-
PDF
- Split View
-
Views
-
Cite
Cite
Nicholas A Turner, Jay Krishnan, Alicia Nelson, Christopher R Polage, Ronda L Cochran, Lucy Fike, David T Kuhar, Preeta K Kutty, Rachel L Snyder, Deverick J Anderson, Assessing the Impact of 2-Step Clostridioides difficile Testing at the Healthcare Facility Level, Clinical Infectious Diseases, Volume 77, Issue 7, 1 October 2023, Pages 1043–1049, https://doi.org/10.1093/cid/ciad334
- Share Icon Share
Abstract
Two-step testing for Clostridioides difficile infection (CDI) aims to improve diagnostic specificity but may also influence reported epidemiology and patterns of treatment. Some providers fear that 2-step testing may result in adverse outcomes if C. difficile is underdiagnosed.
Our primary objective was to assess the impact of 2-step testing on reported incidence of hospital-onset CDI (HO-CDI). As secondary objectives, we assessed the impact of 2-step testing on C. difficile–specific antibiotic use and colectomy rates as proxies for harm from underdiagnosis or delayed treatment. This longitudinal cohort study included 2 657 324 patient-days across 8 regional hospitals from July 2017 through March 2022. Impact of 2-step testing was assessed by time series analysis with generalized estimating equation regression models.
Two-step testing was associated with a level decrease in HO-CDI incidence (incidence rate ratio, 0.53 [95% confidence interval {CI}, .48–.60]; P < .001), a similar level decrease in utilization rates for oral vancomycin and fidaxomicin (utilization rate ratio, 0.63 [95% CI, .58–.70]; P < .001), and no significant level (rate ratio, 1.16 [95% CI, .93–1.43]; P = .18) or trend (rate ratio, 0.85 [95% CI, .52–1.39]; P = .51) change in emergent colectomy rates.
Two-step testing is associated with decreased reported incidence of HO-CDI, likely by improving diagnostic specificity. The parallel decrease in C. difficile–specific antibiotic use offers indirect reassurance against underdiagnosis of C. difficile infections still requiring treatment by clinician assessment. Similarly, the absence of any significant change in colectomy rates offers indirect reassurance against any rise in fulminant C. difficile requiring surgical management.
(See the Editorial Commentary by Gerding on pages 1050–2.)
Clostridioides difficile infection (CDI) is a leading cause of healthcare-associated diarrhea, yet it is responsible for only a minority (4%–25%) of cases of hospital-onset diarrhea [1, 2]. Diagnosis of CDI is challenging, partly because the presence of C. difficile alone is generally insufficient to cause clinical disease. While healthy gut microbiota are protective against CDI, disruption caused by receipt of antibiotics facilitates C. difficile germination and toxin production, ultimately resulting in diarrheal disease [3]. Because traditional nucleic acid amplification testing (NAAT) only detects the presence of the C. difficile toxin genes rather than toxin production, C. difficile–associated disease can be overdiagnosed, which begets unnecessary antibiotic receipt and inaccurate classification of C. difficile colonization as disease for epidemiologic surveillance purposes.
Two-step testing, which combines a highly sensitive test (eg, NAAT or glutamate dehydrogenase) with a more specific assay for toxin production, has proven highly effective at identifying individuals with true disease. Prior cohort studies have demonstrated that nearly all significant CDI-related complications and deaths occur almost exclusively among toxin-positive individuals [4]. Nonetheless, some providers remain fearful of a missed or delayed diagnosis and the potential attendant consequences if an NAAT-positive/toxin-negative patient is not offered treatment but later progresses to C. difficile disease [5].
In this longitudinal cohort study, we sought to examine the effect of conversion to 2-step testing across multiple dimensions, including the effect on the epidemiology of hospital-onset CDI (HO-CDI), the utilization of antibiotics for treatment of CDI, and the rates of emergent colectomy among inpatients. We hypothesized that the greater specificity of 2-step testing would result in a decrease in reported HO-CDI incidence, as previously described by others [6]. Beyond the epidemiology, we also sought to assess CDI-specific antibiotic use and colectomy rates as proxies for safety of 2-step testing at the healthcare system level. If treatment could be avoided on the basis of 2-step testing, one would anticipate a parallel decline in rates of CDI-specific antibiotic use. Conversely, if clinical diagnoses of CDI were still made despite negative 2-step testing (eg, due to lack of clinical response to supportive care or lack of an alternative diagnosis), CDI-specific antibiotic use would not decrease in parallel with HO-CDI incidence inferred from 2-step testing. Finally, if 2-step testing failed to identify instances of true disease—and thus resulted in delays in treatment—one might expect an increase in risk of progression to fulminant CDI, which could be reflected in emergent colectomy rates. On the basis of prior cohort studies demonstrating the safety and effectiveness of 2-step testing [4], we hypothesized that conversion to 2-step testing would be temporally associated with a parallel decline in CDI-specific antibiotic use and no change in emergent colectomy rates.
METHODS
Data Collection
We conducted a multicenter longitudinal cohort study of admissions and CDI across 8 hospitals converting to 2-step C. difficile testing within the Duke Infection Control Outreach Network (DICON) from 1 July 2017 through 31 March 2022. Each hospital had a minimum of 12 months of data available before and after conversion from NAAT to 2-step testing (NAAT with reflex to toxin testing via enzyme-linked immunosorbent assay when positive). Hospital traits and follow-up periods before and after conversion of test protocols are displayed in Table 1. Analysis was performed from March 2022 through June 2022. DICON's surveillance methodology (specifically the informatics used to capture and analyze infection events) has been previously described [7]. The study design was reviewed by Duke University Health System's institutional review board and determined to be exempt, with waiver of consent for data collection. CDI was defined in accordance with the National Healthcare Safety Network LabID definition: Cases were identified by a positive laboratory test result for either a C. difficile toxin A/B assay or a positive laboratory test result by NAAT from stool samples submitted to each hospital's clinical microbiology laboratories for C. difficile. All sites used NAAT with reflex to toxin assay when NAAT was positive for their 2-step algorithm. For sites using 2-step testing, the result of the last test performed is used for C. difficile reporting purposes [8]. HO-CDI cases were defined as those occurring after hospital day 3. HO-CDI incidence rates were calculated monthly, as number of HO-CDI cases per 10 000 patient-days present. Any repeat positive samples sent within 14 days of a prior positive sample were considered to be duplicate samples from the same episode and were not counted separately. CDI-specific antibiotic use rates were gathered for oral vancomycin and oral fidaxomicin, the 2 primary recommended treatments for C. difficile in Infectious Diseases Society of America guidelines throughout the majority of the study period [9].
Hospital . | State . | Bed Capacity, No. . | Follow-up During NAAT-Only Period, m . | Follow-up During 2-Step Period, m . |
---|---|---|---|---|
1 | North Carolina | 212 | 32 | 25 |
2 | Virginia | 186 | 41 | 16 |
3 | North Carolina | 102 | 33 | 24 |
4 | North Carolina | 124 | 32 | 25 |
5 | North Carolina | 332 | 28 | 29 |
6 | North Carolina | 220 | 24 | 31 |
7 | West Virginia | 267 | 36 | 21 |
8 | North Carolina | 978 | 32 | 25 |
Hospital . | State . | Bed Capacity, No. . | Follow-up During NAAT-Only Period, m . | Follow-up During 2-Step Period, m . |
---|---|---|---|---|
1 | North Carolina | 212 | 32 | 25 |
2 | Virginia | 186 | 41 | 16 |
3 | North Carolina | 102 | 33 | 24 |
4 | North Carolina | 124 | 32 | 25 |
5 | North Carolina | 332 | 28 | 29 |
6 | North Carolina | 220 | 24 | 31 |
7 | West Virginia | 267 | 36 | 21 |
8 | North Carolina | 978 | 32 | 25 |
Abbreviation: NAAT, nucleic acid amplification testing.
Hospital . | State . | Bed Capacity, No. . | Follow-up During NAAT-Only Period, m . | Follow-up During 2-Step Period, m . |
---|---|---|---|---|
1 | North Carolina | 212 | 32 | 25 |
2 | Virginia | 186 | 41 | 16 |
3 | North Carolina | 102 | 33 | 24 |
4 | North Carolina | 124 | 32 | 25 |
5 | North Carolina | 332 | 28 | 29 |
6 | North Carolina | 220 | 24 | 31 |
7 | West Virginia | 267 | 36 | 21 |
8 | North Carolina | 978 | 32 | 25 |
Hospital . | State . | Bed Capacity, No. . | Follow-up During NAAT-Only Period, m . | Follow-up During 2-Step Period, m . |
---|---|---|---|---|
1 | North Carolina | 212 | 32 | 25 |
2 | Virginia | 186 | 41 | 16 |
3 | North Carolina | 102 | 33 | 24 |
4 | North Carolina | 124 | 32 | 25 |
5 | North Carolina | 332 | 28 | 29 |
6 | North Carolina | 220 | 24 | 31 |
7 | West Virginia | 267 | 36 | 21 |
8 | North Carolina | 978 | 32 | 25 |
Abbreviation: NAAT, nucleic acid amplification testing.
To support implementation of 2-step testing, infection preventionists from each hospital participated in a series of teleconference calls reviewing and discussing C. difficile testing algorithms. Each hospital was provided with a set of guidance documents offering suggested language for reporting test results and key points for educating staff and clinicians (provided in Supplementary Material S1). Each hospital was permitted to develop its own policies for reporting test results, educating clinicians, and conducting any parallel antimicrobial stewardship measures.
Statistical Modeling
We used generalized estimating equation (GEE) models for the interrupted time series analysis of outcomes to properly account for the violation of independence inherent in repeated measures data clustered by hospital site. Three separate models were created for HO-CDI incidence, CDI-specific antibiotic use, and colectomy rates each with patient-days present as an offset term. Consistent with time series methods suggested by Bernal et al [10], each model included a term for time (in months), test method (reflecting level change), and time since introduction of 2-step testing (reflecting slope change after introduction of 2-step testing). The variables for test method and time since introduction of 2-step testing were time-varying covariates specific to each hospital. All models were constructed using geepack in R software (version 4.1.2, https://www.r-project.org/). All graphs were created using ggplot2.
Data were assessed for evidence of zero-inflation (the more frequent occurrence of zero-valued observations than predicted) and overdispersion. Inspection of quantile-quantile plots suggested the Poisson distribution to be a reasonable assumption. Adjustment for zero-inflation did not improve model fit and did not markedly alter effect estimates. Fitted versus actual plots were constructed as a final visual inspection of each model's accuracy. Throughout this study, a significance threshold of .05 was used; 95% confidence intervals (CIs) were calculated by the Wald method.
As time series modeling can be complex and sensitive to assumptions regarding data distributions and correlation structures, we also constructed mixed-effects models analogous to the 3 main GEE models above as a sensitivity analysis. In mixed-effects modeling, time, test method, and time since introduction of 2-step testing were treated as fixed effects; hospital was treated as a random intercept effect and time as a random slope effect. A variety of correlation structures (exchangeable, compound symmetric, and autoregressive) were assessed.
RESULTS
Our cohort included data from 2 657 324 patient-days across 8 hospitals from July 2017 through March 2022. A total of 1052 cases of HO-CDI among 1 492 862 patient-days were captured prior to adoption of 2-step testing. Two hundred sixty-six cases of HO-CDI among 1 164 462 patient-days were captured after adoption of 2-step testing. On time series analysis, conversion to 2-step testing was temporally associated with a level change in HO-CDI incidence (incidence rate ratio [IRR], 0.53 [95% CI, .48–.60]; P < .001) but no significant trend change afterward (Figure 1, Table 2). The estimated effect of 2-step testing remained consistent through a range of mixed-effects models as well, indicating stability across multiple model structures and assumptions (see Supplementary Supplementary Material S2 and S3).
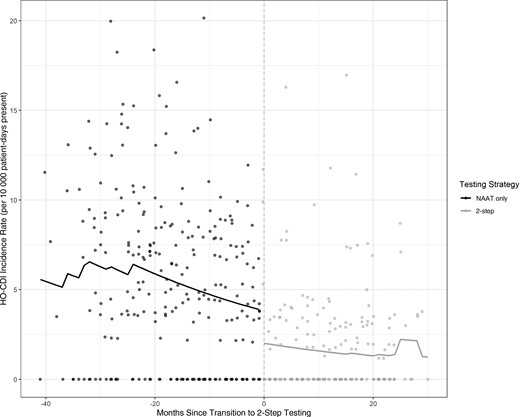
Hospital-onset Clostridioides difficile infection incidence rate by testing strategy. Abbreviations: HO-CDI, hospital-onset Clostridioides difficile infection; NAAT, nucleic acid amplification testing.
Time Series Analysis Results: Incidence Rate Ratios for Nucleic Acid Amplification Testing Versus 2-Step Clostridioides difficile Infection Confirmation
Intervention . | Intervention Effect . | IRRa (95% CI) . | P Value . |
---|---|---|---|
2-step CDI confirmation | Baseline trend | 0.80 (.69–.93) | .003 |
Level change | 0.53 (.48–.60) | <.001 | |
Slope change | 1.03 (.79–1.34) | .855 |
Intervention . | Intervention Effect . | IRRa (95% CI) . | P Value . |
---|---|---|---|
2-step CDI confirmation | Baseline trend | 0.80 (.69–.93) | .003 |
Level change | 0.53 (.48–.60) | <.001 | |
Slope change | 1.03 (.79–1.34) | .855 |
Abbreviations: CDI, Clostridioides difficile infection; CI, confidence interval; IRR, incidence rate ratio.
IRRs expressed per 12-month period.
Time Series Analysis Results: Incidence Rate Ratios for Nucleic Acid Amplification Testing Versus 2-Step Clostridioides difficile Infection Confirmation
Intervention . | Intervention Effect . | IRRa (95% CI) . | P Value . |
---|---|---|---|
2-step CDI confirmation | Baseline trend | 0.80 (.69–.93) | .003 |
Level change | 0.53 (.48–.60) | <.001 | |
Slope change | 1.03 (.79–1.34) | .855 |
Intervention . | Intervention Effect . | IRRa (95% CI) . | P Value . |
---|---|---|---|
2-step CDI confirmation | Baseline trend | 0.80 (.69–.93) | .003 |
Level change | 0.53 (.48–.60) | <.001 | |
Slope change | 1.03 (.79–1.34) | .855 |
Abbreviations: CDI, Clostridioides difficile infection; CI, confidence interval; IRR, incidence rate ratio.
IRRs expressed per 12-month period.
Complete antibiotic use data were available for 7 of the 9 sites. A total of 94 097 days of therapy (DOT) for oral vancomycin and 1305 DOT for fidaxomicin were available prior to conversion to 2-step testing, for a median treatment rate of 59.4 DOT/1000 patient-days present (interquartile range [IQR], 36.7–78.6). A total of 38 643 DOT for oral vancomycin and 1176 DOT of fidaxomicin were available after conversion to 2-step testing for a median monthly treatment rate of 29.8 DOT/1000 patient-days present (IQR, 20.4–46.6). On time series analysis, implementation of 2-step testing was temporally associated with a level change in CDI-specific antibiotic use (use rate ratio [RR], 0.64 [95% CI, .58–.70]; P < .001) with subsequent reductions in CDI-specific antibiotic use over time (use RR, 0.79 [95% CI, .69–.90]; P < .001 (Figure 2, Table 3).
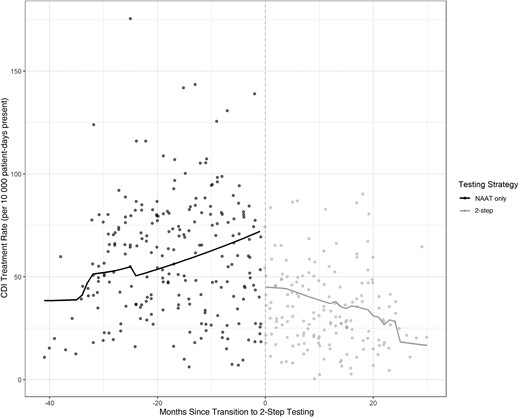
Clostridioides difficile infection treatment rate by testing strategy. Abbreviations: CDI, Clostridioides difficile infection; NAAT, nucleic acid amplification testing.
Time Series Analysis Results: Clostridioides difficile Infection (CDI) Treatment Rate Ratios for Nucleic Acid Amplification Testing Versus 2-Step CDI Confirmation
Intervention . | Intervention Effect . | Use Rate Ratioa (95% CI) . | P Value . |
---|---|---|---|
2-step CDI confirmation | Baseline trend | 1.04 (.99–1.09) | .122 |
Level change | 0.63 (.58–.70) | <.001 | |
Slope change | 0.79 (.69–.90) | <.001 |
Intervention . | Intervention Effect . | Use Rate Ratioa (95% CI) . | P Value . |
---|---|---|---|
2-step CDI confirmation | Baseline trend | 1.04 (.99–1.09) | .122 |
Level change | 0.63 (.58–.70) | <.001 | |
Slope change | 0.79 (.69–.90) | <.001 |
Abbreviations: CDI, Clostridioides difficile infection; CI, confidence interval.
Rate ratios expressed per 12-month period.
Time Series Analysis Results: Clostridioides difficile Infection (CDI) Treatment Rate Ratios for Nucleic Acid Amplification Testing Versus 2-Step CDI Confirmation
Intervention . | Intervention Effect . | Use Rate Ratioa (95% CI) . | P Value . |
---|---|---|---|
2-step CDI confirmation | Baseline trend | 1.04 (.99–1.09) | .122 |
Level change | 0.63 (.58–.70) | <.001 | |
Slope change | 0.79 (.69–.90) | <.001 |
Intervention . | Intervention Effect . | Use Rate Ratioa (95% CI) . | P Value . |
---|---|---|---|
2-step CDI confirmation | Baseline trend | 1.04 (.99–1.09) | .122 |
Level change | 0.63 (.58–.70) | <.001 | |
Slope change | 0.79 (.69–.90) | <.001 |
Abbreviations: CDI, Clostridioides difficile infection; CI, confidence interval.
Rate ratios expressed per 12-month period.
Colectomy data were available for 7 of 8 sites. Four hundred sixty-three emergent colectomies were captured prior to 2-step testing for a median rate of 3.8 colectomies per 10 000 patient-days present (IQR, 0.0–7.6). After adoption of 2-step testing, a total of 463 emergent colectomies were captured for a median rate of 3.9 colectomies per 10 000 patient-days present (IQR, 1.6–7.4). On time series analysis, 2-step testing was not associated with any significant level (RR, 1.16 [95% CI, .93–1.43]; P = .18) or trend (RR, 0.99 [95% CI, .95–1.03]; P = .51) change in emergent colectomy rates (Figure 3, Table 4).
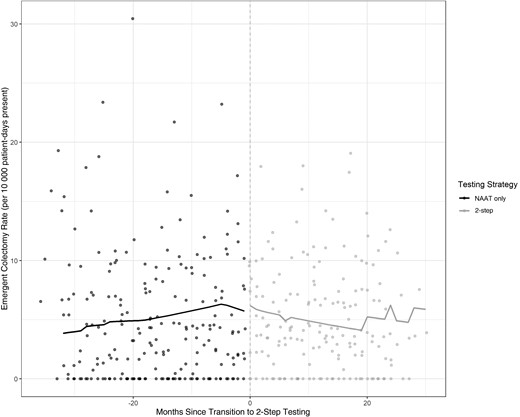
Emergent colectomy rate by testing strategy. Abbreviation: NAAT, nucleic acid amplification testing.
Time Series Analysis Results: Emergent Colectomy Rate Ratios for Nucleic Acid Amplification Testing Versus 2-Step Clostridioides difficile Infection Confirmation
Intervention . | Intervention Effect . | Colectomy Rate Ratioa (95% CI) . | P Value . |
---|---|---|---|
2-step CDI confirmation | Baseline trend | 1.11 (.79–1.56) | .535 |
Level change | 1.16 (.93–1.43) | .184 | |
Slope change | 0.85 (.52–1.39) | .509 |
Intervention . | Intervention Effect . | Colectomy Rate Ratioa (95% CI) . | P Value . |
---|---|---|---|
2-step CDI confirmation | Baseline trend | 1.11 (.79–1.56) | .535 |
Level change | 1.16 (.93–1.43) | .184 | |
Slope change | 0.85 (.52–1.39) | .509 |
Abbreviations: CDI, Clostridioides difficile infection; CI, confidence interval.
Rate ratios expressed per 12-month period.
Time Series Analysis Results: Emergent Colectomy Rate Ratios for Nucleic Acid Amplification Testing Versus 2-Step Clostridioides difficile Infection Confirmation
Intervention . | Intervention Effect . | Colectomy Rate Ratioa (95% CI) . | P Value . |
---|---|---|---|
2-step CDI confirmation | Baseline trend | 1.11 (.79–1.56) | .535 |
Level change | 1.16 (.93–1.43) | .184 | |
Slope change | 0.85 (.52–1.39) | .509 |
Intervention . | Intervention Effect . | Colectomy Rate Ratioa (95% CI) . | P Value . |
---|---|---|---|
2-step CDI confirmation | Baseline trend | 1.11 (.79–1.56) | .535 |
Level change | 1.16 (.93–1.43) | .184 | |
Slope change | 0.85 (.52–1.39) | .509 |
Abbreviations: CDI, Clostridioides difficile infection; CI, confidence interval.
Rate ratios expressed per 12-month period.
Given that the coronavirus disease 2019 (COVID-19) pandemic occurred in close temporal proximity to the change in test methodology for many participating sites, we conducted a post hoc sensitivity analysis assessing the potential for confounding effects related to the pandemic. While the COVID-19 pandemic was not temporally associated with any significant level or slope changes in HO-CDI incidence among participating sites, the potential for pandemic-related effects did introduce sufficient uncertainty that the effect of 2-step testing on HO-CDI incidence rates was partly mitigated (level change IRR, 0.68 [95% CI, .43–1.06]; Supplementary Material S4).
DISCUSSION
While conversion to 2-step testing is believed to improve diagnostic specificity for CDI, some providers fear the possibility of missing or delaying diagnosis of CDI with this approach. In assessing the effects of conversion to 2-step testing at the health system level, we examined trends in HO-CDI incidence, CDI-specific antibiotic use, and emergent colectomy rates as proxies for safety of 2-step testing.
Consistent with prior large-scale epidemiologic studies, implementation of 2-step testing was associated with a significant level reduction in HO-CDI rates [4]. A level change—effectively an immediate shift in incidence—is of course the anticipated result when a test method is changed at a discrete point in time. In this case, the decline is likely attributable to the higher specificity of 2-step testing, though participating hospitals may also have had active antibiotic stewardship and infection prevention efforts operating in parallel. Alternatively, some providers may worry the decline in incidence reflects lower test sensitivity and that 2-step testing could simply inhibit or delay diagnosis in nascent infection, in which case one would expect to see a lagged rebound in CDI incidence after implementation of 2-step testing; fortunately, the absence of trend (slope) changes for months following adoption of 2-step testing provides reassurance against any associated delays in CDI diagnosis.
Similarly, if clinicians observed significant numbers of colonized individuals (NAAT positive, toxin negative) progressing to symptomatic disease despite negative toxin testing, one might expect to see sustained rates of CDI-specific treatment following adoption of 2-step testing. We instead observed a parallel decline in overall CDI-specific antibiotic use (fidaxomicin and vancomycin) that was very similar in magnitude to the decline in HO-CDI incidence (though somewhat heterogenous across hospitals; see Supplementary Material S3). While the overall study relies on health system–level data, we did conduct a subgroup survey of patient-level prescription data across 3 participating hospitals to confirm that the observed decreases in CDI-specific antibiotic use resulted from clinicians appropriately withholding treatment from suspected colonized individuals. In fact, just 2154 of 13 486 (16%) individuals with NAAT-positive, toxin-negative results had a prescription for CDI-specific treatment (oral vancomycin or fidaxomicin) within 14 days of the index test. The absence of discordant treatment trends and low treatment rates among colonized individuals also supports the safety of 2-step testing.
Finally, as fulminant CDI sometimes requires colectomy in the worst of cases, we assessed emergent colectomy rates as a safety signal for the most severe of complications, should CDI progress with untimely or inadequate treatment. We observed no significant changes in emergent colectomy rates, either shortly after onset of 2-step testing or in the ensuing months—offering further reassurance against delayed diagnosis or progression to severe disease.
The COVID-19 pandemic has been variably associated with either no significant change in CDI incidence or, in some settings, a decrease in CDI incidence [11, 12].
The near co-occurrence of the COVID-19 pandemic with change in test method for many participating sites raises the possibility of pandemic-related changes in HO-CDI incidence acting as a confounder in our study. We consequently conducted a post hoc sensitivity analysis adding pandemic terms to our GEE model of HO-CDI incidence rates. While the COVID-19 terms were not associated with significant level or slope changes, the co-occurrence of the pandemic did introduce sufficient uncertainty that the effect of 2-step testing—while still associated with an estimated decrease—was mitigated in significance.
Our study does have several limitations. We conducted our analysis at the health system level, which permitted large-scale assessment without delving into individual-level private health data. However, the absence of individual patient-level outcomes assessment does leave room for some residual confounding. Along similar lines, the lack of individual-level data prevents analysis of other outcome measures such as time to treatment, C. difficile severity, or treatment failure. Additionally, while we focused on HO-CDI to capture the most relevant patient outcomes, it is possible that patients were discharged home before progression to CDI (or progression to severe CDI). Without any guarantee that subjects would return to the same hospital, we could theoretically underestimate outcomes among subjects who were later admitted to hospitals outside of our surveillance network. At the hospital level, we provided guidance on test implementation and interpretation but did not directly assess adherence to 2-step test interpretation. Finally, it is possible that hospitals might have made other changes to their approach to diagnosis and treatment of C. difficile during the study period or to antibiotic stewardship efforts focused on preventing C. difficile. Although unlikely to have occurred early enough to affect our results, lower recurrence rates with fidaxomicin may influence C. difficile incidence over time.
Despite these limitations inherent to health system–level longitudinal cohort studies, our analysis supplements prior literature on this subject with additional large-scale, external validation. We believe this study has several strengths. First, it draws on data from >2.6 million patient-days present across 8 different hospitals for the primary outcome (HO-CDI incidence) and 7 different hospitals for antibiotic use and colectomy rates. Additionally, the availability of at least 12 months of data before and after 2-step testing adoption gives a robust dataset for modeling purposes. Second, we conducted a careful time series analysis with GEE modeling to account for the violation of independence assumption and potential clustering by hospital inherent in repeated measures network data. As an added strength, all of our findings were robust to various modeling approaches, including mixed-effects with various correlation structures. Finally, the fact that all 3 outcomes assessed aligned with one another and preexisting cohort studies increases confidence in this nonrandomized study.
In conclusion, outcomes of 2-step testing at the healthcare system level mirror results from previous individual-level cohort studies. The absence of signal for harm through HO-CDI incidence rates, persistent or delayed increases in CDI-specific antibiotic use rates, or colectomy rates adds support to the safety of 2-step CDI testing strategies.
Supplementary Data
Supplementary materials are available at Clinical Infectious Diseases online. Consisting of data provided by the authors to benefit the reader, the posted materials are not copyedited and are the sole responsibility of the authors, so questions or comments should be addressed to the corresponding author.
Notes
Data availability. All authors had full access to all the data in the study and take responsibility for the integrity of the data and the accuracy of the data analysis. The content of this manuscript is original and has not been previously published. Individual hospital-level data are not publicly available, but summary data and the complete code developed for analysis are provided in Supplementary Material 3.
Disclaimer. The content of this work is solely the responsibility of the authors and does not necessarily represent the official views of the National Institutes of Health (NIH) or the Centers for Disease Control and Prevention (CDC).
Acknowledgments. The authors thank the infection preventionists at participating hospitals for assistance with data collection and for their hard work in implementing updating testing practices.
Financial support. This work was supported by a CDC SHEPheRD grant. N. A. T. was also supported in part by an Antibacterial Resistance Leadership Group (ARLG) Fellowship (National Institute of Allergy and Infectious Diseases award number UM1Al104681).
References
Author notes
Potential conflicts of interest. N. A. T. reports grant funding from the CDC, NIH/ARLG, and Rockefeller Foundation; research contract funding from PDI, Purio, and Basilea; and consulting fees for Techspert. D. J. A. reports grant funding from the CDC and Agency for Healthcare Research and Quality; contracts from CDC; interests as owner of Infection Control Education for Major Sports, LLC; and royalties from UpToDate. All other authors report no potential conflicts.
All authors have submitted the ICMJE Form for Disclosure of Potential Conflicts of Interest. Conflicts that the editors consider relevant to the content of the manuscript have been disclosed.