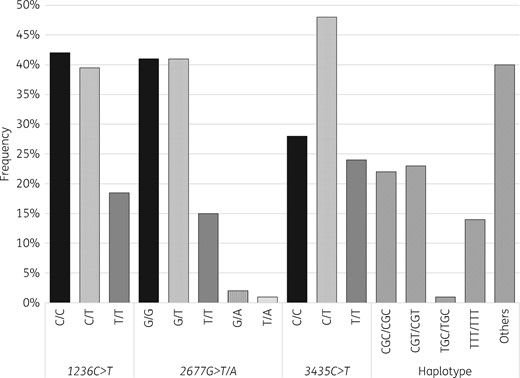
Regarding measured concentrations, mean Cmin (measured pre-dose concentration), Cmax (measured concentration 30 min after the end of the infusion), AUC and t½ on the first TDM occasion were 18.7±14.0 mg/L, 72.0±19.4 mg/L, 949±337 mg·h/L and 18.5±13.3 h, respectively.
We observed 45 Cmin values (22.5%) > 24.3 mg/L, from 30 patients. However, only three patients (10%) showed elevated CK. The elevation was mild (211, 359 and 429 U/L) and asymptomatic in all cases. Of note, three other patients had increased CK levels associated with Cmin < 24.3 mg/L. Overall, 7.4% of patients had elevated CK levels.
Population PK analysis
The final model was a two-compartment model, with four parameters: CLDAP, daptomycin central V (V1) and peripheral V (V2) and intercompartment clearance (Q). Inter-individual variability could not be well estimated for V2 and Q, so only a fixed effect was estimated for these parameters in the final model. The introduction of IOV on CLDAP and V1 much improved the fit, with a 125 point decrease in the objective function (P < 0.001).
The population PK parameter values and parameter–covariate relationships are shown in Table 2. Parameters were all estimated with acceptable standard errors. It is noteworthy that intra-individual variability in CLDAP was greater than inter-individual variability. Four covariates were included in the final model. CLCR and sex were found to influence CLDAP. Median CLDAP was 23% greater in male than in female subjects (0.72 versus 0.585 L/h). Central V was allometrically scaled to body weight and was also influenced by ABCB1 haplotype. Carriers of the CGC/CGC haplotype showed a 25% lower median V1 compared with subjects with any other haplotype (7.56 versus 10.1 L). This was the only significant relationship found between polymorphisms of P-gp and daptomycin PK parameters.
Parameter . | Estimate (RSE, %) . |
---|---|
Population CLDAP (θCL, in L/h) | 0.585 (4) |
Influence of CLCR on CLDAP (βCLCR, in L/h per unit of CLCR) | 0.46 (10)a |
Influence of sex on CLDAP (βSEX) | 0.208 (25)a |
Population V1 (θV1, in L) | 10.1 (8) |
Influence of body weight on V1 (βBW) | 0.543 (29)a |
Influence of ABCB1 haplotype CGC/CGC on V1 (βCGC) | − 0.287 (29)a |
Population V2 (θV2, in L) | 3.39 (19) |
Population Q (θQ, in L/h) | 1.97 (41) |
CLDAP inter-individual variability (ωCL) | 0.135 (20) |
V1 inter-individual variability (ωV1) | 0.231 (25) |
CLDAP intra-individual variability (γCL) | 0.215 (8) |
V1 intra-individual variability (γV1) | 0.0965 (39) |
Residual error term (b, proportional model) | 0.194 (2) |
Parameter . | Estimate (RSE, %) . |
---|---|
Population CLDAP (θCL, in L/h) | 0.585 (4) |
Influence of CLCR on CLDAP (βCLCR, in L/h per unit of CLCR) | 0.46 (10)a |
Influence of sex on CLDAP (βSEX) | 0.208 (25)a |
Population V1 (θV1, in L) | 10.1 (8) |
Influence of body weight on V1 (βBW) | 0.543 (29)a |
Influence of ABCB1 haplotype CGC/CGC on V1 (βCGC) | − 0.287 (29)a |
Population V2 (θV2, in L) | 3.39 (19) |
Population Q (θQ, in L/h) | 1.97 (41) |
CLDAP inter-individual variability (ωCL) | 0.135 (20) |
V1 inter-individual variability (ωV1) | 0.231 (25) |
CLDAP intra-individual variability (γCL) | 0.215 (8) |
V1 intra-individual variability (γV1) | 0.0965 (39) |
Residual error term (b, proportional model) | 0.194 (2) |
RSE, relative standard error of estimate.
Coefficient significantly different from zero (P < 0.001, Wald test).
The relationship between covariates and individual values of CLDAP and V1 were modelled as follows.
For CLDAP:
where CLCRi is the individual value of CLCR, 102.5 is the median value of CLCR in the population (in mL/min), sex is a binary variable (equal to 0 for female and 1 for male), ηCLi is the inter-individual random effect, with ηCLi ∼ (0, ωCL2) in the population, and κCLi is the intra-individual random effect, with κCLi ∼ (0, γCL2) in the population.
For V1:
where BWi is the individual body weight, 83 is the median value of body weight in the population (in kg), CGC is a binary variable relative to ABCB1 haplotype (equal to 1 for haplotype CGC/CGC and 0 for any other haplotype), ηV1i is the inter-individual random effect, with ηV1i ∼ (0, ωV12) in the population, and κV1i is the intra-individual random effect, with κV1i ∼ (0, γV12) in the population.
Note: the standard deviation of the random effects, ωCL and ωV1, are approximate values of the coefficients of variation of CLDAP and V.
Parameter . | Estimate (RSE, %) . |
---|---|
Population CLDAP (θCL, in L/h) | 0.585 (4) |
Influence of CLCR on CLDAP (βCLCR, in L/h per unit of CLCR) | 0.46 (10)a |
Influence of sex on CLDAP (βSEX) | 0.208 (25)a |
Population V1 (θV1, in L) | 10.1 (8) |
Influence of body weight on V1 (βBW) | 0.543 (29)a |
Influence of ABCB1 haplotype CGC/CGC on V1 (βCGC) | − 0.287 (29)a |
Population V2 (θV2, in L) | 3.39 (19) |
Population Q (θQ, in L/h) | 1.97 (41) |
CLDAP inter-individual variability (ωCL) | 0.135 (20) |
V1 inter-individual variability (ωV1) | 0.231 (25) |
CLDAP intra-individual variability (γCL) | 0.215 (8) |
V1 intra-individual variability (γV1) | 0.0965 (39) |
Residual error term (b, proportional model) | 0.194 (2) |
Parameter . | Estimate (RSE, %) . |
---|---|
Population CLDAP (θCL, in L/h) | 0.585 (4) |
Influence of CLCR on CLDAP (βCLCR, in L/h per unit of CLCR) | 0.46 (10)a |
Influence of sex on CLDAP (βSEX) | 0.208 (25)a |
Population V1 (θV1, in L) | 10.1 (8) |
Influence of body weight on V1 (βBW) | 0.543 (29)a |
Influence of ABCB1 haplotype CGC/CGC on V1 (βCGC) | − 0.287 (29)a |
Population V2 (θV2, in L) | 3.39 (19) |
Population Q (θQ, in L/h) | 1.97 (41) |
CLDAP inter-individual variability (ωCL) | 0.135 (20) |
V1 inter-individual variability (ωV1) | 0.231 (25) |
CLDAP intra-individual variability (γCL) | 0.215 (8) |
V1 intra-individual variability (γV1) | 0.0965 (39) |
Residual error term (b, proportional model) | 0.194 (2) |
RSE, relative standard error of estimate.
Coefficient significantly different from zero (P < 0.001, Wald test).
The relationship between covariates and individual values of CLDAP and V1 were modelled as follows.
For CLDAP:
where CLCRi is the individual value of CLCR, 102.5 is the median value of CLCR in the population (in mL/min), sex is a binary variable (equal to 0 for female and 1 for male), ηCLi is the inter-individual random effect, with ηCLi ∼ (0, ωCL2) in the population, and κCLi is the intra-individual random effect, with κCLi ∼ (0, γCL2) in the population.
For V1:
where BWi is the individual body weight, 83 is the median value of body weight in the population (in kg), CGC is a binary variable relative to ABCB1 haplotype (equal to 1 for haplotype CGC/CGC and 0 for any other haplotype), ηV1i is the inter-individual random effect, with ηV1i ∼ (0, ωV12) in the population, and κV1i is the intra-individual random effect, with κV1i ∼ (0, γV12) in the population.
Note: the standard deviation of the random effects, ωCL and ωV1, are approximate values of the coefficients of variation of CLDAP and V.
The final model adequately described the data, as shown in the predictions versus observations plot (Figure 2). The scatterplots of Normalized Prediction Distribution Error (NPDE) showed no tendency and the distribution of NPDE appeared to be Gaussian, as expected (Figure 3). Simulation-based visual predictive check showed overall good agreement between model predictions and observations (Figure 4).
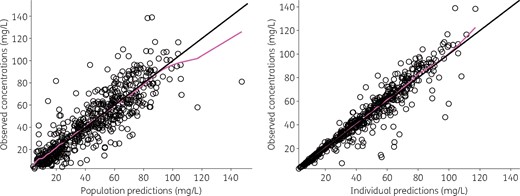
Observed daptomycin concentrations versus model predictions. The black line is the line of identity (y = x) and the magenta line is a spline regression. This figure appears in colour in the online version of JAC and in black and white in the print version of JAC.
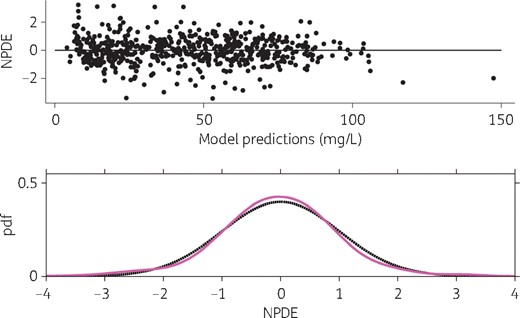
Scatterplot and distribution of NPDE. Upper panel, NPDE versus model predictions; lower panel, probability density function (pdf) of empirical (magenta line) and theoretical (black dashed line) NPDE. This figure appears in colour in the online version of JAC and in black and white in the print version of JAC.
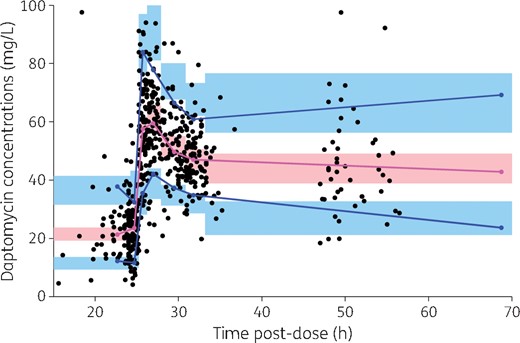
Prediction-corrected visual predictive check from the final model. The black dots are the observations. The blue solid lines are the 10th and 90th percentiles of prediction-corrected daptomycin concentrations and the magenta solid line is the median of observations (i.e. 50th percentile). The blue areas are the 90% CIs of the 10th and 90th simulated percentiles and the pink area is the 90% CI of the simulated median. Simulated percentiles were obtained from 1000 replicates of each individual dataset and the final model parameter estimates. Observations greater than 100 mg/L (n = 9) are not shown for ease of graphical display. Of note, the x-axis represents the time after the previous dose, i.e. the dose administered the day before TDM was performed. This figure appears in colour in the online version of JAC and in black and white in the print version of JAC.
PK simulation
Simulations were performed to calculate PK quantities (Cmax, Cmin and AUC) and derived probabilities to achieve defined PK/PD targets of efficacy (fAUC24/MIC ≥ 66 and fCmax/MIC ≥ 12) and safety (Cmin ≥ 24.3 mg/L). The results were calculated for three daptomycin dosages (6, 8 and 10 mg/kg/24 h) and the four groups of sex/ABCB1 haplotype influencing daptomycin PK. The results are summarized in Table 3. Figure 5 shows the PTA for the two efficacy targets as a function of daptomycin MIC. First, the results shown in Table 3 illustrate the influence of sex and ABCB1 haplotype on drug exposure. On average, daptomycin AUC, Cmax and Cmin were greater in females than in males, as a result of reduced CLDAP. Subjects with CGC/CGC haplotype had higher Cmax but comparable AUC compared with subjects with any mutated haplotype. The largest difference in drug exposure was observed between females with CGC/CGC haplotype and males without CGC/CGC haplotype, the former showing a 27% greater mean AUC and a 23% higher mean Cmax (for the 6 mg/kg dose). Those differences in daptomycin exposure showed implications in terms of target attainment. For a given dosage and MIC, females appeared to have a higher probability of achieving the fAUC24/MIC target, but also a higher probability of having Cmin associated with a risk of CK elevation. P-gp genetic polymorphism mainly influenced the probability of achieving the fCmax/MIC target, with a greater probability in subjects with CGC/CGC haplotype.
Simulated exposure and probability to achieve efficacy and toxicity targets stratified by dosage and categorical covariates
Daptomycin dosage and sex/ABCB1 haplotype . | Cmax (mg/L) . | Cmin (mg/L) . | AUC (mg·h/L) . | PTAafAUC/ MIC ≥ 66 . | CFRbfAUC/ MIC ≥ 66 . | PTAafCmax/ MIC ≥ 12 . | CFRbfCmax/ MIC ≥ 12 . | PTA Cmin ≥ 24.3 mg/L . |
---|---|---|---|---|---|---|---|---|
6 mg/kg | ||||||||
F/other | 55.1±8.5 | 16.9±5.9 | 735±159 | 0.626 | 0.993 | 0 | 0.807 | 0.11 |
M/other | 49.9±7.7 | 12.1±4.9 | 601±133 | 0.278 | 0.987 | 0 | 0.779 | 0.017 |
F/CGC | 63.0±9.5 | 14.9±5.9 | 740±164 | 0.63 | 0.994 | 0 | 0.900 | 0.079 |
M/CGC | 57.7±8.7 | 10.3±4.7 | 603±135 | 0.281 | 0.987 | 0 | 0.859 | 0.012 |
8 mg/kg | ||||||||
F/other | 73.4±11.4 | 22.5±7.9 | 980±212 | 0.967 | 0.999 | 0.002 | 0.947 | 0.365 |
M/other | 66.6±10.3 | 16.1±6.5 | 801±178 | 0.761 | 0.996 | 0.001 | 0.915 | 0.112 |
F/CGC | 83.9±12.6 | 19.9±7.9 | 987±219 | 0.968 | 0.999 | 0.016 | 0.977 | 0.256 |
M/CGC | 76.9±11.6 | 13.8±6.2 | 804±181 | 0.763 | 0.996 | 0.006 | 0.969 | 0.063 |
10 mg/kg | ||||||||
F/other | 91.8±14.2 | 28.1±9.9 | 1225±265 | 0.998 | 0.999 | 0.03 | 0.982 | 0.611 |
M/other | 83.2±12.9 | 20.1±8.1 | 1001±222 | 0.972 | 0.999 | 0.01 | 0.974 | 0.264 |
F/CGC | 104.9±15.8 | 24.9±9.9 | 1233±273 | 0.998 | 0.999 | 0.239 | 0.987 | 0.465 |
M/CGC | 96.2±14.5 | 17.2±7.8 | 1005±226 | 0.972 | 0.999 | 0.142 | 0.985 | 0.17 |
Daptomycin dosage and sex/ABCB1 haplotype . | Cmax (mg/L) . | Cmin (mg/L) . | AUC (mg·h/L) . | PTAafAUC/ MIC ≥ 66 . | CFRbfAUC/ MIC ≥ 66 . | PTAafCmax/ MIC ≥ 12 . | CFRbfCmax/ MIC ≥ 12 . | PTA Cmin ≥ 24.3 mg/L . |
---|---|---|---|---|---|---|---|---|
6 mg/kg | ||||||||
F/other | 55.1±8.5 | 16.9±5.9 | 735±159 | 0.626 | 0.993 | 0 | 0.807 | 0.11 |
M/other | 49.9±7.7 | 12.1±4.9 | 601±133 | 0.278 | 0.987 | 0 | 0.779 | 0.017 |
F/CGC | 63.0±9.5 | 14.9±5.9 | 740±164 | 0.63 | 0.994 | 0 | 0.900 | 0.079 |
M/CGC | 57.7±8.7 | 10.3±4.7 | 603±135 | 0.281 | 0.987 | 0 | 0.859 | 0.012 |
8 mg/kg | ||||||||
F/other | 73.4±11.4 | 22.5±7.9 | 980±212 | 0.967 | 0.999 | 0.002 | 0.947 | 0.365 |
M/other | 66.6±10.3 | 16.1±6.5 | 801±178 | 0.761 | 0.996 | 0.001 | 0.915 | 0.112 |
F/CGC | 83.9±12.6 | 19.9±7.9 | 987±219 | 0.968 | 0.999 | 0.016 | 0.977 | 0.256 |
M/CGC | 76.9±11.6 | 13.8±6.2 | 804±181 | 0.763 | 0.996 | 0.006 | 0.969 | 0.063 |
10 mg/kg | ||||||||
F/other | 91.8±14.2 | 28.1±9.9 | 1225±265 | 0.998 | 0.999 | 0.03 | 0.982 | 0.611 |
M/other | 83.2±12.9 | 20.1±8.1 | 1001±222 | 0.972 | 0.999 | 0.01 | 0.974 | 0.264 |
F/CGC | 104.9±15.8 | 24.9±9.9 | 1233±273 | 0.998 | 0.999 | 0.239 | 0.987 | 0.465 |
M/CGC | 96.2±14.5 | 17.2±7.8 | 1005±226 | 0.972 | 0.999 | 0.142 | 0.985 | 0.17 |
F, female; M, male.
Data are given as mean±SD unless otherwise stated. All PK quantities were calculated after five doses of daptomycin. ‘CGC’ means homozygous CGC/CGC haplotype and ‘other’ means any other genotype.
PTAs are given for a daptomycin MIC of 1 mg/L (ECOFF for S. aureus from EUCAST).
CFR, cumulative fraction of response based on the distribution of daptomycin MIC reported by EUCAST for S. aureus.
Simulated exposure and probability to achieve efficacy and toxicity targets stratified by dosage and categorical covariates
Daptomycin dosage and sex/ABCB1 haplotype . | Cmax (mg/L) . | Cmin (mg/L) . | AUC (mg·h/L) . | PTAafAUC/ MIC ≥ 66 . | CFRbfAUC/ MIC ≥ 66 . | PTAafCmax/ MIC ≥ 12 . | CFRbfCmax/ MIC ≥ 12 . | PTA Cmin ≥ 24.3 mg/L . |
---|---|---|---|---|---|---|---|---|
6 mg/kg | ||||||||
F/other | 55.1±8.5 | 16.9±5.9 | 735±159 | 0.626 | 0.993 | 0 | 0.807 | 0.11 |
M/other | 49.9±7.7 | 12.1±4.9 | 601±133 | 0.278 | 0.987 | 0 | 0.779 | 0.017 |
F/CGC | 63.0±9.5 | 14.9±5.9 | 740±164 | 0.63 | 0.994 | 0 | 0.900 | 0.079 |
M/CGC | 57.7±8.7 | 10.3±4.7 | 603±135 | 0.281 | 0.987 | 0 | 0.859 | 0.012 |
8 mg/kg | ||||||||
F/other | 73.4±11.4 | 22.5±7.9 | 980±212 | 0.967 | 0.999 | 0.002 | 0.947 | 0.365 |
M/other | 66.6±10.3 | 16.1±6.5 | 801±178 | 0.761 | 0.996 | 0.001 | 0.915 | 0.112 |
F/CGC | 83.9±12.6 | 19.9±7.9 | 987±219 | 0.968 | 0.999 | 0.016 | 0.977 | 0.256 |
M/CGC | 76.9±11.6 | 13.8±6.2 | 804±181 | 0.763 | 0.996 | 0.006 | 0.969 | 0.063 |
10 mg/kg | ||||||||
F/other | 91.8±14.2 | 28.1±9.9 | 1225±265 | 0.998 | 0.999 | 0.03 | 0.982 | 0.611 |
M/other | 83.2±12.9 | 20.1±8.1 | 1001±222 | 0.972 | 0.999 | 0.01 | 0.974 | 0.264 |
F/CGC | 104.9±15.8 | 24.9±9.9 | 1233±273 | 0.998 | 0.999 | 0.239 | 0.987 | 0.465 |
M/CGC | 96.2±14.5 | 17.2±7.8 | 1005±226 | 0.972 | 0.999 | 0.142 | 0.985 | 0.17 |
Daptomycin dosage and sex/ABCB1 haplotype . | Cmax (mg/L) . | Cmin (mg/L) . | AUC (mg·h/L) . | PTAafAUC/ MIC ≥ 66 . | CFRbfAUC/ MIC ≥ 66 . | PTAafCmax/ MIC ≥ 12 . | CFRbfCmax/ MIC ≥ 12 . | PTA Cmin ≥ 24.3 mg/L . |
---|---|---|---|---|---|---|---|---|
6 mg/kg | ||||||||
F/other | 55.1±8.5 | 16.9±5.9 | 735±159 | 0.626 | 0.993 | 0 | 0.807 | 0.11 |
M/other | 49.9±7.7 | 12.1±4.9 | 601±133 | 0.278 | 0.987 | 0 | 0.779 | 0.017 |
F/CGC | 63.0±9.5 | 14.9±5.9 | 740±164 | 0.63 | 0.994 | 0 | 0.900 | 0.079 |
M/CGC | 57.7±8.7 | 10.3±4.7 | 603±135 | 0.281 | 0.987 | 0 | 0.859 | 0.012 |
8 mg/kg | ||||||||
F/other | 73.4±11.4 | 22.5±7.9 | 980±212 | 0.967 | 0.999 | 0.002 | 0.947 | 0.365 |
M/other | 66.6±10.3 | 16.1±6.5 | 801±178 | 0.761 | 0.996 | 0.001 | 0.915 | 0.112 |
F/CGC | 83.9±12.6 | 19.9±7.9 | 987±219 | 0.968 | 0.999 | 0.016 | 0.977 | 0.256 |
M/CGC | 76.9±11.6 | 13.8±6.2 | 804±181 | 0.763 | 0.996 | 0.006 | 0.969 | 0.063 |
10 mg/kg | ||||||||
F/other | 91.8±14.2 | 28.1±9.9 | 1225±265 | 0.998 | 0.999 | 0.03 | 0.982 | 0.611 |
M/other | 83.2±12.9 | 20.1±8.1 | 1001±222 | 0.972 | 0.999 | 0.01 | 0.974 | 0.264 |
F/CGC | 104.9±15.8 | 24.9±9.9 | 1233±273 | 0.998 | 0.999 | 0.239 | 0.987 | 0.465 |
M/CGC | 96.2±14.5 | 17.2±7.8 | 1005±226 | 0.972 | 0.999 | 0.142 | 0.985 | 0.17 |
F, female; M, male.
Data are given as mean±SD unless otherwise stated. All PK quantities were calculated after five doses of daptomycin. ‘CGC’ means homozygous CGC/CGC haplotype and ‘other’ means any other genotype.
PTAs are given for a daptomycin MIC of 1 mg/L (ECOFF for S. aureus from EUCAST).
CFR, cumulative fraction of response based on the distribution of daptomycin MIC reported by EUCAST for S. aureus.
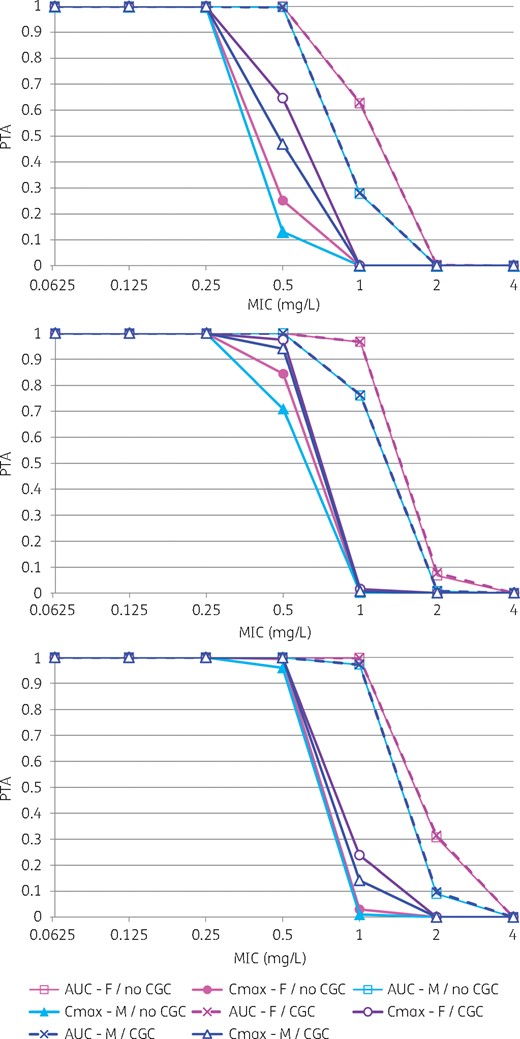
Probability of achieving target values of fAUC24/MIC and fCmax/MIC ratio for various daptomycin dosages and covariate status. Top, 6 mg/kg/24 h; middle, 8 mg/kg/24 h; bottom, 10 mg/kg/24 h. In the figure legend, ‘AUC’ means fAUC24/MIC target, while ‘Cmax’ means fCmax/MIC target. ‘CGC’ means homozygous CGC/CGC haplotype, while ‘no CGC’ means any other genotype. This figure appears in colour in the online version of JAC and in black and white in the printed version of JAC.
The results also provided insights on the influence of daptomycin dosage and bacterial MIC on PTA. For the 6 mg/kg/24 h dosage, PTA was ≥ 90% only when the MIC was ≤ 0.5 mg/L for the fAUC24/MIC target and when the MIC was ≤ 0.25 mg/L for the fCmax/MIC target. An increase in the dosage to 10 mg/kg/24 h was associated with greater PTA for MICs of 0.5–1 mg/L. PTA was still optimal for MIC values up to 1 mg/L for the fAUC24/MIC target and MIC values up to 0.5 mg/L for the fCmax/MIC target. However, such an increase in the dosage was associated with a much greater probability of exceeding the Cmin threshold for CK elevation. The overall probabilities were 5.5% and 37.8% for the 6 mg/kg and 10 mg/kg dosages, respectively.
Discussion
To our knowledge, the present work is the largest population PK study of daptomycin in patients with BJI. In addition, this is the largest study examining the influence of P-gp polymorphism on daptomycin PK. This study has provided several interesting pharmacological and clinical insights.
First, we have found that polymorphisms of ABCB1, the gene encoding P-gp, influenced daptomycin central V. Only the haplotype defined by the three most common ABCB1 SNVs (3435C > T, 2677G > T/A and 1236C > T) showed a significant influence. Previous studies with digoxin (a sensitive substrate of P-gp) have shown that ABCB1 haplotype analysis may be more relevant than single SNP analysis to study genotype–phenotype relationships.28 Patients having the CGC/CGC haplotype showed a 25% lower median V1 compared with subjects with any other haplotype. This suggests that P-gp could play a role in daptomycin tissue distribution. We assume that patients with CGC/CGC haplotype would have a more effective efflux of daptomycin from tissue and so a more restricted V. By contrast, Baietto et al.20 reported that the mutated genotype 3435T/T was associated with lower CLDAP compared with CC or CT genotypes (median of 0.38 L/h and 0.57 L/h, respectively) in 23 patients. This discrepancy may be due to the approach used to estimate PK parameters, as Baietto et al.20 used non-compartmental analysis to derive individual PK parameters. Covariance between parameters and between covariates was probably not taken into account, so P-gp genotype may not be an independent determinant of CLDAP in this study. Physiologically, a possible influence of P-gp activity on CLDAP is intriguing, considering that this agent is mainly eliminated by the kidneys. In addition, the renal CL of unbound daptomycin was found to be lower than the glomerular filtration rate in healthy subjects, which does not support major tubular secretion by transporters.29 Further research is required to clarify the role of P-gp in daptomycin distribution and elimination.
This observation also raises questions about the role of P-gp in daptomycin distribution and antibacterial effect in bone tissue. In previous reports, daptomycin alone did not show significant activity within osteoblasts.30,31 Although P-gp has not so far been described as being present on the osteoblast cell membrane, its presence on the membrane of osteosarcoma cells suggests that it may be found in bone cells.32 Efflux by P-gp could explain the lack of intra-osteoblast activity of daptomycin. Also, as reported by Lemaire et al.,19 inhibitors of P-gp could increase intracellular daptomycin concentration and its activity. It would be interesting to study intra-osteoblastic activity of daptomycin in combination with a P-gp inhibitor. In addition, the implication of ABCB1 genotype on daptomycin tissue concentration and response should be considered in further studies.
The other covariates identified were in accordance with previous studies.13,14 We confirmed the independent influence of sex on CLDAP, which was first reported in the large population PK study conducted by the manufacturer13 and also observed in our first PK study in patients with BJI.16 The magnitude of this sex difference was remarkably similar in our study and that of Dvorchik et al.,13 with about 20% lower CLDAP in females, on average. The rationale for this role of gender on daptomycin PK remains unclear. The confounding role of renal function can be ruled out, as CLCR was also included as a covariate in the CLDAP model. Preliminary runs showed an influence of body weight on CLDAP, but this covariate showed no additional influence once CLCR, which incorporates body weight, was included in the model. One may hypothesize that gender could be a better categorical descriptor of the influence of body size on CLDAP, but this requires further investigation.
PK/PD simulations performed with our final model showed that sex could influence the achievement of efficacy (fAUC/MIC ratio) and safety (Cmin) targets. For the efficacy target, this was especially true for MICs ranging from 0.5 to 2 mg/L, with men showing lower PTA. From the safety perspective, women presented a greater risk than men of achieving Cmin levels associated with CK elevation for a given dosage and P-gp genotype. P-gp haplotype mainly influenced the PTA for the fCmax/MIC target: patients without CGC/CGC haplotype showed lower PTA than patients with CGC/CGC haplotype. P-gp genotype marginally influenced Cmin and the corresponding safety target. Overall, women with CGC/CGC haplotype were identified as a subgroup with the highest daptomycin exposure and a potentially greater risk of toxicity, while men without CGC/CGC haplotype showed the lowest exposure and may be at risk of suboptimal efficacy for relatively high S. aureus MIC values (0.5–1 mg/L).
Simulations also showed that 6 mg/kg/24 h did not achieve sufficient exposure in bone for MIC values up 1 mg/L in patients with normal or moderately impaired renal function (CLCR > 30 mL/min). A dose of 10 mg/kg/24 h appears necessary to achieve the fAUC/MIC target for MIC values up to the S. aureus ECOFF.
While a dose of 10 mg/kg/24 h appears to be better than 6 or 8 mg/kg/24 h for efficacy, it is associated with a significant increase in the probability to attain potentially toxic Cmin. So, one has to balance the need for efficacy against the risk of muscular toxicity when using such a dosage of daptomycin. While the risk of muscular toxicity cannot be neglected, one should recall that the relationship between daptomycin Cmin and CK elevation reported in the reference study from Bhavnani et al.5 was probabilistic, with relatively large imprecision in probability estimates above the threshold of 24.3 mg/L. In their subsequent PK/PD analysis, Bhavnani et al.5 estimated that a dosage of 10 mg/kg/24 h would be associated with a 5.1% risk of muscular adverse events in patients with S. aureus bacteraemia.33 In the present study, only 10% of patients with Cmin > 24.3 mg/L had CK elevation and none showed clinical signs. This lower rate of CK elevation could be explained by the study population, as patients with BJI often have limited mobility, and by the cessation of co-medications with muscular adverse effects such as statins34 during daptomycin therapy, which was applied in the cohort.
An original feature of the present study was the quantification of intra-individual variability of CLDAP and V. Interestingly, intra-individual variability in CLDAP (21.5%) was greater than inter-individual variability unexplained by covariates (13.5%). It is likely that such intra-individual variability may be greater in other patient populations, such as critically ill patients who often present rapid changes in renal function and health status that influence daptomycin exposure.35,36 Repeated TDM may be especially useful in these patients to control drug exposure.
This study has several limitations that are inherent to its design. Data were collected during routine patient care and some errors might have occurred. Except for rifampicin, we did not record all co-medications that may influence P-gp activity. The presence of fever or sepsis, which have been found to influence daptomycin PK,13,14 was not recorded. Regarding P-gp genotyping, only the three most common ABCB1 SNVs were investigated, although others have been described. We did not investigate the influence of possible inducers or inhibitors of P-gp other than rifampicin. Finally, microbiological data (species and MIC values) were not collected and clinical outcome was not analysed, which precludes a thorough PK/PD evaluation based on observed data.
Conclusions
This is the largest population PK study of daptomycin in patients with BJI. In addition to renal function and body weight, this study identified sex and P-gp genotype as covariates influencing daptomycin PK. PK/PD simulations performed with the final model showed that these covariates may influence the risk/benefit profile of daptomycin for high MIC values. This work also suggests that a dosage of 10 mg/kg/24 h would optimize the antibacterial effect of daptomycin in patients with BJI. Prospective clinical studies are necessary to confirm the efficacy and safety of such dosages in this clinical setting.
Acknowledgements
This work was presented in part as an abstract at the European Congress of Clinical Microbiology and Infectious Diseases, Madrid, Spain, 2018 (Abstract #O0804) and at the Annual Meeting of the French Society of Pharmacology and Therapeutics Toulouse, France, 2018 (Abstract #CO-047).
This work was performed within the framework of the LABEX ECOFECT (ANR-11-LABX-0048) of Université de Lyon, within the programme ‘Investissements d’Avenir’ (ANR-11-IDEX-0007) operated by the French National Research Agency (ANR).
We gratefully acknowledge all the members of the Lyon Bone and Joint Infection Study Group.
Members of the Lyon Bone and Joint Infection Study Group
Coordinator: Tristan Ferry. Infectious Diseases Specialists: Tristan Ferry, Florent Valour, Thomas Perpoint, André Boibieux, François Biron, Patrick Miailhes, Florence Ader, Agathe Becker, Sandrine Roux, Claire Triffault-Fillit, Anne Conrad, Alexie Bosch, Fatiha Daoud, Johanna Lippman, Evelyne Braun and Christian Chidiac. Surgeons: Sébastien Lustig, Elvire Servien, Romain Gaillard, Antoine Schneider, Stanislas Gunst, Cécile Batailler, Michel-Henry Fessy, Yannick Herry, Anthony Viste, Philippe Chaudier, Cyril Courtin, Lucie Louboutin, Sébastien Martres, Franck Trouillet, Cédric Barrey, Emmanuel Jouanneau, Timothée Jacquesson, Ali Mojallal, Fabienne Braye, Fabien Boucher, Hristo Shipkov, Joseph Chateau, Philippe Céruse, Carine Fuchsmann and Arnaud Gleizal. Anaesthesiologists: Frédéric Aubrun, Mikhail Dziadzko and Caroline Macabéo. Microbiologists: Frederic Laurent, Jean-Philippe Rasigade, Laetitia Beraut, Céline Dupieux, Camille Kolenda and Jérôme Josse. Imaging: Fabien Craighero, Loic Boussel and Jean-Baptiste Pialat. Nuclear Medicine: Isabelle Morelec, Marc Janier and Francesco Giammarile. PK/PD specialists: Michel Tod, Marie-Claude Gagnieu and Sylvain Goutelle. Clinical research assistant and database manager: Eugénie Mabrut.
Funding
This study was carried out as part of our routine work, which is funded by Hospices Civils de Lyon.
Transparency declarations
None to declare.
References
Author notes
Members are listed in the Acknowledgements section.