-
PDF
- Split View
-
Views
-
Cite
Cite
Kristina Bayer, Lucas Moitinho-Silva, Franz Brümmer, Carlo V. Cannistraci, Timothy Ravasi, Ute Hentschel, GeoChip-based insights into the microbial functional gene repertoire of marine sponges (high microbial abundance, low microbial abundance) and seawater, FEMS Microbiology Ecology, Volume 90, Issue 3, December 2014, Pages 832–843, https://doi.org/10.1111/1574-6941.12441
- Share Icon Share
Abstract
The GeoChip 4.2 gene array was employed to interrogate the microbial functional gene repertoire of sponges and seawater collected from the Red Sea and the Mediterranean. Complementary amplicon sequencing confirmed the microbial community composition characteristic of high microbial abundance (HMA) and low microbial abundance (LMA) sponges. By use of GeoChip, altogether 20 273 probes encoding for 627 functional genes and representing 16 gene categories were identified. Minimum curvilinear embedding analyses revealed a clear separation between the samples. The HMA/LMA dichotomy was stronger than any possible geographic pattern, which is shown here for the first time on the level of functional genes. However, upon inspection of individual genes, very few specific differences were discernible. Differences were related to microbial ammonia oxidation, ammonification, and archaeal autotrophic carbon fixation (higher gene abundance in sponges over seawater) as well as denitrification and radiation-stress-related genes (lower gene abundance in sponges over seawater). Except for few documented specific differences the functional gene repertoire between the different sources appeared largely similar. This study expands previous reports in that functional gene convergence is not only reported between HMA and LMA sponges but also between sponges and seawater.
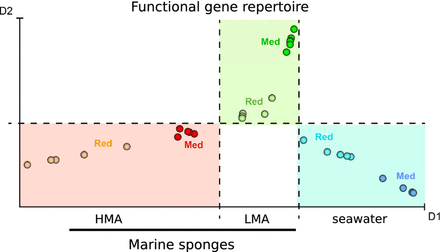
GeoChip analyses of sponge and seawater microbiomes revealed differences in nitrogen-, carbon metabolism, and stress response. The HMA/LMA dichotomy was confirmed on the level of functional genes.
Introduction
Marine sponges (phylum Porifera) are important couplers of the benthic and pelagic ecosystems by virtue of their immense filter-feeding capacities (Bell, 2008; de Goeij et al., 2013). Many marine sponges (hereafter referred to as high microbial abundance sponges, HMA) host stable associations of symbiotic microbial consortia within their mesohyl matrix that can contribute up to 35% of the animal's biomass (Hentschel et al., 2012). Representatives of more than 28 bacterial phyla including new candidate phyla such as Poribacteria and Tectomicrobia and two archaeal lineages were identified in marine sponges so far (Webster et al., 2010; Lee et al., 2011; Schmitt et al.,2012a, b; Simister et al., 2012; Wilson et al., 2014). On the other hand, some sponge species are essentially devoid of microorganisms (hereafter termed low microbial abundance sponges, LMA), and the diversity is largely restricted to Proteobacteria (Alpha-, Gamma-), Cyanobacteria, and Archaea. The classification into these HMA or LMA sponges has long been recognized (Vacelet & Donadey, 1977; Reiswig, 1981; Hentschel et al., 2003).
Our understanding of microbial functions in sponge symbiosis is still at the beginning due to the fact that the vast majority of sponge-associated microorganisms have not been cultured and due to the lack of an established model system for sponge-microbial symbioses. However, as reviewed (Taylor et al., 2007; Hentschel et al., 2012; Webster & Taylor, 2012), several publications have analyzed or inferred metabolic activities of the sponge symbionts, for example, as derived from pure-culture studies, analysis of pathways by measurement of specific products and genes, and inference of metabolic potentials based on 16S rRNA gene sequence analysis. A particular emphasis has been placed on the metabolism of carbon, nitrogen, and sulfur as well as secondary metabolism. Recently, the application of ‘omics’ approaches has contributed to build an integrated view of microbial function in the context of the host sponge. A recent metagenomic study reported functional gene convergence in the microbiome of six demosponge species (Fan et al., 2012). Several metatranscriptomic/metaproteomic studies have provided first insights into the expressed functional gene repertoire of sponge-associated microbial consortia (Liu et al., 2012; Radax et al., 2012; Moitinho-Silva et al., 2014b). Furthermore, single-cell genomic studies have enabled to correlate specific traits with specific symbiont lineages, thus providing a sought-after link between phylogeny and function (Siegl et al., 2011; Kamke et al., 2013; Wilson et al., 2014). However, much remains to be learned about the functional basis of sponge–microorganism interactions.
Functional gene arrays, such as the GeoChip, are one efficient way to assess the functional gene repertoire of environmental microbial communities (Nostrand et al., 2012). Microarrays have been developed to probe a wide diversity of genes in various ecological contexts. For example, functional gene arrays have been useful to establish gene inventories (Mason et al., 2010; Chan et al., 2013), to assess differences between field sites (Kang et al., 2013), and to report shifts in response to environmental parameters such as warming (Yergeau et al., 2012), or following the deep-water Horizon oil spill (Lu et al., 2012). The GeoChip has so far been applied to natural soils and sediments, in particular, in the context of contamination and bioremediation, and at least once before in a marine invertebrate, the tropical coral Montastraea faviolata (Kimes et al., 2010). Since its invention in 2004, the GeoChip has been constantly improved and updated. The newest version, GeoChip 4, contains 83 992 probes targeting 152 414 genes representing a total 410 gene categories (Tu et al., 2014). In comparison to previous versions, the categories stress response, antibiotic resistance, and bacteriophage genes have been added (Nostrand et al., 2012). In this study, we employed the GeoChip 4.2, to our knowledge for the first time, to assess the functional gene repertoire of marine sponge-associated microbiomes.
Materials and methods
Sample collection
Five individuals of the Mediterranean sponges Aplysina aerophoba (HMA) and Dysidea avara (LMA) were collected in April 2012 by scuba diving at a depth of 10–15 m off the coast at Rovinj, Croatia (45°08′N; 13°64′E). The sponges Xestospongia testudinaria (HMA) and Stylissa carteri (LMA) (n = 5 each) were collected by scuba diving at Fsar reef (22°23′N; 39°03′E) at a depth of 13–15 m off the coast of Thuwal, Saudi Arabia in March 2012. The animals were brought to the surface in plastic bags to avoid contact with air. Small tissue pieces were removed with a sterile scalpel, rinsed in 0.22 μm filtered seawater, immediately frozen in liquid nitrogen and stored at −80 °C until use. Sponge pieces were taken such that portions of pinacoderm and mesohyl were included in each sample. Seawater was sampled using folded plastic canisters (camping store), which were filled during the dives in the vicinity of the sponges. Approximately, 7 L of Mediterranean seawater was filtered onto 0.22-μm PES bottle top filters (Merck-Millipore, Schwalbach, Germany) using a manually operated vacuum pump. The same volume of Red Sea seawater was filtered onto 0.22-μm hydrophilic Durapore membrane filters (Millipore) using a Masterflex Easy-Load peristaltic pump (Cole-Parmer). The filters were stored at −80 °C until further processing.
DNA extraction
Frozen sponge samples were ground to fine powder using a sterile mortar and pistil. Genomic DNA was extracted using the Allprep DNA/RNA mini kit (Qiagen, Germany) following the manufacturer's instructions. DNA quality and concentrations were assessed with the NanoDrop 2000c spectrophotometer (Peqlab, Germany) as well as using the Qubit 2.0 fluorometer and the Qubit dsDNA BR Assay Kit (Life Technologies, Germany). DNA was freeze-dried using the ALPHA 1-2 LD lyophilizer (Christ, Germany) and shipped to Glomics Inc. (Norman, OK), where the DNA was processed.
Amplicon sequencing
The 16S rRNA genes were amplified according to Moitinho-Silva et al. (2014a) with minor modifications. Briefly, PCRs were performed in triplicate using multiplex identifier adaptor-ligated primers 533f (5′-GTGCCAGCAGCYGCGGTMA-3′) and 907r (5′-CCGTCAATTMMYTTGAGTTT-3′; Simister et al., 2012). The sequence region between the 533f and 907r primers includes the hypervariable regions V4 and V5. PCR amplification was performed using the Phusion Hot Start II High-Fidelity DNA Polymerase (Thermo Scientific, Germany) with buffer CG. The PCR protocol was as follows: initial denaturation at 98 °C for 3 min, followed by 30 cycles of denaturation (98 °C for 10 s), annealing (65 °C for 30 s), and extension (72 °C for 18 s). An additional elongation step (72 °C for 5 min) was performed at the end of the protocol. Amplified DNA was gel-purified, pooled at equimolar ratios, and sequenced on a Roche 454 GS FLX Titanium platform at the KAUST Genomics Core Lab. Raw pyrosequencing reads were deposited under the NCBI SRA accession numbers SRP043983.
Processing of 454 sequences and taxonomic assignment
The 16S rRNA gene amplicon sequences were processed with the qiime pipeline v 1.4 (Caporaso et al., 2010) according to Moitinho-Silva et al. (2014a). Briefly, denoised, quality-curated sequences from Mediterranean samples were pooled with publically available DNA sequences from Red Sea seawater, X. testudinaria and S. carteri (SRP017932, Moitinho-Silva et al., 2014a). An additional, newly sequenced amplicon data set of S. carteri DNA replicate 2 (ASD2, collected in Moitinho-Silva et al. (2014a) was included in this study. Sequences with > 97% sequence similarity were clustered into operational taxonomic units (OTUs), taxonomically classified and filtered, excluding singletons, chimeras, and eukaryotic sequences.
Ecological indices of amplicon data
Ecological indices and estimators were calculated based on datasets rarefied to minimum number of sequences recovered among all data sets. Richness was estimated by chao1 and ACE (Chao, 1984; Chao & Lee, 1992). Diversity was assessed using Simpson and Shannon indices (Shannon, 1948; Simpson, 1949). Ecological indices were calculated using vegan package v 2.0-4 (Oksanen et al., 2012) in r. UniFrac analysis (Lozupone & Knight, 2005) was performed for amplicon sequences using the qiime pipeline (Caporaso et al., 2010) and visualized as PCoA plot. Jackknife support was obtained based on 100 repetitions at 10 000 sequences per sample.
GeoChip 4 loading and data processing
For each sample, 800 ng of DNA was used directly for labeling followed by a 16.5 h hybridization step. Fluorescence-labeling, array hybridization, and scanning were conducted as described (Lu et al., 2012). Data normalization and preliminary analysis (including removal of poor-quality spots, normalization of spot signal intensity of each spot by mean, removal of spots with low signal intensities based on the signal-to-noise ratio as well as removal of outliers) were conducted as described before (Wu et al., 2006; He et al., 2007) and as provided by Glomics Inc. as customer service. Probes were assigned as positive when at least three of five (or three of four for Mediterranean seawater) biological replicates showed a positive signal. The normalized signal intensities were calculated as the sum of all probes per gene, divided by the signal intensity of all probes per category, and then averaged across all replicates per sample. Statistical analysis was performed with the nonparametric Mann–Whitney U-test using GraphPad Prism version 6.01 for Windows (GraphPad Software). GeoChip data were analyzed in the r environment v 2.15.3 (The R Core Team, 2012).
Minimum curvilinear embedding

These distances were used to construct the minimum spanning tree for each data set. The nonlinear distances stored in the Minimum curvilinear matrices were finally calculated as the traversal distances over the minimum spanning tree between the data points in the multidimensional space. The calculated Minimum curvilinear distance matrices were adopted for MCE dimension reduction. MCE analysis was performed using the singular value-decomposition-based algorithm published in Cannistraci et al. (2013). MCE analysis was performed both in matlab and in r, offering the same results. Principal component analysis (PCA) was performed in matlab.
Results and discussion
Microbial diversity
A total of 234 810 denoised, quality-controlled 16S rRNA gene sequences were generated from three biological replicates each of Mediterranean seawater, D. avara, A. aerophoba, and one biological replicate of S. carteri that had originally been collected by Moitinho-Silva et al. (2014a). These sequences were combined with already published 16S rRNA gene sequences from the Red Sea [seawater (n = 3), S. carteri (n = 2), X. testudinaria (n = 3)] (Moitinho-Silva et al., 2014a).This effort resulted in 1726 OTUs representing a total of 576 444 sequences of which 209 012 sequences were produced in this study.
Rarefaction curves revealed that samples were sequenced with different sampling depth (Supporting Information, Fig. S1a). Therefore, the data sets were rarefied to the minimum sampling effort (8925 sequences per sample). Seawater displayed the highest richness at both locations. The term ‘richness’ is based on the number of species in a given community and is judged from the estimators ACE and chao1 (Fig. S1b). However, the HMA sponges showed the highest diversity. The term ‘diversity’ takes the relative species abundances into account and is judged from Shannon and Simpson indices. The LMA sponges showed the lowest diversity at each location (Fig. S1c).
The distance between the microbiomes under investigation were analyzed by UniFrac and ordered by Principal coordinates analysis (PCoA) plot (Fig. 1a). PCoA of amplicon sequences revealed a clear separation of the three sample types (Fig. 1a). According to PC1 (which explained 43.65% of the variation), samples were ordered according to source (HMA, LMA, seawater) rather than to geographic location (Mediterranean, Red Sea). The LMA sponge samples (D. avara and S. carteri) presented a larger variation between sources and were placed between seawater and the HMA samples. PC2 (which explained 36.74% of the variation) separated D. avara from the other sources.
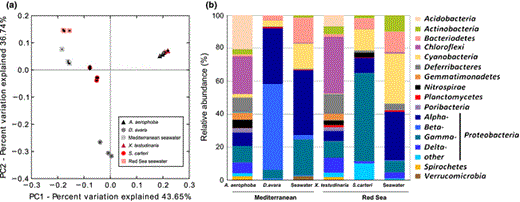
Microbial composition of HMA and LMA sponges as well as of seawater from the Mediterranean and the Red Sea collection site as obtained by 454 pyrosequencing. Relation between samples as performed by principle coordinates analysis (PCoA) based on UniFrac (a), taxon composition of samples (b). Phyla and major classes of Proteobacteria are shown for each source. Taxa which are present at > 1% abundance are shown. See Table S1 for details of taxon composition.
A total of 28 bacterial, archaeal, and candidate phyla were detected in the present study (Fig. 1b, Table S1). The microbial diversity of the Red Sea sponges was published in Moitinho-Silva et al. (2014a), but is included here for comparative purposes. The phylum-level composition of the HMA sponge species A. aerophoba and X. testudinaria was complex and was composed of Proteobacteria (26.6% and 28.0%), Chloroflexi (22.9% and 34.5%), Acidobacteria (20.8% and 6.8%), Deferribacteres (8.7% and 11.7%), Nitrospirae (5.1% and 2.9%), Gemmatimonadetes (4.2% and 3.8%), Actinobacteria (2.9% and 4.6%), Cyanobacteria (2.1% and 0.27%), Spirochaetes (2.2% and 1.6%), the candidate phylum Poribacteria (2.7% and 2.2%), Bacteroidetes (1.0% and 1.7%), and Planctomycetes (0.02% and 1.4%). Each of the remaining 16 phyla contributed < 1% to the HMA sponge data set. The LMA sponge species D. avara and S. carteri were dominated by Proteobacteria (91.9% and 74.1%), Cyanobacteria (3.8% and 12.8%), and Bacteriodetes (2.9% and 6.6%) (Fig. 1b, Table S1). It is noteworthy that the proteobacterial community of D. avara was dominated by Beta- and Alphaproteobacteria, while Gamma- and Alphaproteobacteria were dominant in S. carteri (Table S1). Each of the remaining 25 phyla contributed < 1% to the LMA sponge data set. The seawater samples were dominated by Proteobacteria (64.3 in Mediterranean and 41.3% in Red Sea seawater), followed by Cyanobacteria (15.3% and 30.4%), Bacteriodetes (15.5% and 12.7%), Actinobacteria (1.3% and 9.8%), and Verrucomicrobia (2.3% and 0.08%). Each of the remaining 23 phyla contributed < 1% to the seawater data set.
The microbial diversity of the investigated sponge species and seawater is fully consistent with previous reports (Schmitt et al.,2012a, b; Moitinho-Silva et al., 2014a). The HMA sponges show a more complex phylum-level composition with Proteobacteria and Chloroflexi as most abundant phyla, followed by Acidobacteria, Deferribacteres, Nitrospirae, Gemmatimonadetes, Actinobacteria, and candidate phylum Poribacteria. The phylum-level composition of the LMA sponge species, S. carteri, was dominated by Proteobacteria, Cyanobacteria, and Archaea, as has been reported previously (Giles et al., 2013; Moitinho-Silva et al., 2014a). Several additional studies revealed a distinct and different microbial composition in HMA sponges vs. LMA sponges (Weisz et al., 2007; Kamke et al., 2010; Erwin et al., 2011; Schmitt et al., 2012b; Giles et al., 2013; Gloeckner et al., 2013; Poppell et al., 2013; Moitinho-Silva et al., 2014a). However, even though the seawater samples resemble the composition of LMA sponges on the phylum level, the LMA sponges still contain their own characteristic symbiont guilds (Moitinho-Silva et al., 2014a), as evidenced here by a dominance of Betaproteobacteria in D. avara and Gammaproteobacteria in S. carteri.
GeoChip analysis of microbial functional genes
Using the functional GeoChip 4.2, a total of 20 273 probes were identified that represented 627 functional genes, grouped altogether in 16 functional gene categories in sponge and seawater samples (Fig. S1). The majority of positive gene probes belonged to the categories organic remediation (4577 probes), stress (4178 probes), carbon cycling (2327 probes), metal resistance (2297 probes), nitrogen (1580 probes), as well as in lesser amounts (< 850 probes) to the categories antibiotic resistance, bacteria phage, bioleaching, energy process, fungi function, others, phosphorus, soil benefit, soil borne pathogen, sulfur, and virulence (Table S2).
The exploration of the samples by MCE revealed a clear separation (Fig. 2a). The first dimension (horizontal axis) was discriminative between the three sources (HMA, LMA, seawater) and within that, between the locations (Red Sea, Mediterranean) for the sponge samples. The second dimension separated the LMA sponges from HMA sponges and seawater samples. The fact that the HMA–LMA pattern was stronger than any geographic bias is remarkable considering that the Mediterranean and Red Sea are distinct locations. To our knowledge, this is the first time that the HMA–LMA pattern was identified on the level of microbial functional genes.

Exploration and visualization of the GeoChip 4.2 by MCE. The reciprocal relations between the samples are mapped in a two-dimensional space by nonlinear dimension reduction performed by the MCE algorithm. Each spot represents a sample (a). The label ‘Red’ indicates samples collected in the Red Sea; the label ‘Med’ indicates samples collected in the Mediterranean Sea. Heatmap of functional genes’ normalized signal intensities. The x-axis plots the index of genes which are ordered according to first dimension, D1, of MCE analysis (b).
On the other hand, the exploration of the genes by MCE (Fig. 2b) unveiled very few discernible patterns. Noticeably, the LMA sponges, in particular D. avara, lacked genes as indicated by dark blue boxes. The absence of genes in D. avara resulted in higher than average signal intensities after normalization, as indicated by the yellow color. In conclusion, few specific gene patterns rather than a general overarching trend were revealed by MCE.
When the GeoChip data were analyzed for the presence/absence of genes, the vast majority of genes (> 88% for the Mediterranean site, > 91% for the Red Sea site) were shared between all three sources (Fig. 3). The sets of genes unique to a given source did not reveal any specific genes or pathways, thus the relevance of the unique genes remains unknown (data not shown). It is important to consider that equal DNA concentrations for each sample were applied to the GeoChip. For example, a much larger volume of seawater was necessary to extract the same amount of DNA. Similarly, much more LMA sponge biomass was necessary to yield the same amount of DNA as from HMA sponges. The results show that the microbial functional gene repertoires of HMA and LMA sponges, as well as of seawater, are largely similar. Any differences appear to be due to microbial and/or gene abundances rather than to true differences in the functional gene repertoire. These findings are consistent with previous publications on the microbial communities of tropical corals and also of uranium-contaminated aquifers which also reported on a consistent functional gene repertoire in spite of a variable microbial diversity (Van Nostrand et al., 2009; Kimes et al., 2010).
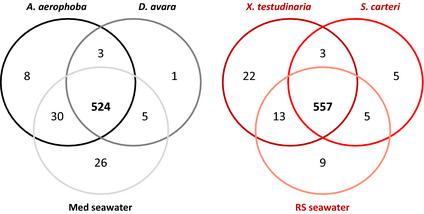
Presence/absence analysis of functional genes identified by the GeoChip. A total of 524 genes (88%) were shared between sources for the Mediterranean site and 557 genes (91%) were shared between sources of the Red Sea site.
Functional convergence of microbial sponge symbionts has previously been reported based on comprehensive metagenomic analyses (Fan et al., 2012) and based on nutrient fluxes in combination with phylogenetic analyses of selected sponge symbionts (Ribes et al., 2012). Here, we extend previous findings in that functional convergence is also reported between sponges (HMA and LMA) and seawater. Several explanations are possible for this observation. For one, the GeoChip may have only limited applicability to sponge microbiomes in the sense that the truly unique microbial genes may not have been covered. Secondly, owing to the presence of seawater bacteria in sponge microbiomes, which has been shown to amount to 5–24% depending on the HMA or LMA status (Moitinho-Silva et al., 2014a), the extracted DNA may have contained a sufficient amount of seawater bacterial DNA to mask true differences between the sponge microbiomes. As a third possible explanation, it may be considered that if seawater was the adaptive driving force, then the functional gene repertoire is going to be the same whether the microorganisms reside inside or outside of a sponge. In the latter hypothesis, it would thus not be surprising to observe functional gene convergence in different sponge or seawater microbiomes.
The few differences that were found and that were of relevance to sponge symbioses are discussed below. Only genes that resulted in statistically significantly different signal intensities in either HMA or HMA sponges in comparison to seawater and where the difference was present at both geographic locations are shown (Figs 4–6). Only the gene categories that were well covered by gene probes (resulting in normalized signal intensity > 5%) are presented below. The unit signal intensity is taken hereafter as a proxy for gene abundance.
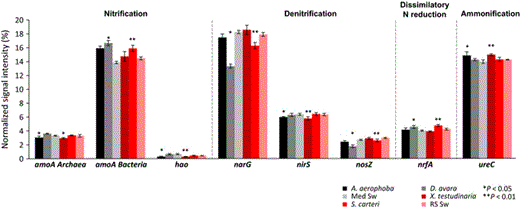
Normalized average signal intensities of genes involved in nitrogen cycling. The microbial processes and corresponding genes are as follows: nitrification (archaeal and bacterial amoA encoding ammonia monooxygenase, hao for hydroxylamine oxidoreductase); denitrification (narG for nitrate reductase, nirS for nitrite reductase, nosZ for nitrate reductase); dissimilatory N reduction to ammonium (nrfA for c-type cytochrome nitrite reductase); ammonification (ureC for urease). Data are presented as the mean ± SE. **P < 0.01, *P < 0.05. ‘Med Sw’ and ‘RS Sw’ stand for Mediterranean and Red Sea seawater.
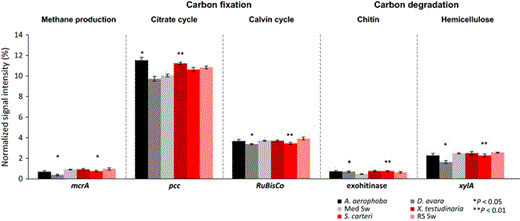
Normalized average signal intensities of gene categories involved in carbon cycling. The microbial processes and corresponding genes are as follows: methane production (mcrA, encoding for methyl coenzyme M reductase A); carbon fixation in the citrate cycle (pcc for propionyl-CoA carboxylase) and in the Calvin cycle (RuBisCO for Ribulose-1, 5-bisphosphate carboxylase/oxygenase); degradation of chitin (with genes encoding for exochitinase) and of hemicellulose (xylA for xylanase). The standard nomenclature of the GeoChip 4 was maintained. Data are presented as the mean ± SE. **P < 0.01, *P < 0.05. ‘Med Sw’ and ‘RS Sw’ stand for Mediterranean and Red Sea seawater.
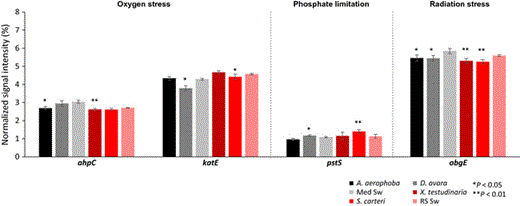
Normalized average signal intensities of gene categories involved in stress. The microbial genes involved in stress response are as follows: ahpC encoding for alkyl hydroperoxide reductase and katE involved in oxygen stress; pstS encoding for a phosphate-binding protein involved in phosphate limitation; obgE encoding for a GTPase involved in radiation stress. Data are presented as the mean ± SE. **P < 0.01, *P < 0.05. ‘Med Sw’ and ‘RS Sw’ stand for Mediterranean and Red Sea seawater.
Nitrogen metabolism
Microbial nitrogen metabolism is a major theme to have emerged out of the past decade of sponge microbiology (Taylor et al., 2007; Hentschel et al., 2012; Webster & Taylor, 2012; Han et al., 2013; Zhang et al., 2014). Sponges excrete ammonia as a metabolic waste product, which makes them a particularly attractive niche for microorganisms in an otherwise nitrogen-poor marine environment. Accordingly, genes corresponding to nearly all major pathways (nitrification, denitrification, ammonification, assimilatory and dissimilatory nitrogen reduction, nitrogen fixation) were identified by GeoChip analyses.
With respect to nitrification, the abundance of the amoA gene, as an indicator for bacterial ammonia oxidation was higher in both HMA and LMA sponges than in seawater (Fig. 4). The archaeal amoA was, however, slightly reduced in HMA sponges and so was the hao gene encoding for hydroxylamine oxidase. In spite of the higher presence of bacterial amoA over archaeal amoA gene, metatranscriptome analyses revealed archaeal ammonia monooxygenase to be among the most highly expressed microbial features in Geodia barretti and S. carteri (Radax et al., 2012; Moitinho-Silva et al., 2014b). Archaea appear to be the main drivers of ammonia oxidation in sponges (Zhang et al., 2014), but also in many other ecosystems such as seawater (Shi et al., 2011) and soil (Leininger et al., 2006).
Several genes encoding for denitrification (narG, nirS, nosZ) were reduced in sponges over seawater (Fig. 4). This observation is consistent with the current perception that sponges have generally aerobic metabolism and that the mesohyl matrix is well oxygenated from the intense pumping activities of the animal. Somewhat contradictory, the nrfA gene abundance, encoding for the dissimilatory reduction of nitrate to ammonia, was slightly increased in LMA sponges over seawater. The sponge tissues were shown to turn anaerobic during periods of nonpumping, so denitrification may still be a likely, if only temporary, scenario (Schlappy et al., 2010).
The symbionts may further cover their nitrogen needs from urea hydrolysation. Urea can originate from a variety of sources (Crandall & Teece, 2012), such as by bacterial degradation of nucleic and amino acids and is therefore a likely product to be encountered in the sponge mesohyl. In fact, the phylogenetic diversity of the ureC gene as well as its transcriptional activity has been demonstrated in the microbial symbionts X. testudinaria (Su et al., 2013). GeoChip analyses revealed higher ureC gene abundance in sponges than in seawater (Fig. 4). This gene encoding for a urease subunit and endows the bacteria with the capacity to hydrolyze urea. Urease-encoding gene clusters, urea transporters, and accessory genes were previously identified in sponge symbiont genomes (Hallam et al., 2006; Siegl et al., 2011). Furthermore, phylogenetic diversity and transcriptional activities of the ureC gene were recently documented for the microbial symbionts of X. testudinaria, providing strong support for an in vivo relevance of microbial urea utilization in sponge symbioses (Su et al., 2013).
Carbon metabolism
Autotrophic carbon fixation is a hallmark of cyanobacteria, including cyanobacterial symbionts of sponges (Taylor et al., 2007). Gene copy numbers for RuBisCO, the key enzyme of cyanobacterial carbon fixation, were however lower in the sponges than in seawater (Fig. 5). On the other hand, the key gene, pcc, encoding for propionyl-CoA-carboxylase and representing the recently discovered autotrophic carbon fixation pathway in archaea (Berg et al., 2007) was higher in HMA sponges than in seawater. It appears likely that the wide-spread sponge symbiont Cenarchaeum symbiosum is responsible for autotrophic carbon fixation via the 3-hydroxypropionate/4-hydroxybutyrate cycle in sponges (Hallam et al., 2006).
With respect to carbon degradation, only two genes were identified as being statistically different between sponges and seawater (Fig. 5). Higher exochitinase gene abundance underlines the potential of sponge symbionts for chitin degradation. In this context, a glycoside hydrolase (GH74) with potential for chitin deacetylation was recently identified on poribacterial genomes by single-cell genomics (Kamke et al., 2013). Alpha-chitin was previously identified as a structural component of the sponge skeleton and may thus likely be encountered in the sponge mesohyl (Ehrlich et al., 2007). The lower abundance the xylA gene indicates that hemicellulose degradation is probably not relevant in sponge microbiomes. Similarly, the lower gene abundance of mcrA, an indicator gene for anaerobic, methanogenic archaea, was lower in sponges than in seawater, indicating that the potential for methanogenesis in sponges is probably limited.
Stress-related genes
A consistently lower abundance of genes involved in stress, particularly with respect to oxygen (ahpC, katE) and radiation stress (obgE) was observed in sponges over seawater (Fig. 6). The finding of reduced obgE gene levels was observed for HMA and LMA sponges at both locations. A microbial existence within animals is frequently correlated with an increased repertoire of stress protection proteins such as chaperonins (Liu et al., 2012; Fan et al., 2013). Whether the symbionts of sponges are more or less stressed than their planktonic counterparts continues to be an interesting topic for future investigations.
The pstS gene, which is involved in phosphate transport, was slightly increased in LMA sponges over seawater, possibly indicating the sponge symbionts are subject to phosphate limitation. In this context, it is interesting to note that the proteobacterial glycerol-3-phosphate ABC transporter was among the highly expressed genes in the S. carteri transcriptome (Moitinho-Silva et al., 2014b). Furthermore, a number of glycerol-3-P ABC-type transporter proteins, termed UgpB, were identified in the metaproteogenome of Cymbastela concentrica (Liu et al., 2012). The Ugp system is thought to be involved in scavenging phosphate-containing compounds (Boos, 1998). Furthermore, single-cell genomic analyses revealed an abundance of phyH genes in poribacterial symbionts of sponges that should endow the bacteria with the ability to utilize 2-aminoethylphosphonate (2-AEPn) as dissolved organic posphorus source (Kamke et al., 2013). However, in spite of several predictions resulting from omics approaches, functional experimental evidence for phosphorous metabolism is still lacking for sponge symbionts.
Conclusions
In conclusion, the following specific insights were obtained: (1) GeoChip analyses by MCE showed, for the first time, that the HMA/LMA dichotomy exists also on the functional gene level. MCE revealed further nonlinear relations between the samples that are only poorly discriminated by conventional methods like PCA (see Fig. S2). Future efforts will be directed at developing algorithms that identify which genes in particular account for the sample separation. (2) Microbial nitrification and ammonification genes were increased in sponges, while denitrification genes were reduced. A higher abundance of archaeal autotrophic carbon fixation genes was noted in sponges than in seawater. Stress genes were found at lower abundances in sponge microbiomes than in seawater. (3) While methodological limitations, such as the applicability of the GeoChip outside of its original ‘soil’ context, cannot be ruled out, it appears nonetheless conceivable that sponge-associated and seawater microorganisms have most of their functional gene repertoire in common.
Authors’ contribution
K.B. and L.M.-S. contributed equally to this work.
Acknowledgements
We thank Martin Pfannkuchen at the Institute Ruder Boskovic (Rovinj, Croatia) as well as the Coastal and Marine Resources Core Lab and the Biosciences Core Laboratory (KAUST, Saudi Arabia) for expert help in sample collection. We thank Zhili He (University of Oklahoma, USA) and Joy D. van Noystrand at Glomics, Inc. for advice. L.M.-S. was supported by a grant of the German Excellence Initiative to the Graduate School of Life Sciences, University of Wuerzburg. Additional financial support was generously provided by KAUST Baseline Funds to T.R.
References
Author notes
Editor: Gary King
German Excellence Initiative to the Graduate School of Life Sciences, University of Wuerzburg
KAUST Baseline Funds