-
PDF
- Split View
-
Views
-
Cite
Cite
Linwei Yu, Weihan Wang, Zhiwei Li, Yi Ren, Jiabin Liu, Lan Jiao, Qiang Xu, Alexithymia modulates emotion concept activation during facial expression processing, Cerebral Cortex, Volume 34, Issue 3, March 2024, bhae071, https://doi.org/10.1093/cercor/bhae071
- Share Icon Share
Abstract
Alexithymia is characterized by difficulties in emotional information processing. However, the underlying reasons for emotional processing deficits in alexithymia are not fully understood. The present study aimed to investigate the mechanism underlying emotional deficits in alexithymia. Using the Toronto Alexithymia Scale-20, we recruited college students with high alexithymia (n = 24) or low alexithymia (n = 24) in this study. Participants judged the emotional consistency of facial expressions and contextual sentences while recording their event-related potentials. Behaviorally, the high alexithymia group showed longer response times versus the low alexithymia group in processing facial expressions. The event-related potential results showed that the high alexithymia group had more negative-going N400 amplitudes compared with the low alexithymia group in the incongruent condition. More negative N400 amplitudes are also associated with slower responses to facial expressions. Furthermore, machine learning analyses based on N400 amplitudes could distinguish the high alexithymia group from the low alexithymia group in the incongruent condition. Overall, these findings suggest worse facial emotion perception for the high alexithymia group, potentially due to difficulty in spontaneously activating emotion concepts. Our findings have important implications for the affective science and clinical intervention of alexithymia-related affective disorders.
Introduction
Alexithymia is a dimensional personality trait characterized by difficulties in identifying, describing, and introspecting emotional feelings (Taylor and Bagby 2013; Taylor et al. 2016). Approximately 10% of the general population exhibits symptoms of alexithymia (Berthoz et al. 2011). It leads to many maladaptive consequences, such as inflexible emotion regulation (Panayiotou et al. 2021). In fact, Alexithymia commonly co-occurs with several somatic and mental health problems, such as depression (Oakley et al. 2020; Preece et al. 2022), anxiety (Oakley et al. 2020), somatic symptom disorders (Cerutti et al. 2020), and self-injury behavior (Bordalo and Carvalho 2022). Therefore, it has been suggested that the alexithymia trait acts as a cross-diagnostic risk factor for emotional difficulties across psychiatric syndromes (Albantakis et al. 2020; Brewer et al. 2021).
Emotion processing deficits are considered a core symptom of alexithymia (Prkachin et al. 2009; van der Velde et al. 2013). Successfully decoding facial expressions is fundamental for social interaction, as expressions convey critical interpersonal signals about feelings, intentions, and motivations (Jack and Schyns 2015). However, growing studies demonstrated that individuals with high levels of alexithymia exhibit emotion processing deficits across attention, appraisals, memory, and language domains (Brewer et al. 2015a; Di Tella et al. 2020; Lee and Lee 2022; Leonidou et al. 2022; Luminet et al. 2021). For instance, those with alexithymia show reduced accuracy in identifying facial expressions (Ola and Gullon-Scott 2020), especially for low-intensity or ambiguous emotions (Brewer et al. 2015b; Grynberg et al. 2012; Parker et al. 2005; Reker et al. 2010). They also require longer presentation times and more emotional intensity to accurately recognize facial emotions (Grynberg et al. 2012; Starita et al. 2018). In fact, alexithymia involves processing problems beyond facial cues, impacting the interpretation of verbal (Goerlich-Dobre et al. 2014), music (Larwood et al. 2021), taste (Suslow and Kersting 2021), and contextual emotion information (Wang et al. 2021). In summary, alexithymia is associated with broad deficits in processing emotions across modalities.
Importantly, some studies illuminated the precise nature of these emotion processing impairments in alexithymia. For instance, alexithymia is correlated with reduced levels of emotional awareness (Maroti et al. 2018). Additionally, Rosenberg et al. (2020) found alexithymia features are related to less sensitivity for covert facial expressions of anger. At the neural level, those with high levels of alexithymia display low reactivity to barely visible negative emotion stimuli in regions like the amygdala, occipitotemporal cortex, and insula, which are responsible for emotional appraisal, encoding, and affective response generation (Donges and Suslow 2017). Thus, these findings suggest that alexithymia involves dysregulated automatic processing of emotional stimuli, reflected in the fundamental deficits in detecting emotional signals.
Although prior research establishes a link between alexithymia and emotional processing deficits, the underlying reasons require further study. The theory of constructed emotions proposes that emotions are not triggered automatically inside people simply by facial movements, but rather constructed based on conceptual knowledge (Barrett 2017; Lindquist 2017). This constructive process is supported by internal context within an observer’s mind (Russell and Barrett 1999; Lindquist and Barrett 2008; Hoemann et al. 2020). In particular, concept knowledge represents a crucial part of the internal context (Lindquist et al. 2006; Gendron et al. 2012). It refers to an individual’s understanding of various emotion categories (e.g. anger, disgust, fear), obtained through direct experience and language (Lindquist et al. 2015; Lee et al. 2017; Lindquist 2017). Such knowledge helps make sense of ambiguous affective states (e.g. unpleasant sensations) into discrete and specific emotion categories (e.g. anger) (Lindquist et al. 2015; Lindquist 2017; Hoemann et al. 2020). Growing evidence indicates that concept knowledge routinely activates during emotion perception (Gendron et al. 2012; Fugate et al. 2018; Betz et al. 2019; Wormwood et al. 2022). For instance, Fugate et al. (2018) demonstrated that accessible emotion concepts influenced the perceptual boundaries for categorizing facial expressions. Thus, the constructed emotion perspective may provide an important theoretical framework for understanding the emotion processing deficits in alexithymia.
The theory of constructed emotions proposes that emotion perception involves a conceptualization process (Lindquist et al. 2015; Lindquist 2017; Hoemann et al. 2020). The language hypothesis of alexithymia posits that language impairment disrupts the development of discrete emotion concepts from ambiguous affective states, leaving individuals unable to identify and describe their own feelings (Lee et al. 2022). Thus, in the case of alexithymia, deficits in conceptual knowledge around emotions may contribute to the poorer facial emotion perception frequently observed. Some studies link alexithymia to impair processing of emotion words. High alexithymia (HA) individuals generate fewer emotion-related terms to describe their experiences (Roedema and Simons 1999; Luminet et al. 2004; Wotschack and Klann-Delius 2013). For example, Wotschack and Klann-Delius (2013) found that HA individuals produce fewer types of emotion words than low alexithymia (LA) individuals in interviews, suggesting a diminished diversity in their emotion semantic space. Neuroimaging evidence also reports that alexithymia is robustly associated with brain damage in the pars triangularis subregion of the inferior frontal gyrus (IFG) and anterior insula (Hobson et al. 2018), which are regions consistently implicated in emotion word and semantic processing (Brooks et al. 2017). Language and emotion words are unambiguous representations of emotion concepts, effectively triggering conceptual knowledge (Lupyan and Thompson-Schill 2012; Lupyan and Clark 2015). Thus, such findings suggest that impaired activation of emotion concepts may contribute to the emotional processing deficits present in alexithymia.
Event-related potentials (ERPs) of the N400 component have been used to index conceptual knowledge activation (Kutas and Federmeier 2011; Hoemann et al. 2021). The N400 is a negative-going deflection component that peaks around 400 ms post-onset, and it received widespread attention for its sensitivity to semantic mismatch (Kutas and Federmeier 2011; Dudschig et al. 2016). Recent studies have also reported N400 effects in response to emotional incongruities between facial expressions or emojis and contextual cues (Tang et al. 2020; Yu et al. 2022). For example, Yu et al. (2022) report more negative N400 amplitudes for both facial expressions and facial emojis when presented in emotionally incongruent versus congruent contexts. Such findings indicate that conceptual knowledge is recruited to construct emotional meaning from faces. Furthermore, it appears more difficult to access emotion concepts in the emotionally inconsistent condition without a coherent contextual background. Therefore, if alexithymia facial expression processing deficits relate to problems activating emotion concepts, high-alexithymia individuals may be expected to show more negative N400 amplitudes when processing facial expression, particularly in contexts lacking congruent emotion concepts.
The present study used ERP technology to investigate facial expression processing in alexithymia. We hypothesized that (i) HA exhibits poorer performance in emotion perception than LA, as evidenced by slower response times (RTs) or lower accuracy (ACC); (ii) the N400 amplitudes elicited by facial expressions would be significantly more negative in the emotion incongruent condition compared with the emotion congruent condition (i.e. the N400 effects of emotional violation); (iii) the N400 amplitudes elicited by facial expressions would be modulated by alexithymia levels, with HA showing more negative N400 amplitudes than LA.
Materials and methods
Participants
We conducted a power analysis using G*Power 3.1 (Faul et al. 2007). Based on a medium effect size (f = 0.25), the analysis estimated that a total sample of 46 participants would provide 90% power to detect a within-between interaction effect. We recruited 401 college students to complete the Chinese version of the Toronto Alexithymia Scale (TAS-20; Bagby et al. 1994; Zhu et al. 2007). Following the previous study, participants were categorized into 2 groups based on their TAS-20 scores. Participants with TAS-20 scores ≥ 61 were categorized as HA group (Di Tella et al. 2020; Wang et al. 2021), which accounted for 14.70% of the total pool in the present study. Those with TAS-20 scores ≤ 51 were categorized as LA group (Di Tella et al. 2020; Wang et al. 2021), constituting 50.90% of the pool. We sequentially invited HA participants from the highest TAS-20 scores down to 61. Similarly, LA participants were sequentially invited from the lowest scores up to 51.
Before the main experiment, participants were instructed to complete a set of scales including the Beck Depression Inventory (BDI), Beck Anxiety Inventory (BAI), and Short Autism Spectrum Quotient (AQ-10). All participants included in the final sample satisfied the following criteria: (i) no history of substance abuse and psychiatric diseases; (ii) no serious depression or high anxiety, as indicated scores lower than 13 on the BDI (Beck et al. 1996) and lower than 45 on the BAI (Beck et al. 1993); (iii) no typical autistic traits, as indicated by scores lower than 6 on the AQ-10 (Allison et al. 2012).
In total, 49 college students were recruited to participate in the experiment. One participant was excluded due to excessive artifacts in the electroencephalogram (EEG) data. The final sample consisted of 24 participants in the HA group (12 females, Mage = 20.25, SD = 2.09) and 24 participants in the LA group (13 females, Mage = 20.63, SD = 2.46). The 2 groups did not significantly differ in gender ratio [χ2(1) = 0.08, P > 0.05], age, BDI, BAI, and AQ-10 scores, with details on these characteristics presented in Table 1. All participants were right-handed and had normal or corrected-to-normal vision. All participants provided informed consent before participation and received financial compensation. Ethical approval for this study was obtained from the ethics committee of the corresponding author’s affiliated institution.
Variables . | HA (n = 24, 12 females) . | LA (n = 24, 13 females) . | t . | P . |
---|---|---|---|---|
Age | 20.25 (2.09) | 20.63 (2.46) | 0.57 | >0.05 |
BDI | 4.29 (3.87) | 3.17 (3.73) | 1.02 | >0.05 |
BAI | 4.75 (4.12) | 4.45 (4.54) | 0.23 | >0.05 |
AQ-10 | 3.25 (1.26) | 3.04 (1.63) | 0.50 | >0.05 |
TAS-20 | 68.46 (3.13) | 33.83 (3.74) | 34.77 | <0.001 |
Variables . | HA (n = 24, 12 females) . | LA (n = 24, 13 females) . | t . | P . |
---|---|---|---|---|
Age | 20.25 (2.09) | 20.63 (2.46) | 0.57 | >0.05 |
BDI | 4.29 (3.87) | 3.17 (3.73) | 1.02 | >0.05 |
BAI | 4.75 (4.12) | 4.45 (4.54) | 0.23 | >0.05 |
AQ-10 | 3.25 (1.26) | 3.04 (1.63) | 0.50 | >0.05 |
TAS-20 | 68.46 (3.13) | 33.83 (3.74) | 34.77 | <0.001 |
Variables . | HA (n = 24, 12 females) . | LA (n = 24, 13 females) . | t . | P . |
---|---|---|---|---|
Age | 20.25 (2.09) | 20.63 (2.46) | 0.57 | >0.05 |
BDI | 4.29 (3.87) | 3.17 (3.73) | 1.02 | >0.05 |
BAI | 4.75 (4.12) | 4.45 (4.54) | 0.23 | >0.05 |
AQ-10 | 3.25 (1.26) | 3.04 (1.63) | 0.50 | >0.05 |
TAS-20 | 68.46 (3.13) | 33.83 (3.74) | 34.77 | <0.001 |
Variables . | HA (n = 24, 12 females) . | LA (n = 24, 13 females) . | t . | P . |
---|---|---|---|---|
Age | 20.25 (2.09) | 20.63 (2.46) | 0.57 | >0.05 |
BDI | 4.29 (3.87) | 3.17 (3.73) | 1.02 | >0.05 |
BAI | 4.75 (4.12) | 4.45 (4.54) | 0.23 | >0.05 |
AQ-10 | 3.25 (1.26) | 3.04 (1.63) | 0.50 | >0.05 |
TAS-20 | 68.46 (3.13) | 33.83 (3.74) | 34.77 | <0.001 |
Stimuli
The stimuli were adopted from a previous study (Yu et al. 2022). Facial expression stimuli were taken from the NimStim Picture System (Tottenham et al. 2009), comprising 6 angry and 6 happy facial expressions. The contextual material consisted of 60 sentences, including 30 happy (e.g. “I had a delicious meal”) and 30 angry (e.g. “I was splashed by a car”) sentences. Emotional valence and arousal levels were matched between the happy and angry facial expressions. Additionally, valence, arousal, and comprehensibility levels were matched between the happy and angry sentences (see Yu et al. 2022 for details of the results based on scoring measures from 2 independent samples).
Procedure
Drawing on prior works showing emotion incongruency effects in sentences and faces (Schauenburg et al. 2019; Tang et al. 2020; Yu et al. 2022), we employed an emotional consistency judgment task combining affective sentences and facial expressions to directly probe the activation of conceptual knowledge during facial emotion perception (Hoemann et al. 2021). This paradigm allows examination of whether HA individuals show differences in leveraging conceptual information from facial expressions. The procedure consisted of 4 conditions with 60 trials per condition, totaling 240 trials. Trials were randomly presented for each participant, with a break provided every 80 trials. In each trial, a contextual sentence was combined with a facial expression using the phrase “I feel” to form a complete sentence. This sentence was divided into 5 segments based on semantic meaning, and these segments were presented one by one in the center of the screen.
Specifically, each trial began with a fixation cross displayed for 1200 ms, followed by sequential presentation of the 5 segmented sentence parts (800 ms each). A blank screen was presented for 500 ms between segments and 800 ms before the facial expression. The facial expression was then presented, followed by a randomized inter-trial interval (ITI) of 1000–1200 ms. Participants were instructed to judge the emotional consistency between the facial expression and the sentence context by pressing “F” for emotion-congruent or “J” for incongruent (refer to Fig. 1). The response keys were counterbalanced across participants. Before the formal experiment, participants completed 8 practice trials with stimuli not used in the formal experiment. Participants were encouraged to respond as quickly and accurately as possible. Facial expressions were presented centrally on a gray background, subtending 9.50° × 10.97° visual angle at the viewing distance of 80 cm.
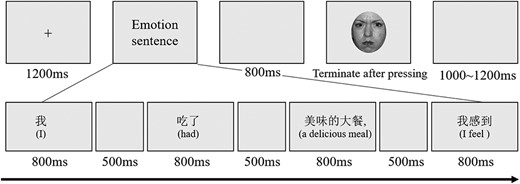
Experimental procedure. Each trial began with a fixation cross, followed by a segmented presentation of a contextual sentence. After a blank delay, a facial expression stimulus was presented. Participants indicated whether the facial expression was emotionally congruent or incongruent with the preceding contextual sentence by button press.
EEG recording and preprocessing
E-Prime 3.0 software was used to present stimulus and record behavioral responses. EEG data were recorded from 64 scalp sites based on the 10–20 international system, using Brain Vision Recorder software (Brain Products GmbH, Munich, Germany), with online referencing to the FCz site. Electrode impedances were kept below 10 kΩ. Signals were recorded continuously with a digitization sampling rate of 1000 Hz.
EEG data were analyzed in MATLAB (MathWorks, Natick, Massachusetts, United States of America) using EEGLAB (Delorme and Makeig 2004) and ERPLAB (Lopez-Calderon and Luck 2014). Data were notch filtered at 49–51 Hz to eliminate the 50-Hz interference. Continuous data were high-pass filtered at 0.1 Hz and low-pass filtered at 40 Hz. The data were then segmented into 1000 ms epochs, starting 200 ms before facial expression onset. Next, artifacts were corrected using independent component analysis (ICA). Contaminated epochs were removed via computerized artifact rejection with a peak-to-peak threshold (± 100 μV). The data were then re-referenced offline to the average of the left and right mastoids. Only trials that responded correctly were included in the analysis. On average, over 85% of trials per condition were retained after preprocessing. The mean amplitudes of the 200 ms window before onset were subtracted to correct for baseline differences. Based on previous studies (Kuipers and Thierry 2011; Tarantino et al. 2014), N400 was quantified as the mean amplitude from 300–500 ms post-stimulus onset, averaged across a center-frontal electrode cluster (F3, Fz, F4, FC3, FC4, C3, Cz, C4).
Statistical analyses
Statistical analyses were conducted in IBM SPSS Statistics software (Version 19; SPSS INC, IBM Company, Chicago, Illinois, United States of America). The behavioral performance and EEG response results were submitted to a 2 (Group: HA, LA) × 2 (Contextual emotion: angry, happy) × 2 (Emotion congruency: congruent, incongruent) repeated-measures ANOVA. Greenhouse–Geisser corrections were applied when Mauchly’s test indicated a violation of sphericity. Bonferroni corrections were applied to correct the P-values for multiple comparisons.
We investigated the predictive value of N400 amplitudes in distinguishing HA individuals and LA individuals, utilizing a machine learning algorithm (i.e. decision tree). Specifically, N400 amplitudes served as the classification feature, and the alexithymia group (HA vs. LA) was the classification label. Decision tree is a supervised machine-learning algorithm that has been previously used to predict affective disorders (e.g. depression) with EEG data (Mahato and Paul 2019). We employed a 10-fold cross-validation approach, conducted separately for congruent (angry-congruent, happy-congruent) and incongruent (angry-incongruent, happy-incongruent) conditions, to validate the analysis. The classification performance was evaluated by classification accuracy scores (ACC) and area under the receiver operating characteristic curve (AUC), which have been used in previous studies (Mahato and Paul 2019; Khare and Acharya 2023). Finally, a permutation test determined the statistical significance of the obtained ACC compared with chance.
Results
Behavioral performance
Response times
Incorrect responses and RTs exceeding 3 standard deviations from condition means were excluded; on average, 7.71% of the trials were removed. The 3-way ANOVA revealed a main effect of Group, F (1, 46) = 6.39, P = 0.015, ηp2 = 0.12, HA showed significantly longer RTs than LA (MHA = 905.43 ms, SE = 34.09; MLA = 783.46 ms, SE = 34.09).
The results revealed significant main effects of Contextual emotion [F (1, 46) = 56.33, P < 0.001, ηp2 = 0.55] and Emotion congruency [F (1, 46) = 8.67, P = 0.005, ηp2 = 0.16], as well as a significant interaction effect between Contextual emotion and Emotion congruency [F (1, 46) = 91.20, P < 0.001, ηp2 = 0.67]. Further analysis showed that RTs were longer for the emotion-congruent condition (M = 923.30 ms, SE = 28.65) than for the emotion-incongruent condition (M = 857.78 ms, SE = 26.95) in the angry context. Conversely, RTs were longer for the emotion incongruent condition (M = 862.94 ms, SE = 22.46) than for the emotion congruent condition (M = 733.77 ms, SE = 25.39) in the happy context (see Fig. 2).
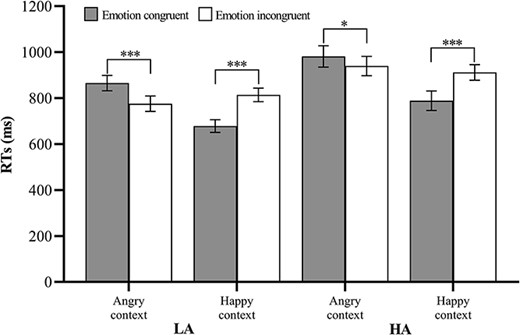
Mean RTs (ms) and standard error (SE) correspond to the different facial expressions and context conditions. Note. *P < 0.05, ***P < 0.001.
Accuracy rates
For the analysis of ACC, the interaction effect of Contextual emotion and Emotion congruency was significant, F (1, 46) = 7.24, P = 0.01, ηp2 = 0.14. Further analysis showed that ACC rates were higher for the emotion-incongruent condition (M = 0.967, SE = 0.006) than the emotion-congruent condition (M = 0.949, SE = 0.006; P = 0.004) in the angry context, whereas the difference between the emotion congruent condition (M = 0.960, SE = 0.009) and the emotion incongruent condition was not significant (M = 0.944, SE = 0.011; P > 0.05) in the happy context. Other main effects and interactions did not reach statistical significance (Ps > 0.05).
EEG results
N400 component
The results showed a main effect for Group, F (1, 46) = 5.22, P = 0.027, ηp2 = 0.10, HA showed a significantly more negative-going N400 amplitude than LA (MHA = 1.35 μV, SE = 0.87; MLA = 4.14 μV, SE = 0.87).
The results also revealed a significant interaction effect among Group and Emotion congruency, F (1, 46) = 5.25, P = 0.027, ηp2 = 0.10. Based on further analyses, the N400 amplitudes for emotion incongruent condition were more negative-going than for emotion congruent condition both in HA (Incongruent: M = 0.42 μV, SE = 0.87; Congruent: M = 2.28 μV, SE = 0.88; P < 0.001) and LA group (Incongruent: M = 3.69 μV, SE = 0.87; Congruent: M = 4.60 μV, SE = 0.88; P = 0.003). Critically, HA showed significantly more negative-going N400 amplitudes than LA in emotion-incongruent conditions (MHA = 0.42 μV, SE = 0.87; MLA = 3.69 μV, SE = 0.87; P = 0.011), but not for emotion congruent condition (MHA = 2.28 μV, SE = 0.88; MLA = 4.60 μV, SE = 0.88; P > 0.05).
The interaction effect of Contextual emotion and Emotion congruency was significant, F (1, 46) = 9.85, P = 0.003, ηp2 = 0.18. Further analysis showed that N400 amplitudes for emotion incongruent condition were more negative-going than for emotion congruent condition both in happy (Incongruent: M = 2.04 μV, SE = 0.62; Congruent: M = 3.91 μV, SE = 0.63; P < 0.001) and angry context (Incongruent: M = 2.06 μV, SE = 0.64; Congruent: M = 2.98 μV, SE = 0.63; P = 0.001). In addition, N400 amplitudes for the angry context were more negative-going than the happy context in emotion congruent condition (MAngry = 2.98 μV, SE = 0.64; MHappy = 3.91 μV, SE = 0.63; P < 0.001), but the difference between angry and happy context was not significant in emotion incongruent condition (MAngry = 2.06 μV, SE = 0.64; MHappy = 2.04 μV, SE = 0.62; P > 0.05; see Fig. 3).
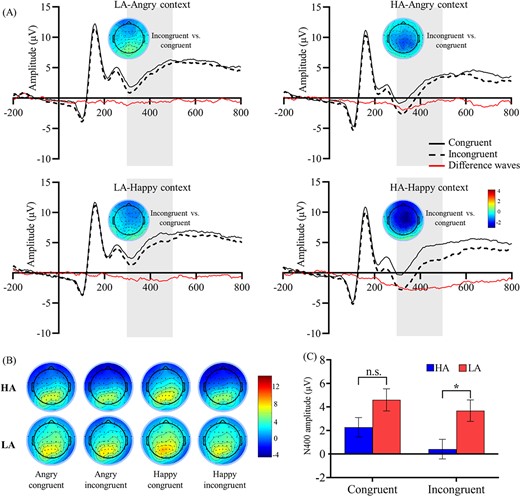
The grand-average ERPs and scalp topographic distribution. (A) Grand-averaged ERPs for the N400 ROIs (F3, Fz, F4, FC3, FC4, C3, Cz, C4) in each condition. (B) Scalp topographic distribution of N400 amplitudes and N400 difference waves from 300 to 500 ms in each condition. (C) N400 amplitudes results of Group × Emotion congruency interaction effects (error bars indicate standard errors). Note. Shaded areas correspond to the time window for the N400 (300–500 ms).
N400 difference waves
Mean amplitudes were calculated for the N400 difference waves (emotion incongruent condition minus emotion congruent condition) and submitted to a 2-way repeated-measures ANOVA with factors of Group (HA vs. LA) and Contextual emotion (angry vs. happy). Results showed a significant main effect of Group, F (1, 46) = 5.25, P = 0.027, ηp2 = 0.10, the HA exhibited a significantly larger N400 effect than LA (MHA = −1.86 μV, SE = 0.29; MLA = −0.92 μV, SE = 0.29). The main effect of Contextual emotion was significant, F (1, 46) = 9.85, P = 0.003, ηp2 = 0.18, N400 effect for happy context (M = −1.86 μV, SE = 0.27) was larger than for angry context (M = −0.92 μV, SE = 0.25; see Fig. 3).
N400 amplitudes and RTs correlations
Correlation analyses revealed significant negative relationships between N400 amplitude and reaction times (RTs) across conditions. Specifically, in the angry context, more negative N400 amplitudes correlated with slower RTs for both emotionally congruent and incongruent conditions. In the happy context, more negative N400 amplitudes were linked to slower RTs during congruent trials, with a marginally significant association for the incongruent condition (see Fig. 4). These results indicate that more negative N400 amplitudes were associated with slower responses to emotional expressions.
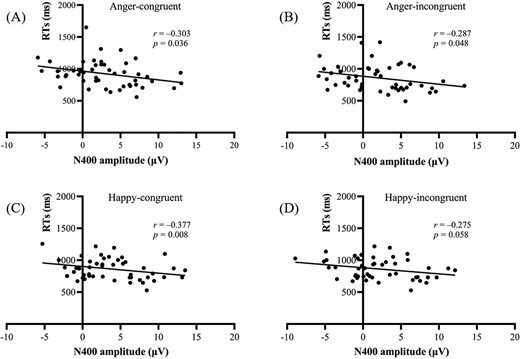
Association between N400 amplitudes and RTs. Correlations between N400 amplitudes and RTs to facial expressions in the (A) angry-congruent, (B) angry-incongruent, (C) happy-congruent, and (D) happy-incongruent conditions.
Prediction of alexithymia based on N400 data
The current study examined whether a decision tree algorithm based on N400 amplitude features could distinguish HA versus LA individuals. The analysis successfully distinguished HA from LA with high accuracy (ACC = 0.80, P = 0.006) and an AUC of 0.83 under incongruent condition. However, the classifier was insufficient to reliably distinguish HA and LA in the congruent condition (AUC = 0.60, ACC = 0.40, P > 0.05). These findings suggest the potential utility of N400 responses as capable of identifying alexithymia, particularly in conditions of emotional incongruity (see Fig. 5).
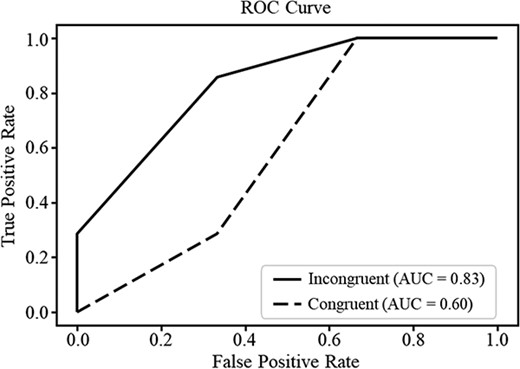
N400 amplitudes prediction of HA or LA. The receiver operating characteristic (ROC) curves for classification of HA versus LA based on N400 amplitude features in the congruent and incongruent conditions.
Discussion
The current study examined the facial expression processing in alexithymia using behavioral and ERP measures. Behavioral results found that the HA showed longer RTs to facial expression versus the LA. ERP results revealed more negative-going N400 amplitudes for HA compared with LA in the incongruent condition. More negative N400 amplitudes were associated with slower responses to emotional expressions. The decision tree classifier based on N400 amplitudes could reliably distinguish HA from LA in the incongruent condition. Collectively, the findings suggest that individuals with alexithymia involve deficits in emotion perception, potentially due to difficulty in activating emotion concepts spontaneously.
The results demonstrated faster facial expression recognition of congruent versus incongruent conditions in the happy context. This aligns with previous work showing that semantic and conceptual congruency facilitates facial expression processing through top-down effects (e.g. Yu et al. 2022). Interestingly, the opposite pattern occurred within an angry context, with shorter RTs in the incongruent condition. The findings may relate to faster recognition of happy faces than angry faces. Indeed, prior research shows processing advantages for happy faces in similar tasks (Dieguez-Risco et al. 2015; Aguado et al. 2019; Yu et al. 2022), potentially reflecting positivity advantages in expression recognition (Nummenmaa and Calvo 2015). Such an advantage may arise because happy expressions carry intrinsic reward value and hold relevance for adaptive social functioning (Calvo and Beltrán 2013; Nummenmaa and Calvo 2015; Yuan et al. 2019). From an evolutionary perspective, rapidly detecting happy faces could promote approach motivation and social bonding.
Importantly, we observed that HA performed longer RTs than LA in facial expression processing. This result indicates that HA exhibited a poorer ability to recognize facial expressions compared with LA. Previous studies have also found that HA shows poorer emotion processing abilities (Brewer et al. 2015a, 2015b; Di Tella et al. 2020), as evidenced by reduced emotion awareness (Maroti et al. 2018), as well as deficits in the automatic and unconscious processing of emotional information (Donges and Suslow 2017; Rosenberg et al. 2020). However, most of these studies used isolated emotional expressions devoid of context. In daily life, facial expressions naturally arise embedded within surrounding contexts (Xu et al. 2015). For instance, happy expressions typically appear in the conversation of receiving praise; sad faces appear in sickrooms more often than at birthday parties. Thus, our study extends prior results by demonstrating impaired facial emotion recognition in alexithymia persisted even when facial expressions appear in a supportive semantic context. This provides behavioral evidence that alexithymia individuals associate with struggles in understanding others’ emotions from facial expressions during social interactions.
The present study found that the HA group shows more negative-going N400 amplitudes than the LA group in emotion-inconsistent conditions, but not in emotion-consistent conditions. More negative N400 amplitudes were associated with slower expression recognition. Furthermore, a decision tree classifier based on N400 amplitudes distinguished HA from LA individuals in the incongruent condition. Previous studies have shown that emotional incongruities elicit more negative N400 amplitudes (Tang et al. 2020; Yu et al. 2022), reflecting difficulties in accessing conceptual knowledge (Kutas and Federmeier 2011; Dudschig et al. 2016). In our paradigm, individuals completed judgments about the emotional congruency between sentences and facial expressions, requiring constructing meaning from the faces. This process involves activating emotion concepts, with more negative N400 reflecting greater effort in conceptual recruitment. The semantic context in emotionally congruent conditions appears to support emotion concept access (Shablack et al. 2020). Thus, our findings indicate that HA individuals expend greater effort to retrieve conceptual knowledge from facial expressions in the absence of semantically consistent context. More broadly, the result suggests that deficits in spontaneously activating emotion concepts without contextual scaffolding may be a key characteristic of alexithymia. Such finding is supported by a prior work (Nook et al. 2015), which found selective deficits in alexithymia for face-face but not face-word emotion judgments. Our findings extend previous behavioral evidence (Nook et al. 2015) by demonstrating difficulties with flexible concept activation in alexithymia based on direct neurophysiological evidence, providing key insights into cognitive mechanisms behind their facial emotion processing deficits.
Prior evidence indicates that emotional information processing varies as a function of individual differences. For example, Lee et al. (2017) found that individual differences in accessing and utilizing conceptual knowledge influenced the emotional experience. Additionally, Lindquist et al. (2014) showed that patients with semantic dementia, who exhibited substantial semantic processing deficits, failed to spontaneously perceive discrete emotions like anger, disgust, fear, or sadness. Taken together with these studies, the present study suggests that a person’s emotional perception or experience is ultimately limited by their ability to access and use conceptual knowledge.
The present study has some theoretical and clinical implications. First, our findings contribute to the growing evidence supporting the theory of constructed emotions. Prior work has shown that increased accessibility to emotion concepts facilitates emotion judgments (Gendron et al. 2012; Fugate et al. 2018), while reduced access to emotion concepts impairs emotion judgments (Lindquist et al. 2006). Our results support these claims and extend these findings by linking individual differences in emotion perception to variability in activating emotion concepts. This provides an explanation for the underlying cause of alexithymia, i.e. alexithymia associated with deficits in the retrieval of emotion concepts. This aligns with the language hypothesis of alexithymia (Hobson et al. 2019; Lee et al. 2022), which suggests that alexithymia’s language deficits may limit the conceptual representation of emotion into discrete categories (Lee et al. 2022). Based on this hypothesis, this deficit may be more pronounced during subtle or contradictory contexts, as such contexts require more complex and nuanced conceptual information. Indeed, our results confirmed that individuals with alexithymia have more difficulty in activating emotion concepts when confronted with inconsistent context. Thus, this result provides empirical evidence for the language hypothesis of alexithymia.
Second, the findings have implications for identifying and understanding individuals with alexithymia and related affective disorders. Specifically, the decision tree classifier based on N400 amplitudes successfully distinguished between HA and LA groups. This non-invasive and objective measure has the potential to facilitate earlier detection of individuals at risk for psychological disorders associated with alexithymia. Prior work identified the insula’s structural integrity and frontal EEG asymmetry patterns as biomarkers of alexithymia (Flasbeck et al. 2017; Hogeveen et al. 2016; Valdespino et al. 2017). For example, alexithymia is associated with decreased gray matter insula volume (Ihme et al. 2013). By directly indexing semantic retrieval of emotion concepts, N400 activity provides a process-specific indicator sensitive to transient state changes relevant to emotion perception. This shows the potential utility of applying EEG data to develop neural metrics forecasting emotion perception capacities. However, additional research across various samples is still needed before conclusions about the predictive utility of N400.
Third, on the neural level, our study highlights the important role of semantic processing in facial expression perception. The enhanced N400 incongruity effects observed in the HA group provide electrophysiological evidence that these individuals have greater difficulty spontaneously activating conceptual knowledge around emotions. The N400 response, one of the best-studied ERP components associated with semantic processing (Kutas and Federmeier 2011; Dudschig et al. 2016; Hoemann et al. 2021), involves distributed semantic networks comprising temporal and prefrontal areas (Lau et al. 2008). Thus, the increased N400 amplitudes likely reflect the recruitment of compensatory semantic processing in higher-order regions to make sense of emotionally incongruent expressions in alexithymia individuals. This finding aligns with emerging models distinguishing between bottom-up perceptual pathways (e.g. occipital face areas) and top-down routes engaging higher-order regions like IFG and middle frontal gyrus (MFG) in facial processing (Liu et al. 2021). As Brooks et al. (2017) observed, explicit access to emotion concepts elicits robust engagement of IFG, superior temporal, and middle temporal areas in emotion perception tasks. These regions fall within an interconnected network representing semantic knowledge (Visser et al. 2010). Collectively, the current findings suggest that semantic networks play an important top-down role during emotion perception.
Finally, these findings also provide insight into the neurocognitive mechanisms underlying the development of alexithymia. The language hypothesis of alexithymia suggests that the link between alexithymia and emotion perception may be the result of a disruption in the conceptualization of ambiguous emotional states (Lee et al. 2022). Given that alexithymia is comorbid with a host of psychological disorders (Cook et al. 2013; Oakley et al. 2020; Preece et al. 2022), such as autism, training to enhance the acquisition and flexible utilization of emotion concepts may improve their ability to recognize others’ emotions.
Several limitations of the present study should be noted. First, the correlational nature of our cross-sectional data limits the inferences about causation. Longitudinal designs would be useful in elucidating the mechanistic pathways linking alexithymia and emotion conceptual knowledge. Second, we used a general college students sample rather than a psychiatric sample. Future studies would usefully extend this work by replicating it with a more representative clinical sample.
Conclusions
The present study investigated the effects of alexithymia on neural responses during facial expression processing. Behavioral evidence indicated that individuals with high levels of alexithymia performed poorer in facial expression processing compared with those with low levels of alexithymia. The difficulty observed in the HA individuals may be attributed to their difficulty in spontaneously activating emotion concepts. The findings have important implications for understanding the mechanisms of emotion deficits in alexithymia-related affective disorders.
Author contributions
Qiang Xu and Lan Jiao conceived the work. Linwei Yu draft the original manuscript. Linwei Yu, Qiang Xu, and Lan Jiao revised the manuscript. Linwei Yu, Weihan Wang, Zhiwei Li, Yi Ren, and Jiabin Liu contributed to the data acquisition and data analyses. All authors approved the final version of the manuscript.
Funding
This study was supported by the Humanities and Social Sciences Fund of Ministry of Education of China (grant number: 18YJC190027).
Conflict of interest statement: None declared.