-
PDF
- Split View
-
Views
-
Cite
Cite
Sára Šardzíková, Marta Gajewska, Norbert Gałka, Matúš Štefánek, Andrej Baláž, Martina Garaiová, Roman Holič, Wiesław Świderek, Katarína Šoltys, Can longer lifespan be associated with gut microbiota involvement in lipid metabolism?, FEMS Microbiology Ecology, Volume 100, Issue 11, November 2024, fiae135, https://doi.org/10.1093/femsec/fiae135
- Share Icon Share
Abstract
Biological aging is linked to altered body composition and reduced neuroactive steroid hormones like dehydroepiandrosterone sulfate (DHEAS), which can stimulate the GABA signaling pathway via gut microbiota. Our study examined the association of gut microbiota with lifespan in mice through comprehensive analysis of its composition and functional involvement in cholesterol sulfate, a precursor of DHEAS, metabolism. We used 16S rRNA and metagenomic sequencing, followed by metabolic pathway prediction and thin layer chromatography and MALDI-TOF cholesterol sulfate identification. Significant increases in bacteria such as Bacteroides, typical for long-lived and Odoribacter and Colidextribacter, specific for short-lived mice were detected. Furthermore, for males (Rikenella and Alloprevotella) and females (Lactobacillus and Bacteroides), specific bacterial groups emerged as predictors (AUC = 1), highlighting sex-specific patterns. Long-lived mice showed a strong correlation of Bacteroides (0.918) with lipid and steroid hormone metabolism, while a negative correlation of GABAergic synapse with body weight (−0.589). We found that several Bacteroides species harboring the sulfotransferase gene and gene cluster for sulfonate donor synthesis are involved in converting cholesterol to cholesterol sulfate, significantly higher in the feces of long-lived individuals. Overall, we suggest that increased involvement of gut bacteria, mainly Bacteroides spp., in cholesterol sulfate synthesis could ameliorate aging through lipid metabolism.
Introduction
Aging populations represent a global challenge for governments and healthcare. Age-related conditions, such as osteoarthritis, diabetes, depression, and dementia, are increasing. Improved living standards have extended the lifespan expectations (https://www.who.int/news-room/fact-sheets/detail/ageing-and-health). Although genetics, environment, and lifestyle have been studied for longevity, recent research has highlighted the crucial role of the gut microbiota (Kato et al. 2018, Badal et al. 2020). However, the precise mechanisms linking gut microbes to the lifespan remain unclear. These findings open exciting avenues for research, delving into the intricate symbiosis between gut microbes and aging pathways and offering potential insights for healthier aging.
Throughout life, the composition and function of the gut microbiota are influenced by diet, circadian rhythms, food timing, and sex, with a reciprocal relationship with steroid hormones (Ursell et al. 2012, Hasan and Yang 2019). Hormonal imbalances, a natural part of aging, involve hormonal changes, such as estrogen, testosterone, thyroid hormones, and growth hormones, leading to various age-related health issues, such as menopause, decreased bone density, and slowed metabolism (Pataky et al. 2021, Mooradian 2023). Hormones are crucial for extending lifespan through pathways such as insulin, insulin-like growth factor, dafachronic acids, and transforming growth factor-beta (Tatar et al. 2003, Granata 2016, Duran-Ortiz et al. 2021, Harrison et al. 2021). For an increase in longevity, the involvement of steroid hormones synthesized in gonads and other tissues, like the brain (neurosteroids), has been suggested. A specific abundance of steroid hormones circulates in the periphery, chemically modified by sulfation, representing a reservoir with potential for future activity as neurosteroids. In Caenorhabditis elegans, germline ablation or inactivation of steroid sulfatase leads to increased levels of sulfated hormones directly associated with increased longevity (Pérez-Jiménez et al. 2021). Aging is characterized by an imbalance between anabolic hormones (testosterone and insulin-like growth factor-1) and sulfated steroids (DHEAS), which decrease with age, and catabolic hormones (cortisol and thyroid hormones), which remain stable (Maggio et al. 2007, 2010). Research suggests that testosterone insufficiency may increase the risk of death (Laughlin et al. 2008), while higher secretion may shorten men's lifespan (Nieschlag et al. 1993).
In contrast, estrogen levels are associated with longer lifespan (Stanhewicz et al. 2018). Reducing estrogen levels before puberty may extend the period between birth and puberty, potentially leading to extended body maturation and, thus, a longer lifespan (Faubion et al. 2015, Yu et al. 2022). On the other hand, maintaining high estrogen levels in the reproductive stage and postmenopause may also extend lifespan (Nappi et al. 1999, Faubion et al. 2015). Cortisol, another steroid hormone, is associated with a higher perceived age (Noordam et al. 2012). Nevertheless, the mechanisms by which hormones influence the length of life remain unclear.
Gut microbiota plays a crucial role within the gut–brain axis and is involved in the hypothalamic–pituitary–adrenal axis (Carabotti et al. 2015). It can also affect hormone regulation and production beyond the gut, with correlations found between serum sex hormone levels and gut microbiota diversity and composition (Shin et al. 2019). Steroids, such as progesterone, testosterone, and their metabolites dihydroprogesterone or 17β-estradiol, can also be synthesized within the nervous system, calling them neurosteroids, and regulating behavior, neurogenesis, neurite growth, and catecholamine production (Schumacher et al. 2000, Larson 2018). Specific pathogen-free and germ-free mice display different levels of these neurosteroids in specific brain areas. Higher levels of allopregnanolone together with decreased 5α-androstane-3α, 17β-diol in the plasma, and changes in dihydroprogesterone and isoalloprenanolone levels in the hippocampus, cerebral cortex, and cerebellum were observed in germ-free mice, which supports the evidence regarding the impact of the gut microbiota on neuroendocrine pathways (Diviccaro et al. 2021). These steroids are also critical regulators of neuroendocrine pathways, where they can change gene expression through classical hormone pathways, primarily for maintaining sexual differentiation and the neural control of reproduction. Neurosteroid derivatives of progesterone or testosterone can affect the brain through allosteric modification of gamma-aminobutyric acid (GABA) receptors (Majewska et al. 1986, Wang et al. 2002, Locci and Pinna 2017). However, most of these studies have been performed in animal models. Mice are valuable human disease models due to their controlled conditions and genetics (Beresford-Jones et al. 2021); although their gut microbiota differs slightly, their similarity with humans is ~90% (Park and Im 2020). Functional capabilities and genetics are essential for the selection of mouse models for research.
As some studies have conflicting results, our research suggests that the gut microbiota may influence lifespan, possibly influenced by the gut–brain axis through neuroendocrine pathways. We demonstrated that the composition and function of gut microbiota can predict longevity without dietary intervention. We also identified sex-based differences in gut microbiota composition and function, uncovering metabolic pathway variations between males and females. This study highlights the microbial genetic predisposition to body mass and lifespan and offers insights into targeted interventions to improve overall health and well-being.
Materials and methods
Subjects
The study was carried out on 3-months-old mice (~90 days) of the Kghz:H (short-lived) line and the Kghz:L (long-lived) line (hereafter referred to as H and L, respectively), which were selected in opposite directions for body weight at weaning (at 21 days of age) by 148 generations. Totally, 24 mice were involved in this study, 12 mice from H line (six males and six females) and 12 mice from L line (six males and six females). The H-line animals are generally significantly larger than the L-line mice (Fig. 1.) and differ in body weight at day 90 (40.62 g H males; 31.98 g H females, 24.33 g L males, and 19.94 g L females). Observed changes in the body weight of selected animals must result from a proportional increase (in the H line) or decrease (in the L line) in the size of all organs and tissues without connection to body fat deposition (Wirth-Dzieçiołowska and Czumińska 2000) (Table S1). A tendency to increase the amount of adipose tissue develops in some individuals with age and is observed mainly in males of both lines. Moreover, these lines differ in behavior, length of reproduction period (20–23), and rate of aging (median in days) (510 H males, 490 H females, 615 L males, and 675 L females). Short-lived mice matured early (40 days), whereas L mice matured slowly (70 days) (Wirth-Dzieciolowska et al. 1997, 2005, Wirth-Dzieçiołowska and Czumińska 2000, Rosochacki et al. 2005).
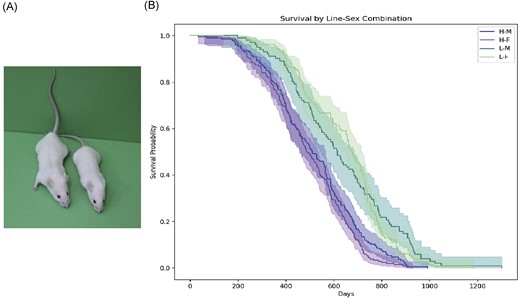
Mice lines Kghz involved in the study. Short-lived line mouse (left), long-lived line mouse (right).
The outbred lines were maintained in a conventional breeding run by the Department of Animal Genetics and Conservation, University of Life Sciences, Warsaw, Poland. Tested mice from both lines were housed in the same animal room under the following environmental conditions:temperature 20°C–24°C, relative humidity 45%–65%, and 12/12 h light/dark cycle. Animals had ad libitum access to water and a standard pellet diet (LABOFEED H; Morawski Pasze, Kcynia, Poland) containing 23% crude protein, 4.1% fat, and 50% carbohydrates.
Stool sample collection and DNA extraction
Stool samples were collected from 3-month-old randomly chosen animals from generation 148 (six males and six females from each line, a total of 24 animals). The number of animals was selected based on the E value calculation (E = total number of animals−total number of groups). Based on the freedom of calculation with ANOVA, 24 animals had an adequate sample size (20) (Charan and Kantharia 2013). All samples were collected on the same day using a noninvasive protocol. Each mouse was placed separately in a sterile empty plastic cage (Tecniplast, Italy) for 10–15 min. After spontaneous defecation, three to four fecal boli per individual were collected in sterile microtubes and stored immediately at −80°C until further processing. The samples were shipped to the laboratory for analysis of the dried ice. According to the manufacturer’s protocol, feces—150–200 mg were isolated using bead-beating lysis and column-based DNA extraction via Zymobiomics DNA/RNA Miniprep Kit (Zymo Research Scientific, Irvine, CA, USA). The DNA concentration was assessed using a Qubit fluorometer (Thermo Fisher Scientific, Waltham, MA, USA).
High-throughput 16S rRNA sequencing
16S rRNA V4 primer sets (Caporaso et al. 2011) were used for polymerase chain reaction (PCR) amplification of the 16S rRNA gene for partial sequencing of the gut metagenome. The V4 region of 16S rRNA was amplified with primers ligated with Illumina adapters, and the PCR products were verified by agarose gel electrophoresis and purified with Agencourt AMPure magnetic beads (Beckman Coulter, Brea, CA, USA). The obtained fragments were indexed using low-cycle PCR (Illumina Inc., San Diego, CA, USA) and purified again. Fluorometric quantification with Qubit 3.0 (Thermo Fisher Scientific Inc.) was performed, and the quality of DNA was determined using an Agilent 2100 Bioanalyzer (Agilent Technologies, Santa Clara, CA, USA). The final DNA libraries were analysed using the Illumina MiSeq platform (Illumina Inc.) with 2 × 300 bp reads.
High-throughput shot-gun sequencing
The DNA libraries were prepared and indexed using Nextera Kit (Illumina Inc.) from the input concentration 2 ng/µl. The preparation was performed according to manufacturer’s protocol. The DNA libraries were purified with Agencourt AMPure magnetic beads (Beckman Coulter). The quality was checked through Agilent Bioanalyzer (Agilent Technologies) and quantity was determined using a Qubit 3.0 (Thermo Fisher Scientific Inc.). The DNA libraries we sequenced using the Illumina NexSeq platform 2 × 150 bp (Illumina Inc.).
Lipid extraction and thin layer chromatography
Total lipids were extracted from about 100 mg lyophilized feces samples according to Kraus et al. (2015). Briefly, 5 ml of normal saline was added to the pooled samples of H and L groups of powdered feces and lipids were extracted with 5 ml of chloroform:methanol (2:1). After centrifugation at 1000 g for 10 min, the lower phase was dried under nitrogen stream and lipids were dissolved in chloroform:methanol (1:2) (VWR International, Radnor, PA, USA).
Lipids were analysed by thin layer chromatography (TLC) on TLC Silica Gel 60 plates (VWR International). The plates were washed with chloroform:methanol (1:1) and activated at 110°C before use. Lipids standards (cholesterol sulfate, cholesterol, Avanti Polar Lipids, AL, USA) and aliquot of lipid extracts corresponding to 5 mg of DCW mice feces were loaded on TLC plates by a Linomat 5 semiautomatic sample applicator (CAMAG Linomat 5, Muttenz, Switzerland). Lipids were separated by a two-step TLC solvent system according to Wertz and Downing (1988). The TLC plate was developed to half of the plate in the first mobile phase consisting of chloroform:methanol:acetic acid:water (40:10:1:1) and after drying in the second mobile phase of hexane:ether:acetic acid (70:30:1) to the top of the plate. Individual lipid spots were identified using lipid standards run on the same plate and visualized by dipping the TLC plate into charring solution consisting of 5.16 g MnCl2.4H2O (Slavus s.r.o., Bratislava, Slovakia), 465 ml of water, 500 ml of methanol and 33 ml of concentrated sulfuric acid (Slavus s.r.o.) with subsequent incubation at 130°C for 10 min. For semiquantitative analysis, the relative amount of cholesterol sulfate in individual lipid spots was determined via densitometry at 475 nm (CAMAG TLC Scanner 3, Muttenz, Switzerland).
MALDI TOF MS analysis
Lipid spots corresponding to cholesterol sulfate of mice feces samples were scraped from thin-layer chromatography with mass spectrometry (TLC–MS)-grade silica gel 60 F254 plates (Merck, Darmstadt, Germany). Lipids were extracted from silica with chloroform:methanol (2:1) and then analysed by matrix assisted laser desorption ionization-time of flight mass spectrometry (MALDI-TOF/MS) The samples were dissolved in chloroform:methanol solution (2:1) and 1 µl was mixed with 1 µl of matrix (norharmane, 10 mg/ml in chloroform:methanol solution) on the ground steel MALDI target plate (Bruker Daltonics, MA, USA). The mixture was then left to dry to facilitate crystallization. Subsequently, mass spectra (a minimum of 3000) were acquired in reflectron negative mode using the UltrafleXtreme II MALDI-TOF mass spectrometer (Bruker Daltonics), with red phosphorus clusters serving as the mass calibrant (PMID: 19714708 DOI:10.1002/rcm.4230). The data obtained were processed using Flex-Analysis v.3.4 software (Bruker Daltonics).
Data processing
Samples were analysed using a Qiime2 2022.8 pipeline (Bolyen et al. 2019). Quality control and feature table construction were performed using the DADA2 QIIME2 plugin (Callahan et al. 2016) with the following parameters:–p-trunc-len-f 200, –p-trunc-len-r 200, –p-trunc-q 2, and –p-max-ee-r 3. For taxonomic classification of the V4 region of 16S rRNA, we used the pretrained Silva 138 515F/806R classifier (Quast et al. 2013) running the q2-feature-classifier plugin. Alpha diversity was assessed using three different indices (Shannon index, Simpson index, and Chao1). Functional prediction of metagenomes was performed using the Tax4Fun2 pipeline freely available at https://github.com/ZihaoShu/Tax4Fun2 (Wemheuer et al. 2020) running the Ref99NR reference dataset (Quast et al. 2013). The reference datasets were generated as follows:we downloaded all complete genomes and all genomes with the status “chromosome” from NCBI RefSeq (accessed on 18 August 2018), resulting in 275 archaeal and 12 102 bacterial genomes. OTUs classified with the QIIME2 2022.8 pipeline (Bolyen et al. 2019) were used to create a sample-specific bacterial profile. These profiles were summarized based on Kyoto Encyclopedia of Genes and Genomes (KEGG) pathways after passing the default similarity threshold (97%) (Wemheuer et al. 2020).
The shot-gun sequencing data were processed using Galaxy web instance (Afgan et al. 2018). The raw data of were trimmed using Trimmomatic (Galaxy version 0.38.1) with parameters CROP 150, HEADCROP 15, SLIDING WINDOW 4 :20, and MINLEN 50 (Bolger et al. 2014). The quality was checked with the FastQC tool. Trimmed reads were then classified by Kraken version 2.1.1, with a minimum base quality of 20 and default settings (Lu et al. 2022). The database used was Standard plus protozoa and fungi (12 September 2022). Paired reads were used for de novo assembly of contigs using MEGAHIT v1.1.3, with a minimum contig length of 200 bp (Li et al. 2015). The contigs were then translated into protein sequences through the Prokka tool with default settings (Seemann 2014). BlastKOALA annotated genes (Kanehisa et al. 2016), and these data were then used for specific gene mapping through Geneious Prime (https://www.geneious.com/) tool Align/Annotate with Medium Sensitivity/Fast using Geneious Mapper. Fine-tuning was set to “Iterate 5 times.”
Statistical analysis
The sample normality was checked using the Shapiro–Wilk test for statistical data processing using SPSS (IBM Corp. Released 2012. IBM SPSS Statistics for Windows, Version 21.0. Armonk, NY: IBM Corp.). Nonparametric data were further analysed using the Kruskal–Wallis or Mann–Whitney test with established α = 0.05/α = 0.01 and LDA score 2.0 applied. These analyses were performed using LefSe (Segata et al. 2011) (P ≤ .05 with 2.0 threshold on the logarithmic LDA score) and heat tree (Foster et al. 2017), which used the default layout with P ≤ .05, while the input consisted of OTU table of relative abundances of OTUs and metadata file. The analysis was performed using the MicrobiomeAnalyst web-based platform (Lu et al. 2023). The data were analysed also using SPSS Kruskal–Wallis test and false discovery rate according to Benjamini–Hochberg procedure was calculated to correct for multiple comparisons. For bivariate correlation analysis, the Spearman rank correlation coefficient was calculated using a two-tailed test (P ≤ .05) to estimate possible links between body weight, bacterial genera, and predicted functions. Significant correlations were visualized using the Gephi software (Gephi version 0.10.1,). We used Force Atlas layout with 10 000 repulsion strength and the “Betweenness Centrality” parameter from the “Average Path Size” algorithm, which measures how often a node appears on the shortest paths between nodes in the network. A modularity algorithm is used for community colorization and detection. Box plots and bar charts were generated using GraphPad (GraphPad Prism version 8.0.0 for Windows; GraphPad Software, www.graphpad.com).
Random forest and machine learning
The machine learning library scikit-learn (v1.2.1) was used to construct the Random Forest classifier, with the groups used as labels and the proportions of abundances from the QIIME2 pipeline as features. The number of trees in the random forest was set to 100. Each tree was trained using default parameters. At each node, a random subset with the size of the square root of the number of features was sampled, and a feature from this subset that minimized the Gini coefficient of the partition was used as the decision rule of the node. The importance of features in the classifier was extracted using the default feature importance from scikit-learn and was used to estimate the discriminative power of the microbiome markers.
Results
Gut microbiota composition based on 16S rRNA gene
Six different phyla were identified, of which Bacteroidota (L—68%; H—65%) was the most abundant, followed by Bacillota (L—25%; H—31%) and Campylobacterota (L—1.3%; H—2.8%). Proteobacteria and Verrucomicrobiota with >1% abundance were detected only in the cohort of long-lived mice (Pseudomonadota—1.1%; Verrucomicrobiota—2%). Cyanobacteria (long-lived and short-lived) and Desulfobacterota (long-lived) were detected with relative abundances lower than 1% (Fig. 2). There was no significant difference in the gut microbiota composition between short-lived and long-lived mice at the phylum level. However, for the group of short-lived mice, a significant increase in Desulfobacterota (P < .05) was observed.
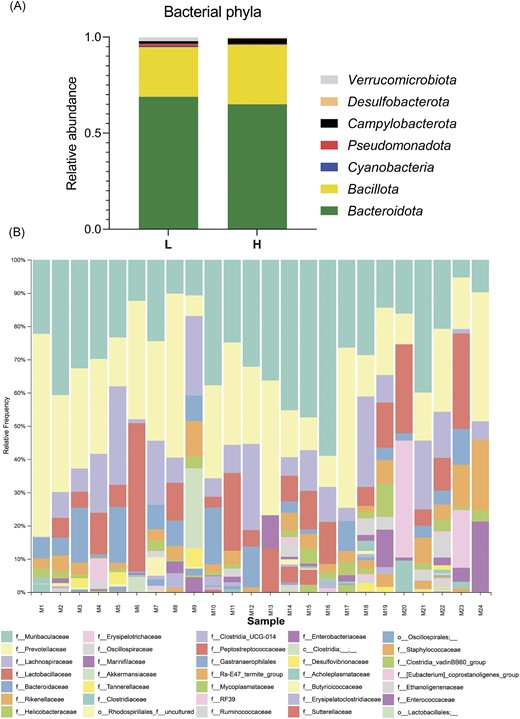
Prevotellaceae and Bacteroidaceae dominaed in long lived mice. (A)—Relative abundance of bacterial phyla detected in the gut microbiome of light (L) and heavy (H) mice group. (B)—Graphical visualization of bacterial composition represented by OTUs of gut microbiome at family level; 1–6—L males; 7–12—L females; 12–18—H males; and 19–24—H females.
A more detailed analysis revealed the presence of 27 families identified in the L group and 27 in the H group, of which Prevotellaceae was the dominant one in the L group (L—30%; H—21%), followed by Muribaculaceae dominant in the H group (L—25%; H—29%), Lachnospiraceae (L—13%; H—9%), Lactobacillaceae (L—9%; H—10%), Bacteroidaceae (L—8%, H—3%). Rikenellaceae (L—3%; H—5%), Marinifilaceae (4%), and Erysipelotrichaceae (4%) were detected at higher relative abundances in the H group (L group <1%). Other families were identified with a lower abundance (less than 2%) (Fig. 2).
Among all detected bacterial genera (60), Alloprevotella, unidentified Muribaculaceae, Prevotellaceae UCG-001, and Lactobacillus were the most abundant, each of them with >5% relative abundance within the group L. Similar dominant genera were also identified in group H; moreover, Odoribacter was included. Altogether, 79 species were identified (L—53; H—63). Species within the H group were often detected only selectively in some individuals, so higher intragroup diversity was observed (Table S2).
To understand the role of the gut microbiome underlying the aging processes, our study focused on changes stemmed from real body composition. Based on 16S V4 rRNA analysis, the gut microbiome diversity of mice did not differ significantly, between females and males either of the long-lived or of the short-lived group. Although a trend was observed in the increase in alpha diversity in long-lived individuals, no significant differences were defined by the Shannon index (L—4.3; H—4.7) (P = .6) (Fig. 3.), Simpson index (L—0.919; H—0.918) (P = 0.729) and Chao1 index (L—44.13; H—69.29) (P = .525).
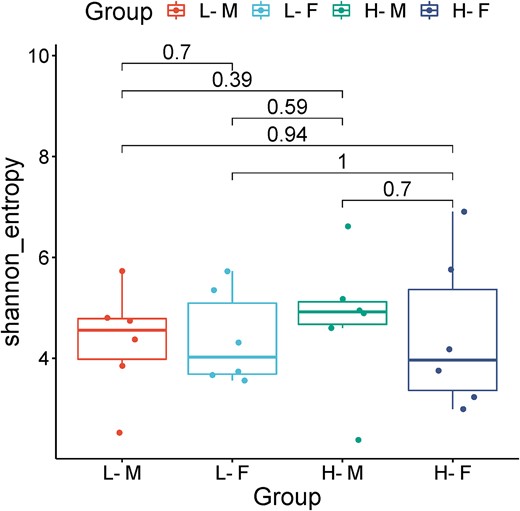
Alpha diversity of gut microbiota determined by Shannon diversity index. Group H-M—short-lived heavy males, L-M—long-lived light males, H-F—short-lived heavy females, and L-F—long-lived light females.
Gut microbiota is linked to body composition
Two-group Kruskal–Wallis test of gut microbiota composition between short-lived (H) and long-lived (L) mice at different taxonomic levels enabled the identification of significant changes observed at the family and genus taxonomic levels, including the bacterial family Desulfovibrionaceae. However, this effect was ameliorated by Clostridiaceae and Marinifilaceae, which were more abundant in the H group, and Bacteroidaceae in the L group. Glucose metabolism-associated Odoribacter and Clostridium sensu stricto 1 and Colidextribacter associated with antidepressive-like behavior (intake of sucrose) were genera with higher relative abundances in the H group (Duan et al. 2021, Huber-Ruano et al. 2022). At the same time, Alloprevotella and Bacteroides were more enriched in the L group (Fig. 4).

Gut microbiota is linked to body composition. Comparison of gut microbiota composition of heavy (H group) and light (L group) mice based on the partial 16S rRNA sequence (V4) analysis. Significant (P ≤ .05) differences in the relative abundance of bacterial taxa at different taxonomical levels are presented.
Comparison of gut microbiota composition between short-lived and long-lived groups
We evaluated sex-based differences between males and females within and between short-lived (H) and long-lived (L) groups of individuals. Based on V4 16S rRNA sequence analysis using Mann–Whitney statistical test, the gut microbiome of males with lower weight was richer in Alloprevotella than of the heavier ones. At the same time, higher abundance of Cyanobacteria, Vampirivibrionia, Gastranaerophilales, and Helicobacter ganmani was detected in L females and unidentified Odoribacter and Helicobacter typhlonius in H females (Fig. 5).
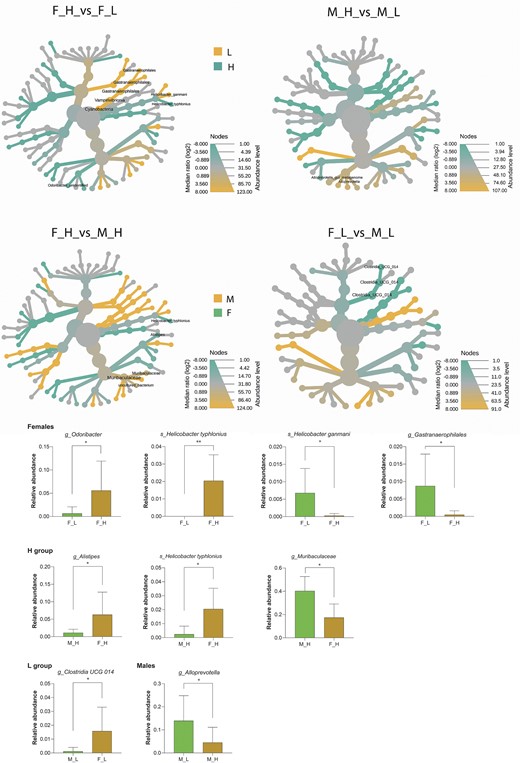
Sex-specific gut microbiome bacterial features within and between mice lines L and H. Significant (P ≤ .05) differences in gut microbiota composition at different taxonomical levels within the groups of heavy (F_H/M_H) and light (F_L/M_L) individuals between females (F) and males (M) and between L and H lines (F_H/F_L, M_H/M_L). Diferentially abundant bacteria were visualized using box-plots.
Comparison of gut microbiota composition between females and males within short-lived and long-lived groups
Mann–Whitney analysis of all taxonomic levels revealed that within the group of short-lived mice, Muribaculaceae was more abundant in females, and Alistipes and H. typhlonius were significantly more abundant in males. For L female mice, the abundance of Clostridia UCG-014 was higher than in the gut of their male counterparts (Fig. 5).
Gut microbiota is involved in longevity through the nervous system and lipid metabolism-associated pathways
The 16S rRNA-based bacterial function prediction showed several differentially altered metabolic pathways with the involvement of the gut bacteriome, such as the peroxisome proliferator-activated receptor (PPAR) signaling pathway or biosynthesis of unsaturated fatty acids included in lipid metabolism, all of which increased in the L group. Moreover, based on Two-group Kruskal–Wallis test, steroid hormone biosynthesis and lipoic acid metabolism were significantly more enriched in the L group. Among others, gut bacteria of long-lived mice were more involved in nervous system-associated pathways, including GABAergic synapses and glutamatergic synapses. In contrast, in the H group, a higher involvement of the gut microbiome in glycerophospholipid metabolism and cardiac muscle contraction-associated pathways was detected. Furthermore, higher activation of tryptophan and butanoate metabolism was observed in males than in females in the H group. Intergroup analysis revealed increased activation of the GABAergic synapse metabolic pathway in males from the L group compared to the H group (Fig. 6). The identified metabolic pathways are listed in Table S3.
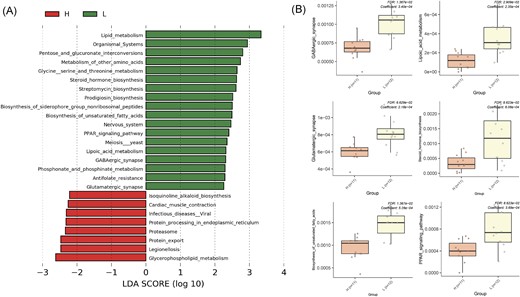
Involvement of gut microbiota in biological processes based on functional prediction. (A) Comparison of 16S rRNA-based functional prediction of heavy (H-group) and light (L-group) mice based on the partial 16S rRNA sequence (V4) analysis. Significant (P ≤ .01) differences in the relative abundance of bacterial taxa at different taxonomical levels are presented as log10FC (fold change). (B) The boxplots represent selected metabolic pathways.
Of the significantly different metabolic pathways, we emphasize the functional involvement of gut bacteria in long-lived individuals, without any preference for sex, in the increased metabolism of prodigiosin, a secondary metabolite (red substance) produced naturally by Streptomyces with antimicrobial and immunosuppressant activity.
The bacteria provide the host (long-lived mice) with amino acid synthesis (glycine, serine, and threonine metabolism). In contrast, glycerophospholipid metabolism, involving nitrogen and sulfur metabolism, was increased more in short-lived mice. Moreover, the ubiquinol–cytochrome c reductase iron–sulfur subunit is known to be involved in cardiac muscle contractions (also in nonalcoholic fatty liver disease, Alzheimer’s, and Parkinson’s disease), chaperonin GroEL (diabetes mellitus type I), and uracil-DNA glycosylase included in the primary immunodeficiency pathway. Long-lived mice had a higher predicted abundance of 3-oxoacyl-[acyl-carrier-protein] synthase I and 3-oxoacyl-[acyl-carrier protein] reductase involved in the fatty acid synthesis and biotin metabolism, NDP-hexose C3-ketoreductase/dTDP-4-oxo-2-deoxy-alpha-d-pentos-2-ene and dTDP-glucose 4,6-dehydratase involved in polyketide sugar unit biosynthesis, and 2,3-reductase phosphonoacetaldehyde hydrolase involved in phosphonate and phosphinate metabolism (Table S4).
According to KEGG pathways analysis, enzymes for glycine, serine, and threonine metabolism, GABAergic and glutamatergic synapses, fatty acid metabolism, biotin synthesis, and glyoxylate/dicarboxylate metabolism were associated with the metabolic pathways of Bacteroides and Alloprevotella significantly more abundant in L group. On the other hand, Odoribacter, which is significant for short-lived mice, possessed genes for the insulin signaling pathway polyketide sugar unit biosynthesis and glycine, serine, and threonine metabolism (Table S5).
Spearman correlation determined functional and taxonomical correlations to body weight
Spearman correlation analysis revealed 31 functions and six bacterial genera, including Odoribacter, Alloprevotella, Rombutsia, Clostridium sensu stricto 1, Rs-E47 termite group, and Bacteroides (Fig. 7). were correlated with body weight (P < .05). Four hundred and eighty-two correlations were observed (Table S6). between functions and bacterial genera were determined (Fig. 8). Functions included synthesis of antimicrobial peptides (streptomycin, neomycin, kanamycin, gentamicin, or prodigiosin), glutamatergic and GABAergic synapses, lipoic acid metabolism, biotin metabolism, adipocytokine signaling pathway, PPAR signaling pathway, insulin signaling pathway, type 1 diabetes mellitus, and primary immunodeficiency.

Possible interaction network between selected bacteria and metabolic functions. The network included selected bacterial genera and predicted metabolic functions correlated to body weight. Only significant (P ≤ .05) correlations based on Spearman rank correlation were visualized.
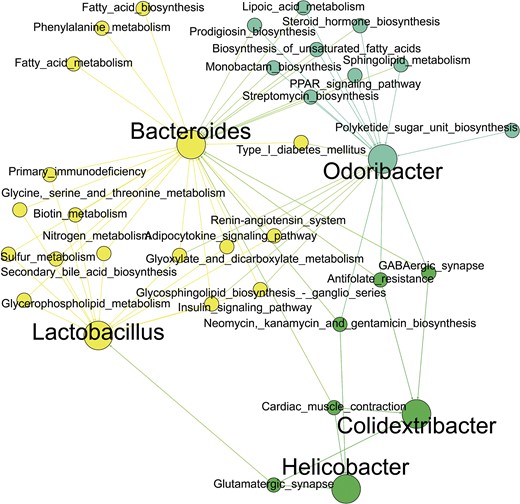
Interaction network of bacterial genera significantly correlated to the GABAergic and glutamatergic synapses. Visualization of significant correlations based on Spearman Rank coefficient (P ≤ .05).
GABAergic synapses (−0.589) and glutamatergic synapses (−0.487) were significantly negatively correlated with body weight. They play a crucial role in the gut–brain axis, whereas gut bacteria produce the neurotransmitters GABA and glutamate. The correlations between these synapses and bacterial genera were delimited, with Bacteroides (0.658), Odoribacter (−0.422), and Colidextribacter (−0.448) correlated with GABAergic synapses, while Lactobacillus (0.414), Helicobacter (−0 514), and Colidextribacter (−0.402) correlated with glutamatergic synapses. Thus, Bacteroides was correlated with fatty acid metabolism and biosynthesis. Furthermore, the PPAR signaling pathway and the biosynthesis of unsaturated fatty acids and lipoic acid metabolism were positively correlated with Bacteroides (0.868, 0.741, and 0.842) and negatively correlated with Odoribacter (−0.512; −0.533; and 0.544). Steroid hormone biosynthesis also correlated with Odoribacter (−0.548) and Bacteroides (0.918). The adipocytokine signaling pathway was strongly positively correlated with Bacteroides (0.876) and negatively correlated with Odoribacter (−0.528) and Lactobacillus (−0.462). Type I diabetes mellitus was correlated with both Bacteroides (0.780) and Odoribacter (−0.416), together with the insulin signaling pathway, which was also correlated with Lactobacillus (−0.580). Similarly, the insulin signaling pathway was strongly positively correlated with Bacteroides (0.758) and negatively correlated with Odoribacter (−0.457) (Fig. 8).
The most relevant bacterial features of long-lived individuals based on random forest machine learning prediction
The machine learning prediction-based significant biomarkers for estimating the difference between short-lived and long-lived mice were based on bacterial phyla and all bacterial taxa as joint predictors. Campylobacteria and Cyanobacteria were identified as the strongest bacterial phyla as predictors for short-lived male mice and Campylobacteria with Bacteroidota in female mice. Of the joint predictors of the long-lived individuals, Rikenella, Alloprevotella, and Muribaculaceae were found to be the strongest predictors in male mice, and Lactobacillus, Rikenellaceae RC9 gut group, Gastranaerophilales, Bacteroides, and Prevotellaceae UCG-001 in female mice. The machine learning analysis with bacterial phyla for males and bacterial genera for females, used as joint predictors, led to a ROC curve with an AUC = 0.96 for males and AUC = 1, for females, which represents a set of variables discriminating individuals between L and H groups. Both bacterial phyla and genera led to a ROC curve with an AUC of 1 for females. (Fig. 9).
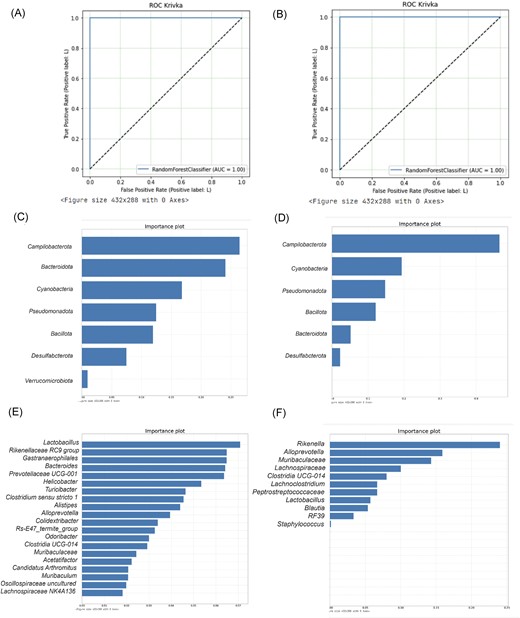
Selected features of gut microbiome identified by machine learning. ROC (receiver operating characteristic) curves (A and B) and importance plots (C, D, E, and F) with significant discriminators between L and H females (C, E), L and H males (D, F). AUC value, representing predictive power of biomarkers (bacterial phylum (C, D) and genus (E, F).
Bacteroides spp. community of the gut microbiome
In order to determine the potential involvement of Bacteroides spp. in longevity, we investigated the gut microbiome composition also through metagenomic analysis. The feces samples of mice were found rich in Bacteroides spp. represented by 31 species. Among them, Bacteroides caecimuris (H—2.26%; L—12.3%), Bacteroides sp. CBA7301 (H—0.93%; L—6.15%), Bacteroides uniformis (H—0.86%; L—1.14%), Bacteroides thetaiotaomicron (H—0.34%; L—1.14%), Bacteroides xylanisolvens (H—0.34%; L—1.52%), and Bacteroides fragilis (H—0.30%; L—0.59%) exhibited the highest relative abundance in L group. The total relative abundance of Bacteroides spp. was estimated to 8 ± 2% in H group and 29 ± 6% in L group. The statistical analysis revealed that eight of 31 Bacteroides species were significantly more abundant in L group including B. caecimuris, Bacteroides luhongzhouii, Bacteroides ovatus, Bacteroides sp. D2, Bacteroides sp. DH3716P, Bacteroides sp. M10, B. thetaiotaomicron, and B. xylanisolvens (P < .05) (Fig. 10).
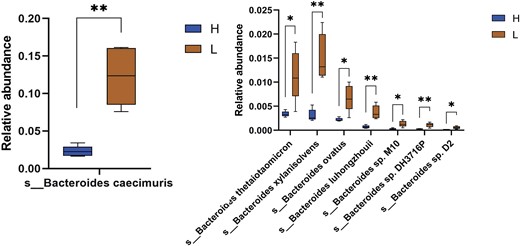
Graphical visualization of eight significantly differentially abundant Bacteroides species in the gut microbiome of H and L mice.
Furthermore, the potential involvement of Bacteroides spp. in longevity through lipid metabolism was determined. The analysis of the metagenome revealed genes involved in cholesterol sulfate conversion, especially sulfotransferase, in selected Bacteroides genomes, as well as several other genes involved in lipid metabolism including 3-oxoacyl-[acyl-carrier protein] reductase, 3-oxoacyl-[acyl-carrier protein] synthase I, enoyl-[acyl-carrier protein] reductase I and II, and long-chain acyl-CoA synthetase. We concentrated on genes identified in the functional prediction analysis as significantly discriminating between L and H groups. This approach allowed us to pinpoint key genetic factors driving the differences between the two groups. Furthermore, also selected genes identified in the prediction analysis (3-oxoacyl-[acyl-carrier protein] reductase, 3-oxoacyl-[acyl-carrier protein] synthase I), despite not being statistically significant, were still incorporated into the screening of bacterial genomes, because of their relevance. Increased sequencing coverage of selected single-copy genes associated with lipid metabolism was positively correlated with the relative abundance of Bacteroides spp. Moreover, the regression analysis confidently established a correlation (R-square 0.53–0.75, P = .001–.02) (Fig. 11.) between the presence of these genes and the relative abundance of the Bacteroides genus. Our findings strongly indicate a substantial variation in gene presence among the groups.
![The relative abundance of selected genes involved in lipid metabolism corelate with the relative abundance of Bacteroides spp. in gut microbiome of mice. Regression analysis identified significantly positive correlation between Bacteroides and sulfotransferase gene (P = .0018; Rsquare = .7246); 3-oxoacyl-[acyl-carrier protein] reductase (P = .0012; R-square = 0.7523) and 3-oxoacyl-[acyl carrier protein] synthase 1 (p = 0.0175; R-square = 0.5267). Each point represents a mouse individual of the L and H group.](https://oup.silverchair-cdn.com/oup/backfile/Content_public/Journal/femsec/100/11/10.1093_femsec_fiae135/6/m_fiae135fig11.jpeg?Expires=1750399676&Signature=V91stsK-aMTiI1qZbhR9kCPCh66vt0mFqpmZWiqdZPJbQaub6xG-4MapOKXzU9srg0n4JXe1qLjLP8J4iITyeOT-20kQiLcXo-1k~qovXZD-4KnIGbdUcjY8NTaefDZV0bEZ8etCUXmxzviuyx8xtSeplMot9HC6uDjPZ~5XaAe8hHf5D4T1Iaj6JnDaLmhZzEI-ZmOPlnr-60VEUJ~ePg5YHo1kH6IpSzx2jtW7-vqsYW7PayaVfNpxYfCmSJQFwXU5L51feMBy3-FHVyEH2b-5HgKxORF5y-97bQg9001COdJS9pZemhfIwqD5eAI1qWBgpLn47cafU2tVg1oTmA__&Key-Pair-Id=APKAIE5G5CRDK6RD3PGA)
The relative abundance of selected genes involved in lipid metabolism corelate with the relative abundance of Bacteroides spp. in gut microbiome of mice. Regression analysis identified significantly positive correlation between Bacteroides and sulfotransferase gene (P = .0018; Rsquare = .7246); 3-oxoacyl-[acyl-carrier protein] reductase (P = .0012; R-square = 0.7523) and 3-oxoacyl-[acyl carrier protein] synthase 1 (p = 0.0175; R-square = 0.5267). Each point represents a mouse individual of the L and H group.
We conducted an analysis of the sulfotransferase gene SULT in Bacteroides species to investigate its potential relevance in our study. Through multiple alignment analysis in silico, we observed significant similarity in the sulfotransferase gene sequences among various Bacteroides species. Subsequently, we extended our investigation to examine the presence of this gene in mice stool. Strikingly, our findings revealed distinct patterns. While in the gut microbiome of H-mice, B. uniformis was the dominant gene carrier; in four out of five L mice the gene was harbored by B. caecimuris I48 in combination with B.faecium, B.intestinalis, and B.uniformis, while in one individual only B.faecium was detected.
In addition, we expanded our investigation to encompass the wide-spread biosynthetic gene cluster comprising six genes within the Bacteroides species including adenylyl-sulfate kinase (cysC), sulfate adenylyltransferase subunit 1 and 2 (cysD and cysN), putative Na+/sulfate symporter, and 3′-phosphoadenosine 5′-phosphate phosphatase (cysQ). Enzymes encoded by this gene cluster participate in the synthesis of the donor molecule of sulfonate, adenosine 3′-phosphate-5′-phosphosulfate (PAPS). We have identified this gene cluster within the same bacterial species with prevailing B. caecimuris I48 in L-group of individuals, in H-group, this cluster was identified in B. uniformis or B.intestinalis. Notably, the presence of this gene cluster was consistently observed among all analysed samples, regardless of the individuals’ body composition.
Additionally, except of Bacteroides spp. also Parabacteroides distasonis, as the carrier of sulfotransferase gene and SULT gene cluster, was identified in three individuals belonging to the L-group.
Comparison of cholesterol sulfate level between short-lived and long-lived groups
Cholesterol sulfate level is increased in long-lived group of mice
It was shown that Bacteroides can convert cholesterol to cholesterol sulfate via the activity of sulfotransferases (Le et al. 2022). Cholesterol sulfate was proposed to be involved in development of various diseases including ulcerative colitis and atherosclerosis. The neuroprotective effect of cholesterol sulfate was determined in primary astrocytes, suggesting that cholesterol sulfate can have a protective role in neurodegenerative disorders associated with oxidative stress and aging (Prah et al. 2019). We hypothesized that high abundance of sulfotransferase genes in Bacteroides species can be related to longevity of L group mice through the increase synthesis of cholesterol sulfate. To support our hypothesis, we determined the level of cholesterol sulfate by TLC analysis in both L and H mice groups. In lipid extract of pooled samples of L mice stool we observed 1.7-fold elevated level of cholesterol sulfate compared to H group (Fig. 12). In addition, no significant changes were determined in the level of cholesterol between the two groups of mice.
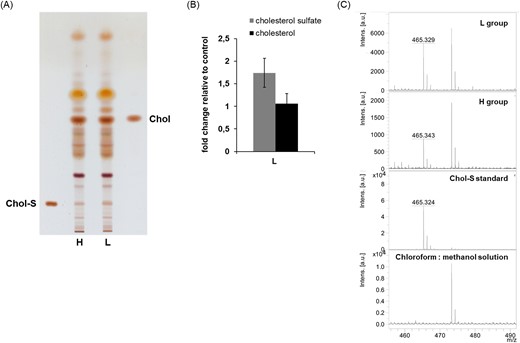
Analysis of cholesterol sulfate and cholesterol in mice feces lipid extracts. (A)—representative TLC analysis of lipid profile of short-lived individuals (H) - left side, and long-lived individuals (L) - right side, (B)—graph shows the fold change in cholesterol sulfate and cholesterol signal of L group relative to H group. TLC plates were scanned at 475 nm using CAMAG TLC scanner and relative quantification was performed using the WinCat software. Values represent the averages of five experiments ± SD. Abbreviations: Chol-S—cholesterol sulfate, Chol—cholesterol. (C)—Confirmation of cholesterol sulfate by negative ion mode MALDI-TOF/MS analysis. Lipid extracts from H and L groups were separated by TLC and lipid spots corresponding to cholesterol sulfate were scraped from silica gel plate. Lipids were extracted from silica with chloroform:methanol (2:1) and then analysed by MALDI-TOF/MS as described in the section “Material and methods.”
The identity of the cholesterol and cholesterol sulfate was verified by MALDI-TOF/MS (Fig. 12c). This result indicates that increased level of cholesterol sulfate through the metabolic activity of Bacteroides species in gut can play a significant role in longevity of L group mice. However, we cannot exclude other factors contributing to longevity of L group.
Discussion
The intestinal microbiome is an essential factor for the proper functioning of the body. However, we still do not know how its composition is shaped or how strongly it can change during an individual’s life. We also have only fragmentary knowledge of the groups of bacteria that influence host traits.
An increasing amount of data shows that the host genotype is one of the critical factors determining the composition of the intestinal microbiome (Bubier et al. 2021), and even minor genetic differences can significantly affect the intestinal microbial composition and functionality, and thus, the specific characteristics of the host (Siersbæk et al. 2020). However, environmental standardization used in breeding laboratory animals does not protect against differences in the microbiome composition of animals belonging to the same strain/stock from different suppliers (Unger et al. 2020).
Our research focuses on a particular model. The L and H mice came from the same parental stock (a cross of four inbred strains), and for 50 years (almost 150 generations), they were kept in the same animal facility, in the same microbiological unit, and under the same environmental conditions. Moreover, their reproduction was synchronized (breeding pairs of both lines were mated simultaneously, in the same number—50 pairs/generation, in the same breeding plan, avoiding inbreeding). The only factor that differentiated the two lines was the selection criterion. In the H line, the heaviest individuals are selected as parents of the next generation; in the L line, the lightest individuals are selected.
Selection lines with significantly different body weights were created as a result of this consistent breeding. However, it should be noted that “body weight” is a very capacious term. These changes may result from a proportional increase in the mass of various body elements and changes in the individual organs and tissues. In a common perception, weight gain is often associated with fat gain. In the described lines, a higher percentage of fat has been observed in animals from the L line (Wirth-Dzieciolowska et al. 1997), contrary to skeletal muscles and bones, whereas muscle mass is increased in animals from the H line (Rosochacki et al. 2005). Additionally, H mice grow faster and reach puberty earlier (Wirth-Dzieciolowska 1992, Wirth-Dzieciolowska et al. 1997).
The differentiation markers of the two groups of mice were body weight, lifespan, and timing of body maturation. There is an apparent connection between these three markers in terms of growth and sex hormone levels. Except for the genetic differences occurring in the tested lines, the role of the gut microbiota in these mechanisms may be significant. Some studies have shown a bilateral relationship between gut microbiota and sex hormone levels. Compared to several, or relatively few, significantly different bacterial OTUs described as potential markers of the gut microbiota, our results correlate with increasing evidence of the importance of functional bacterial profiles. Notably, the balance between steroids and nonsteroids is strongly mediated by hydroxysteroid dehydrogenases (HSD) (Blum and Maser 2003). The prominent bacterial phyla that produce HSD enzymes are Actinobacteria, Proteobacteria, and Bacillota. Although the roles of gut bacteria and fungi in steroid hormone degradation are known, to our knowledge, steroid hormone biosynthesis by gut bacteria has yet to be adequately described.
The main question is whether these differences are also visible in the microbiomes of the tested animals. It should be emphasized that no alteration in gut microbiota composition was found in any of the studied groups of animals. The gut of H mice contained more taxa of microorganisms than that of L mice. However, increased richness is likely conducive to a more effective acquisition of nutrients from the food supplied, which, in the case of H mice, secures their needs during the period of intensive growth and development. Other studies have indicated that increasing microbiome richness may lead to changes in metabolism and weight gain (Kulecka et al. 2016). Nevertheless, to the best of our knowledge, no study has described the diversity of the gut bacteriome as a critical regulator of healthy aging or a biomarker of longevity. This indicates that the flexibility and stability of the gut microbiota could be a biomarker of longevity (Badal et al. 2020).
Biomarker identification is a primary goal of translational medicine studies. Early identification of biomarkers from omics data can help in the early diagnosis of complex diseases or stratification of patients. Machine learning was used to determine the bacterial biomarkers. The most potent biomarker discriminating short-lived and long-lived females was Lactobacillus, which belongs to the group of lactic acid bacteria (LAB). The significant role of LAB has been described in the context of prolonging the lifespan in other animal models, as well as in mice (Ikeda et al. 2007, Nakagawa et al. 2016, Kumar et al. 2022).
Moreover, some strains of Lactobacillus spp. have been studied as potential probiotics for lifespan improvement (Nakagawa et al. 2016, Kumar et al. 2022). The mechanism of action includes antioxidant activity of some Lactobacillus strains. In the C. elegans model, prolonged activity through the regulation of SKN-1, a known regulator of mechanisms involved in detoxification and protection against oxidative stress (Ikeda et al. 2007) was observed. ROS can activate SKN-1 through p38 MAPK signaling. SKN-1 is phosphorylated and translocated to the nuclei of intestinal cells, where it activates the genes responsible for detoxification (Inoue et al. 2005). Lactobacillus, as well as Rikenellaceae RC9 gut group, are short-chain fatty acids (SCFA) producing bacteria. SCFA are known to regulate redox homeostasis (Daschner et al. 2017) and have an anti-inflammatory potential. They can upregulate the expression of the anti-inflammatory cytokine IL-10 and influence the expression of TNF-α (Wang et al. 2022). SCFA are substrates for colonocytes and help improve gut barrier stability and mucus production (Silva et al. 2020).
Muribaculaceae was strongly correlated with propionate levels and was determined to be a predictor of SCFA concentrations (Smith et al. 2019). Gastranaerophilales are poorly studied bacteria, but they are known to produce vitamins B and K (Di Rienzi et al. 2013, Monchamp et al. 2019) related to slower aging (Mikkelsen and Apostolopoulos 2018, Simes et al. 2019). Recent studies have attempted to identify dietary fiber nutritional longevity patterns, and Lactobacillus has been positively correlated with dietary fiber intake. However, whether the abundance of Bacteroides increases or decreases with dietary fiber intake (Shi et al. 2022) is still unclear.
The main bacterial genera associated with weight were Bacteroides, Odoribacter, Clostridium sensu stricto 1, and Colidextribacter. While all of them belong to the core gut microbiome of healthy mice and SCFA-producing microorganisms, according to the literature, they have all been associated with obesity. In our study, Bacteroides and Odoribacter were correlated with insulin metabolism and GABAergic and glutamatergic synapse pathways. While the Rs-E47 termite group (c_Bacteroidia) and Romboutsia (c_Clostridia) positively correlated with body weight, Bacteroidia also showed a positive correlation with longevity, together with members of Clostridia (Kato et al. 2018), which metabolize estrogen, suggesting their impact on body maturation (Yuan et al. 2020).
Prediction of the functional role of gut microbiota revealed impressive involvement of gut bacteria in lipid metabolism, represented by a bundle of metabolic pathways correlated with aging and longevity. It includes lipoic acid and unsaturated fatty acid biosynthesis, which are significant biomarkers in long-lived mice. The biosynthesis of fatty acids is one of the pathways of lipid metabolism that can be strongly affected by bacterial metabolism. Deficiency in the triglyceride synthesis enzyme acyl‐CoA or treatment with steroid 17‐α‐estradiol was associated with a higher median age in Mus musculus (Johnson and Stolzing 2019). In another study, monounsaturated or polyunsaturated fatty acid intake was found to increase the lifespan of animal models (Honda et al. 2011). In contrast, α-lipoic acid is described as a regulator of lipid metabolism via the deacetylase sirtuin 1 (Chen et al. 2012). Gastranaerophilales and Cyanobacteriaceae are commonly associated with lipid metabolism in blood and white adipose tissue (Gao et al. 2020). In our study, the predicted function of the gut microbiota, the biosynthesis of unsaturated fatty acids, was significantly associated with body weight, which could be a possible mechanism by which the gut microbiota influences and prolongs the lifespan of L mice. Collino et al. (2013) showed that complex lipid remodeling is a crucial regulator of processes related to longevity in humans, where markers of lipid peroxidation are decreased as a result of an antioxidative response. Age-related changes in lipid metabolism involve ectopic lipid accumulation induced by increased fatty acid uptake and de novo lipogenesis, and decreased fatty acid oxidation and lipid catabolism, leading to lipotoxicity and accelerated aging.
Ren et al. (2021) also confirmed that predicted functions related to SCFA and amino acid metabolism were higher in long-lived subjects. The vital producer of acetate, an SCFAs produced by bacteria, is Bacteroides, a prominent bacterial genus in the gut. Acetate is a product of saccharolytic metabolism (Garrett and Onderdonk 2015) through the fermentation of dietary fibers and complex carbohydrates. It has gained attention for its potential impact on various aspects of host physiology, especially cholesterol metabolism, where acetate has been shown to influence hepatic lipid metabolism and can serve as a substrate for de novo lipogenesis (Zambell et al. 2003). Otherwise, Bacteroides spp. was described as a bacterial species that helps regulate cholesterol levels in the blood. Simultaneously, they can metabolize it to cholesterol sulfate and consequently reduce cholesterol absorption in the intestine (Kenny et al. 2020). Cholesterol sulfate is used as a precursor for sulfated adrenal steroids, resulting in sulfated steroid hormones. These hormones, both found in males and females, such as dehydroepiandrosterone sulfate (DHEAS) or estrone-3-sulfate, which represent long-lasting reservoirs of estrone or estradiol (Rezvanpour and Don-Wauchope 2017) are considered inactive hormones, but they cannot activate classical steroid receptors (Geyer et al. 2017). During aging, the concentration of DHEA decreases, making it a reliable endocrine marker of aging (Urbanski et al. 2013). In our study, the gut microbiome of long-lived mice was predicted to have a significantly higher involvement in steroid hormone biosynthesis and lipid metabolism, which is in accordance with previous studies. Modified hormones can be transferred into different target cells through, for example, sodium-dependent organic anion transporter, and after steroid sulfatase is provided, hydrolysis can contribute to a steroid hormone response regulation, suggesting the ability of the Bacteroides to metabolize cholesterol while producing steroid hormone precursors and consequently altered or delayed maturation of the host. Neurosteroid hormones, including sulfated steroids, pregnane, and androstane, can penetrate the blood–brain barrier and influence brain activity through interaction with ligand-gated ion channels or other receptors, although they have therapeutic potential in several brain disorders (Grube et al. 2018). Sulfated steroids are GABA neurotransmitter antagonists (Seljeset et al. 2015). DHEAS and estrogen sulfate can bind GABA-A receptors in the hippocampus and synchronize the activity of different hippocampal regions (Urbanski et al. 2013). The functioning of DHEA in the brain is supported by the contribution of astrocytes, oligodendrocytes, and neurons modulating N-methyl-d-aspartate receptors, enhancement of memory and sleep, and decreased anxiety (Yen 2001).
Adrenal hyperplasia, observed in H mice, is a group of autosomal recessive disorders that lead to cortisol synthesis enzyme deficiency. In some cases, the individuals are unable to produce aldosterone. Consequently, overproduction of androgens, such as testosterone and 17-hydroxyprogesterone, occurs. Another intriguing aspect is the role of gut microbiota, which can adapt to new conditions and is inherited. Throughout the mouse line selection, the gut microbiota of the L mice adapted to the new conditions and changes and led to an unintended indirect influence on steroid hormone synthesis through gut bacteria metabolites, with a potential effect on physiological processes within the host body, including the endocrine system, while the gut microbiota has proven to have an impact on the hypothalamic-pituitary-adrenal (HPA) axis (Frankiensztajn et al. 2020). Although there is evidence showing the local absence of 17α-hydroxylase cytochrome P450 (P450c17) in the adrenal glands of adult rodents, which is necessary for DHEA synthesis from its precursor pregnenolone, the enzyme is still expressed in other body parts of adult animals, including the brain and gonads (Keeney et al. 1995, Cascio et al. 2000). The presence of P450c17 in the gut suggests its potential role in influencing DHEA synthesis within the gastrointestinal tract, supported by higher enzyme synthesis in the duodenum, followed by the stomach, colon, and esophagus (Dalla Valle et al. 1995). Furthermore, gut bacteria can influence steroid hormone metabolism through enzymes such as HSD, which is involved in active and inactive steroid balance regulation (García-Gómez et al. 2013).
It is the link with the host’s genotype, where gut microbiota could not be associated with steroid hormone synthesis in the H mice when it was genetically determined that their levels were significantly different. Whether the gut microbiota can affect the host body, according to the previous theory, is part of upcoming research. However, experimental data from animal studies indicate that the gut microbiota can also act as an endocrine organ that is sensitive to physiological changes (Bermon et al. 2015).
The gut–brain axis represents a dynamic bidirectional communication network between the gut and the central nervous system, and emerging evidence suggests that the gut microbiota plays a pivotal role in this axis, making it a potential therapeutic target for various neurological and psychological disorders, as well as aging. Key functional players within the gut microbiota include Lactobacillus and Bacteroides genera, which are known for their ability to produce GABA (Yunes et al. 2016), a neurotransmitter with far-reaching implications for brain function. It is an amino acid with a primary function as an inhibitory neurotransmitter, which reduces neuronal excitability by inhibiting nerve transmission (Allen et al. 2023) and is known to control anxiety, fear, and stress. GABA production by these bacteria is facilitated through the decarboxylation of glutamate, using glutamate decarboxylase, and influences gut–brain signalling. Both neurotransmitters are crucial for modulating longevity and impacting learning, memory, and mood. GABA is a well-known inhibitory neurotransmitter produced by gut microbiota. GABA associated with calorie restriction helps improve lipid metabolism, mitochondrial biogenesis, and insulin sensitivity (Rathwa et al. 2022). GABA can influence appetite and food intake by modulating hypothalamic circuits and GABA signaling pathways (Delgado 2013).
GABAergic neurotransmission has also been implicated in lipid metabolism. At the same time, GABA receptors are present in adipose tissue (Ikegami et al. 2018) and play a role in the differentiation of adipocytes through an increase in the inflow of Ca2+ (Kim and Yoon 2023), which is in accordance with our previous findings of a higher abundance of adipose tissues detected in lean long-lived mice than in short-lived ones. Its implications on lifespan and longevity were experimentally confirmed by its interventions, followed by extending lifespan in nematodes, following the fact that GABA gradually decreases during adulthood (Porges et al. 2021). This is one of the potential mechanisms involved in stress resistance and regulation of energy metabolism. The gut microbiome can produce GABA, which is an integral part of the gut–brain axis. Changes in the microbial composition could lead to mood swings and changes in the stress response (Strandwitz et al. 2018). Both host and gut microbiota-derived GABA can influence appetite and food intake (Han et al. 2021), and host nutrient signaling pathways can benefit an individual’s lifespan through the gut microbiota. Furthermore, since dysbiosis can lead to chronic inflammation and age-related degenerative pathologies, disturbance of signaling pathways, including gut microbiota communication, can affect health and lifespan (Mikkelsen and Apostolopoulos 2018).
On the other hand, it is well-known that gut microbiota is a complex ecosystem, and not all interactions are beneficial for the host. Odoribacter, a member of the microbial consortium, is negatively correlated with GABAergic synapses and GABA receptors. This correlation is particularly noteworthy in the context of the expression of the GABA receptor alpha within the prefrontal cortex (Yao et al. 2022), a region implicated in cognitive and emotional regulation. This intricate microbial involvement underscores the complexity of the gut–brain axis. It is also essential to note that Bacteroides species have been associated with depression-like behavior modulation through differential alterations of tryptophan pathway metabolites and neurotransmitters (Zhang et al. 2022). Notably, B. fragilis, Bacteroides caccae, and B. uniformis have been associated with increased levels of GABA in the intestine, while concurrently, hippocampal GABA concentrations were significantly decreased (Zhang et al. 2022).
Additionally, B. ovatus colonization has been linked to increased intestinal GABA concentrations and reduced tryptophan levels (Horvath et al. 2022). A previous study reported higher anxiety and poorer spatial learning in L mice (Wirth-Dzieçiołowska et al. 2005). These behavioral alterations underscore the potential impact of microbial interactions, such as those involving Odoribacter and select Bacteroides species, on the neurobehavioral tapestry of the host.
The nematode C. elegans is one of the most extensively studied model organisms in longevity research. Notably, recent investigations have highlighted GABA as a pivotal regulator of lifespan in C. elegans, shedding light on the intricate mechanisms underpinning aging processes (Chun et al. 2015). A study by Wu et al. (2022) showed that metabotropic GABA receptors are required for the antiaging functions of GABA in neurons, and activation of GABA receptors exerts a protective effect against neuronal aging, accomplished through the inhibition of the PLCβ-PKC pathway. Furthermore, it has become increasingly apparent that maintaining cognitive function and attaining exceptional longevity are intricately linked to factors within the glutamatergic synapse and precise modulation of glutamate signaling pathways (Cox et al. 2022). Specific microbial taxa within the gut microbiota, including Bacteroides, Parabacteroides, and Escherichia species, are the primary GABA producers (Strandwitz et al. 2018). Intriguingly, these findings dovetail seamlessly with our research, which underscores the compelling associations between Parabacteroides and Escherichia/Shigella, as well as Ruminococcus, in the context of GABA. Moreover, our investigation detected a connection between these microorganisms and sphingolipid metabolism, aging, and longevity of cellular processes.
We suggest that the observed changes in the microbiome of animals from the tested lines are an indirect result of breeding work and bidirectional selection that changed the body weight of the animals. We believe that the microbiome should be treated as a characteristic feature of a given breeding group (the same as, for example, size or susceptibility to certain diseases) because, like other traits, it is inherited from generation to generation and coevolves with the host (Wei et al. 2022); however, as our research shows, it can also be subject to selection.
Beyond the realm of body weight, the impact of gut microbiota extends to biological processes that encompass behavior, lifespan, reproduction, and susceptibility to specific diseases in the subject organisms (Wirth-Dzieçiołowska and Czumińska 2000, Wirth-Dzieçiołowska et al. 2005). Therefore, the multiplicity of the biological processes with which different bacterial taxa are correlated is not surprising. However, it should be noted that the detected microorganisms operate in close concert with the host genome, and their actions potentially yield synergistic or antagonistic effects that are further susceptible to modification by various physiological factors, such as hormonal fluctuations or environmental variables, including stress. Their detailed effects should be carefully investigated in both females and males, given the gender differences observed in this study. Despite promising results of our investigation, it is important to admit limitations of the study including one timepoint analysis as well as possibility of the overfitting in random forest analysis. The gut microbiota, with its functional constituents such as Lactobacillus and Bacteroides, has emerged as a promising frontier for therapeutic interventions targeting the gut–brain axis. Their roles in GABA production, SCFA generation, and intricate connections with aging-related processes underscore the multifaceted roles of the microbiome in brain health and metabolic regulation.
Acknowledgements
We would like to thank Peter Barath (Institute of Chemistry, Slovak Academy of Sciences) for MALDI-TOF/MS analyses. We confirm that the study has received approval from the Ethics Committee of Warsaw University of Life Sciences, namely, the II—local Ethics Committee for Animal Experiments. The study follows the principles of the Declaration of Helsinki.
Author contributions
Sára Šardzíková (Formal analysis, Investigation, Visualization, Writing – original draft, Writing – review & editing), Marta Gajewska (Conceptualization, Formal analysis, Investigation, Methodology, Writing – review & editing), Norbert Gałka (Investigation, Writing – review & editing), Matúš Štefánek (Methodology), Andrej Baláž (Software), Martina Garaiová (Investigation, Methodology, Writing – original draft), Roman Holič (Investigation, Methodology, Writing – original draft), Wiesław Świderek (Conceptualization, Formal analysis, Funding acquisition, Project administration, Resources, Supervision, Validation, Writing – review & editing), and Katarína Šoltys (Conceptualization, Data curation, Formal analysis, Funding acquisition, Investigation, Methodology, Project administration, Resources, Software, Supervision, Visualization, Writing – original draft, Writing – review & editing)
Conflict of interest
None declared.
Funding
None declared.
Data Availability
The datasets generated and analysed during the current study are available in the NCBI SRA database under BioProject number PRJNA932774.