-
PDF
- Split View
-
Views
-
Cite
Cite
Jonathan L Ciofani, Daniel Han, Karan Rao, Dipender Gill, Benjamin Woolf, Kazem Rahimi, Usaid K Allahwala, Ravinay Bhindi, Lipid-lowering therapies for aortic stenosis: a drug-target Mendelian randomization study, European Heart Journal - Cardiovascular Pharmacotherapy, Volume 11, Issue 2, March 2025, Pages 136–142, https://doi.org/10.1093/ehjcvp/pvae092
- Share Icon Share
Abstract
Large observational and Mendelian randomization (MR) studies have demonstrated a strong association between both elevated LDL cholesterol (LDL-c) and triglycerides (TG) with risk of aortic stenosis (AS), although randomized trials showed no benefit of statins for AS. It consequently remains uncertain whether lipid-lowering therapies have a role to prevent or treat AS. We used a drug-target MR approach to investigate the genetically predicted effect of lipid-lowering therapies on risk of AS.
We collected summary statistics for LDL-c, TG, and AS from genome-wide association studies (GWAS) including 1 320 016, 1 253 277, and 412 181 European participants from the Global Lipids Genetics Consortium and FinnGen study, respectively. We identified genetic proxies for PCSK9 inhibitors, statins, bempedoic acid, and ezetimibe as single nucleotide polymorphisms in or within 200 kb of the target genes (PCSK9, HMGCR, ACLY, and NPC1L1, respectively), which were also significantly associated with LDL-c at P < 5 × 10−8. We used a similar approach to identify genetic proxies for the TG-lowering agents fenofibrates, APOC3 inhibitors, and ANGPTL3 inhibitors using the target genes PPARA, APOC3, and ANGPTL3, respectively. Inverse variance-weighted was the primary analysis method. Sensitivity analyses included weighted median, weighted mode, and MR-Egger, followed by the outlier-exclusion approaches MR-PRESSO and Cook's distance. We also performed multivariable analyses to evaluate whether the predicted effect of PCSK9 inhibition may be mediated by lipoprotein(a). We performed replication and negative control analyses using GWAS of AS and height including 653 867 and 408 112 participants, respectively. Genetically proxied PCSK9 inhibition was significantly associated with reduced AS risk (odds ratio [OR] 0.61, 95% confidence interval [CI] 0.52–0.72, P < 0.0001) on main, replication, and all sensitivity analyses. Genetically proxied ezetimibe (OR 0.49, 95% CI 0.31–0.78, P = 0.003), bempedoic acid (OR 0.0054, 95% CI 0.0002–0.12, P = 0.0009), and statins (OR 0.61, 95% CI 0.46–0.81, P = 0.0006) were similarly associated with reduced AS risk, although the latter were not significant on replication analyses. Amongst the TG-lowering agents, genetically proxied APOC3 inhibition was associated with reduced AS risk (OR 0.78, 95% CI 0.70–0.88, P < 0.0001), but fenofibrate (OR 0.64, 95% CI 0.09–4.53, P = 0.65) and ANGPTL3 inhibitors (OR 1.05, 95% CI 0.77–1.43, P = 0.74) were not.
Genetically proxied lipid-lowering therapies are significantly associated with reduced risk of AS. Early initiation and sustained administration of lipid-lowering therapies may prevent AS progression and warrants further research in the clinical trial setting.
Introduction
Calcific aortic stenosis (AS) is the most common valvular pathology in the developed world, yet despite its rising prevalence there remains no evidence-based medical therapy. Large observational and Mendelian randomization (MR) studies demonstrated significant associations between elevated LDL cholesterol (LDL-c) and triglycerides (TG) with AS risk.1 Concordantly registry studies have demonstrated increased risk of aortic valve calcification among patients with familial hypercholesterolaemia.2,3 However, the randomized trials SEAS, SALTIRE, and ASTRONOMER consistently showed no benefit of statins for AS.4–6 There are several potential explanations for these discrepant findings. The observational findings may be biased by confounding since individuals with dyslipidaemia may have been enriched for investigation of coronary artery disease and incidentally detected AS. Conversely, the randomized trials may have evaluated AS at a stage that was too far progressed for lipid-lowering therapy to provide significant benefit. Indeed, the mechanisms of both coronary atherosclerosis and AS progression are recognized to be multistep processes, and consequently disease-modifying therapies for early- versus late-stage disease may differ. In ApoE knockout mice, for example, the atheroprotective effects of infusing ApoA-I, the main protein constituent of HDL cholesterol, was seen when administered to young mice with early-stage disease but was substantially attenuated in older mice with late-stage disease.7 Not dissimilarly, the current understanding of AS pathophysiology comprises initiation and propagation processes. The initiation step involves endothelial insult, pathogenic lipoprotein deposition into the valve fibrosa, lipid oxidation, and inflammatory cell infiltration. The propagation phase is then characterized by fibrosis, which acts as a nidus for subsequent progressive calcification.8,9 It is plausible that early commencement of lipid-lowering therapies may reduce the risk of AS development and progression, but be ineffective if used for late-stage disease.
Drug-target MR provides a methodological opportunity to predict the effect of early initiation and long-term administration of drug therapies before committing to costly and time-consuming randomized controlled trials (RCTs) (Figure 1). MR leverages the fact that a proportion of an individual's phenotype is determined by genetic polymorphisms, which are inherited randomly at birth, and consequently overcomes many issues with observational studies including confounding and reverse causation.10,11 Drug-target MR extends conventional MR by identifying polymorphisms in the gene for a drug target that perturbs downstream mediators, and thus the randomly inherited polymorphisms proxy the drug effect.12,13 Indeed, well-conducted MR studies have repeatedly demonstrated concordant results with RCTs,14 and provide the benefit of predicting the effect of long-term exposure since the drug-proxying polymorphisms are inherited at birth. When interpreting the results of drug-target MR analyses, the effect sizes are typically larger compared to comparable RCTs due to the lifelong exposure to genetic variants. The current study applies the drug-target MR approach to predict the effects of early initiation and long-term administration of lipid-lowering therapies on AS.
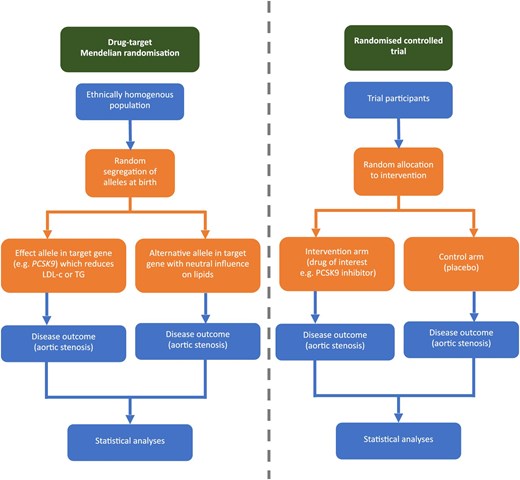
Drug target Mendelian randomization study design. LDL-c, LDL cholesterol; TG, triglyceride. Adapted from Ciofani et al. 2023.28
Methods
Study design
This study uses a two-sample MR approach with summary data from published genome-wide association studies (GWAS)15–19 (see Supplementary material online, Table S1). First, conventional MR analyses were performed to evaluate for causal relationships between genetically predicted LDL-c, TG, and lipoprotein(a) with risk of AS. Then two drug groups were considered: agents that predominantly act by LDL-c reduction (PCSK9 inhibitors, statins, bempedoic acid, and ezetimibe) and those that predominantly reduce TG (APOC3 inhibitors, ANGPTL3 inhibitors, and fenofibrate). We performed the drug-target MR analyses using two independent AS datasets to evaluate the reproducibility of the results, and included MR analyses for the relationship between lipid-lowering therapy and height as a negative control.
Population homogeneity is a requirement for two-sample MR analyses, and thus European ancestry populations were used for both the exposure and outcome datasets. Mendelian randomization makes three further core assumptions, which are that the genetic variants should be (i) strongly associated with the exposure; (ii) exclusively associated with the outcome via the exposure; and (iii) independent of potential confounders. To address the first criterion, uncorrelated genetic variants were selected that were significantly associated with the exposure subsets at P < 5 × 10−8; and to evaluate the latter two criteria, sensitivity analyses were performed as described below.
All data and material used in this MR study are publicly available. Informed consent and ethical approval were obtained by the original GWAS. The latest STROBE-MR guidelines were consulted to prepare this manuscript.20
Data sources
The data sources for this study are summarized in Supplementary material online, Table S1. Data for LDL-c and TG were obtained from a GWAS meta-analysis performed by the Global Lipids Genetics Consortium using data from up to 146 cohorts including 1 320 016 participants15; and the lipoprotein(a) summary statistics were sourced from a GWAS of UK Biobank including 361 194 participants.16 In the original GWAS meta-analysis, individuals on cholesterol-lowering therapy had their pre-medication levels approximated by dividing the LDL-c by 0.7.15 To evaluate the reproducibility of our MR analyses, we used two independent GWAS datasets for AS: the main analysis summary statistics for AS were extracted from a GWAS of the FinnGen database including 9870 cases coded as calcific AS requiring operation and 402 311 controls18; then replication analyses were performed using a GWAS meta-analysis of 10 cohorts including 13 765 cases and 640 102 controls with varying definitions for AS inclusion, the details of which are available in the original manuscript.17 Height was used as the negative control outcome variable, with data extracted from a GWAS of UK Biobank participants including 283 749 participants.19
Genes encoding the protein targets of the drugs under investigation were identified using the DrugBank database. This included PCSK9 for PCSK9 inhibitors, HMGCR for statins, ACLY for bempedoic acid, and NPC1L1 for ezetimibe amongst the LDL-c-lowering drugs; and APOC3 for APOC3 inhibitors such as volanesorsen, ANGPTL3 for ANGPTL3 inhibitors such as evinacumab, and PPARA for fenofibrate among the TG-lowering drugs. We identified single nucleotide polymorphisms (SNPs) in or within 200 kb of the target genes, which were also significantly associated with LDL-c or TG, respectively, at P < 5 × 10−8 (Supplementary Table S10). Palindromic SNPs were excluded. Single nucleotide polymorphisms were initially clumped using a linkage disequilibrium threshold of r2 < 0.1 and 10 Mb distance cut-off using the 1000 genomes European reference panel. A stricter clumping threshold of r2 < 0.01 was subsequently used to evaluate the robustness of the initial findings. F statistics were identified to determine the strength of each SNP as an instrumental variable, and weak instruments (F statistic < 10) were excluded from analyses to reduce the risk of weak instrument bias.
Statistical analyses
The primary MR analysis was an inverse variance-weighted (IVW) random effect model for each of LDL-c, TG, and lipoprotein(a) on risk of AS. We then performed drug-target MR for each of the proxied drugs. Sensitivity analyses included weighted median, weighted mode, MR-Egger, and outlier exclusion using MR-PRESSO and Cook's distance. Each method makes different assumptions and concordance between methods provides confidence in the conclusions. Weighted median assumes at least half the instrumental variables are valid. Weighted mode assumes the most common causal effect is consistent with the true effect. MR-Egger uses the Instrument Strength Independent of Direct Effect assumption, which states that the strength of pleiotropic effects from the genetic variants to the outcome is independent of the strength of the association between genetic variants and exposure. Under this assumption, the average pleiotropic effect of the genetic variants is estimated by the intercept from MR-Egger analysis. MR-PRESSO and Cook's distance approaches provide revised causal estimates after outlier correction.21 When reporting results, we only included MR-PRESSO and Cook's distance analysis results when outliers were identified and removed, otherwise the results were consistent with the standard IVW. Horizontal pleiotropy was evaluated using the Egger intercept, and heterogeneity assessed by Cochran's Q statistic. For PCSK9 inhibition, we additionally performed multivariable MR to evaluate whether the predicted effects may be mediated by lipoprotein(a). Sensitivity analyses were also included for multivariable MR using the generalized method of moments22 and two-step cis-MR approaches.23 Bonferroni correction was applied for multiple testing such that the threshold for statistical significance for the drug-target MR analyses was set at 0.007 (0.05 divided by 7, which is the number of lipid-lowering drugs evaluated in the current manuscript).
Statistical analyses were performed using R version 1.4.1106. Analyses with a continuous outcome were reported using beta values, and analyses with a binary outcome were reported using odds ratios (OR).
Results
Genetically predicted higher LDL-c (OR 1.63 per standard deviation increase in LDL-c, 95% confidence interval [CI] 1.45–1.82, P < 0.0001), TG (OR 1.23 per standard deviation increase in TG, 95% CI 1.09–1.39, P = 0.0006), and lipoprotein(a) (OR 1.22 per standard deviation increase in lipoprotein(a), 95% CI 1.07–1.39, P = 0.0003) were all significantly associated with increased risk of AS (Figure 2). For each lipid parameter, the association was robust to sensitivity analyses (see Supplementary material online, Table S2).
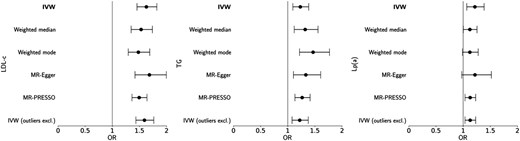
Conventional Mendelian randomization (MR) analyses for the effect of one standard deviation increase in genetically proxied LDL cholesterol (LDL-c), triglycerides (TG), and lipoprotein(a) (Lp(a)) on risk of aortic stenosis. Primary inverse variance-weighted (IVW) and sensitivity analyses are presented; outliers excluded based on Cook's distance method.
Genetically proxied PCSK9 inhibition by 36 independent SNPs in the main analysis was significantly associated with reduced risk of AS (OR 0.61 per standard deviation decrease in LDL-c, 95% CI 0.52–0.72, P < 0.0001), which was consistent on all sensitivity analyses including weighted median, weighted mode, MR-Egger, outlier-exclusion, stricter clumping threshold, and replication analyses (Figure 3 and Table 1; see Supplementary material online, Tables S3–S4 and S8, Figures S1–S2). For the main analysis, the Egger intercept did significantly differ from zero (intercept 0.012, P = 0.048); there was no statistically significant heterogeneity detected (Q statistic 40.7, P = 0.23) (see Supplementary material online, Table S3). No SNPs in the PCSK9 gene region were significantly associated with lipoprotein(a) and, concordantly, there was no attenuation of the relationship between genetically predicted PCSK9 inhibition by LDL-c and AS risk when accounting for the relationship with lipoprotein(a) (OR 0.64, 95% CI 0.50–0.82, P < 0.001). This was consistent on multivariable analyses using the generalized method of moments and two-stage cis-MR approaches (see Supplementary material online, Table S7).
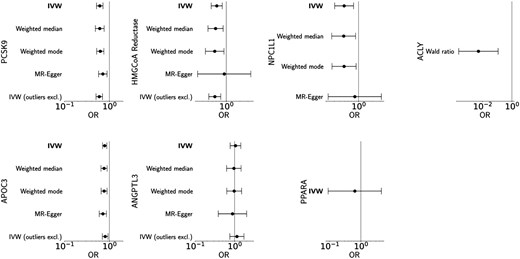
Drug-target Mendelian randomization (MR) analyses. Top panel: genetically proxied effects of PCSK9, HMGCoA reductase, NPC1L1, and ACLY inhibition on risk of aortic stenosis per standard deviation decrease in LDL cholesterol. Bottom panel: genetically proxied effects of APOC3, ANGPTL3, and PPARA inhibition on risk of aortic stenosis per standard deviation decrease in triglycerides. Primary inverse variance-weighted (IVW) and sensitivity analyses are presented; outliers excluded based on Cook's distance method.
Replication analyses for the effect of genetically proxied lipid-lowering therapies on risk of aortic stenosis (summary statistics extracted from Chen et al.17)
Drug target . | Odds ratio (95% CI) . | P value . |
---|---|---|
PCSK9 inhibition | 0.66 (0.54–0.80) | <0.0001 |
Statins | 0.92 (0.66–1.27) | 0.60 |
Ezetimibe | 0.47 (0.28–0.81) | 0.006 |
Bempedoic acid | 2.27 (0.08–66.70) | 0.64 |
APOC3 inhibition | 0.72 (0.65–0.81) | <0.0001 |
ANGPTL3 inhibition | 1.00 (0.75–1.33) | 1.00 |
Fenofibrate | 0.50 (0.09–2.76) | 0.43 |
Drug target . | Odds ratio (95% CI) . | P value . |
---|---|---|
PCSK9 inhibition | 0.66 (0.54–0.80) | <0.0001 |
Statins | 0.92 (0.66–1.27) | 0.60 |
Ezetimibe | 0.47 (0.28–0.81) | 0.006 |
Bempedoic acid | 2.27 (0.08–66.70) | 0.64 |
APOC3 inhibition | 0.72 (0.65–0.81) | <0.0001 |
ANGPTL3 inhibition | 1.00 (0.75–1.33) | 1.00 |
Fenofibrate | 0.50 (0.09–2.76) | 0.43 |
Odds ratios are based on inverse variance-weighted Mendelian randomization analyses and reported per standard deviation decrease in LDL cholesterol (for PCSK9 inhibition, statins, ezetimibe, and bempedoic acid) or triglycerides (for APOC3 inhibition, ANGPTL3 inhibition, and fenofibrate).
Replication analyses for the effect of genetically proxied lipid-lowering therapies on risk of aortic stenosis (summary statistics extracted from Chen et al.17)
Drug target . | Odds ratio (95% CI) . | P value . |
---|---|---|
PCSK9 inhibition | 0.66 (0.54–0.80) | <0.0001 |
Statins | 0.92 (0.66–1.27) | 0.60 |
Ezetimibe | 0.47 (0.28–0.81) | 0.006 |
Bempedoic acid | 2.27 (0.08–66.70) | 0.64 |
APOC3 inhibition | 0.72 (0.65–0.81) | <0.0001 |
ANGPTL3 inhibition | 1.00 (0.75–1.33) | 1.00 |
Fenofibrate | 0.50 (0.09–2.76) | 0.43 |
Drug target . | Odds ratio (95% CI) . | P value . |
---|---|---|
PCSK9 inhibition | 0.66 (0.54–0.80) | <0.0001 |
Statins | 0.92 (0.66–1.27) | 0.60 |
Ezetimibe | 0.47 (0.28–0.81) | 0.006 |
Bempedoic acid | 2.27 (0.08–66.70) | 0.64 |
APOC3 inhibition | 0.72 (0.65–0.81) | <0.0001 |
ANGPTL3 inhibition | 1.00 (0.75–1.33) | 1.00 |
Fenofibrate | 0.50 (0.09–2.76) | 0.43 |
Odds ratios are based on inverse variance-weighted Mendelian randomization analyses and reported per standard deviation decrease in LDL cholesterol (for PCSK9 inhibition, statins, ezetimibe, and bempedoic acid) or triglycerides (for APOC3 inhibition, ANGPTL3 inhibition, and fenofibrate).
Genetically proxied HMGCoA reductase inhibition using 19 independent SNPs in the main analysis was similarly associated with reduced risk of AS on main and sensitivity analyses (OR 0.61 per standard deviation decrease in LDL-c, 95% CI 0.46–0.81, P = 0.0006; see Supplementary material online, Table S3), although this result did not remain significant using a stricter clumping threshold (9 SNPs; OR 0.59, 95% CI 0.33–1.07, P = 0.08; see Supplementary material online, Table S4) or on replication analyses (17 SNPs; OR 0.92, 95% CI 0.66–1.27, P = 0.60; see Supplementary material online, Table S8). Similarly genetically proxied ezetimibe using 12 independent SNPs in the main analysis was significantly associated with reduced risk of AS (OR 0.49 per standard deviation decrease in LDL-c, 95% CI 0.31–0.78, P = 0.003), which was reproduced in the replication analysis (13 SNPs; OR 0.47, 95% CI 0.28–0.81, P = 0.006) but no longer significant using the stricter clumping threshold (3 SNPs; OR 0.60, 95% CI 0.24–1.49, P = 0.27). Only one SNP was identified to proxy bempedoic acid, rs13412, which is located upstream of the ACLY gene. This was significantly associated with reduced AS risk on main (OR 0.005, 95% CI 0.0002–0.12, P = 0.0009) but not replication analysis (OR 2.27, 95% CI 0.08–67.0, P = 0.64) (see Supplementary material online, Tables S3 and S8).
Amongst the TG-lowering drugs, genetically proxied APOC3 inhibition was significantly associated with reduced AS risk on main analysis (43 SNPs; OR 0.78 per standard deviation decrease in TG, 95% CI 0.70–0.88, P < 0.0001) and replication analysis (49 SNPs; OR 0.72, 95% CI 0.65–0.81, P < 0.0001), with a similar numerical but not statistically significant association with a stricter clumping threshold (19 SNPs; OR 0.88, 95% CI 0.71–1.09, P = 0.25). Inhibition of ANGPTL3 (22 SNPs; OR 1.05, 95% CI 0.77–1.43, P = 0.74) and fenofibrates (2 SNPs; OR 0.64, 95% CI 0.09–4.53, P = 0.65) were not predicted to significantly reduce the risk of AS (see Supplementary material online, Tables S5, S6, and S8).
Negative control drug-target MR analyses were performed to evaluate whether the lipid-lowering therapies investigated above are significantly associated with height; these analyses were expectedly null (Table 2 and see Supplementary material online, Table S9).
Negative control for the effect of genetically proxied lipid-lowering therapies on height
Drug target . | Beta (SE) . | P value . |
---|---|---|
PCSK9 inhibition | 0.099 (0.12) | 0.42 |
Statins | −0.11 (0.29) | 0.71 |
Ezetimibe | 0.015 (0.39) | 0.97 |
Bempedoic acid | 1.15 (2.00) | 0.56 |
APOC3 inhibition | −0.042 (0.11) | 0.70 |
ANGPTL3 inhibition | 0.070 (0.28) | 0.80 |
Fenofibrate | 3.70 (3.13) | 0.24 |
Drug target . | Beta (SE) . | P value . |
---|---|---|
PCSK9 inhibition | 0.099 (0.12) | 0.42 |
Statins | −0.11 (0.29) | 0.71 |
Ezetimibe | 0.015 (0.39) | 0.97 |
Bempedoic acid | 1.15 (2.00) | 0.56 |
APOC3 inhibition | −0.042 (0.11) | 0.70 |
ANGPTL3 inhibition | 0.070 (0.28) | 0.80 |
Fenofibrate | 3.70 (3.13) | 0.24 |
Beta values are based on inverse variance-weighted Mendelian randomization analyses and reported per standard deviation decrease in LDL cholesterol (for PCSK9 inhibition, statins, ezetimibe, and bempedoic acid) or triglycerides (for APOC3 inhibition, ANGPTL3 inhibition, and fenofibrate).
SE, standard error.
Negative control for the effect of genetically proxied lipid-lowering therapies on height
Drug target . | Beta (SE) . | P value . |
---|---|---|
PCSK9 inhibition | 0.099 (0.12) | 0.42 |
Statins | −0.11 (0.29) | 0.71 |
Ezetimibe | 0.015 (0.39) | 0.97 |
Bempedoic acid | 1.15 (2.00) | 0.56 |
APOC3 inhibition | −0.042 (0.11) | 0.70 |
ANGPTL3 inhibition | 0.070 (0.28) | 0.80 |
Fenofibrate | 3.70 (3.13) | 0.24 |
Drug target . | Beta (SE) . | P value . |
---|---|---|
PCSK9 inhibition | 0.099 (0.12) | 0.42 |
Statins | −0.11 (0.29) | 0.71 |
Ezetimibe | 0.015 (0.39) | 0.97 |
Bempedoic acid | 1.15 (2.00) | 0.56 |
APOC3 inhibition | −0.042 (0.11) | 0.70 |
ANGPTL3 inhibition | 0.070 (0.28) | 0.80 |
Fenofibrate | 3.70 (3.13) | 0.24 |
Beta values are based on inverse variance-weighted Mendelian randomization analyses and reported per standard deviation decrease in LDL cholesterol (for PCSK9 inhibition, statins, ezetimibe, and bempedoic acid) or triglycerides (for APOC3 inhibition, ANGPTL3 inhibition, and fenofibrate).
SE, standard error.
Discussion
In this study, we found that genetically predicted lipid-lowering therapies were associated with reduced risk of AS. Amongst the LDL-c-lowering drugs, genetically predicted PCSK9 inhibition, ezetimibe, bempedoic acid, and statins all demonstrated benefit for AS, with the most robust and reproducible evidence found for PCSK9 inhibition. Amongst the TG-lowering drugs, genetically predicted APOC3 inhibition was associated with lower risk of AS. These results suggest that early- and long-term administration of lipid-lowering therapies may reduce the risk of AS.
The findings of the present study are consistent with previous large observational and MR studies. The observational Cardiovascular Health Study of 5201 participants showed a significant association of both LDL-c and lipoprotein(a) with risk of AS,24 and this was supported by previous MR analyses demonstrating higher risk of AS with elevated LDL-c and TG.1 However, previous RCTs have not supported the use of statins for AS.4–6 The most notable characteristic of the clinical trial populations has been the inclusion of only patients with established AS, with the trials aiming to evaluate whether statins can slow AS progression. Additionally, the SALTIRE and ASTRONOMER RCTs were small trials (150 and 269 participants, respectively) with <4 years of median follow-up, and the SEAS trial enrolled only patients with relatively advanced AS demonstrating a mean peak velocity 3.1 m/s. These factors are of particular relevance since AS is a multistage process with different pathomechanistic processes at each stage, and dyslipidaemia is recognized to have a role in the early pathophysiological stages. Consequently, interventions affecting disease incidence versus progression may differ, and specifically lipid-lowering therapies may exclusively or predominantly have benefit prior to establishment of advanced AS disease. Importantly, the current drug-target MR analyses proxy the effect of early initiation and sustained administration of lipid-lowering therapies, since the polymorphisms are inherited at birth and persist lifelong, and thus predict intervention at an earlier stage than the clinical trials to date. The present results are consistent with recent work demonstrating that rare genetic variants in LDLR, APOB, and PCSK9 are associated with AS.25 Together, these results support the hypothesis that the risk of AS attributable to dyslipidaemia may be due to cumulative long-term exposure and that lipid-lowering therapies should be administered prior to and/or early in the disease process if they are to provide benefit for AS risk reduction.
In the present study the most robust evidence for reduced risk of AS was with genetically predicted PCSK9 inhibition, based on concordance across all sensitivity and replication analyses. Several of the other genetically predicted drugs, including statins, ezetimibe, and APOC3 inhibitors, were significantly associated with lower AS risk on main analysis but were no longer significant after applying a stricter clumping threshold to SNP selection. The stricter threshold was applied to further reduce the risk of correlated instrumental variables in the final analysis, although it is worth noting that the upfront main analysis threshold of r2 < 0.1 was already reasonably conservative compared to the existing literature to obviate this potential bias.26 Regardless, the re-analysis with SNPs restricted to a stricter clumping threshold resulted in fewer eligible SNPs, wider confidence intervals but relatively preserved point estimates, suggesting that the lack of significance may have been due to underpowering. Similarly, genetically predicted statins and bempedoic acid were both significantly associated with reduced AS risk on main but not replication analyses, the reasons for which are not entirely clear. The data extracted from the FinnGen study were restricted to calcific AS that was operated upon. This likely represents a more severe phenotype compared to the replication analysis, which used a meta-analysis of 10 cohorts with varying definitions of AS ranging from severe AS confirmed on echocardiography in a French biobank to several constituent studies which defined AS based on International Classification of Diseases codes.17 It is also recognized that statins are associated with increased vascular calcification27; whilst statins may stabilize vascular plaque and be protective in coronary artery disease, they may counteract the benefit of LDL-c reduction in AS, which may explain the lack of significant association on replication. Furthermore, it was not possible with the existing data to stratify participants based on concurrent comorbidities, such as concomitant atherosclerotic disease, which would be of interest for substudy. It is also important to note that while the present study predicts beneficial effects of lipid-lowering therapies on risk of AS, the exact effect sizes demonstrated in the present study cannot be extrapolated to predict clinical effect sizes. The present data therefore provide impetus to conduct RCTs, which recruit individuals at high risk of AS to evaluate the effect of lipid-lowering therapies in this cohort.
Several important limitations of the present study should be considered. Firstly, since MR studies presume lifelong exposure from birth, the present study cannot inform the optimal timing of initiating medical therapy other than to suggest that early- and long-term drug administration is expected to reduce the risk of AS. Secondly, there remains the possibility of pleiotropic pathways not mediated by LDL-c or TG reduction, but which are still mediated by inhibition of the target gene product. This would not alter the validity of the overall conclusions, but may explain differences in the results for each LDL-c- and TG-lowering drug. Thirdly, the multivariable analysis for the effect of PCSK9 inhibition via LDL-c after accounting for lipoprotein(a) should be interpreted with caution given that no association was identified between SNPs in the PCSK9 gene region and lipoprotein(a) at conventional significance threshold. Fourthly, discrepant results between the main and replication analyses may be explained by differences in the definition of AS, particularly since several studies in the replication dataset used hospital diagnosis codes for study inclusion raising the possibility of misclassification biases. Future studies would benefit from datasets with standardized echocardiographic inclusion criteria for AS. Finally, the present study used data from European ancestry individuals, which may limit generalizability to the broader population.
Conclusions
We found evidence that lipid-lowering therapies which reduce LDL-c may be expected to reduce the risk of AS, with the most robust evidence identified for genetically proxied PCSK9 inhibition. Amongst the TG-lowering therapies, genetically proxied APOC3 inhibition was associated with reduced risk of AS. These findings warrant further investigation in the RCT setting with early- and long-term drug administration.
Funding
The work for the present study received no direct funding. K.R. reports grants outside the submitted work from the British Heart Foundation, Horizon Europe AI4HF consortium (R79992/CN001), Novo Nordisk Oxford Big Data Partnership University of Oxford, the National Institute for Health Research Oxford Biomedical Centre, Oxford Martin School, and UKRI's Global Challenge Research Fund Grant Ref: ES/P011055/1. K.R. has previously received consulting fees from Medtronic CRDN, and honoraria or fees from BMJ Heart, PLoS Medicine, AstraZeneca MEA Region, Medscape, and WebMD Medscape UK. J.C. reports grant funding from Heart Research Australia. All other authors declare no relevant funding.
Conflict of interest: All authors declare no competing interests.
References
- aortic valve stenosis
- ldl cholesterol lipoproteins
- triglycerides
- statins
- lipoproteins
- drug delivery systems
- single nucleotide polymorphism
- genetics
- lipid-lowering therapy
- ezetimibe
- pcsk9 gene
- sensitivity analysis
- genome-wide association study
- apoc3 gene
- angptl3 gene
- mendelian randomization analysis
- bempedoic acid
- angiopoietin-like protein 3