-
PDF
- Split View
-
Views
-
Cite
Cite
Lulu Cheng, Hongyu Xi, Haiyan Gu, Yanyan Gao, Su Hu, Mengting Li, Zeqi Hao, Jianjie Wen, Jianxin Zhang, Yating Lv, Linlin Zhan, Xize Jia, Abnormalities of regional spontaneous brain activity in poststroke aphasia: a meta-analysis, Cerebral Cortex, Volume 33, Issue 12, 15 June 2023, Pages 7771–7782, https://doi.org/10.1093/cercor/bhad078
- Share Icon Share
Abstract
Poststroke aphasia is an acquired language disorder and has been proven to have adverse effects on patients’ social skills and quality of life. However, there are some inconsistencies in the neuroimaging studies investigating poststroke aphasia from the perspective of regional alterations. A meta-analysis has been employed to examine the common pattern of abnormal regional spontaneous brain activity in poststroke aphasia in the current study. Specifically, the Anisotropic effect-size version of seed-based d mapping was utilized, and 237 poststroke aphasia patients and 242 healthy controls (HCs) from 12 resting-state functional magnetic resonance imaging studies using amplitude of low-frequency fluctuations (ALFF), fractional ALFF, or regional homogeneity were included. The results showed that compared with HCs, patients with poststroke aphasia demonstrated increased regional spontaneous brain activity in the right insula, right postcentral gyrus, left cerebellar lobule IX, left angular gyrus, right caudate nucleus, left parahippocampal gyrus, and right supplementary motor area, and decreased regional spontaneous brain activity in the left cerebellar lobule VI, left median cingulate and paracingulate gyri, right cerebellar crus I, and left supplementary motor area. The study could provide further evidence for pathophysiological mechanism of poststroke aphasia and help find targets for treatment.
Introduction
Stroke is the second-leading cause of death and disability in the world (Bonita et al. 2004; Feigin et al. 2022; Thayabaranathan et al. 2022) and one-third of stroke survivors suffering from aphasia are termed as patients with poststroke aphasia (Flöel 2019). Poststroke aphasia impacts individuals’ language systems such as phonological system (Alyahya et al. 2022; Ziegler et al. 2022) and semantic system (Litovsky et al. 2022; Souter et al. 2022). In addition to language dysfunctions, poststroke aphasia patients are also with cognitive (Schumacher et al. 2019) and motor (Sangtian et al. 2021) disabilities. These deficits have caused problems of communicating (Popescu et al. 2022), participating in society (Thiel and Conroy 2022) and working (Graham et al. 2011; Gilmore et al. 2022). Poststroke aphasia patients take longer time in rehabilitation compared with stroke patients without aphasia (Guzek et al. 2022). As stated above, poststroke aphasia caused enormous survival and medical pressure to patients (Wu et al. 2020) and exerted a lot of pressure on society (Ellis et al. 2012; Brady et al. 2016; Flowers et al. 2016; Liang et al. 2022) such as the utilization of healthcare resources (Berthier 2005) and healthcare cost (Breitenstein et al. 2017). Therefore, the investigation of poststroke aphasia from the perspective of neuroimaging is meaningful for further understanding neural mechanisms of poststroke aphasia, and finding therapeutic targets and interventions.
Resting-state functional magnetic resonance imaging (rs-fMRI), which is a noninvasive technology, has been a promising method to investigate spontaneous brain activity in recent years (Biswal et al. 1995; Biswal 2012). It has been used to reveal the pathophysiology of many diseases (Cortese et al. 2021; Galloway 2021), including poststroke aphasia (Klingbeil et al. 2019). Among rs-fMRI studies, two kinds of analytic methods are widely used, one is for describing the functional relations between 2 brain regions and the other for local brain activity (Zang et al. 2015). According to local brain activity, amplitude of low-frequency fluctuations (ALFF), fractional amplitude of low-frequency fluctuations (fALFF), and regional homogeneity (ReHo) are three main methods measuring abnormalities of regional spontaneous brain activity (Wang et al. 2022). ALFF and fALFF were used to reflect the intensity of regional spontaneous brain activity, and fALFF has higher sensitivity and specificity in regional spontaneous brain activity (Zang et al. 2007; Zou et al. 2008). ReHo was used to assess the similarity in time series between a given voxel and its neighbors, which means the ReHo value of each voxel in the brain was calculated based on the correlation analysis between the time series of this voxel and its neighbors (Zang et al. 2004). Studies using the ReHo methods have explored the abnormal local brain activity in stroke patients and identified impairments in cognition, consciousness, memory processing, speech, and motor (Liu et al. 2014; Jiang et al. 2019; Li, Hu, et al. 2022). The application of the combined utilization of ALFF, fALFF, and ReHo displayed a better predictive performance than a single metric (Huang et al. 2022) and could provide more detailed information (Feng et al. 2021). Studies about stroke also utilized two of these three methods to explore abnormal regional brain activity in stroke patients, finding that stroke survivors showed deficits in learning, memory, language processing, and cognition (Quan et al. 2022) and the parietal cortex could serve as a target region for certain therapies (Zhu et al. 2015). These three methods were also widely utilized in many studies to explore the alterations of regional spontaneous brain activity in various diseases, such as Parkinson’s disease with apathy (Xu et al. 2022), vestibular migraine (Li, Si, et al. 2022), bipolar disorder during euthymia (Chrobak et al. 2021), anorexia nervosa (Seidel et al. 2019), and obsessive–compulsive disorder (Bu et al. 2019). What is more, the combination of these three methods was also applied in the meta-analyses to investigate the common pattern of abnormal regional spontaneous brain activity in various diseases (Xu et al. 2015; Liu et al. 2021; Su et al. 2022; Wang et al. 2022; Yuan et al. 2022). Therefore, the integration of ALFF, fALFF, and ReHo could be helpful to further detect the pathological mechanisms of diseases in meta-analyses.
In past 10 years, an increasing number of studies have investigated the neurophysiological mechanisms of poststroke aphasia employing ALFF, fALFF, or ReHo. A study based on fALFF measured the efficacy mechanisms of intermittent theta-burst stimulation (iTBS) in poststroke aphasia (Xu et al. 2021). ALFF and ReHo were used to reveal the neuroimaging mechanism of acupuncture for poststroke aphasia (Li, Deng, et al. 2022). In addition, previous studies also revealed the significant differences of spontaneous brain activity between poststroke aphasia patients and healthy controls (HCs; Zhang et al. 2021). However, there are some differences between the results shown by different studies. For instance, one study found increased ALFF in patients with poststroke aphasia in right precentral gyrus, but another study detected decreased ALFF in the same brain region (Yang et al. 2016; Xie et al. 2022). Also, increased ALFF was observed in poststroke aphasia patients in left insula in one study, but decreased ALFF was detected in another one (Li et al. 2013; Yao et al. 2021). Given the inconsistency between results in different studies, a meta-analysis of abnormalities of regional spontaneous brain activity in poststroke aphasia is necessary to be performed because meta-analysis is an objective and quantitative method to synthesize findings of different studies to provide less biased evidence for certain topic (Cheung and Vijayakumar 2016). In addition, although previous studies have explored the brain structural abnormalities (Na et al. 2022) and functional alterations based on different language-related tasks (LaCroix et al. 2021; Stefaniak et al. 2021) in poststroke aphasia patients using the meta-analytic approach, the consistent dysfunctional brain activity of poststroke aphasia patients remains largely unknown. The present study might be complementary to the already available meta-analysis relevant to poststroke aphasia.
Therefore, this study conducted a quantitative and coordinate-based meta-analysis of abnormalities of regional spontaneous brain activity in patients with poststroke aphasia based on rs-fMRI studies by utilizing Anisotropic effect-size version of seed-based d mapping (AES-SDM). Besides, we performed analysis on the sensitivity, heterogeneity, and publication bias analysis in order to make the findings more reliable. This meta-analysis could provide further support for the exploration of etiology and pathophysiological mechanism of poststroke aphasia.
Methods
Literature search and selection criteria
In this meta-analysis, we searched PubMed, Web of Science, Embase, CNKI, and Wanfang databases until 2022 July 18, using key terms “aphasia” for the disorder, and using “amplitude of low-frequency fluctuations,” “ALFF,” “fractional amplitude of low-frequency fluctuations,” “fALFF,” “regional homogeneity,” and “ReHo” for the methods. HX and SH independently searched for all articles on the specific topic.
We selected the studies exploring abnormalities of regional spontaneous brain activity in poststroke aphasia, which met all the following criteria: (i) patients were diagnosed as aphasia based on Western Aphasia Battery (WAB) or Aphasia Battery of Chinese (ABC); (ii) scanning was without task; (iii) ALFF, fALFF, or ReHo were used in the analyses; (iv) there were comparisons between patients with poststroke aphasia and HCs; (v) the results with significant differences were reported with Montreal Neurological Institute (MNI) or Talairach coordinates; and (vi) the results were reported in the conventional frequency band (0.01–0.08 Hz). Moreover, studies were excluded if they were accompanied by one of the following two features: (i) the study was an abstract or a review; (ii) no functional magnetic resonance imaging data were acquired in the study. In addition, when the sample in one study was the same as that of another study, the one providing more information was selected and the other one is excluded.
Data extraction
There are 72 studies identified after searching several databases. Next, the two authors independently screened the studies by reading the titles and abstracts and 33 studies are left. Seven studies were excluded because of nonaphasia studies, 5 studies because of nonempirical, and 27 studies because of non-fMRI studies. Then, the authors read the whole articles and 16 studies are excluded. Moreover, there are nine studies sharing four datasets, among which four datasets with more information were retained. Finally, there are 12 studies including 12 datasets in the main meta-analysis. Additionally, all studies were assessed by a 20-point checklist which was used in prior studies (Iwabuchi et al. 2015; Su et al. 2022). Two authors independently did the literature search, study selection, data extraction, and quality assessment. The third author would make the decision when there was a divergence of opinion.
Voxel-wise meta-analysis
The meta-analysis was conducted using AES-SDM version 5.15 (http://www.sdmproject.com/software), which is a statistical technique for meta-analyzing studies on neuroimaging. First of all, we inputted the peak coordinates and t statistics that represented the significant differences between poststroke aphasia patients and HCs collected from the studies which used ALFF, fALFF, and ReHo into the text files. If t values were not reported in the study, the z values reported in the study were converted into t values online (http://www.sdmproject.com/utilities/?show=Statistics). It was notable that one text file incorporated the results of one study. Then, we recreate the effect-size brain maps for each study and create randomized SDM maps by permuting the location of the voxels. An anisotropic Gaussian kernel (Radua et al. 2014) was used and setting full width at half maximum to 20 mm was employed because it can optimally balance sensitivity and specificity (Radua et al. 2012). Next, we conducted the mean analysis using random-effects model by taking account of sample size, intrastudy variability, and interstudy heterogeneity (Radua and Mataix-Cols 2012). We employed recommended threshold values of P < 0.005, Z > 1, and cluster size > 10 voxels, which have been verified to effectively protecting against the false positives (Radua et al. 2012). In addition, we performed the meta-analysis using a more liberal threshold (P < 0.01, peak height Z > 1, and cluster size > 10 voxels) to provide more information about the brain activity in poststroke aphasia patients and the results were put in the supplementary material.
Subgroup analyses
We performed the subgroup analyses on basis of ALFF, fALFF, and ReHo separately to further characterize the abnormalities of regional spontaneous brain activity in poststroke aphasia.
Analyses of sensitivity, heterogeneity, and publication bias
A jackknife analysis was performed to assess the robustness by discarding one dataset every time (Radua and Mataix-Cols 2009). Thus, we performed 12 meta-analyses for the analysis of sensitivity. The heterogeneity analysis was conducted by using random-effect model to explore which brain regions were heterogeneous (Dugré et al. 2020). For publication bias, it was assessed with Egger test (Stanley et al. 2021). The threshold value of P < 0.05 was employed.
Meta-regression analysis
The potential effect of related demographic and clinical variables was examined by simple linear regression. The variables explored by regression were mean age (available in 11 studies), education level (available in 8 studies), and percentage of males (available in 11 studies) in poststroke aphasia patients. A more conservative threshold of P < 0.0005, Z > 1, and cluster size > 10 voxels was applied in order to reduce spurious findings (Radua and Mataix-Cols 2009; Zhou et al. 2017) and discarded findings in brain regions other than those detected in the main meta-analysis.
Activation likelihood estimation meta-analysis
To provide more information about the consistent brain dysfunctions, we also conducted the meta-analysis to investigate the abnormalities of regional spontaneous brain activity in poststroke aphasia using activation likelihood estimation (ALE) method which was a widely used method in neuroimaging (Eickhoff et al. 2005, 2012; Bucur and Papagno 2021), and the results were put in the supplementary material (Table S6 and Figure S5).
Results
Included studies and sample characteristics
In this meta-analysis, we included twelve studies including 237 patients with poststroke aphasia and 242 HCs, and 11 studies showed the gender distribution and 1 study did not include the information about gender, and 7 of the 12 studies were based on WAB to assess aphasia patients and 5 on ABC. Specific selections were shown in Fig. 1 and more information about participants was shown in Table 1.
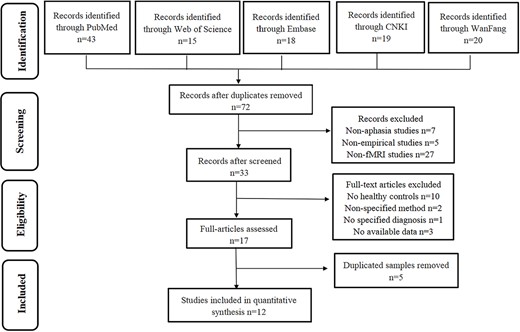
Flowchart describing the identification and selection of studies.
Study . | Diagnostic . | Methods . | Subjects (female) . | Mean age (SD) . | Mean education level (SD) . | Medication (on/off) . | Statistical threshold . | Quality scores (out of 20)a . | ||
---|---|---|---|---|---|---|---|---|---|---|
Patients . | HCs . | Patients . | HCs . | Patients . | ||||||
(Dai et al. 2021) | ABC | fALFF | 15 (6) | 18 (8) | 56.13 (7.01) | 53.83 (5.55) | 10.07 (2.84) | off | Alphasim (P < 0.005) | 19 |
(Li et al. 2014) | WAB | ALFF | 12 (4) | 20 (8) | 60.5 (8.0) | 59.0 (7.8) | NA | off | Alphasim (P < 0.005) | 20 |
(Li et al. 2021) | WAB | ALFF | 15 (2) | 15 (2) | 59.87 (11.53) | 56.73 (10.29) | 12.07 (2.87) | off | GRF (NA) | 20 |
(Liu et al. 2014) | ABC | ALFF | 10 (4) | 10 (4) | 59 (17.4) | 50 (3.8) | 10.5 (2.1) | off | Alphasim (P < 0.001) | 19 |
(Wang et al. 2020a) | ABC | ReHo | 14 (6) | 16 (5) | 65.8 (6.4) | 64.4 (3.2) | 7.5 (4.1) | off | Alphasim (P < 0.05) | 17 |
(Xie et al. 2022) | ABC | ALFF | 40 (9) | 37 (12) | 57.35 (11.79) | 55.14 (11.39) | 7.58 (5.09) | off | FWE (P < 0.05) | 19 |
(Xu et al. 2013) | ABC | ReHo | 17 (7) | 19 (6) | 49.61 (2.92) | 52.71 (3.21) | 10.29 (3.05) | off | Alphasim (P < 0.01) | 20 |
(Yang et al. 2016) | ABC | ReHo | 17 (6) | 20 (8) | 53.53 (14.06) | 54.05 (8.43) | 8.71 (1.26) | off | FDR (P < 0.05) | 20 |
(Yang and Mao 2020) | ABC | ALFF | 24 (9) | 24 (10) | 58.94 (6.71) | 43.56 (8.77) | NA | off | Uncorrected (P < 0.05) | 18 |
(Zhang et al. 2021a) | WAB | ALFF | 30 (NA) | 24 (7) | NA | 55.17 (6.13) | NA | off | TFCE (P < 0.05) | 17 |
(Zhang et al. 2021b) | WAB | ALFF | 19 (4) | 17 (5) | 48.63 (7.50) | 45.76 (7.41) | NA | off | Uncorrected (P < 0.05) | 18 |
(Zhou et al. 2021) | WAB | ALFF | 24 (10) | 22 (10) | 62.58 (12.63) | 68.36 (7.67) | 8.00 (1.91) | off | Alphasim (P < 0.001) | 20 |
Study . | Diagnostic . | Methods . | Subjects (female) . | Mean age (SD) . | Mean education level (SD) . | Medication (on/off) . | Statistical threshold . | Quality scores (out of 20)a . | ||
---|---|---|---|---|---|---|---|---|---|---|
Patients . | HCs . | Patients . | HCs . | Patients . | ||||||
(Dai et al. 2021) | ABC | fALFF | 15 (6) | 18 (8) | 56.13 (7.01) | 53.83 (5.55) | 10.07 (2.84) | off | Alphasim (P < 0.005) | 19 |
(Li et al. 2014) | WAB | ALFF | 12 (4) | 20 (8) | 60.5 (8.0) | 59.0 (7.8) | NA | off | Alphasim (P < 0.005) | 20 |
(Li et al. 2021) | WAB | ALFF | 15 (2) | 15 (2) | 59.87 (11.53) | 56.73 (10.29) | 12.07 (2.87) | off | GRF (NA) | 20 |
(Liu et al. 2014) | ABC | ALFF | 10 (4) | 10 (4) | 59 (17.4) | 50 (3.8) | 10.5 (2.1) | off | Alphasim (P < 0.001) | 19 |
(Wang et al. 2020a) | ABC | ReHo | 14 (6) | 16 (5) | 65.8 (6.4) | 64.4 (3.2) | 7.5 (4.1) | off | Alphasim (P < 0.05) | 17 |
(Xie et al. 2022) | ABC | ALFF | 40 (9) | 37 (12) | 57.35 (11.79) | 55.14 (11.39) | 7.58 (5.09) | off | FWE (P < 0.05) | 19 |
(Xu et al. 2013) | ABC | ReHo | 17 (7) | 19 (6) | 49.61 (2.92) | 52.71 (3.21) | 10.29 (3.05) | off | Alphasim (P < 0.01) | 20 |
(Yang et al. 2016) | ABC | ReHo | 17 (6) | 20 (8) | 53.53 (14.06) | 54.05 (8.43) | 8.71 (1.26) | off | FDR (P < 0.05) | 20 |
(Yang and Mao 2020) | ABC | ALFF | 24 (9) | 24 (10) | 58.94 (6.71) | 43.56 (8.77) | NA | off | Uncorrected (P < 0.05) | 18 |
(Zhang et al. 2021a) | WAB | ALFF | 30 (NA) | 24 (7) | NA | 55.17 (6.13) | NA | off | TFCE (P < 0.05) | 17 |
(Zhang et al. 2021b) | WAB | ALFF | 19 (4) | 17 (5) | 48.63 (7.50) | 45.76 (7.41) | NA | off | Uncorrected (P < 0.05) | 18 |
(Zhou et al. 2021) | WAB | ALFF | 24 (10) | 22 (10) | 62.58 (12.63) | 68.36 (7.67) | 8.00 (1.91) | off | Alphasim (P < 0.001) | 20 |
Abbreviations: FDR, false discovery rate; FEW, family wise error; NA, not available; SD, standard deviation; TFCE, threshold-free cluster enhancement.
aThe quality scores of each included study were assessed by a 20-point checklist which mainly consisted of 13 items. Each study was evaluated by the items in the checklist and the study would obtain the corresponding scores in an item if it was qualified. The total score obtained from all the items represents the quality of the study. The checklist with criteria for objective assessment was put in the Supplementary Material (Table S1).
Study . | Diagnostic . | Methods . | Subjects (female) . | Mean age (SD) . | Mean education level (SD) . | Medication (on/off) . | Statistical threshold . | Quality scores (out of 20)a . | ||
---|---|---|---|---|---|---|---|---|---|---|
Patients . | HCs . | Patients . | HCs . | Patients . | ||||||
(Dai et al. 2021) | ABC | fALFF | 15 (6) | 18 (8) | 56.13 (7.01) | 53.83 (5.55) | 10.07 (2.84) | off | Alphasim (P < 0.005) | 19 |
(Li et al. 2014) | WAB | ALFF | 12 (4) | 20 (8) | 60.5 (8.0) | 59.0 (7.8) | NA | off | Alphasim (P < 0.005) | 20 |
(Li et al. 2021) | WAB | ALFF | 15 (2) | 15 (2) | 59.87 (11.53) | 56.73 (10.29) | 12.07 (2.87) | off | GRF (NA) | 20 |
(Liu et al. 2014) | ABC | ALFF | 10 (4) | 10 (4) | 59 (17.4) | 50 (3.8) | 10.5 (2.1) | off | Alphasim (P < 0.001) | 19 |
(Wang et al. 2020a) | ABC | ReHo | 14 (6) | 16 (5) | 65.8 (6.4) | 64.4 (3.2) | 7.5 (4.1) | off | Alphasim (P < 0.05) | 17 |
(Xie et al. 2022) | ABC | ALFF | 40 (9) | 37 (12) | 57.35 (11.79) | 55.14 (11.39) | 7.58 (5.09) | off | FWE (P < 0.05) | 19 |
(Xu et al. 2013) | ABC | ReHo | 17 (7) | 19 (6) | 49.61 (2.92) | 52.71 (3.21) | 10.29 (3.05) | off | Alphasim (P < 0.01) | 20 |
(Yang et al. 2016) | ABC | ReHo | 17 (6) | 20 (8) | 53.53 (14.06) | 54.05 (8.43) | 8.71 (1.26) | off | FDR (P < 0.05) | 20 |
(Yang and Mao 2020) | ABC | ALFF | 24 (9) | 24 (10) | 58.94 (6.71) | 43.56 (8.77) | NA | off | Uncorrected (P < 0.05) | 18 |
(Zhang et al. 2021a) | WAB | ALFF | 30 (NA) | 24 (7) | NA | 55.17 (6.13) | NA | off | TFCE (P < 0.05) | 17 |
(Zhang et al. 2021b) | WAB | ALFF | 19 (4) | 17 (5) | 48.63 (7.50) | 45.76 (7.41) | NA | off | Uncorrected (P < 0.05) | 18 |
(Zhou et al. 2021) | WAB | ALFF | 24 (10) | 22 (10) | 62.58 (12.63) | 68.36 (7.67) | 8.00 (1.91) | off | Alphasim (P < 0.001) | 20 |
Study . | Diagnostic . | Methods . | Subjects (female) . | Mean age (SD) . | Mean education level (SD) . | Medication (on/off) . | Statistical threshold . | Quality scores (out of 20)a . | ||
---|---|---|---|---|---|---|---|---|---|---|
Patients . | HCs . | Patients . | HCs . | Patients . | ||||||
(Dai et al. 2021) | ABC | fALFF | 15 (6) | 18 (8) | 56.13 (7.01) | 53.83 (5.55) | 10.07 (2.84) | off | Alphasim (P < 0.005) | 19 |
(Li et al. 2014) | WAB | ALFF | 12 (4) | 20 (8) | 60.5 (8.0) | 59.0 (7.8) | NA | off | Alphasim (P < 0.005) | 20 |
(Li et al. 2021) | WAB | ALFF | 15 (2) | 15 (2) | 59.87 (11.53) | 56.73 (10.29) | 12.07 (2.87) | off | GRF (NA) | 20 |
(Liu et al. 2014) | ABC | ALFF | 10 (4) | 10 (4) | 59 (17.4) | 50 (3.8) | 10.5 (2.1) | off | Alphasim (P < 0.001) | 19 |
(Wang et al. 2020a) | ABC | ReHo | 14 (6) | 16 (5) | 65.8 (6.4) | 64.4 (3.2) | 7.5 (4.1) | off | Alphasim (P < 0.05) | 17 |
(Xie et al. 2022) | ABC | ALFF | 40 (9) | 37 (12) | 57.35 (11.79) | 55.14 (11.39) | 7.58 (5.09) | off | FWE (P < 0.05) | 19 |
(Xu et al. 2013) | ABC | ReHo | 17 (7) | 19 (6) | 49.61 (2.92) | 52.71 (3.21) | 10.29 (3.05) | off | Alphasim (P < 0.01) | 20 |
(Yang et al. 2016) | ABC | ReHo | 17 (6) | 20 (8) | 53.53 (14.06) | 54.05 (8.43) | 8.71 (1.26) | off | FDR (P < 0.05) | 20 |
(Yang and Mao 2020) | ABC | ALFF | 24 (9) | 24 (10) | 58.94 (6.71) | 43.56 (8.77) | NA | off | Uncorrected (P < 0.05) | 18 |
(Zhang et al. 2021a) | WAB | ALFF | 30 (NA) | 24 (7) | NA | 55.17 (6.13) | NA | off | TFCE (P < 0.05) | 17 |
(Zhang et al. 2021b) | WAB | ALFF | 19 (4) | 17 (5) | 48.63 (7.50) | 45.76 (7.41) | NA | off | Uncorrected (P < 0.05) | 18 |
(Zhou et al. 2021) | WAB | ALFF | 24 (10) | 22 (10) | 62.58 (12.63) | 68.36 (7.67) | 8.00 (1.91) | off | Alphasim (P < 0.001) | 20 |
Abbreviations: FDR, false discovery rate; FEW, family wise error; NA, not available; SD, standard deviation; TFCE, threshold-free cluster enhancement.
aThe quality scores of each included study were assessed by a 20-point checklist which mainly consisted of 13 items. Each study was evaluated by the items in the checklist and the study would obtain the corresponding scores in an item if it was qualified. The total score obtained from all the items represents the quality of the study. The checklist with criteria for objective assessment was put in the Supplementary Material (Table S1).
Differences in regional spontaneous brain activity
This meta-analysis revealed that there were significant differences in regional spontaneous brain activity between poststroke aphasia patients and HCs. And the results were based on the threshold of P < 0.005, peak height Z > 1, and cluster size > 10 voxels. As described in Table 2 and Fig. 2, patients with poststroke aphasia showed increased regional spontaneous brain activity in the right insula (Insula_R), right postcentral gyrus (Postcentral_R), left cerebellar lobule IX (Cerebellum_9_L), left angular gyrus (Angular_L), right caudate nucleus (Caudate_R), left parahippocampal gyrus (ParaHippocampal_L), and right supplementary motor area (Supp_Motor_Area_R). Poststroke aphasia patients showed decreased regional spontaneous brain activity in the left cerebellar lobule VI (Cerebellum_6_L), left median cingulate and paracingulate gyri (Cingulum_Mid_L), right cerebellar crus I (Cerebellum_Crus1_R), and left supplementary motor area (Supp_Motor_Area_L).
Regions with abnormal regional spontaneous brain activity in poststroke aphasia patients relative to HCs.
Location . | MNI coordinate . | Cluster size . | SDM-Z value . | Effect size . | P-value . | Jacknife sensitivity analysis . | Heterogeneity . | Egger test (P-value) . | ||
---|---|---|---|---|---|---|---|---|---|---|
x . | y . | z . | ||||||||
Patients > HCs | ||||||||||
Insula_R (aal) | 42 | 4 | −6 | 569 | 1.950 | 0.18045 | 0.000106573 | 12/12 | no | 0.593 |
Postcentral_R (aal) | 36 | −30 | 56 | 169 | 1.611 | 0.15076 | 0.000996053 | 11/12 | no | 0.758 |
Cerebellum_9_L (aal) | −8 | −48 | −40 | 93 | 1.664 | 0.06858 | 0.000704467 | 11/12 | no | 0.081 |
Angular_L (aal) | −36 | −60 | 34 | 63 | 1.490 | 0.14089 | 0.002001107 | 10/12 | no | 0.982 |
Caudate_R (aal) | 8 | 10 | 10 | 74 | 1.391 | 0.05246 | 0.003449976 | 11/12 | no | 0.025 |
ParaHippocampal_L (aal) | −22 | −38 | −8 | 37 | 1.637 | 0.15185 | 0.000835299 | 11/12 | no | 0.036 |
Supp_Motor_Area_R (aal) | 6 | −2 | 70 | 11 | 1.389 | 0.12831 | 0.003506243 | 6/12 | no | 0.025 |
Patients< HCs | ||||||||||
Cerebellum_6_L (aal) | −36 | −50 | −28 | 386 | −1.584 | −0.32515 | 0.001183629 | 10/12 | yes | 0.040 |
Cingulum_Mid_L (aal) | 6 | −26 | 28 | 118 | −2.000 | −0.18614 | 0.000073254 | 12/12 | yes | 0.586 |
Cerebellum_Crus1_R (aal) | 14 | −84 | −24 | 292 | −2.025 | −0.18724 | 0.000064254 | 11/12 | no | 0.159 |
Supp_Motor_Area_L (aal) | 0 | 16 | 44 | 155 | −1.450 | −0.23477 | 0.002647519 | 10/12 | yes | 0.098 |
Location . | MNI coordinate . | Cluster size . | SDM-Z value . | Effect size . | P-value . | Jacknife sensitivity analysis . | Heterogeneity . | Egger test (P-value) . | ||
---|---|---|---|---|---|---|---|---|---|---|
x . | y . | z . | ||||||||
Patients > HCs | ||||||||||
Insula_R (aal) | 42 | 4 | −6 | 569 | 1.950 | 0.18045 | 0.000106573 | 12/12 | no | 0.593 |
Postcentral_R (aal) | 36 | −30 | 56 | 169 | 1.611 | 0.15076 | 0.000996053 | 11/12 | no | 0.758 |
Cerebellum_9_L (aal) | −8 | −48 | −40 | 93 | 1.664 | 0.06858 | 0.000704467 | 11/12 | no | 0.081 |
Angular_L (aal) | −36 | −60 | 34 | 63 | 1.490 | 0.14089 | 0.002001107 | 10/12 | no | 0.982 |
Caudate_R (aal) | 8 | 10 | 10 | 74 | 1.391 | 0.05246 | 0.003449976 | 11/12 | no | 0.025 |
ParaHippocampal_L (aal) | −22 | −38 | −8 | 37 | 1.637 | 0.15185 | 0.000835299 | 11/12 | no | 0.036 |
Supp_Motor_Area_R (aal) | 6 | −2 | 70 | 11 | 1.389 | 0.12831 | 0.003506243 | 6/12 | no | 0.025 |
Patients< HCs | ||||||||||
Cerebellum_6_L (aal) | −36 | −50 | −28 | 386 | −1.584 | −0.32515 | 0.001183629 | 10/12 | yes | 0.040 |
Cingulum_Mid_L (aal) | 6 | −26 | 28 | 118 | −2.000 | −0.18614 | 0.000073254 | 12/12 | yes | 0.586 |
Cerebellum_Crus1_R (aal) | 14 | −84 | −24 | 292 | −2.025 | −0.18724 | 0.000064254 | 11/12 | no | 0.159 |
Supp_Motor_Area_L (aal) | 0 | 16 | 44 | 155 | −1.450 | −0.23477 | 0.002647519 | 10/12 | yes | 0.098 |
Abbreviations: aal, anatomical automatic labeling; Angular_L, left angular gyrus; Caudate_R, right caudate nucleus; Cerebellum_Crus1_R, right cerebellar crus I; Cerebellum_9_L, left cerebellar lobule IX; Cerebellum_6_L, left cerebellar lobule VI; Cingulum_Mid_L, left median cingulate and paracingulate gyri; Insula_R, right insula; ParaHippocampal_L, left parahippocampal gyrus; Postcentral_R, right postcentral gyrus; SDM, seed-based d mapping; Supp_Motor_Area_L, left supplementary motor area; Supp_Motor_Area_R, right supplementary motor area.
Regions with abnormal regional spontaneous brain activity in poststroke aphasia patients relative to HCs.
Location . | MNI coordinate . | Cluster size . | SDM-Z value . | Effect size . | P-value . | Jacknife sensitivity analysis . | Heterogeneity . | Egger test (P-value) . | ||
---|---|---|---|---|---|---|---|---|---|---|
x . | y . | z . | ||||||||
Patients > HCs | ||||||||||
Insula_R (aal) | 42 | 4 | −6 | 569 | 1.950 | 0.18045 | 0.000106573 | 12/12 | no | 0.593 |
Postcentral_R (aal) | 36 | −30 | 56 | 169 | 1.611 | 0.15076 | 0.000996053 | 11/12 | no | 0.758 |
Cerebellum_9_L (aal) | −8 | −48 | −40 | 93 | 1.664 | 0.06858 | 0.000704467 | 11/12 | no | 0.081 |
Angular_L (aal) | −36 | −60 | 34 | 63 | 1.490 | 0.14089 | 0.002001107 | 10/12 | no | 0.982 |
Caudate_R (aal) | 8 | 10 | 10 | 74 | 1.391 | 0.05246 | 0.003449976 | 11/12 | no | 0.025 |
ParaHippocampal_L (aal) | −22 | −38 | −8 | 37 | 1.637 | 0.15185 | 0.000835299 | 11/12 | no | 0.036 |
Supp_Motor_Area_R (aal) | 6 | −2 | 70 | 11 | 1.389 | 0.12831 | 0.003506243 | 6/12 | no | 0.025 |
Patients< HCs | ||||||||||
Cerebellum_6_L (aal) | −36 | −50 | −28 | 386 | −1.584 | −0.32515 | 0.001183629 | 10/12 | yes | 0.040 |
Cingulum_Mid_L (aal) | 6 | −26 | 28 | 118 | −2.000 | −0.18614 | 0.000073254 | 12/12 | yes | 0.586 |
Cerebellum_Crus1_R (aal) | 14 | −84 | −24 | 292 | −2.025 | −0.18724 | 0.000064254 | 11/12 | no | 0.159 |
Supp_Motor_Area_L (aal) | 0 | 16 | 44 | 155 | −1.450 | −0.23477 | 0.002647519 | 10/12 | yes | 0.098 |
Location . | MNI coordinate . | Cluster size . | SDM-Z value . | Effect size . | P-value . | Jacknife sensitivity analysis . | Heterogeneity . | Egger test (P-value) . | ||
---|---|---|---|---|---|---|---|---|---|---|
x . | y . | z . | ||||||||
Patients > HCs | ||||||||||
Insula_R (aal) | 42 | 4 | −6 | 569 | 1.950 | 0.18045 | 0.000106573 | 12/12 | no | 0.593 |
Postcentral_R (aal) | 36 | −30 | 56 | 169 | 1.611 | 0.15076 | 0.000996053 | 11/12 | no | 0.758 |
Cerebellum_9_L (aal) | −8 | −48 | −40 | 93 | 1.664 | 0.06858 | 0.000704467 | 11/12 | no | 0.081 |
Angular_L (aal) | −36 | −60 | 34 | 63 | 1.490 | 0.14089 | 0.002001107 | 10/12 | no | 0.982 |
Caudate_R (aal) | 8 | 10 | 10 | 74 | 1.391 | 0.05246 | 0.003449976 | 11/12 | no | 0.025 |
ParaHippocampal_L (aal) | −22 | −38 | −8 | 37 | 1.637 | 0.15185 | 0.000835299 | 11/12 | no | 0.036 |
Supp_Motor_Area_R (aal) | 6 | −2 | 70 | 11 | 1.389 | 0.12831 | 0.003506243 | 6/12 | no | 0.025 |
Patients< HCs | ||||||||||
Cerebellum_6_L (aal) | −36 | −50 | −28 | 386 | −1.584 | −0.32515 | 0.001183629 | 10/12 | yes | 0.040 |
Cingulum_Mid_L (aal) | 6 | −26 | 28 | 118 | −2.000 | −0.18614 | 0.000073254 | 12/12 | yes | 0.586 |
Cerebellum_Crus1_R (aal) | 14 | −84 | −24 | 292 | −2.025 | −0.18724 | 0.000064254 | 11/12 | no | 0.159 |
Supp_Motor_Area_L (aal) | 0 | 16 | 44 | 155 | −1.450 | −0.23477 | 0.002647519 | 10/12 | yes | 0.098 |
Abbreviations: aal, anatomical automatic labeling; Angular_L, left angular gyrus; Caudate_R, right caudate nucleus; Cerebellum_Crus1_R, right cerebellar crus I; Cerebellum_9_L, left cerebellar lobule IX; Cerebellum_6_L, left cerebellar lobule VI; Cingulum_Mid_L, left median cingulate and paracingulate gyri; Insula_R, right insula; ParaHippocampal_L, left parahippocampal gyrus; Postcentral_R, right postcentral gyrus; SDM, seed-based d mapping; Supp_Motor_Area_L, left supplementary motor area; Supp_Motor_Area_R, right supplementary motor area.
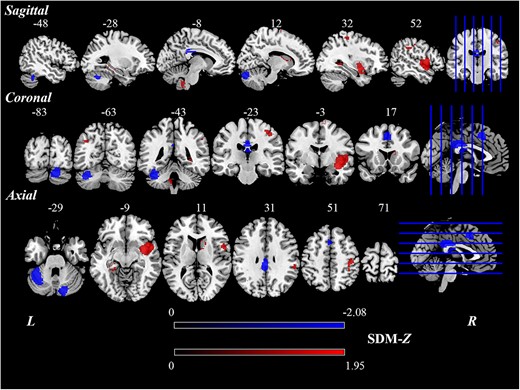
Brain regions showing significant differences of regional spontaneous brain activity between poststroke aphasia patients and HCs based on the meta-analysis.
Subgroup analyses
The subgroup analysis of ALFF studies (n = 10) showed that, compared with HCs, poststroke aphasia patients displayed increased ALFF values in the left cerebellar lobule VIII (Cerebellum_8_L), right superior temporal pole (Temporal_Pole_Sup_R), left hippocampus (Hippocampus_L), Caudate_R, Postcentral_R, and Supp_Motor_Area_R, and decreased ALFF values in the left fusiform gyrus (Fusiform_L), Cerebellum_Crus1_R, Supp_Motor_ Area_L, and Cingulum_Mid_L (Table S2 and Fig. S1). For fALFF studies (n = 2), poststroke aphasia patients showed increased fALFF values in the right middle temporal gyrus (Temporal_Mid_R), Angular_L, right superior parietal gyrus (Parietal_Sup_R), right middle frontal gyrus (Frontal_Mid_R), Postcentral_R, left superior frontal gyrus (Frontal_Sup_L), and left paracentral lobule (Paracentral_Lobule_L), and decreased fALFF values in the right cerebellar lobule VI (Cerebelum_6_R) (Table S3 and Fig. S2). As for ReHo subgroup analysis (n = 3), poststroke aphasia patients exhibited increased ReHo values in Insula_R (Table S4 and Fig. S3).
Analyses of sensitivity, heterogeneity, and publication bias
It was found in the sensitivity analysis that Insula_R and Cingulum_Mid_L were replicable in all 12 meta-analyses. Postcentral_R, Cerebellum_9_L, Angular_L, Caudate_R, ParaHippocampal_L, Cerebellum_6_L, Cerebellum_Crus1_R, and Supp_Motor_Area_L remained significant in at least 10 meta-analyses. The difference in Supp_Motor_Area_R was replicable in six meta-analyses. For heterogeneity analysis, we found heterogeneity in Cerebellum_6_L, Cingulum_Mid_L, and Supp_Motor_Area_L. Egger tests were calculated and revealed that publication bias was detected in Caudate_R, ParaHippocampal_L, Supp_Motor_Area_R, and Cerebellum_6_L.
Meta-regression analysis
Meta-regression analysis showed that no significant associations were found between the regional activity in differential brain regions and mean age, education level, and percentage of males in poststroke aphasia patients.
Discussion
Judged by the information we have, we find that this is the first meta-analysis to identify the most consistent pattern of abnormal regional spontaneous brain activity in individuals with poststroke aphasia. We found that poststroke aphasia patients showed significantly increased regional spontaneous brain activity in Insula_R, Postcentral_R, Cerebellum_9_L, Angular_L, Caudate_R, ParaHippocampal_L, and Supp_Motor_Area_R, and decreased brain activity in Cerebellum_6_L, Cingulum_Mid_L, Cerebellum_Crus1_R, and Supp_Motor_Area_L relative to HCs. Given that previous meta-analyses of poststroke aphasia mainly focused on the treatment for the disease (Fu et al. 2022; Harvey et al. 2022; Kielar et al. 2022), this study is complementary to the neural mechanism of poststroke aphasia.
In the present meta-analysis, Insula_R showed consistently increased regional spontaneous brain activity in poststroke aphasia compared with healthy participants. The insula is an invisible brain region located within the lateral sulcus (Uddin et al. 2017). It is in connection with cognition (Augustine 1996; Ramsay et al. 2022) such as poor performance in immediate and delayed recall showed in patients with insula injury (Gasquoine 2014). In addition, the insula is associated with certain linguistic function as well (Mutschler et al. 2009; Stefanova et al. 2020). It has been proposed to play an important role in coordinating the speech production not only in lesion studies (Duffau et al. 2001; Cereda et al. 2002) but also in Neuroimaging studies on basis of healthy participants (Murphy et al. 1997). Specifically, the insula has been considered to play a major role as the filter which is necessary to coordinate a lot of muscles participated in the articulation (Ackermann and Riecker 2004). A meta-analysis provided evidence that Insula_R is involved in the production of language (Oh et al. 2014). The abnormality of Insula_R in poststroke aphasia was also observed in a fMRI study whose finding was similar to the result displayed in the current meta-analysis (van Hees et al. 2014). The abnormality of regional spontaneous brain activity in Insula_R might be related to abnormal articulatory functions in poststroke aphasia patients.
Besides, it was found in the present study that Angular_L showed increased regional brain activity in poststroke aphasia compared with HCs in the present study. The angular gyrus was proposed to serve as a necessary negotiator for all kinds of functions, particularly for the development of language (Joseph 1982; Benischek et al. 2020), including reading, comprehension, and semantic processing (Seghier 2013). Poststroke aphasia patients were accompanied by phonological ability abnormality which was related to hemodynamic changes in angular gyrus (Zhao et al. 2018). The Angular_L was associated with auditory comprehension, including single word and sequential commands (Lwi et al. 2021), and it plays a major role in comprehension of speech at sentence level (Hartwigsen et al. 2015). Besides, the angular gyrus engages in the thematic processing. Besides, the angular gyrus engages in the thematic processing which refers to the expression of certain meaning by organizing the order of words (Leech 1974), and the inhibition of irrelevant semantic information (Lewis et al. 2019). A fMRI study showed that Angular_L was activated by semantic matching tasks (Seghier et al. 2010). Furthermore, angular lesions were detected in poststroke aphasia patients with auditory comprehension deficits (Lwi et al. 2021). The abnormality showed in the meta-analysis might be associated with the dysfunctions in reading, comprehension, and semantic processing in poststroke aphasia patients.
The abnormalities of regional spontaneous brain activity in poststroke aphasia patients were detected in Cerebellum_9_L, Cerebellum_6_L, and Cerebellum_Crus1_R. The cerebellum was considered to play a crucial role in the control of movement and the importance of cerebellum in coordinating movement was also proved in many lesion studies (Thach et al. 1992; Chan et al. 2009; D'Angelo 2018; Therrien and Bastian 2019). Besides, the role of cerebellum was not limited to motor functions (Paulin 1993; Fritz et al. 2022) but also involved in cognition (Schmahmann 2019; Van Overwalle et al. 2020) and linguistic functions (Marien et al. 2001). For example, Gordon (2007) noted that the cerebellum was activated in a series of cognitive processes including spatial organization and visual memory. Ackermann et al. (2007) found that cerebellum contributes to the control of vocal tract muscles which were involved in the speech production. McDermott et al. (2003) noted that the Cerebellum_Crus1_R was active when making the semantic decisions. And lower gray matter volumes were detected in Cerebellum_Crus1_R in poststroke aphasia patients relative to HCs (Zhang et al. 2022). The abnormality in cerebellum might be associated with disorders in motor, cognition, and language in poststroke aphasia patients.
What is more, the supplementary motor area was another brain region showing the abnormalities of regional spontaneous brain activity in patients with poststroke aphasia. The supplementary motor area performed the function of controlling speech communication and language reception (Hertrich et al. 2016), and it also exhibited different contributions between the left and right cerebral hemispheres. Specially, a greater tendency for activation in the Supp_Motor_Area_L was observed during the language task (Chung et al. 2005; Dalacorte et al. 2012). A PET study provided the evidence for compensatory role of the right hemisphere in some poststroke aphasia patients with left hemispheric damage (Weiller et al. 1995). Increased activation of Supp_Motor_Area_R was also observed in poststroke aphasia patients during comprehension tasks compared with HCs, and this brain region also showed correlation between improved language function and increased activation (Saur et al. 2006). Thus, we speculate that decreased brain activity in Supp_Motor_Area_L might due to the dysfunctions related with language and increased brain activity due to compensatory mechanism in Supp_Motor_Area_R.
Moreover, increased regional spontaneous brain activity was found in the Caudate_R and Postcentral_R in poststroke aphasia patients. It has been proved that these two brain regions are connected with the impairments of language functions, such as speech (Vanburen 1963), verbal fluency (Hsieh et al. 2017), and articulation planning (Mirman et al. 2019). Meanwhile, the alterations of regional spontaneous brain activity in these two brain regions were also detected in stroke studies (Jiang et al. 2019; Li, Hu, et al. 2022). It might be difficult to differentiate the effect between stroke and aphasia, and some comparative analyses between stroke and aphasia from the perspective of neuroimaging might be meaningful in further research.
We also found increased regional spontaneous brain activity in ParaHippocampal_L, Cingulum_Mid_L in patients with poststroke aphasia relative to HCs. The parahippocampal gyrus and cingulate gyrus are parts of limbic systems (Kötter and Meyer 1992). There is a neural interface between limbic and motor systems, and the interface makes the translation of motivation into action (Mogenson et al. 1980). The cingulate cortex is involved in negative cognition and emotion regulation (Su et al. 2021). The parahippocampal gyrus mainly performed the functions of visuospatial processing and episodic memory (Aminoff et al. 2013; Liu et al. 2016). In addition, the parahippocampus contributes to information processing and semantic fluency (Zhou et al. 2016; Biesbroek et al. 2021). A meta-analysis showed that parahippocampal gyrus was involved in the network activated by semantic processing (Binder et al. 2009). The increased regional spontaneous brain activity showed in the current study might be associated with the compensation for language deficits in poststroke aphasia patients.
Abnormal regional spontaneous brain activity in the left temporal pole was detected based on a more liberal threshold (P < 0.01, peak height Z > 1, and cluster size > 10 voxels) as shown in the supplementary material (Table S5 and Fig. S4). The temporal pole plays an important role in semantic cognition and provides support for the conceptual knowledge (Lambon Ralph et al. 2009; Simmons and Martin 2009). And the damage to the left temporal pole was associated with impaired naming of landmarks (Tranel 2006) and famous faces (Grabowski et al. 2001) which were related to semantic information (Schneider et al. 2018). The abnormality in poststroke aphasia patients might be relevant to the defects of semantic function.
Although the integration of ALFF, fALFF, and ReHo could provide more comprehensive information about the abnormalities of regional spontaneous brain activity in poststroke aphasia patients, we also conducted the subgroup analyses based on these three methods, respectively, to further explore the characteristics of brain activity in poststroke aphasia patients. The results of subgroup analyses further supported the results of meta-analysis in the current study and they also revealed specific brain regions dependent on ALFF, fALFF, or ReHo. For instance, the subgroup analysis of ALFF studies found abnormal brain activity in Hippocampus_L and Fusiform_L, and fALFF studies found the abnormalities in Temporal_Mid_R, Parietal_Sup_R, Frontal_Mid_R, Frontal_Sup_L, and Paracentral_Lobule_L. These brain regions have been revealed to be associated with impaired functions related to poststroke aphasia patients (Guo et al. 2019; Zhao et al. 2020; Zhang et al. 2021; Liu et al. 2022; Schevenels et al. 2022).
Previous studies indicated that there was no association between age and the incidence of aphasia in stroke patients, and no significant differences in the ages between stroke patients with aphasia and those without aphasia (Kyrozis et al. 2009; Gialanella et al. 2011). However, many studies also examined the influence of age on the likelihood of having aphasia following stroke finding that older stroke patients were more likely to develop aphasia compared with younger stroke patients (Miceli et al. 1981; Pedersen et al. 1995; Engelter et al. 2006; Tsouli et al. 2009; Dickey et al. 2010). In addition, there was also the influence of age on aphasia type in stroke patients (Obler et al. 1978; Miceli et al. 1981). The findings in our study might be treated with caution given the potential association between age and aphasia in stroke patients.
ALE treats activation foci in included studies as spatial probability distributions centered at the given coordinates rather than as single points (Eickhoff et al. 2012). In the present study, besides the results consistent with the AES-SDM, the ALE method also provided additional findings about the brain abnormalities in poststroke aphasia patients. The left calcarine (Calcarine_L) was highly activated in the processing of pseudowords and real words (Xiao et al. 2005). There were studies suggesting that the superior frontal gyrus was involved in the processing of working memory (Awh et al. 1995; Cornette et al. 2001) and the lesions in this brain region could result in deficits in working memory (du Boisgueheneuc et al. 2006). The posterior middle temporal gyrus (Temporal_Pole_Mid) played an essential role in syntactic comprehension (Yu et al. 2022), and it was also related to lexical comprehension and semantic processing in relevant tasks (Briggs et al. 2021). The abnormalities in these brain regions might be associated with the aberrant functions of language processing and memory.
In addition, the heterogeneity might be due to the clinical differences between different studies, such as the types of participants or methodological differences (Ioannidis 2008; Sterne et al. 2011). The publication bias might be the result of incomplete data reporting (Rothstein et al. 2005). The jackknife sensitivity analysis was conducted to test the replicability of the results and found that the result in Supp_Motor_Area_R was not very robust which might be due to the effect size of the cluster being small. The abnormalities of regional spontaneous brain activity in these brain regions should be treated with caution.
Limitations
The meta-analysis in the present study has some limitations. First, due to the limited clinical information obtained from the included studies, the illness duration was not provided in the current study. The second limitation of the study was that the included studies in the meta-analysis are cross-sectional and future longitudinal studies are needed to further explore the prognosis of poststroke aphasia. Next, although the meta-regression results revealed that there is no association between mean age and the differential brain region signals, the results might be influenced by the age distribution of the included studies. It reminds the cautious interpretation of the results. Finally, although the present study revealed the consistent dysfunction of poststroke aphasia patients, it was hard to distinguish the effect of stroke and aphasia. The comparative analysis between stroke and poststroke aphasia from the perspective of neuroimaging might be meaningful to provide more evidence for stroke and poststroke aphasia in future studies.
Conclusion
As far as we know, the present study is the first meta-analysis for the abnormalities of regional spontaneous brain activity in poststroke aphasia. We found abnormalities in Insula_R, Postcentral_R, Angular_L, Caudate_R, ParaHippocampal_L, cerebellum, Cingulum_Mid_L, and supplementary motor area with great consistency. These findings provided further evidence that the abnormalities of regional spontaneous brain activity might have great significance for studying the etiology of poststroke aphasia. In addition, this study could offer further support for the exploration of the pathophysiological mechanism in the poststroke aphasia.
Acknowledgments
The authors thank all the participants.
Funding
Ministry of Education Humanities and Social Sciences Research Youth Fund Project of the People’s Republic of China “Assessment and Intervention of Children with Autism in Chinese” (20YJC740008); Fundamental Research Funds for the Central Universities “The cognitive research of autistic children with language disorders” (22CX04014B); 71st Batch of China Postdoctoral Science Foundation “A Study on metaphor and metonymy acquisition of Chinese autistic children” (2022 M712151); “National Natural Science Foundation of China” (82001898).
Conflict of interest statement: None declared.
Data availability statement
The data that support the findings of this study are available on request from the corresponding author.
References
Awh E, Smith EE, Jonides J.

Souter NE, Wang X, Thompson H, Krieger-Redwood K, Halai AD, Lambon Ralph MA, Thiebaut de Schotten M, Jefferies E.
Thiel L, Conroy P.
Vanburen JM.
Author notes
Lulu Cheng and Hongyu Xi have contributed equally to the study.