-
PDF
- Split View
-
Views
-
Cite
Cite
Alice Retter, Christian Griebler, R Henrik Nilsson, Johannes Haas, Steffen Birk, Eva Breyer, Federico Baltar, Clemens Karwautz, Metabarcoding reveals ecologically distinct fungal assemblages in river and groundwater along an Austrian alpine to lowland gradient, FEMS Microbiology Ecology, Volume 100, Issue 11, November 2024, fiae139, https://doi.org/10.1093/femsec/fiae139
- Share Icon Share
Abstract
Biodiversity, the source of origin, and ecological roles of fungi in groundwater are to this day a largely neglected field in fungal and freshwater ecology. We used DNA-based Illumina high-throughput sequence analysis of both fungal gene markers 5.8S and internal transcribed spacers region 2 (ITS2), improving taxonomic classification. This study focused on the groundwater and river mycobiome along an altitudinal and longitudinal transect of a pre-alpine valley in Austria in two seasons. Using Bayesian network modeling approaches, we identified patterns in fungal community assemblages that were mostly shaped by differences in landscape (climatic, topological, and geological) and environmental conditions. While river fungi were comparatively more diverse, unique fungal assemblages could be recovered from groundwater, including typical aquatic lineages such as Rozellomycota and Olpidiomycota. The most specious assemblages in groundwater were not linked to the input of organic material from the surface, and as such, seem to be sustained by characteristic groundwater conditions. Based on what is known from closely related fungi, our results suggest that the present fungal communities potentially contribute to mineral weathering, carbon cycling, and denitrification in groundwater. Furthermore, we were able to observe the effects of varying land cover due to agricultural practices on fungal biodiversity in groundwater ecosystems. This study contributes to improving our understanding of fungi in the subsurface aquatic biogeosphere.
Introduction
Fungi are a diverse group of heterotrophic eukaryotic organisms with a multitude of ecological roles. Recent estimates suggest that there may be up to 6 million extant species of fungi (Lücking et al. 2020, Baldrian et al. 2021). They include economically and ecologically relevant groups such as molds, mushrooms, and yeasts, as well as many less studied fungi that do not seem to produce tangible fruiting bodies. Fungal communities are ubiquitous (Naranjo-Ortiz and Gabaldón 2020, Debeljak and Baltar 2023) and have been reasonably well characterized in a number of habitats, such as soils, rivers, lakes, and oceans, or in association with plants or animals (James et al. 2020, Mikryukov et al. 2023). In spite of all scientific progress, fungal communities remain difficult to study due to the limited number of distinct morphological features, high physiological variation, and often cryptic ecology, especially in an ecosystem as inaccessible as groundwater (Grossart et al. 2019, Retter and Nawaz 2022).
Terrestrial groundwater, Earth’s largest reservoir of liquid freshwater, is found below the land surface, and is generally described as thermally stable. The lack of light makes groundwater oligotrophic by nature (Overholt et al. 2022), being characterized by a scarcity of organic matter (OM). This allows groundwater organisms to maintain slow metabolic rates while exhibiting a comparatively lower productivity than surface water ecosystems (Griebler and Lueders 2009). Nonetheless, groundwater is known to support a diverse variety of microbes, for instance bacteria and archaea, but also protozoa (Korbel et al. 2013, Herrmann et al. 2020, Karwautz and Griebler 2022, Fillinger et al. 2023). It provides a relatively stable environment and furthermore shelters its inhabitants from desiccation. In terms of energy, underground layers of water-bearing material (aquifers) may exhibit various redox gradients that microorganisms can exploit for their metabolic processes (Maamar et al. 2015, Fillinger et al. 2023).
To date, our knowledge of the ecological importance of fungi in groundwater, including the suitability of groundwater as a fungal habitat, their diversity and distribution, as well as the pathways of their entry, or the overall existence of resident communities is limited. Recent studies have shown that fungi play fundamental roles in the subsurface ecosystem through their widespread anaerobic metabolism (Müller et al. 2012, Drake and Ivarsson 2018, Hoque and Fritscher 2023), trophic relationships (e.g. symbiosis, parasitism, predation) (Bengtson et al. 2014, Galindo et al. 2018, Grabner et al. 2020), and evolutionary traits such as flagellated unicellular forms and hyphae formation (Rojas-Jimenez et al. 2017, Voigt et al. 2021). To this end, we sampled 45 groundwater wells and 14 river sites at two times of the year along a 300 km altitudinal gradient in the Austrian alps stretching from the alpine region to the lowland (i.e. the foothills of the alps). Hydrological exchange between groundwater and river systems, and subsequent groundwater recharge is largely determined by climatic conditions, soil properties, the depth of the groundwater, and the properties of the surface of the Earth (e.g. altered by anthropogenic degradation such as increased surface sealing, or surface erosion due to intensive agriculture) (Uhl et al. 2022). Thus, fungal communities along the groundwater and river network should potentially be influenced by various landscape (present climatic, topologic, and geologic) and land use characteristics, and we are expecting corresponding patterns in their biodiversity. Understanding the ecological dynamics that shape microeukaryotes across freshwater habitats enables us to relate groundwater to an ecosystem (i.e. the river) that is both hydrologically interdependent and much better understood in terms of fungal and freshwater ecology (Grossart et al. 2019, Swan et al. 2021, Barros and Seena 2022). Additionally, the majority of fungi have been shown to be saprotrophic, feeding on detritus in groundwater (Foulquier et al. 2011, Inkinen et al. 2019, Shamshedenova et al. 2019, Somervuori et al. 2021). We therefore propose that fungi play a vital role in the element and nutrient cycle of groundwater through their versatile metabolic and physiological characteristics. We hypothesize that the effects of local landscape characteristics and environmental conditions, e.g. as a result of hydrologic fluctuations, are reflected in the presence of locally adapted fungi (De Cáceres and Legendre 2009). Our study addresses the complexity of fungal communities in groundwater and the adjacent river in an alpine valley and intents to unravel drivers that determine their biodiversity, ecology, and distribution in the context of landscape characteristics, hydrological and environmental conditions as well as local land cover.
Materials and methods
Study area, field sampling, and data acquisition
Sampling took place in the provinces Styria and Salzburg (Austria) from June to July (spring) and October to November (autumn) 2020. A total of 118 samples were collected in total, consisting of 90 groundwater and 28 surface water samples, including the Source of the Mur River in the Hohen Tauern national park as well as the Lassnitz and Pöls tributaries (Fig. 1). To roughly categorize the river catchment, which follows an altitudinal, land use, and climatic gradient, we defined three different basins, which we called the alpine region, the Graz basin, and the lowland (Fig. 1). To determine land cover in the Mur valley catchment at each sampling site, we used the CORINE Land Cover data (2012–2018; https://www.data.gv.at/, accessed on 1 February 2021). For categorizing lithological characteristics, we utilized the OneGeology survey (https://onegeology.org/), with the permission of OneGeology. Bioclimatic variables of mean annual air temperature (bio1,°C), annual precipitation amount (bio12, kg m−2), and seasonal precipitation as the coefficient of variation (bio15, kg m−2) from the years 1981–2010 were downloaded from CHELSA Bioclim (Karger et al. 2017) to subsequently calculate zonal statistics of our sampling locations.

Map showing the sampling locations of groundwater and surface water as points along the Mur valley in Styria (outlined boundaries) and Salzburg, as well as groundwater bodies along the valley depicted in their regional landscape types (alpine region, Graz basin with the city of Graz, and the lowland).
Sample collection for fungal communities, physicochemical parameters, and basic microbial variables
Groundwater was withdrawn from observation wells established within the past few decades by the provinces of Styria and Salzburg to monitor groundwater quality and quantity (locations, groundwater table, and well depths can be found in Table S1). Consequently, nearly all the wells from which groundwater was sampled have extensive long-term datasets on hydraulic head (groundwater table) and temperature, accessible via www.ehyd.gv.at. Groundwater was withdrawn using a Grundfos submersible MP1 pump (Eijkelkamp Soil & Water Corp., the Netherlands), placed 2 m below the groundwater table. The pumping rate maintained a maximum drawdown of 0.5 m of water table in the well vicinity. Before sample collection, stagnant well water was purged by pre-pumping twice the well water volume, and stability of key physicochemical parameters (electrical conductivity: EC, pH, water temperature, concentration of dissolved oxygen: DO) was considered. During sample collection, the pumping rate was reduced to avoid dislodgement of microbial biofilms and withdrawal of fine sediments. River water samples were collected from the riverbank, if possible, from the free-flowing section. Water temperature, pH, EC, and DO of groundwater and river water were measured on site using field sensors (WTW, Weilheim, Germany). All samples were filled into sterilized canisters washed with a 2% sodium hypochlorite solution and rinsed three times with MilliQ lab water, as well as with sample water. Within 48 h of sampling, in which canisters were stored in a dark cool place, a total of 10 l of groundwater and 4 l of surface water were filtered onto 0.22 µm Sterivex filters (Merck Millipore, Darmstadt, Germany) with a peristaltic pump. Filters were stored right away at −80°C until molecular analysis.
All water samples were analyzed for the concentration of major ions, nutrients (NO3−, NO2−, NH4+, PO43−), dissolved organic carbon (DOC), dissolved inorganic carbon, composition of dissolved organic matter (DOM), and water stable isotope (δ18O, δ2H) signatures as previously described in Retter et al. (2021, 2023). A detailed description of the environmental characteristics of sampling sites is also included therein. DOM was characterized in terms of different fluorescence indices, i.e. HIX (humification index), BIX (freshness/biological index), and FI (fluorescence index), as well as the commonly referenced peaks obtained from aquatic DOM (i.e. Coble peaks b, t, a, m, c; Coble 1996, Coble et al. 2014, Parlanti et al. 2000).
Nucleic acid extraction and Illumina sequencing
Genomic fungal nucleic acids were extracted in two batches using the Norgen RNA/DNA purification kit (Norgen Biotek, ON, Canada). In brief, filters were first removed under sterile conditions and cut up, and 800 µl of the kit’s lysis buffer was added to the filter strips in a sterile 1.5 ml screw cap micro tube containing 0.2 ml of a mix of 1:1 0.1 mm and 0.7 mm Zirconia/Silica beads (Biospec, Bartlesville, USA). Cell lysis was enhanced by incubation for 10 min at 55°C followed by bead-beating. The entire liquid was transferred to a new microcentrifuge tube and centrifuged at 14 000 × g for 2 min. The supernatant was then purified according to the manufacturer’s protocol. DNA was quantified using a Qubit 4 dsDNA assay. Three negative control samples were co-extracted from sterile 0.22 µm Sterivex filters. A primer combination of forward primer ITS3-Mix2 (Tedersoo et al. 2015) and reverse primers ITS4-cwmix1 and ITS4-cwmix2 (Wurzbacher et al. 2017), annealing to the 5.8S gene and the ITS2 region, respectively, was used for amplification following the PCR protocol described by Pjevac et al. (2021), with PCR conditions as described in Retter et al. (2019). Sequencing was done at the Joint Microbiome Facility (JMF) of the Medical University of Vienna and the University of Vienna on a MiSeq platform (Illumina, San Diego, CA, USA), producing 2×300 b paired-end sequences. Sequencing data, generated by the JMF under Project IDs JMF-2009-1 and JMF-2106-09, were deposited under the BioProject accession number PRJNA926931.
Sequence data processing and classification
We applied a two-marker metabarcoding approach making use of a conserved region that helps to anchor the ITS (Heeger et al. 2018, Lücking et al. 2020). Raw reads were processed and classified using the metabarcoding pipeline of Heeger et al. (2019) utilizing the two marker regions 5.8S and ITS2 with default settings (pipeline configurations: conflictBehavior = “mark,” primerError = 0.2, maxAmplLen = 550, minAmplLen = 150). Here, operational taxonomic units (OTUs) were classified by the lowest common ancestor classification using the UNITE reference database release for eukaryotes (ITS2) (v. 9.0, 29.11.2022, Abarenkov et al. 2022) and RFAM database (5.8S) (v. 14.2, Kalvari et al. 2021). OTUs that were classified by either 5.8S or ITS2 as fungi were retained. Taxonomic annotations of the 600 largest (i.e. most abundant) OTUs were manually examined and verified as suggested by Nilsson et al. (2012) using BLAST (Altschul et al. 1997). Clustering sequences into OTUs has previously been shown to outperform amplicon sequence variants in regards to recovering species diversity when it comes to fungal metabarcoding datasets (Tedersoo et al. 2022).
The dataset was decontaminated in R (v. 4.3.1, R Foundation for Statistical Computing 2023) with “decontam” (v. 1.18.0, Davis et al. 2018) with a prevalence-based identification of contaminated sequences using a strict threshold of 0.3. Spring and autumn sequencing batches were analyzed separately against their respective negative control samples. Samples with <100 reads were discarded.
Statistical analysis
In this study, we aim to conduct a comprehensive biodiversity assessment of fungal communities in groundwater and adjacent river ecosystems based on high-throughput amplicon sequencing. To test for differences between groundwater and the river in terms of total estimated richness and Shannon diversity based on OTU abundances, we used the R packages breakaway (v. 4.8.4, Willis and Bunge 2015) and divnet-rs (v. 0.2.1, Willis and Martin 2020). Shannon diversity was calculated as effective number of species (Jost 2006) as Hill-numbers (q = 1) and was calculated from model matrices of tested co-variates with 6 replicates, perturbation set to 0.01, and a medium abundant base taxon, otherwise using default tuning settings. To test diversity estimates between fixed combinations of the covariate-wise differences, we used the functions “betta” and “betta_random” incorporating the sequencing batch affiliation as a random effect, as well as computing a global P-value with an F-test using a bootstrap analysis with 10 000 iterations implemented in the function “test_submodel” in the R package breakaway. To test for differentially relative abundant phyla across groundwater, and the river, we used the “differentialTest” function using a Wald test with a false discovery rate cut-off of 5%, implemented in the corncob package (v. 0.2.0, Martin 2017).
The interpretation of complex multidimensional sequencing datasets requires the implementation of efficient dimensionality reduction techniques to effectively retrieve relevant fungal communities rather than single species/OTUs (Bálint et al. 2016, Leite and Kuramae 2020). To that end, we employed a Latent Dirichlet allocation (LDA) analysis, which employs a probabilistic model to identify groups of OTUs that frequently appear together i.e. communities of fungi, which we term “assemblages.” Here, each sample represents a mixture of assemblages computed as Dirichlet-distributed proportions and thereby reduced to a probability distribution on a fixed set of assemblages (Blei et al. 2003). This method of deconstructing samples into overlapping assemblages of co-occurring fungal taxa has been shown to be a powerful and computationally efficient model-based approach (Sommeria-Klein et al. 2020, 2021). It also enables the linkage of community composition of local communities with environmental data, a task that is frequently challenging due to the size and complexity of metabarcoding datasets. LDA is suited for this task due to its adaptability to accommodate uneven sample sizes (i.e. no prior rarefaction required) and large, sparse datasets commonly encountered in these environments (Sommeria-Klein et al. 2020, Weiss et al. 2016).
We used the R version of the pipeline for LDA analysis of metabarcoding data provided by Sommeria-Klein et al. (2021). We determined the optimal range for the number of assemblages, denoted as K, spanning from 5 to 40. This evaluation of K involved the utilization of two distinct methodologies: (i) a comparison based on Akaike’s information criterion between different algorithm realizations and (ii) perplexity-based cross-validation. The perplexity defines the level of coherence within the dataset. For cross-validation, we randomly split the dataset into 10-sample validation sets. Median perplexity across all validation sets was used to compare K-values. Given the relatively unstructured nature of the groundwater dataset, we employed Gibbs sampling for LDA with 100 Markov Chain Monte Carlo chains, each running for 5000 iterations. The process began with random assemblages, and concentration parameters for the distribution of assemblages over sites and for the taxonomic composition over assemblages were set to α = 0.067 and δ = 0.067, respectively. Following an initial 2000 iterations (burn-in), we captured samples every 25 iterations during the final 3000 iterations. We followed the approach by Sommeria-Klein et al. (2021) and used a heuristic approach to select a K-value near the plateau’s onset of mean perplexity (K = 35). We began by fitting the model to the entire dataset with the K-value that yielded the lowest mean perplexity. We then refined this K-value based on repeating the model computation with a range of K-values (5–15, where 15 was the number of assemblages that had a cumulative prevalence above one sample i.e. the ideal number of assemblages) and choosing the number of K (K = 10) that lead to the least spatial autocorrelation, as well as retrieving similar assemblages across K-values based on correlations with environmental parameters. Correlations of environmental data and assemblage distributions were done by computing the Pearson correlations between the best realization and environmental variables. Spatial autocorrelation of assemblage probabilities was assessed by computing Moran’s I (“Moran.I” in the R package ape; Paradis and Schliep 2019) on each assemblage distribution based on hydrological distances between sites. Hydrological distances were computed as network distances in meters between all sample pairs based on their sub catchment IDs created from the Mur catchment stream network with the function “get_distance_graph” in the hydrographr package in R (Schürz et al. 2023). The stream network graph was calculated based on the Hydrography 90 m dataset taking flow accumulation, flow direction, drainage basins, outlets, stream distance metrics along the network into account (Amatulli et al. 2022). Zonal statistics of bioclimatic variables derived from CHELSA Bioclim were also computed in hydrographr in R with the function “extract_zonal_stats.”
To determine how much of the changes in fungal assemblage composition across ecosystems could be explained by broader categories such as landscape characteristics (climatic variables, water temperature, lithological bedrock characteristics, topology), hydrological (hydrological distances), and environmental (DO, EC, pH, nutrients, major ions, DOM coble peaks, DOM fluorescence indices) conditions as well as local land cover, we applied a variation partitioning analysis based on distance-based redundancy analysis (dbRDA) on response matrices of Euclidean distances of assemblage probabilities between samples. Environmental variables were represented by the first 10 axes of principal components (PCs) derived from a principal component analysis on z-score transformed environmental data using the function “prcomp.” Climatic variables were specified according to the CHELSA Bioclim codes bio1, bio12, and bio15, as well as water temperature. Hydrological conditions were given as hydrological distances between sites based on distance-based Moran’s eigenvector maps (db-MEMs) (Declerck et al. 2011). The db-MEM matrix was created with the “dbmem” function in the R package adespatial (Guénard and Legendre 2022). All variables were selected by forward selection using the adjusted R2 of the full db-RDA model (landscape characteristics, environmental variables, hydrology, land use) as stopping criterion with the function “ordiR2step” (10 000 permutations). The variance inflation factor (VIFs) for each full model was checked before, and collinear variables (VIF > 10) were excluded (Kleinbaum 1978). The marginal significances of each group of variables tested were determined using the “anova.cca” function with 10 000 permutations both implemented in the R package vegan (Oksanen et al. 2022).
Results
Different fungal diversity in river and groundwater
Based on relative OTU abundances, the river water contained a higher total estimated fungal richness (P < .001, F-stat = 237.456) as well as Shannon diversity given as effective number of species (Table 1) (P < .001, F-stat = 728.2094). It furthermore harbored a larger proportion of unique fungal OTUs (51%). Groundwater and river water shared 16% of the OTUs, and these shared fungi (i.e. generalist or transient taxa) were classified as, e.g. Cladosporium, Fusarium, Epicoccum, Vishniacozyma, Filobasidium, Pyrenochaetopsis, Paranamyces, and Mrakia. Especially Cladosporium and Epicoccum showed large log2-fold increases in relative abundance in groundwater (2.1, and 1.5, P < .05), and significant increases for Betamyces and Mrakia (−1.3, −0.95, P < .05) were observed in the river water. More generally, some fungi were significantly differently abundant in surface and groundwater. Members of Chytridiomycota and Basidiomycota were more abundant in the river, whereas Mortierellomycota, Ascomycota, and Rozellomycota were relatively more abundant in groundwater (P < .05, Table S1). Olpidiomycota only occurred in groundwater (Table 1).
The summary statistics of taxon richness and relative abundances in the different types of habitats (groundwater and river) of the most abundant fungal phyla.
. | Groundwater . | Relative richness % . | Relative abundance % . | River . | Relative richness % . | Relative abundance % . |
---|---|---|---|---|---|---|
tot # reads | 64 740 | 26 434 | ||||
tot # OTUs | 1406 | 1942 | ||||
Total estimated Richness | 46.7 ± 9.8 | 240.2 ± 15.1 | ||||
Shannon diversity | 142.8 ± 0.3 | 219.3 ± 2.8 | ||||
Ascomycota | 26 970 | 48.1 | 41.7 | 7979 | 41.8 | 30.2 |
Basidiomycota | 9276 | 30.6 | 14.3 | 6738 | 25.4 | 25.5 |
Mortierellomycota | 12 562 | 3.3 | 19.4 | 22 | 0.2 | 0.1 |
Chytridiomycota | 2137 | 2.8 | 3.3 | 8480 | 12.9 | 32.1 |
Rozellomycota | 3314 | 3.4 | 5.1 | 354 | 3.0 | 1.3 |
Olpidiomycota | 1505 | 0.4 | 2.3 | 1 | 0.0 | 0.0 |
Unclassified Fungi | 8749 | 9.9 | 13.5 | 2808 | 16.2 | 10.6 |
Mucoromycota | 101 | 0.7 | 0.2 | 9 | 0.0 | 0.0 |
. | Groundwater . | Relative richness % . | Relative abundance % . | River . | Relative richness % . | Relative abundance % . |
---|---|---|---|---|---|---|
tot # reads | 64 740 | 26 434 | ||||
tot # OTUs | 1406 | 1942 | ||||
Total estimated Richness | 46.7 ± 9.8 | 240.2 ± 15.1 | ||||
Shannon diversity | 142.8 ± 0.3 | 219.3 ± 2.8 | ||||
Ascomycota | 26 970 | 48.1 | 41.7 | 7979 | 41.8 | 30.2 |
Basidiomycota | 9276 | 30.6 | 14.3 | 6738 | 25.4 | 25.5 |
Mortierellomycota | 12 562 | 3.3 | 19.4 | 22 | 0.2 | 0.1 |
Chytridiomycota | 2137 | 2.8 | 3.3 | 8480 | 12.9 | 32.1 |
Rozellomycota | 3314 | 3.4 | 5.1 | 354 | 3.0 | 1.3 |
Olpidiomycota | 1505 | 0.4 | 2.3 | 1 | 0.0 | 0.0 |
Unclassified Fungi | 8749 | 9.9 | 13.5 | 2808 | 16.2 | 10.6 |
Mucoromycota | 101 | 0.7 | 0.2 | 9 | 0.0 | 0.0 |
Total estimated richness and Shannon diversity based on OTU abundances are shown as mean values and standard errors. Read numbers of each phylum are also shown.
The summary statistics of taxon richness and relative abundances in the different types of habitats (groundwater and river) of the most abundant fungal phyla.
. | Groundwater . | Relative richness % . | Relative abundance % . | River . | Relative richness % . | Relative abundance % . |
---|---|---|---|---|---|---|
tot # reads | 64 740 | 26 434 | ||||
tot # OTUs | 1406 | 1942 | ||||
Total estimated Richness | 46.7 ± 9.8 | 240.2 ± 15.1 | ||||
Shannon diversity | 142.8 ± 0.3 | 219.3 ± 2.8 | ||||
Ascomycota | 26 970 | 48.1 | 41.7 | 7979 | 41.8 | 30.2 |
Basidiomycota | 9276 | 30.6 | 14.3 | 6738 | 25.4 | 25.5 |
Mortierellomycota | 12 562 | 3.3 | 19.4 | 22 | 0.2 | 0.1 |
Chytridiomycota | 2137 | 2.8 | 3.3 | 8480 | 12.9 | 32.1 |
Rozellomycota | 3314 | 3.4 | 5.1 | 354 | 3.0 | 1.3 |
Olpidiomycota | 1505 | 0.4 | 2.3 | 1 | 0.0 | 0.0 |
Unclassified Fungi | 8749 | 9.9 | 13.5 | 2808 | 16.2 | 10.6 |
Mucoromycota | 101 | 0.7 | 0.2 | 9 | 0.0 | 0.0 |
. | Groundwater . | Relative richness % . | Relative abundance % . | River . | Relative richness % . | Relative abundance % . |
---|---|---|---|---|---|---|
tot # reads | 64 740 | 26 434 | ||||
tot # OTUs | 1406 | 1942 | ||||
Total estimated Richness | 46.7 ± 9.8 | 240.2 ± 15.1 | ||||
Shannon diversity | 142.8 ± 0.3 | 219.3 ± 2.8 | ||||
Ascomycota | 26 970 | 48.1 | 41.7 | 7979 | 41.8 | 30.2 |
Basidiomycota | 9276 | 30.6 | 14.3 | 6738 | 25.4 | 25.5 |
Mortierellomycota | 12 562 | 3.3 | 19.4 | 22 | 0.2 | 0.1 |
Chytridiomycota | 2137 | 2.8 | 3.3 | 8480 | 12.9 | 32.1 |
Rozellomycota | 3314 | 3.4 | 5.1 | 354 | 3.0 | 1.3 |
Olpidiomycota | 1505 | 0.4 | 2.3 | 1 | 0.0 | 0.0 |
Unclassified Fungi | 8749 | 9.9 | 13.5 | 2808 | 16.2 | 10.6 |
Mucoromycota | 101 | 0.7 | 0.2 | 9 | 0.0 | 0.0 |
Total estimated richness and Shannon diversity based on OTU abundances are shown as mean values and standard errors. Read numbers of each phylum are also shown.
Biodiversity of fungal assemblages across ecosystems
In this dataset, 10 distinct fungal assemblages could be recovered by LDA modeling, and average assemblage probabilities across samples were the highest in assemblage 1 (∼0.20) and decreasing towards assemblage 10 (∼0.03) (Figure S2). Some assemblage probabilities differed significantly along the river valley basins (Fig. 1, alpine region, the Graz basin, and lowland) as well as between ecosystems (groundwater and river) and seasons (spring and autumn) (Table S2). Assemblage 10 showed significant spatial autocorrelation and was not well resolved by the model in terms of fungal taxa (i.e. all fungal OTUs present in the data had a non-zero probability to occur in this assemblage) and was therefore excluded from further discussion (Table S2).
Linking LDA derived assemblages to environmental drivers
Assemblage 2 was strongly associated (i.e. high probabilities) with the river during spring season (Fig. 2A, Table S3) and correlated positively with microbial productivity (prokaryotic cell counts and cellular ATP concentrations), pH, annual precipitation, DOC, DO, DOM peaks for protein-like coble fluorophores b (Tyrosine-like DOM) and t (Tryptophan-like DOM), and negatively with annual mean air temperature (°C), major ions and conductivity, and HIX (humified DOM) (Fig. 3). Autumn river fungal communities were characterized by a shift towards assemblage 4 (Fig. 2), when the river system became less productive and generally lower in water temperature. Assemblage 4, in contrast to the river spring assemblage, was correlating to a lesser extent with microbial productivity and protein-like DOM, but additionally with DOM derived coble peaks a, c (both terrestrial humic substances), and m (i.e. marine and terrestrial humic material).
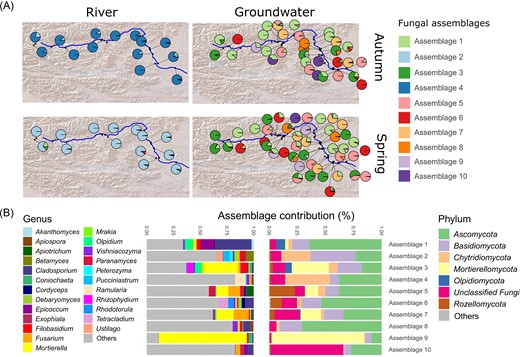
Groundwater and river assemblages of co-occurring OTUs along the alpine gradient. (A) Base map (ESRI World Shaded Relief) with sites depicting the relative assemblage proportion of the 10 recovered assemblages as pie charts with assemblages 1–10 shown in their respective colors. (B) Relative contributions of fungal taxa to assemblages on genus (left) and phylum (right) level, respectively.
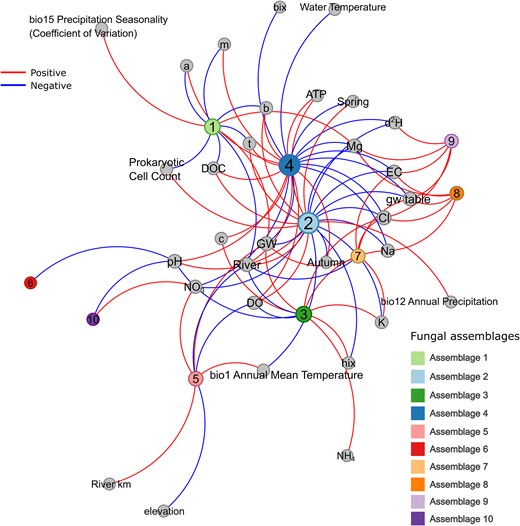
Network plot of assemblages correlating with environmental variables (Pearson correlation; P < .05) visualized using the Force Atlas layout in Gephi (https://gephi.org/) with blue edges depicting negative correlations and red edges depicting positive correlations. The size of nodes represents the node degree, which depicts the total number of edges (environmental variables) connected to that node (c = coble peak c, b = coble peak b, t = coble peak t, a = coble peak a, m = coble peak m, hix = humification index, bix = biological/freshness index, d2H = ratio of stable hydrogen isotopes, DO = dissolved oxygen, ATP = intracellular ATP).
The assemblages 1, 3, 5, and 8 were predominantly associated with groundwater and are further characterized in the following (Table S3). High assemblage 1 probabilities were associated with groundwater low in organic material (DOC and DOM) and located in areas with high seasonal variation in precipitation. The combined effect of season, ecosystem type, and regionality (Table S3) hints at this assemblage being more prevalent in autumn throughout the alpine mountain range. Assemblage 3 on the other hand seemed to predominate groundwater high in ions (Na, K, Cl), ammonium, and humified organic material of terrestrial origin (HIX, coble peak c) coinciding with a lower pH (on average 6.8) and lower DO. This groundwater assemblage weakly coincided with the spring season (Dunn’s test, with Bonferroni adjusted P-value = .025). In contrast, assemblage 5 was characteristic for the lowland and correlated positively with nitrate concentrations and annual mean air temperature, and negatively with DO. Assemblage 8 showed high probabilities in groundwater recovered from the Graz basin (Table S3), and the assembly correlated strongly with ions (Mg, Na, Cl), EC, and was predominantly recovered from deeper groundwater (i.e. low groundwater levels).
Fungal taxa linked to LDA depicted assemblages
River assemblages 2, and 4 are the most similar in terms of the presence of fungal taxa (Fig. 4B). Assemblage 2 (River spring) contains the highest diversity in terms of OTU richness (1305 OTUs), and most of the fungal taxa belong to Ascomycota (34.2%), Basidiomycota (25.7%), unclassified fungi (24.4%), and Chytridiomycota (11%). Common genera (i.e. high proportions) in this assemblage are Ustilago, Pucciniastrum, Filobasidium, Peterozyma, Betamyces, Mrakia, and Aureobasidium, where members of the genus Pucciniastrum are unique to this assemblage (Fig. 4A). Some of the most OTU-rich genera that occur uniquely in this assemblage belong to Mrakia, Betamyces, and Rhizophydium. In assemblage 4 (River autumn), fungal richness is 884, of which 48.8% are Ascomycota, 21.2% Basidiomycota, 17.1% unclassified fungi, and 8.9% Chytridiomycota. Genera predominantly linked to this assemblage are Ramularia, Fusarium, and Flagellospora. Here, members of the genus Ramularia present only in this assemblage are also comparatively rich in OTUs.
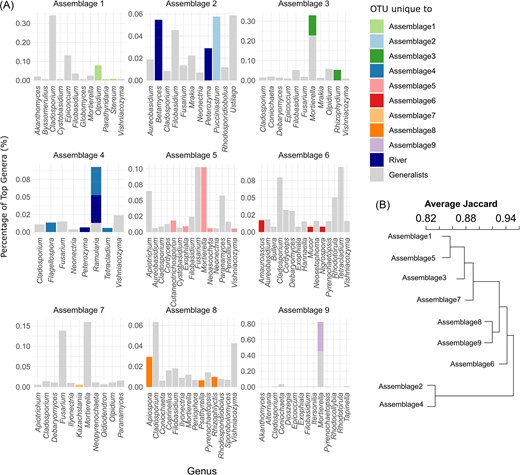
(A) Taxonomic composition of individual assemblages showing the most prevalent OTUs on genus level and proportions shown in respective assembly colors when OTUs are unique to an assembly, colored in gray when they occur in all assemblies (Generalists) or are colored in blue when they are unique to the river assemblies (River). (B) Dendrogram based on the Jaccard distance between assemblies computed from the presence or absence of fungal OTUs in each assemblage.
OTU diversity further decreases towards the groundwater assemblages. Assemblage 1 richness is 411, which is the highest among groundwater assemblages. It is mainly composed of Ascomycota (43.1%), Basidiomycota (34.5%), unclassified fungi (13.4%), and Chytridiomycota (4.9%). Genera prevalent in this assemblage are Cladosporium, Epicoccum, and Olpidium, where some members of Olpidium are unique to this assembly, but only containing two species. The second richest assemblage in groundwater is 3 (379 OTUs), which is mainly characterized by Ascomycota (45.1%), Basidiomycota (36.4%), and unclassified Fungi (10.6%). Common genera include Mortierella, Olpidium, and Rhizophydium, with some species of Mortierella and Rhizophydium being unique to this assemblage. In both assemblages, 1 and 3 Vishniacozyma, Dioszegia, Mortierella, and Filobasidium are particularly rich in OTUs. Assemblage 5 has a fungal richness of 329, and most fungi belong to Ascomycota (49.8%), Basidiomycota (31.3%), and unclassified fungi (10.3%). Common genera are Fusarium, Mortierella, Apiotrichum, and Paranamyces with some species belonging to Mortierella, Cutaneotrichosporon, and Exophiala being unique to this assemblage. Here, Exophiala, Mortierella, and Fusarium are especially rich in OTUs. The groundwater associated assemblage with the lowest diversity (130 OTUs) is assemblage 8 and comprises mainly of Ascomycota (45.5%), Basidiomycota (38.5%), and unclassified fungi (9.2%). Genera tightly linked to this assembly are Cladosporium, Vishniacozyma, and Apiospora, where Apiospora, Rhizophlyctis, and Psathyrella are uniquely occurring. The genus richest in OTUs is Filobasidium.
Trophic modes of fungal assemblages
In this dataset, OTUs present in each assemblage were matched to growth form and primary lifestyle at genus level utilizing the database of Põlme et al. (2020). Fungal river assemblages (2 and 4) contained a larger share of OTUs not classifiable to the genus level (mean proportion = 64.4%) compared to groundwater (mean proportion = 51.6%) (Figure S3A). Fungi forming filamentous mycelia as well as dimorphic yeasts were more prevalent in groundwater assemblages compared to the river communities (Dunn’s multiple comparisons, Bonferroni adjusted P < .05). Dung saprotrophs, pollen saprotrophs, and algal parasites were significantly more prevalent in river assemblages, whereas groundwater assemblages contained a relatively larger share of soil saprotrophic, animal, and mycoparasitic fungal OTUs (Dunn’s multiple comparisons, Bonferroni adjusted P < .05) (Figure S3B).
Landscape characteristics shape fungal assemblages
To estimate the contribution of landscape characteristics, environmental and hydrological conditions, and local land cover to the observed distribution patterns of fungal assemblages along the river valley, a variation partitioning analysis was done. When assessing the total variation explained by broader categories, landscape characteristics explained the largest portion of total variation in fungal probabilities between sites (10.8%) and was followed by environmental differences (9.5%), and land use patterns (4.6%) (Table 2). Hydrological connectivity between sites played only a minor role.
Partition of variation in assemblage probabilities over sites between environmental, biogeographical, and hydrological conditions and local land cover.
Group . | Significant variables . | R2 adj . | DF . |
---|---|---|---|
Environment | PC1 + PC6 + PC3 + PC4 | 0.176 | 4 |
Landscape | Water temp + lithological char + bio15 | 0.105 | 11 |
Land use | Land use 2nd level | 0.053 | 12 |
Hydrology | dbMEM1 | 0.009 | 1 |
Environment | landscape + land use + hydrology | 0.095 | 4 | |
Landscape | environment + land use + hydrology | 0.108 | 9 | |
Land use| environment + landscape + hydrology | 0.046 | 10 | |
Hydrology| landscape + land use + environment | 0.010 | 1 | |
Residuals | 0.701 |
Group . | Significant variables . | R2 adj . | DF . |
---|---|---|---|
Environment | PC1 + PC6 + PC3 + PC4 | 0.176 | 4 |
Landscape | Water temp + lithological char + bio15 | 0.105 | 11 |
Land use | Land use 2nd level | 0.053 | 12 |
Hydrology | dbMEM1 | 0.009 | 1 |
Environment | landscape + land use + hydrology | 0.095 | 4 | |
Landscape | environment + land use + hydrology | 0.108 | 9 | |
Land use| environment + landscape + hydrology | 0.046 | 10 | |
Hydrology| landscape + land use + environment | 0.010 | 1 | |
Residuals | 0.701 |
Group|Group+Group represents the marginal fraction of variation explained by each group after controlling for the respective others. Significance of each individual group was assessed by 10 000 permutations.
Partition of variation in assemblage probabilities over sites between environmental, biogeographical, and hydrological conditions and local land cover.
Group . | Significant variables . | R2 adj . | DF . |
---|---|---|---|
Environment | PC1 + PC6 + PC3 + PC4 | 0.176 | 4 |
Landscape | Water temp + lithological char + bio15 | 0.105 | 11 |
Land use | Land use 2nd level | 0.053 | 12 |
Hydrology | dbMEM1 | 0.009 | 1 |
Environment | landscape + land use + hydrology | 0.095 | 4 | |
Landscape | environment + land use + hydrology | 0.108 | 9 | |
Land use| environment + landscape + hydrology | 0.046 | 10 | |
Hydrology| landscape + land use + environment | 0.010 | 1 | |
Residuals | 0.701 |
Group . | Significant variables . | R2 adj . | DF . |
---|---|---|---|
Environment | PC1 + PC6 + PC3 + PC4 | 0.176 | 4 |
Landscape | Water temp + lithological char + bio15 | 0.105 | 11 |
Land use | Land use 2nd level | 0.053 | 12 |
Hydrology | dbMEM1 | 0.009 | 1 |
Environment | landscape + land use + hydrology | 0.095 | 4 | |
Landscape | environment + land use + hydrology | 0.108 | 9 | |
Land use| environment + landscape + hydrology | 0.046 | 10 | |
Hydrology| landscape + land use + environment | 0.010 | 1 | |
Residuals | 0.701 |
Group|Group+Group represents the marginal fraction of variation explained by each group after controlling for the respective others. Significance of each individual group was assessed by 10 000 permutations.
Discussion
In the present study, we explored an Austrian alpine-river gradient for fungal diversity and distribution across groundwater and river water. Diversity was considered both in terms of taxonomy and ecological roles. A number of significant patterns were recovered for both ecosystem types and are discussed in detail below.
The ecology of fungal river assemblages
It is intriguing that in this study, some fungal taxa have been found exclusively in certain assemblages, potentially serving as indicators for local environmental conditions. The river community in spring (assemblage 2) was the richest in fungal taxa and exhibited a strong association with key environmental parameters as well as with concentrations of DOC and protein-like DOM. Elevated protein-like DOM and DOC during spring have shown to be associated with the breakdown products of lignin, or leaching of exudates from leaf emergence (Singh et al. 2014). It could also correspond to recent in-stream production (i.e. phytoplankton growth) and processing of OM, or could indicate influence by human activities (i.e. a shift from more plant/soil derived DOM to more microbial/algal DOM) (Jørgensen et al. 2011, Lambert et al. 2017, Ren et al. 2021). This assemblage contained the only Pucciniastrum species present in this data set, P. areolatum, which is the causal agent of spruce rust and produces basidiospores during spring. These basidiospores are propagated by wind and are probably introduced into and spread by the river system (Zhang et al. 2021). Many species of Mrakia associated with this assemblage are psychrophilic and produce both yeast and hyphal states (dimorphic). They have the ability to produce cold-active pectinases and different hydrolytic enzymes, which indicates their potential to decompose OM in cold environments (Bezus et al. 2022). A number of Mrakia species have previously been reported from freshwater ecosystems, including polar regions, glacial meltwater, snow, rivers, and wetlands (Fell 2011, Grossart et al. 2019, Jones et al. 2014). The chytrid genus Rhizophydium contains many zoosporic taxa known to be parasitic on algae and cyanobacteria (e.g. Planktothrix) or saprotrophic (e.g. cellulosic and keratinophilic) in soil, aquatic habitats, or on pollen. This chytrid potentially coincides with phytoplankton river spring blooms (Figure S4) (Letcher et al. 2004, Longcore and Simmons 2012, Maier and Peterson 2017, Wagner et al. 2023). The zoosporic chytrid Betamyces was also characteristic of and specious in this assemblage, including the only known species Betamyces americae-meridionalis. Betamyces spp. have previously been reported from pollen and soil, and they have been found to be highly abundant in association with microplastics in Arctic freshwater lakes (Gonçalves et al. 2022, González-Pleiter et al. 2021, Letcher et al. 2012).
The fungal river assemblage coinciding with autumn (assemblage 4) was characterized by significantly cooler water temperatures and higher terrestrial and humic, but fewer freshly produced DOM compared to spring river conditions. It only contained one exclusive fungal genus, namely the plant pathogen Ramularia, above all its species R. miae and R. unterseheri. R. miae was first observed causing black leaf spots in a bloodwort species native to South Africa, but has since been isolated from multiple hosts (Videira et al. 2015a). Ramularia unterseheri is also plurivorous, often recovered from dead or alive leaves of Fagus (Videira et al. 2015b). Most plants undergo changes in growth and physiology during autumn and are therefore more vulnerable and susceptible to Ramularia infections, probably leading to higher prevalence of infections and completion of the pathogen’s life cycle and the dispersal of spores during that time. An increase in terrestrial organic and humic substances can be linked to an increased import of usually fungi-rich substrates (e.g. leaf litter—a primary source of allochthonous OM in rivers and streams) during autumn and winter (Chrismas et al. 2023). Indeed, we found that the remaining extant unique genera were well-known leaf-litter decomposers and/or leaf inhabitants (i.e. “hyphomycetes,” Flagellospora, Tetracladium, Tetrachaetum, Moellerodiscus), some of them being sensitive to water pollution (Baschien et al. 2013, Charcosset and Gardes 1999, Duarte et al. 2012, Ghate and Sridhar 2015, Pietryczuk et al. 2018). The most specious genera in this assemblage, Dioszegia, and Vishniacozyma, belong to psychrophilic/psychrotolerant, halotolerant, and/or osmotolerant yeasts often recovered from glacial, cold, and/or arid regions from e.g. meltwater, groundwater and volcanic springs (Buzzini et al. 2018, Jones et al. 2014, Wurzbacher et al. 2020, Nuppunen-Puputti et al. 2021). They are known, for instance, to inhabit soil rich in OM and potentially contribute to nutrient cycling and the decomposition of diverse types of organic compounds (Abu-Mejdad et al. 2019, Maeng et al. 2022).
The prevalence of dung and pollen saprotrophs as well as algal parasites in the river is compatible with the available OM sources and hosts. River systems are heavily impacted by their surrounding environments (through land cover), and they are typically characterized by high primary production. Dung and pollen saprotrophs benefit from the influx of OM from terrestrial sources, while algal parasites thrive in environments with abundant host biomass. Taxonomic classification to genus level was only possible for around 36% of the OTUs from the river assemblages, probably due to the high abundance of early diverging fungi (i.e. Chytridiomycota) in the river known to fail during ITS classification, as well as their underrepresentation in the UNITE database (Heeger et al. 2019).
The ecology of fungal groundwater assemblages
The specific environmental conditions shaping the specious groundwater assemblage 1 follow the regional distribution pattern of the number of rainy days. A higher coefficient of variation in seasonal precipitation indicates a larger variability in precipitation throughout the year due to convective precipitation (i.e. increased rain and storm events) along the mountain range compared to the lowland. This is also corresponding to the existing weather gradient between the rainy north and the less rain-rich south of the alpine region, and in the south-eastern foothills of the Alps to a decrease in rainy days with distance from the mountain range (Wakonigg et al. 2008). Higher variation in precipitation can potentially lead to increased hydrological mixing in groundwater, promoting the dispersal and introduction of fungal propagules (e.g. spores or filaments), and subsequently a higher fungal diversity. The assemblage was also typical for groundwater largely deprived of protein-like, terrestrial, and aquatic humic OM (e.g. stream, and algal DOM), possibly due to dilution effects (Søndergaard et al. 2003, Foulquier et al. 2010, Coble et al. 2014). Unique fungal taxa were identified as Olpidium brassicae, Stereum hirsutum, Parathyridaria clematidis, and Pseudotaeniolina globosa. Olpidium brassicae is a unicellular, zoosporic plant endoparasite, often in the roots of vascular plants, and known to be transmitted when water is recirculated and exchanged (Runia 1994). Stereum hirsutum is a lignicolous white-rot fungus that is known to produce a wide range of metabolites (terpenes, acetylenic aromatic, alkyl, and benzoate derivates) (Pu et al. 2021, Liu et al. 2023). Not much is known about P. clematidis on the other hand, even though a few other members of the genus have previously been isolated from the marine environment (Dayarathne et al. 2020, Poli et al. 2020). Pseudotaeniolina globosa is a known rock inhabiting fungi that is meristematic and melanized and associated with mineral weathering (Necropolis et al. 2021).
The second richest assemblage in groundwater (assemblage 3) was characterized by elevated ammonium concentrations under more reducing conditions and lower pH, possibly due to denitrification of infiltrated nitrate as a result of the application of fertilizers and irrigation (Huang et al. 2016, Korbel et al. 2022). Additionally, potassium and recalcitrant, aromatic, fulvic-like DOM (coble peak c) were comparatively higher in groundwater coinciding with assemblage 3. This DOM component seems to be the major form of DOM found in groundwater throughout the year and could be elevated in spring due to the input of terrestrial derived OM following snow melt (Harjung et al. 2023). Its degradation in a reducing environment could additionally lead to increased ammonium levels in groundwater (Huang et al. 2021). Unique fungal taxa in this assemblage belonged to the genera Mortierella, and Rhizophydium, the latter being more variable than what was previously understood (Longcore 2004, Sigee 2005, James et al. 2006). Species rich genera present in both groundwater assemblages 1 and 3 were members of the yeast genera Dioszegia, and Vishniacozyma, as well as Mortierella, including M. alpina. The latter is an oleaginous species that accumulates a diverse range of fatty acids, comprising of up to 50% of its dry weight (Wang et al. 2011). It has previously been found in association with soil, seaweed, and peatlands (Asemaninejad et al. 2017, Poli et al. 2022, Gonçalves et al. 2023). More generally, members of Mortierella are saprotrophic, known for decomposing labile carbon. They are commonly isolated from soil and have previously been identified as one of the dominant genera in groundwater (Asemaninejad et al. 2017, Nawaz et al. 2018, Nuppunen-Puputti et al. 2021).
Assemblage 5 was indicative of reduced groundwater influenced by agricultural land use, as suggested by high nitrate concentrations at these sites and the fact that these land use practices intensify in the lowland, spatially coinciding with increased assemblage probabilities (Graeber et al. 2012) (Fig. 4A). Here, unclassified members of Peniophora were particularly rich in taxa. Peniophora encompasses corticioid fungi with lignolytic and antiprotozoal activities, some of them causing white-rot, but also found to be associated with beetles and sea grass (Valmaseda et al. 1990, Heitman et al. 2017, Xu et al. 2023). Moreover, dominant taxa, such as members of Fusarium, Apiotrichum, and Mortierella, are known for their nitrate utilization capabilities, and fungal denitrification is indeed a major pathway in natural systems, sometimes exceeding bacterial denitrification capacities (Takaya 2002, Shoun et al. 2012, Zhong et al. 2022, Zuo et al. 2023). The oleaginous yeast Cutaneotrichosporon, unique to this assemblage, has been shown to utilize a broad spectrum of especially aromatic compounds for fatty acid biosynthesis (Yaguchi et al. 2020). These are compounds that most bacteria are not capable of degrading. Some species of Cutaneotrichosporon, as well as the other exclusively associated fungal genus with assemblage 5, Exophiala, may furthermore be parasitic on animals, including humans (Najafzadeh et al. 2018, Aliyu et al. 2020).
The last groundwater assemblage (8), which was also lowest in species diversity, coincided with higher concentrations of ions and lower groundwater levels in the Graz basin, suggesting a community locally adapted to infiltration of, e.g. road salt, industrial discharges, or effluents from local wastewater treatment systems (Howard and Gerber 2018, Becher et al. 2022). Fungi unique to this assemblage were, e.g. Apiospora marii and Rhizophlyctis, specifically R. rosea. A. marii could previously be isolated from stems and roots of plants causing plant dieback and wilt (Agustí-brisach et al. 2023). Rhizophlyctis rosea is one of the most ubiquitous cellulose degrading zoosporic soil chytrids, and their presence might suggest a dynamic groundwater-surface exchange in urban areas (Longcore and Simmons 2012, Jones and Bennett 2014). Since it produces motile zoospores, we propose that the vadose zone could serve as a potential habitat for this chytrid, as long as oxygen is abundant (Willoughby 2001, Gleason et al. 2019).
The overall high prevalence of soil saprotrophic fungi in groundwater could putatively be explained by ongoing exchange processes of groundwater with the surface via the soil. We think it is likely that soil fungi may find a suitable habitat in groundwater, or the vadose zone, contributing to ongoing ecosystem processes with their unique, versatile metabolic capabilities. This would have to be further investigated by targeting not the total but the active fungal communities in groundwater via e.g. RNA sequencing or omics approaches. Our results agree with previous findings that filamentous fungi, as well as fungi that form yeast stages are more often found in groundwater compared to the river system (Wurzbacher et al. 2020, Afonso et al. 2021). The fact that animal pathogens (some of which are also known to infect humans) are primarily present in groundwater affected by intensive agriculture should raise concerns regarding their increased transport facilitated by habitat fragmentation and human use. These pathogenic fungi are possibly diluted in streams after their introduction via fertilization with manure, whereas they can reside in groundwater for a prolonged period of time.
Landscape characteristics drive differences in fungal assemblages
Our analysis showed that local landscape characteristics, including climatic conditions that translate into river and groundwater temperatures, bedrock lithology, as well as precipitation patterns were detrimental factors in shaping fungal communities across groundwater and river ecosystems. Differences in local environmental conditions, as well as variations in land use were additional drivers. Even though lithological conditions do not seem to be a major driver for bacterial communities (Maamar et al. 2015), we show that they are significantly shaping fungal assemblages in groundwater. For instance, some fungal species are known for their granite weathering capabilities, and different rock surfaces have been found to harbour a distinct selection of fungal communities (Knudsen and Vesterholt 2008, Nuppunen-Puputti et al. 2021, Li et al. 2022, Liu et al. 2022a).
In conclusion, our study revealed significant differences in fungal diversity and relative abundances of fungal groups between groundwater and the river. Unlike bacterial communities, where biodiversity in groundwater exceeds that of surface waters, fungi are less likely to establish diverse communities in groundwater (Ji et al. 2022, Retter et al. 2023). We attribute this difference both to the restricted exchange of groundwater systems with the land surface and surface waters and to the reduced input of organic material compared to riverine environments. While it is apparent that fungal communities in groundwater contain numerous degraders of OM and that fungal diversity was relatively high in groundwater receiving terrestrial input in the form of humic and fulvic OM, the most specious assemblage was existing at sites containing lower OM. This, in turn, is likely attributable to the highly variable precipitation and storm events at these sites, possibly diluting organic material and at the same time introducing transient fungi into groundwater. The presence of transient fungal taxa found in both groundwater and the river supports the notion of ecological connectivity between these two contrasting ecosystems. Even though spanning both ecosystems, OTUs belonging to Cladosporium, and Epicoccum seem to be abundant in—and characteristic of—groundwater, while Dioszegia and Vishniacozyma are found across ecosystems in comparable proportions (Nawaz et al. 2018, Perkins et al. 2019, Afonso et al. 2021, Liu et al. 2022b). Moreover, parasitic fungi regularly recovered from aquatic ecosystems are likely to require a certain amount of hosts—mostly phototrophs—and are therefore absent in the groundwater environment (Frenken et al. 2017, Van den Wyngaert et al. 2022, Klawonn et al. 2023). Our study emphasizes that a consistent and diverse group of fungi perceives the vast, energy limited, and light deprived groundwater as a suitable habitat and that these fungi could be linked to specific environmental conditions, corresponding ecological functions, and niches.
Acknowledgements
We thank P.R., B.S. (Steiermärkische Landesregierung), H.H., and M.G.-K. (Salzburger Landesregierung) for their valuable information and help in selecting appropriate groundwater sampling wells. Further, we want to acknowledge P.P. at the Joint Microbiome Facility (JMF) for sequencing and quality control of raw reads. We also thank L.F., C.E., F.S., G.R.H.K., and S.G. for their help and support during sampling, lab work, and data analysis.
Author contributions
Alice Retter (Conceptualization, Data curation, Formal analysis, Methodology, Software, Validation, Visualization, Writing – original draft, Writing – review & editing), Christian Griebler (Conceptualization, Funding acquisition, Investigation, Methodology, Project administration, Resources, Supervision, Writing – original draft, Writing – review & editing), R. Henrik Nilsson (Supervision, Validation, Writing – original draft, Writing – review & editing), Johannes Haas (Formal analysis, Visualization, Writing – original draft, Writing – review & editing), Steffen Birk (Conceptualization, Funding acquisition, Project administration, Resources, Supervision, Writing – original draft, Writing – review & editing), Eva Breyer (Writing – original draft, Writing – review & editing), Federico Baltar (Supervision, Validation, Writing – original draft, Writing – review & editing), and Clemens Karwautz (Methodology, Validation, Writing – original draft, Writing – review & editing)
Conflict of interest
None declared.
Funding
This work was supported by the ÖAW (Österreichische Akademie der Wissenschaften), grant number ESS-Project 2018: “Integrative Groundwater Assessment.”