-
PDF
- Split View
-
Views
-
Cite
Cite
Neeraj Mediratta, John Chalmers, Mark Pullan, James McShane, Mathew Shaw, Michael Poullis, In-hospital mortality and long-term survival after coronary artery bypass surgery in young patients, European Journal of Cardio-Thoracic Surgery, Volume 43, Issue 5, May 2013, Pages 1014–1021, https://doi.org/10.1093/ejcts/ezs459
- Share Icon Share
Abstract
Coronary artery bypass graft (CABG) is performed for symptomatic and prognostic reasons. We aimed to determine the factors that contribute to in-hospital mortality and long-term survival in young patients (aged less than 65) undergoing CABG.
A prospective database was retrospectively analysed and cross-correlated with the United Kingdom's Strategic Tracing Service to evaluate survival in patients under the age of 65, following isolated primary CABG. Univariate-, multivariate logistic with Cox regression- and neural network analyses were performed.
Patients under the age of 65, who had undergone isolated CABG between April 1997 and March 2010 were studied;n = 5967. In-hospital mortality was 1.1% and long-termmortality was 13.5%; median follow-up 7.9 years. Multivariate analysis demonstrated that atrial fibrillation, ‘urgent’ operation status, postoperative creatinine kinase (CKMB), moderate or poor left ventricular (LV) function, and female sex were significant factors predicting in-hospital mortality. Cox regression demonstrated that age, diabetes (oral and insulin controlled), moderate and poor LV function, cerebrovascular disease, dialysis, left internal mammary artery (LIMA) usage, postoperative CKMB, atrial fibrillation, ‘urgent’ operation status, and peripheral vascular disease were significant factors determining long-term survival. Radial artery use, off-pump surgery, composite arterial grating and graft number had no effect on in-hospital mortality or long-term survival. Neural network analysis confirmed the factors identified by logistic and Cox multivariate analysis.
The risk factors for in-hospital mortality in patients under the age of 65 include postoperative CKMB, urgent operation status, LV function, female sex and atrial fibrillation. Significant factors determining long-term survival in the under-65 age group include age, atrial fibrillation, diabetes (diet and insulin controlled), LV function, cerebrovascular disease, dialysis, LIMA usage, ‘urgent’ operation status, CKMB and peripheral vascular disease.
INTRODUCTION
Risk prediction in cardiac surgery initially focussed on in-hospital mortality [1–3]. Subsequently, prediction of long-term survival became of interest as the debate between angioplasty and stenting intensified [4, 5].
Previous work has utilized the EuroSCORE as a possible predictor of long-term survival, despite its derivation being based on in-hospital operative mortality [6, 7]. This work was in patients of all ages. The American Heart Association (AHA) recently published a model for long-term survival in patients over the age of 65 [8].
Young patients potentially have the most to gain in terms of possible increased life expectancy after coronary artery bypass graft (CABG). We sought to investigate the role of EuroSCORE as a prognostic factor—with regard to survival after primary coronary artery bypass surgery—in patients under the age of 65.
METHODS
Local institutional review board approval was granted for this study.
Database
We utilized a prospective institutional cardiac surgery database, n = 18 706, validated by the hospital's data analysis department and accredited by the Society of Cardiothoracic Surgeons of Great Britain and Ireland (SCTS), in conjunction with the Strategic Tracing Service for long-term follow-up, which exists under the United Kingdom's National Health Service (NHS), as has previously been described [9].
Patients
Only patients under the age of 65, who had undergone isolated CABG between 1 April 1997 and 31 March 2010, were included; n = 6343.
Benchmarking
We benchmarked our in-hospital mortality figures against the United Kingdom (UK) national results (http://www.scts.org).
Univariate analysis
The characteristics of the patients in this study are shown in Table 1. The distribution of logistic EuroSCOREs for our population is shown in Fig. 1a; those with a EuroSCORE of less than 10 in Fig. 1b and the survival of our study population is shown in Fig. 1c. The Kaplan-Meier survival curves for patients, broken down by EuroSCORE, are shown in Figs 2a and -b. Discrimination ability of EuroSCORE was assessed by the Somers' Dxy test. For comparative purposes, we calculated the survival of an age- and sex-matched general population, based on survival figures from the National Office of Population and Statistics in the UK.
. | Data n = 5967 . |
---|---|
Preoperative | |
Age (Years) | 57.2 (45.6–64.6) |
Female (%) | 859 (14.4) |
BMI (Kg/m2) | 29.0 (22.6–37.1) |
Heart failure (%) | 6 (0.10) |
Critical pre-op state (%) | 18 (0.3) |
Previous CVA (%) | 18 (0.3) |
Diabetes (%) | 2136 (35.8) |
Pre-op dialysis (%) | 36 (0.6) |
Previous MI (%) | 2888 (48.4) |
PVD (%) | 680 (11.4) |
Hypertension (%) | 3341 (56) |
Pre-op IABP (%) | 48 (0.8) |
Ejection Fraction | |
Good (%) | 3706 (62.1) |
Moderate (%) | 1772 (29.7) |
Poor (%) | 489 (8.2) |
Previous PCI (%) | 459 (7.7) |
Status | |
Elective (%) | 4994 (83.7) |
Urgent (%) | 901 (15.1) |
Emergency (%) | 72 (1.2) |
Log. EuroSCORE | 2.4 (0.9–6.4) |
Operative | |
LIMA (%) | 5555 (93.1) |
No. of grafts | 3.3 (2.0–5.0) |
Off-pump | |
Cases (%) | 2156 (36.1) |
No. of grafts | 2.9 (1.1–4.9) |
Composite arterial (LIMA – Radial) (%) | 1334 (62) |
LIMA and SVG | 822 (38) |
On-pump | |
Cases (%) | 3811 (63.9) |
No. of grafts | 3.5 (1.6–5.5) |
LIMA ±SVG | 3052 (80) |
LIMA, radial ±SVG | 759 (20) |
CPB time (min) | 109 (61–165) |
Postoperative | |
CKMB | 29.1 (0 to 108) |
Perioperative MI | 266 (4.5) |
Stroke (%) | |
Total | 50 (0.8) |
Permanent | 30 (0.48) |
Temporary | 20 (0.32) |
Re-operation for bleeding | 228 (3.8) |
ICU LOS (Days) | 1.7 (1–5) |
Hospital LOS (Days) | 8.4 (5–15) |
Median follow-up (Days) | 2871 |
In-hospital mortality (%) | 66 (1.1) |
Study period mortality (%) | 806 (13.5) |
. | Data n = 5967 . |
---|---|
Preoperative | |
Age (Years) | 57.2 (45.6–64.6) |
Female (%) | 859 (14.4) |
BMI (Kg/m2) | 29.0 (22.6–37.1) |
Heart failure (%) | 6 (0.10) |
Critical pre-op state (%) | 18 (0.3) |
Previous CVA (%) | 18 (0.3) |
Diabetes (%) | 2136 (35.8) |
Pre-op dialysis (%) | 36 (0.6) |
Previous MI (%) | 2888 (48.4) |
PVD (%) | 680 (11.4) |
Hypertension (%) | 3341 (56) |
Pre-op IABP (%) | 48 (0.8) |
Ejection Fraction | |
Good (%) | 3706 (62.1) |
Moderate (%) | 1772 (29.7) |
Poor (%) | 489 (8.2) |
Previous PCI (%) | 459 (7.7) |
Status | |
Elective (%) | 4994 (83.7) |
Urgent (%) | 901 (15.1) |
Emergency (%) | 72 (1.2) |
Log. EuroSCORE | 2.4 (0.9–6.4) |
Operative | |
LIMA (%) | 5555 (93.1) |
No. of grafts | 3.3 (2.0–5.0) |
Off-pump | |
Cases (%) | 2156 (36.1) |
No. of grafts | 2.9 (1.1–4.9) |
Composite arterial (LIMA – Radial) (%) | 1334 (62) |
LIMA and SVG | 822 (38) |
On-pump | |
Cases (%) | 3811 (63.9) |
No. of grafts | 3.5 (1.6–5.5) |
LIMA ±SVG | 3052 (80) |
LIMA, radial ±SVG | 759 (20) |
CPB time (min) | 109 (61–165) |
Postoperative | |
CKMB | 29.1 (0 to 108) |
Perioperative MI | 266 (4.5) |
Stroke (%) | |
Total | 50 (0.8) |
Permanent | 30 (0.48) |
Temporary | 20 (0.32) |
Re-operation for bleeding | 228 (3.8) |
ICU LOS (Days) | 1.7 (1–5) |
Hospital LOS (Days) | 8.4 (5–15) |
Median follow-up (Days) | 2871 |
In-hospital mortality (%) | 66 (1.1) |
Study period mortality (%) | 806 (13.5) |
Continuous variables are shown as mean with 5 to 95% range shown in brackets. Categorical variables are shown as a percentage. BMI: Body mass index; CVA: cerebral vascular accident; MI: myocardial infarction; PVD: peripheral vascular disease; IABP: intra aortic balloon pump; PCI: percutaneous coronary intervention; LIMA: left internal mammary artery; CPB: cardiopulmonary bypass; CKMB: creatinine kinase myocardial isoenzyme; ICU: intensive care unit; LOS: length of stay; SVG: saphenous vein graft. Perioperative MI, based on a day one CKMB > 110.
. | Data n = 5967 . |
---|---|
Preoperative | |
Age (Years) | 57.2 (45.6–64.6) |
Female (%) | 859 (14.4) |
BMI (Kg/m2) | 29.0 (22.6–37.1) |
Heart failure (%) | 6 (0.10) |
Critical pre-op state (%) | 18 (0.3) |
Previous CVA (%) | 18 (0.3) |
Diabetes (%) | 2136 (35.8) |
Pre-op dialysis (%) | 36 (0.6) |
Previous MI (%) | 2888 (48.4) |
PVD (%) | 680 (11.4) |
Hypertension (%) | 3341 (56) |
Pre-op IABP (%) | 48 (0.8) |
Ejection Fraction | |
Good (%) | 3706 (62.1) |
Moderate (%) | 1772 (29.7) |
Poor (%) | 489 (8.2) |
Previous PCI (%) | 459 (7.7) |
Status | |
Elective (%) | 4994 (83.7) |
Urgent (%) | 901 (15.1) |
Emergency (%) | 72 (1.2) |
Log. EuroSCORE | 2.4 (0.9–6.4) |
Operative | |
LIMA (%) | 5555 (93.1) |
No. of grafts | 3.3 (2.0–5.0) |
Off-pump | |
Cases (%) | 2156 (36.1) |
No. of grafts | 2.9 (1.1–4.9) |
Composite arterial (LIMA – Radial) (%) | 1334 (62) |
LIMA and SVG | 822 (38) |
On-pump | |
Cases (%) | 3811 (63.9) |
No. of grafts | 3.5 (1.6–5.5) |
LIMA ±SVG | 3052 (80) |
LIMA, radial ±SVG | 759 (20) |
CPB time (min) | 109 (61–165) |
Postoperative | |
CKMB | 29.1 (0 to 108) |
Perioperative MI | 266 (4.5) |
Stroke (%) | |
Total | 50 (0.8) |
Permanent | 30 (0.48) |
Temporary | 20 (0.32) |
Re-operation for bleeding | 228 (3.8) |
ICU LOS (Days) | 1.7 (1–5) |
Hospital LOS (Days) | 8.4 (5–15) |
Median follow-up (Days) | 2871 |
In-hospital mortality (%) | 66 (1.1) |
Study period mortality (%) | 806 (13.5) |
. | Data n = 5967 . |
---|---|
Preoperative | |
Age (Years) | 57.2 (45.6–64.6) |
Female (%) | 859 (14.4) |
BMI (Kg/m2) | 29.0 (22.6–37.1) |
Heart failure (%) | 6 (0.10) |
Critical pre-op state (%) | 18 (0.3) |
Previous CVA (%) | 18 (0.3) |
Diabetes (%) | 2136 (35.8) |
Pre-op dialysis (%) | 36 (0.6) |
Previous MI (%) | 2888 (48.4) |
PVD (%) | 680 (11.4) |
Hypertension (%) | 3341 (56) |
Pre-op IABP (%) | 48 (0.8) |
Ejection Fraction | |
Good (%) | 3706 (62.1) |
Moderate (%) | 1772 (29.7) |
Poor (%) | 489 (8.2) |
Previous PCI (%) | 459 (7.7) |
Status | |
Elective (%) | 4994 (83.7) |
Urgent (%) | 901 (15.1) |
Emergency (%) | 72 (1.2) |
Log. EuroSCORE | 2.4 (0.9–6.4) |
Operative | |
LIMA (%) | 5555 (93.1) |
No. of grafts | 3.3 (2.0–5.0) |
Off-pump | |
Cases (%) | 2156 (36.1) |
No. of grafts | 2.9 (1.1–4.9) |
Composite arterial (LIMA – Radial) (%) | 1334 (62) |
LIMA and SVG | 822 (38) |
On-pump | |
Cases (%) | 3811 (63.9) |
No. of grafts | 3.5 (1.6–5.5) |
LIMA ±SVG | 3052 (80) |
LIMA, radial ±SVG | 759 (20) |
CPB time (min) | 109 (61–165) |
Postoperative | |
CKMB | 29.1 (0 to 108) |
Perioperative MI | 266 (4.5) |
Stroke (%) | |
Total | 50 (0.8) |
Permanent | 30 (0.48) |
Temporary | 20 (0.32) |
Re-operation for bleeding | 228 (3.8) |
ICU LOS (Days) | 1.7 (1–5) |
Hospital LOS (Days) | 8.4 (5–15) |
Median follow-up (Days) | 2871 |
In-hospital mortality (%) | 66 (1.1) |
Study period mortality (%) | 806 (13.5) |
Continuous variables are shown as mean with 5 to 95% range shown in brackets. Categorical variables are shown as a percentage. BMI: Body mass index; CVA: cerebral vascular accident; MI: myocardial infarction; PVD: peripheral vascular disease; IABP: intra aortic balloon pump; PCI: percutaneous coronary intervention; LIMA: left internal mammary artery; CPB: cardiopulmonary bypass; CKMB: creatinine kinase myocardial isoenzyme; ICU: intensive care unit; LOS: length of stay; SVG: saphenous vein graft. Perioperative MI, based on a day one CKMB > 110.
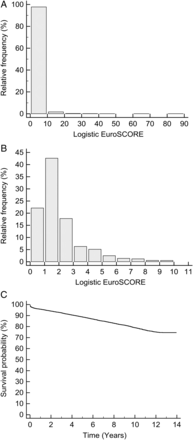
(A) Frequency distribution of logistic EuroSCORE for our study population, (B) frequency distribution of logistic EuroSCOREs under 10 and (C) Kaplan-Meier survival of our study population.
![Kaplan-Meier survival curve based on logistic EuroSCORE: (A) 0 to <5; 5 to <10; 10 to <15; 15 to <20; 20 to <25 and 25 or greater: (B) patients with a EuroSCORE less than 5. (The integer function of EuroSCORE was utilized to turn continuous data into discrete groupings for part b) [24].](https://oup.silverchair-cdn.com/oup/backfile/Content_public/Journal/ejcts/43/5/10.1093_ejcts_ezs459/1/m_ezs45902.gif?Expires=1747923941&Signature=B0UZYUUpykm-jmgO3Y4E9cSMEtBTsYdpPUW77HMEL5XwK2LaX63exKsIZ-WIlTdL~Ui0Ik1hNlUT2WNb6nauObXHuvmvqwd0DXciT5N8fWJNYNscZzD7wfHmdZi0HAPyzPoFR1YI9~T70WrGYDWLA5Ad-bczWyfXGI61L6ltd6OWeI8hGKJJmuJSYmaYrIEMDnixWwB8pHj2Cbg3ancVThc8MtvFNyNITJXAdxf9xYOGW3cFtlfQeaH8QpL4EeBxIjXDxPV152m9mz5IJ0jxUMOYdxUpQxUVRSM9XPXhwk2tgm-yeEK6hdy0OmBa5LEBHXoFd7q8O0-jyQ5gZM90bA__&Key-Pair-Id=APKAIE5G5CRDK6RD3PGA)
Kaplan-Meier survival curve based on logistic EuroSCORE: (A) 0 to <5; 5 to <10; 10 to <15; 15 to <20; 20 to <25 and 25 or greater: (B) patients with a EuroSCORE less than 5. (The integer function of EuroSCORE was utilized to turn continuous data into discrete groupings for part b) [24].
Multivariate analysis
In-hospital mortality
Stepwise logistic regression analysis was used to identify potential significant determining factors with regard to long-term survival. Entry criterion was P < 0.05 and removal criterion was P > 0.1 (Table 2a). Bootstrap analysis was performed with 1000 iterations.
Long-term survival
Stepwise Cox proportional hazards regression analysis was used to identify potential significant determining factors with regard to long-term survival. Entry criterion was P < 0.05 and removal criterion was P > 0.1 (2b).
Neural network analysis
To cross-validate the logistic and Cox regression analyses, a neural network was then trained and run, to create a receiver operating characteristic (ROC) value for assessment of the network ‘goodness of fit’ for predicting in-hospital mortality and long-term survival. All studied variables utilized in the regression analyses were used as input variables for the neural network construction. The basis of this network is multi-layer perceptions that have feed-forward, back-propagation topology. The network consists of four layers (Fig. 3).
The input layer is of neurons into which variables were entered, following their normalization through a process of standard rescaling (subtracting the mean and dividing by the standard deviation).
Two hidden layers composed of neurons, in which computation and differential weighing of the different variables are performed.
The output layer is two neurons, into which the end result is entered (i.e. “alive” or “dead”). Batch training, using 70% of the dataset was employed, with the remaining 30% used for testing.
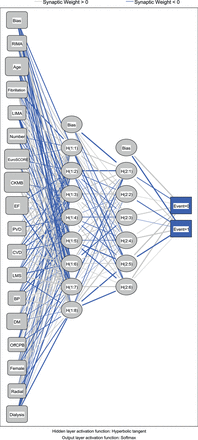
Neural network topography. RIMA: use of right internal mammary artery; Fibrillation: Pre operative atrial fibrillation; LIMA: use of left internal mammary artery; Number: Number of bypass grafts; EuroSCORE: logistic EuroSCORE; CKMB: postoperative CK-MB; EF: Ejection fraction; PVD: peripheral vascular disease; CVD: cerbrovascular vascular disease; LMS: left main stem stenosis; BP: hypertension; DM: diabetes; OffCPB: off pump; Radial: use of radial artery; Dialysis: pre operative dialysis.
Normalized importance output
This was used to determine factors that are important in predicting in-hospital mortality and long-term survival following isolated CABG (Fig. 4).
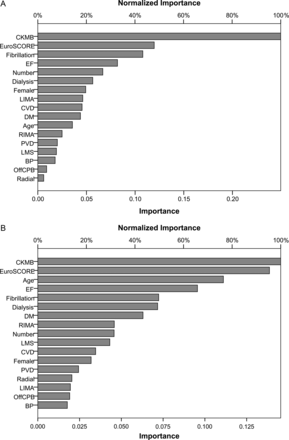
Normalized importance output. See Figure 3 legend for key. (A) In-hospital mortality, (B) long-term survival.
Statistical software
All statistical analyses, other than the neural network and Somers’ Dxy, were performed with MedCalc for Windows, version 12.2.1 (MedCalc Software, Mariakerke, Belgium). The neural network analysis was performed with SPSS for Windows, version 17.0 (SPSS Inc., Chicago, IL, USA). Somers’ C statistic and Dxy was performed with R (http://www.r-project.org).
RESULTS
Univariate analysis
Study group characteristics are shown in Table 1. One hundred percent long-term follow-up was achieved via the UK's national Strategic Tracing Service. Benchmarking of our institutional in-hospital mortality rates, compared to the UK overall, did not reveal any differences; P = 0.4, (part of the continuous UK cardiac surgery quality assessment program by the Society of Cardiothoracic Surgeons Of Great Britain and Ireland). The logistic EuroSCORE ranged from 0.881 to 80.411 (mean 2.441; 95% CI 2.364–2.518); however, over 90% were ≤10 (Fig. 1A). Long-term follow-up was from 6 months to 12 years; median 7.9 years (Fig. 1c).
Long-term survival
Kaplan-Meier survival curves, broken down by quartiles of logistic EuroSCORE, are shown in Fig. 2a. The overall sensitivity and specificity of EuroSCORE for predicting long-term survival was 68% and 61%, respectively, with a C-statistic of 0.69 and a Somers’ Dxy of 0.38.
For patients with a EuroSCORE less than 5 (Fig. 2b), EuroSCORE had a sensitivity and specificity of 58% and 65%, respectively, for predicting long-term survival, with a C-statistic of 0.65 and a Somers’ Dxy of 0.30.
For patients with a EuroSCORE above 25, EuroSCORE had a sensitivity and specificity of 88% and 63%, respectively, for predicting long-term survival, with a C-statistic of 0.8 and a Somers’ Dxy of 0.59.
Age- and sex-matched survival of the general UK population revealed a 1, 2, 3, 4 and 5-year survival rates of 99.2%, 99.1%, 99.0%, 98.9%, and 98.8%, respectively.
Multivariate analysis
In-hospital mortality
Logistic regression (Table 2) demonstrated that CKMB, female sex, preoperative atrial fibrillation and ‘urgent’ operation status were the only factors predicting in-hospital death (ROC 0.80; Hosmer-Lemeshow 0.98). LIMA, right internal mammary artery (RIMA) and radial artery usage, body mass index (BMI), off-pump surgery and composite grafting were not significant factors. The results of the bootstrap analysis are shown in Table 3. Predicted mortality from the logistic regression analysis can be calculated as follows: logit risk = −6.077 + (0.966 × Female sex) + (0.008 × CKMB) + (1.064 × Moderate LV) + (2.261 × Poor LV) + (3.613 × preoperative atrial fibrillation) + (1.798 × Operation status if urgent).
Parameter . | Odds Ratio (OR) . | 95% CI OR . | Coefficient . | Standard error . | P . |
---|---|---|---|---|---|
Constant | −6.077 | ||||
Operation status ‘urgent’ | 6.0364 | 1.9550–18.6389 | 1.798 | 0.57522 | 0.002 |
CKMB | 1.0078 | 1.0060–1.0095 | 0.008 | 0.00089 | <0.0001 |
LV function | |||||
Moderate | 2.8969 | 1.5535–5.4020 | 1.064 | 0.31792 | 0.002 |
Poor | 9.5917 | 4.9676–18.5203 | 2.261 | 0.33570 | <0.0001 |
Female | 2.6282 | 1.4764–4.6785 | 0.966 | 0.29422 | 0.0010 |
Preoperative atrial fibrillation | 37.0631 | 3.5382–388.2433 | 3.613 | 1.19847 | 0.003 |
Parameter . | Odds Ratio (OR) . | 95% CI OR . | Coefficient . | Standard error . | P . |
---|---|---|---|---|---|
Constant | −6.077 | ||||
Operation status ‘urgent’ | 6.0364 | 1.9550–18.6389 | 1.798 | 0.57522 | 0.002 |
CKMB | 1.0078 | 1.0060–1.0095 | 0.008 | 0.00089 | <0.0001 |
LV function | |||||
Moderate | 2.8969 | 1.5535–5.4020 | 1.064 | 0.31792 | 0.002 |
Poor | 9.5917 | 4.9676–18.5203 | 2.261 | 0.33570 | <0.0001 |
Female | 2.6282 | 1.4764–4.6785 | 0.966 | 0.29422 | 0.0010 |
Preoperative atrial fibrillation | 37.0631 | 3.5382–388.2433 | 3.613 | 1.19847 | 0.003 |
CKMB: creatinine kinase muscle brain type; LV: left ventricular.
Parameter . | Odds Ratio (OR) . | 95% CI OR . | Coefficient . | Standard error . | P . |
---|---|---|---|---|---|
Constant | −6.077 | ||||
Operation status ‘urgent’ | 6.0364 | 1.9550–18.6389 | 1.798 | 0.57522 | 0.002 |
CKMB | 1.0078 | 1.0060–1.0095 | 0.008 | 0.00089 | <0.0001 |
LV function | |||||
Moderate | 2.8969 | 1.5535–5.4020 | 1.064 | 0.31792 | 0.002 |
Poor | 9.5917 | 4.9676–18.5203 | 2.261 | 0.33570 | <0.0001 |
Female | 2.6282 | 1.4764–4.6785 | 0.966 | 0.29422 | 0.0010 |
Preoperative atrial fibrillation | 37.0631 | 3.5382–388.2433 | 3.613 | 1.19847 | 0.003 |
Parameter . | Odds Ratio (OR) . | 95% CI OR . | Coefficient . | Standard error . | P . |
---|---|---|---|---|---|
Constant | −6.077 | ||||
Operation status ‘urgent’ | 6.0364 | 1.9550–18.6389 | 1.798 | 0.57522 | 0.002 |
CKMB | 1.0078 | 1.0060–1.0095 | 0.008 | 0.00089 | <0.0001 |
LV function | |||||
Moderate | 2.8969 | 1.5535–5.4020 | 1.064 | 0.31792 | 0.002 |
Poor | 9.5917 | 4.9676–18.5203 | 2.261 | 0.33570 | <0.0001 |
Female | 2.6282 | 1.4764–4.6785 | 0.966 | 0.29422 | 0.0010 |
Preoperative atrial fibrillation | 37.0631 | 3.5382–388.2433 | 3.613 | 1.19847 | 0.003 |
CKMB: creatinine kinase muscle brain type; LV: left ventricular.
Parameter . | Observed Odds Ratio . | Bias . | 95% bias-corrected Confidence Interval . |
---|---|---|---|
Constant | 0.0028 | ||
Operation status ‘urgent’ | 1.2562 | 0.0398 | 0.5854–2.0829 |
CKMB | 1.0076 | 0.0001 | 1.0055–1.0094 |
LV function | |||
Moderate | 2.6190 | 0.1672 | 1.4781–5.0024 |
Poor | 9.3016 | 0.4483 | 5.1379–18.0641 |
Female | 2.3089 | 0.0511 | 1.2328–4.1409 |
Preoperative atrial fibrillation | 30.2331 | 2.0380 | 0.0001–212.8238 |
Parameter . | Observed Odds Ratio . | Bias . | 95% bias-corrected Confidence Interval . |
---|---|---|---|
Constant | 0.0028 | ||
Operation status ‘urgent’ | 1.2562 | 0.0398 | 0.5854–2.0829 |
CKMB | 1.0076 | 0.0001 | 1.0055–1.0094 |
LV function | |||
Moderate | 2.6190 | 0.1672 | 1.4781–5.0024 |
Poor | 9.3016 | 0.4483 | 5.1379–18.0641 |
Female | 2.3089 | 0.0511 | 1.2328–4.1409 |
Preoperative atrial fibrillation | 30.2331 | 2.0380 | 0.0001–212.8238 |
CKMB: creatinine kinase muscle brain type; LV: left ventricular.
Parameter . | Observed Odds Ratio . | Bias . | 95% bias-corrected Confidence Interval . |
---|---|---|---|
Constant | 0.0028 | ||
Operation status ‘urgent’ | 1.2562 | 0.0398 | 0.5854–2.0829 |
CKMB | 1.0076 | 0.0001 | 1.0055–1.0094 |
LV function | |||
Moderate | 2.6190 | 0.1672 | 1.4781–5.0024 |
Poor | 9.3016 | 0.4483 | 5.1379–18.0641 |
Female | 2.3089 | 0.0511 | 1.2328–4.1409 |
Preoperative atrial fibrillation | 30.2331 | 2.0380 | 0.0001–212.8238 |
Parameter . | Observed Odds Ratio . | Bias . | 95% bias-corrected Confidence Interval . |
---|---|---|---|
Constant | 0.0028 | ||
Operation status ‘urgent’ | 1.2562 | 0.0398 | 0.5854–2.0829 |
CKMB | 1.0076 | 0.0001 | 1.0055–1.0094 |
LV function | |||
Moderate | 2.6190 | 0.1672 | 1.4781–5.0024 |
Poor | 9.3016 | 0.4483 | 5.1379–18.0641 |
Female | 2.3089 | 0.0511 | 1.2328–4.1409 |
Preoperative atrial fibrillation | 30.2331 | 2.0380 | 0.0001–212.8238 |
CKMB: creatinine kinase muscle brain type; LV: left ventricular.
Cox regression analysis
Cox regression analysis, to determine the importance of logistic EuroSCORE and other possible predictive factors with regard to long-term survival, is shown in Table 4. Age, atrial fibrillation, diabetes (diet- and insulin-controlled), LV function, cerebrovascular disease, dialysis, LIMA usage, ‘urgent’ operation status, CKMB and peripheral vascular disease were all identified as significant factors. Variables excluded from the model included female sex, hypertension, left main stem stenosis, BMI, number of grafts, off-pump surgery, composite grafting, radial artery utilisation and use of the RIMA.
Covariate . | Relative Risk (RR) . | 95% CI of RR . | P . |
---|---|---|---|
Age | 1.0533 | 1.0375–1.0694 | <0.0001 |
Diabetes | |||
Diet | 1.6545 | 1.3071–2.0943 | <0.0001 |
Insulin | 2.3779 | 1.8677–3.0276 | <0.0001 |
LV function | |||
Moderate | 1.5052 | 1.2630–1.7940 | <0.0001 |
Poor | 3.6293 | 2.9580–4.4528 | <0.0001 |
Cerebrovascular disease | 1.5236 | 1.1798–1.9675 | 0.0013 |
Dialysis | 4.9347 | 2.9218–8.3343 | <0.0001 |
LIMA not used | 1.6626 | 1.3170–2.0988 | <0.0001 |
Logistic EuroSCORE | 1.0024 | 1.0014–1.0034 | <0.0001 |
CKMB | 1.8764 | 1.2540–2.8076 | 0.0023 |
Preoperative atrial fibrillation | 1.3046 | 1.0642–1.5993 | 0.0109 |
Operation status ‘urgent’ | 1.5053 | 1.2325–1.8385 | 0.0001 |
Peripheral vascular disease | 1.0533 | 1.0375–1.0694 | 0.0013 |
Covariate . | Relative Risk (RR) . | 95% CI of RR . | P . |
---|---|---|---|
Age | 1.0533 | 1.0375–1.0694 | <0.0001 |
Diabetes | |||
Diet | 1.6545 | 1.3071–2.0943 | <0.0001 |
Insulin | 2.3779 | 1.8677–3.0276 | <0.0001 |
LV function | |||
Moderate | 1.5052 | 1.2630–1.7940 | <0.0001 |
Poor | 3.6293 | 2.9580–4.4528 | <0.0001 |
Cerebrovascular disease | 1.5236 | 1.1798–1.9675 | 0.0013 |
Dialysis | 4.9347 | 2.9218–8.3343 | <0.0001 |
LIMA not used | 1.6626 | 1.3170–2.0988 | <0.0001 |
Logistic EuroSCORE | 1.0024 | 1.0014–1.0034 | <0.0001 |
CKMB | 1.8764 | 1.2540–2.8076 | 0.0023 |
Preoperative atrial fibrillation | 1.3046 | 1.0642–1.5993 | 0.0109 |
Operation status ‘urgent’ | 1.5053 | 1.2325–1.8385 | 0.0001 |
Peripheral vascular disease | 1.0533 | 1.0375–1.0694 | 0.0013 |
LV: left ventricular; LIMA: left internal mammary artery; CKMB: creatinine kinase muscle brain type.
Covariate . | Relative Risk (RR) . | 95% CI of RR . | P . |
---|---|---|---|
Age | 1.0533 | 1.0375–1.0694 | <0.0001 |
Diabetes | |||
Diet | 1.6545 | 1.3071–2.0943 | <0.0001 |
Insulin | 2.3779 | 1.8677–3.0276 | <0.0001 |
LV function | |||
Moderate | 1.5052 | 1.2630–1.7940 | <0.0001 |
Poor | 3.6293 | 2.9580–4.4528 | <0.0001 |
Cerebrovascular disease | 1.5236 | 1.1798–1.9675 | 0.0013 |
Dialysis | 4.9347 | 2.9218–8.3343 | <0.0001 |
LIMA not used | 1.6626 | 1.3170–2.0988 | <0.0001 |
Logistic EuroSCORE | 1.0024 | 1.0014–1.0034 | <0.0001 |
CKMB | 1.8764 | 1.2540–2.8076 | 0.0023 |
Preoperative atrial fibrillation | 1.3046 | 1.0642–1.5993 | 0.0109 |
Operation status ‘urgent’ | 1.5053 | 1.2325–1.8385 | 0.0001 |
Peripheral vascular disease | 1.0533 | 1.0375–1.0694 | 0.0013 |
Covariate . | Relative Risk (RR) . | 95% CI of RR . | P . |
---|---|---|---|
Age | 1.0533 | 1.0375–1.0694 | <0.0001 |
Diabetes | |||
Diet | 1.6545 | 1.3071–2.0943 | <0.0001 |
Insulin | 2.3779 | 1.8677–3.0276 | <0.0001 |
LV function | |||
Moderate | 1.5052 | 1.2630–1.7940 | <0.0001 |
Poor | 3.6293 | 2.9580–4.4528 | <0.0001 |
Cerebrovascular disease | 1.5236 | 1.1798–1.9675 | 0.0013 |
Dialysis | 4.9347 | 2.9218–8.3343 | <0.0001 |
LIMA not used | 1.6626 | 1.3170–2.0988 | <0.0001 |
Logistic EuroSCORE | 1.0024 | 1.0014–1.0034 | <0.0001 |
CKMB | 1.8764 | 1.2540–2.8076 | 0.0023 |
Preoperative atrial fibrillation | 1.3046 | 1.0642–1.5993 | 0.0109 |
Operation status ‘urgent’ | 1.5053 | 1.2325–1.8385 | 0.0001 |
Peripheral vascular disease | 1.0533 | 1.0375–1.0694 | 0.0013 |
LV: left ventricular; LIMA: left internal mammary artery; CKMB: creatinine kinase muscle brain type.
Neural network analysis
In-hospital mortality
The neural network created had a ROC of 0.78, virtually identical to the logistic regression analysis, which was 0.79. The normalized predictive values (Fig. 4a) of the neural network identified atrial fibrillation, postoperative CKMB and logistic EuroSCORE as the three most important variables predicting in-hospital mortality, while hypertension, PVD, off-pump surgery, radial artery usage, and left main stem stenosis (LMS) were of minimal importance; findings similar to the logistical model.
Long-term survival
The neural network created had a C-statistic of 0.69. The normalized predictive values (Fig. 4b) of the neural network identified atrial fibrillation, postoperative CKMB, diabetes and logistic EuroSCORE as important variables predicting poor long-term survival, while hypertension, PVD, off-pump surgery, radial artery usage and LIMA use were of minimal importance in predicting poor survival; findings similar to the Cox modelling.
DISCUSSION
The risk factors for in-hospital mortality in patients under the age of 65 include preoperative atrial fibrillation and CKMB release, in addition to the factors contained in EuroSCORE. Risk factors for long-term survival include LIMA usage, diabetes, preoperative atrial fibrillation and CKMB release after surgery, in addition to the factors contained in EuroSCORE.
Prognostic factors affecting survival have become increasingly important since the introduction of stenting [5]. Identification of patient subgroups that may have a better or worse prognosis is important, especially for comparative studies involving surgery and stenting. The syntax score was not available for our patients, but its value in surgical patients has been questioned [10]. The long-term mortality of 13.5% may be indicative of the underlying aggressive disease that predisposes this cohort of patients to require surgery at a young age.
Atrial fibrillation has previously been described as a risk factor for in-hospital mortality and long-term survival. We have confirmed this finding in a younger, isolated CABG cohort. The possible role of ablative surgery at the time of CABG, to decrease in-hospital mortality and improve long-term survival, remains unanswered.
EuroSCORE remains of prognostic significance following primary CABG, even in the under-65 age group; however, the use of the additional risk factors of diabetes, preoperative atrial fibrillation, LIMA usage, CKMB and use of the radial artery increases the predictive accuracy.
Two large recent series of isolated CABG have documented the risk factors determining long-term survival in all cases and, separately, in those over the age of 65. The first study identified that age, diabetes, ejection fraction, BMI, dialysis, logistic EuroSCORE, CKMB, LIMA usage and peripheral arterial disease were among significant factors affecting long-term survival [11]. Except for diabetes, BMI, CKMB and LIMA usage, all these variables are incorporated in the EuroSCORE; however, their weighting will vary. This explains why EuroSCORE is a reasonable approximation as a predictor for long-term survival, although the incidence of diabetes, CKMB and LIMA usage will affect its accuracy. These additional factors, not included in the EuroSCORE, have been described previously [12–14]. The second study, which was limited in having a median follow-up of only four years, identified numerous factors but did not include LIMA, bilateral internal mammary artery (BIMA), radial artery, or off-pump surgery as potential factors influencing long-term survival [8].
Body mass index has previously been described as a risk factor for in-hospital mortality in cardiac surgery and as a factor affecting long-term survival post-CABG; however, in patients under the age of 65, it is not a significant factor for either [15].
Use of the internal mammary artery is known to be associated with increased survival following CABG; however, this has not been included in a number of previous long-term predictive studies [16–18]. The radial artery was utilized in a large number of our patients (over 2000); however, this was not associated with a significantly increased long-term survival.
Intra-operative myocardial damage is the main cause of cardiac dysfunction following cardiac surgery, be it myocardial stunning, inadequate cardioplegia or coronary graft dysfunction. The finding that CKMB was a significant factor affecting in-hospital and long-term outcome demonstrates the importance of intra-operative myocardial protection and coronary anastomosis technique, and has been previously identified [12].
In respect of in-hospital mortality and long-term survival, debate continues on the advantages or disadvantages of on- or off-pump surgery, composite grafting, radial artery use and number of grafts performed [19–21]. Inclusion of these factors in our multivariate analysis demonstrated that none have any effect on in-hospital mortality or long-term survival.
Neural networks remain contentious due to their ‘black box’ technology; however the technique predicted exactly the same additional factors to EuroSCORE as did logistic regression for in-hospital mortality and Cox regression for long-term survival. The normalized output option with neural networks demonstrated the importance of atrial fibrillation and postoperative CKMB, in addition to logistic EuroSCORE, as predictors of in-hospital mortality and long-term survival; findings that are similar to the logistic- and Cox modelling performed.
We made no attempt to break down the follow-up period into time intervals, as has been done previously [16, 22, 23]. It should be noted that previous work on developing models of long-term outcomes has not included CKMB, BMI, LIMA, RIMA, radial artery use and off-pump surgery usage as possible factors affecting survival, despite their known association with long-term outcome [12, 13].
Quality improvement via unit benchmarking is becoming increasingly commonplace. With regard to in-hospital mortality and long-term survival comparisons based solely on logistic EuroSCORE, risk adjusted figures may lead to incorrect conclusions: a type I error. We suggest that LIMA utilisation and an enzyme marker of myocardial damage, such as CKMB, could be used as potential key performance indicators, as both are significant factors determining long-term survival.
The risk factors for in-hospital mortality in patients under the age of 65 include postoperative CKMB, ‘urgent’ operation status, LV function, female sex and atrial fibrillation. Significant factors determining long-term survival in the under-65 age group include age, atrial fibrillation, diabetes (diet- and insulin controlled), LV function, cerebrovascular disease, dialysis, LIMA usage, ‘urgent’ operation status, CKMB, and peripheral vascular disease.
Limitations
The 13-year time period from which the patients in this study were recruited may introduce a time factor error. This is a common confounding factor of any large study that covers a long period of time. We do not have cause of death for any of the patients after hospital discharge; a frequent limitation of retrospective analyses. In addition, we do not have long-term major adverse cardiac event (MACE) data available. The use of arterial grafting was at the discretion of the surgeon, potential introducing statistical bias.
Conflict of interest: none declared.
REFERENCES
- atrial fibrillation
- peripheral vascular diseases
- ventricular function, left
- coronary artery bypass surgery
- hemodialysis
- diabetes mellitus
- internal thoracic artery
- hospital mortality
- radial artery
- surgical procedures, operative
- dialysis procedure
- surgery specialty
- cox proportional hazards models
- creatine kinase mb isoenzyme