-
PDF
- Split View
-
Views
-
Cite
Cite
Zhibing Xiao, Zhiyi Chen, Wanting Chen, Wei Gao, Li He, Qiang Wang, Xu Lei, Jiang Qiu, Tingyong Feng, Hong Chen, Ofir Turel, Antoine Bechara, Qinghua He, Maladaptive changes in delay discounting in males during the COVID-19 pandemic: the predictive role of functional connectome, Cerebral Cortex, Volume 32, Issue 20, 15 October 2022, Pages 4605–4618, https://doi.org/10.1093/cercor/bhab505
- Share Icon Share
Abstract
The Coronavirus disease of 2019 (COVID-19) and measures to curb it created population-level changes in male-dominant impulsive and risky behaviors such as violent crimes and gambling. One possible explanation for this is that the pandemic has been stressful, and males, more so than females, tend to respond to stress by altering their focus on immediate versus delayed rewards, as reflected in their delay discounting rates. Delay discounting rates from healthy undergraduate students were collected twice during the pandemic. Discounting rates of males (n=190) but not of females (n=493) increased during the pandemic. Using machine learning, we show that prepandemic functional connectome predict increased discounting rates in males (n=88). Moreover, considering that delay discounting is associated with multiple psychiatric disorders, we found the same neural pattern that predicted increased discounting rates in this study, in secondary datasets of patients with major depression and schizophrenia. The findings point to sex-based differences in maladaptive delay discounting under real-world stress events, and to connectome-based neuromarkers of such effects. They can explain why there was a population-level increase in several impulsive and risky behaviors during the pandemic and point to intriguing questions about the shared underlying mechanisms of stress responses, psychiatric disorders and delay discounting.
Introduction
The coronavirus disease of 2019 (COVID-19) grew to be a stressful global pandemic. It had many unforeseen impacts on society, including noticeable changes in impulsive and risky behaviors, predominantly in males. For example, although lockdown measures reduced crime in the USA, the reduction was mostly in minor offenses committed in peer groups. In contrast, the pandemic and attempts to curb it (e.g., lockdowns, curfews) drove an increase in homicide and intimate partner violence in the USA (Boman and Gallupe 2020), an increase in gun-violence in major US cities (Sutherland et al. 2021), elevated levels of drug offenses in Victoria, Australia (Rmandic et al. 2020), a surge in family violence in China (Zhang 2020), and an increase in sports gambling in Sweden (Håkansson 2020) compared with the prepandemic levels. One common thread in these behaviors is that they can be considered largely impulsive and risky in that they harbor a reasonable chance of adverse consequences, are often done at the spur of the moment, and encapsulate a preference for immediate rewards or a myopic view, combined with some disregard for potential long-term harms. Another common thread in these behaviors, is that like many risky behaviors, they tend to be male-dominant (Wong et al. 2013; Korn and Bonny-Noach 2018; Magee et al. 2020; Kuo et al. 2021).
In this article, we posit that one possible explanation for the abovementioned trends is changes in the impulsivity of males during the pandemic, as reflected in their delay discounting, that is, the rate of depreciation in reward value as time passes. When rewards are delayed, people on average have the tendency to prefer smaller but immediate rewards (Lv et al. 2019). Delay discounting is commonly assessed through intertemporal choice tasks involving choices between immediate and delayed rewards. Impulsive and risky behaviors are rooted in unhealthy delay discounting rates that reflect myopic preferences for immediate rewards, and disregard to potential future harms (Mishra and Lalumière 2017). Thus, the first objective of this study is to examine if the pandemic has resulted in noticeable changes in delay discounting, specifically in males; if true, this can serve as one possible explanation for the abovementioned population-level behavioral changes.
It is reasonable to expect changes in delay discounting, because the COVID-19 pandemic is a major stressor (Varma et al. 2021), that can even lead to posttraumatic stress disorder in postinfection cured people (Janiri et al. 2021). The stress emerges from the unpredictable duration and magnitude of the effects of the pandemic on life; these have led to a climate of high uncertainty during the pandemic, with concerns around health, relationship, finances, and job security (Koffman et al. 2020). Although correlational studies suggest that stress can be associated with greater delay discounting during COVID-19 (Malesza 2019; Lloyd et al. 2021; DeAngelis et al. 2021), there is limited insight regarding possible changes in delay discounting that may accrue with ongoing COVID-19 stress. Such changes are expected because ongoingly distressed people do not have the cognitive resources to inhibit impulsive behaviors (Enticott et al. 2006; Raio et al. 2020); their cognitive and neural foci shift to regulating the stress (Lempert et al. 2012).
Further extending this view, we posit that there may be important individual difference in brain functioning that can alter the effect of the pandemic on one’s delay discounting. Uncovering such mechanisms can not only help understating why some people and not others increase their delay discounting during stressful events, such as the pandemic, but also provide insights for a broad range of research disciplines that focus on risky, impulsive and problematic behaviors, for example, psychology, psychiatry, and criminology. Specifically, delay discounting has been associated with activations of the frontal part of the dorsal medial prefrontal cortex and the right frontal pole (Kable and Glimcher 2007; Luo et al. 2013; Wang et al. 2014; Wang et al. 2016), and an intrinsic functional network pattern (Chen et al. 2017; Johnson et al. 2020) including default-mode, subcortical, somato-motor, dorsal attention, and visual systems. However, few studies have addressed the neural basis of delay discounting under stress (Starcke and Brand 2012), especially real-world stressful events, and the possible effects of such events on changes in delay discounting. Such studies have also typically focused on specific networks, and here, we extend this view to focus also on connectivity among networks.
We further note that although stress has been associated with steeper discounting rates (Lempert et al. 2012; Fields et al. 2014; Malesza 2019), females physiological reactions to stress are typically of a smaller magnitude than those of males, including the secretion of Cortisol (Kajantie and Phillips 2006). There is also increased risk-aversion in males but not in females (Cahlíková and Cingl 2017). In part, this is because there are sex-based differences in Cortisol levels and stress responses (Kajantie and Phillips 2006; Paris et al. 2010). Importantly, Cortisol levels are associated with delay discounting (Takahashi 2004; Takahashi et al. 2011; Lempert et al. 2012; Kimura et al. 2013).
Nevertheless, findings on the association between sex and delay discounting have been inconsistent. Some studies find that males are higher discounters (Kirby and Maraković 1996; Wilson and Daly 2004), some find that females are higher discounters (Reynolds et al. 2006), and others find no sex-based differences in delay discounting (Hirsh et al. 2008; Lucas and Koff 2010). One explanation is that mood and stressors moderate the relationship between sex and delay discounting (Koff and Lucas 2011). Stress-induced changes in delay discounting can also drive sex-based differences in risky decision-making (Lighthall et al. 2009; Takahashi et al. 2011). Considering the evidence that females’ psychological reactions to stress are usually smaller than males’ (Kajantie and Phillips 2006), and that COVID-19 is a major life stressor (Varma et al. 2021), we hypothesize that COVID-19 has greater impact on changes in delay discounting in males than in females. Considering evidence that stress (Soares et al. 2013; Zhang et al. 2020) and delay discounting (Chen et al. 2017; Cai et al. 2020) share a synchronous activation of a network of brain regions as manifested in the brain connectome, we also expect that the degree of resting-state functional connectome would influence the susceptibility of individuals to change their delay discounting in response to stressors, such as the pandemic.
We test our assertions with a longitudinal design that involves capturing the brain connectome of individuals before the pandemic (t0), and delay discounting twice (t1 and t2), during the pandemic. Lastly, we further note that delay discounting is associated with multiple maladaptive behaviors (Alessi and Petry 2003; Hu et al. 2015) and psychiatric disorders (Amlung et al. 2019), such as drug abuse and problem gambling (Reynolds 2006), major depression, and schizophrenia (Sanchez-Roige et al. 2018). Thus, to increase the validity of our results relating the brain connectome to changes in delay discounting, we use multiple clinical (schizophrenia and major depression) datasets and examine whether the neuromarkers we identified in this study truly represent a maladaptive delay discounting network that can distinguish psychiatric patients from normal controls.
Methods
Participants
After obtaining approval from the local institutional review board, potential participants’ contact information was retrieved from the Behavioral Brain Research Project of Chinese Personality (BBP). A total of 901 participants were invited via text messaging to complete the first online survey on 22 February 2020 and the second survey on 24 April 2020. Both surveys were conducted during the COVID-19 pandemic in mainland China (see Fig. 1A for details). Responses within 1 week of the invitation were considered valid. Out of the invitees, 735 responded to both surveys (209 males; 16–22 yeas, mean age = 18.907 ± 0.891 years; 2 participants over the age of 26 were excluded to increase age homogeneity). We had resting-state functional magnetic resonance imaging (rs-fMRI), collected from October 2019 to December 2019, for 500 of these participants. Two of these 500 imaging records were excluded for head motion over 2 mm. All participants reported no history of neurological or psychiatric illness and gave written informed consent that was approved by local IRB prior to the MRI scanning.
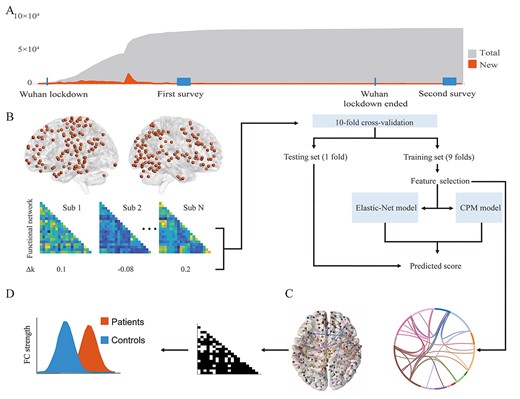
(A) X-axis indicates timeline of the data acquisition of the 2 surveys and 2 important events during the COVID-19 pandemic in China. The Y-axis indicates new cases per day and total cases of COVID-19 in China. On 23 January 2020, China imposed a strict lockdown in Wuhan. We conducted the first online survey on 22 February, and the second survey on 24 April 2020. Both surveys completed within a week. (B) The pipeline of brain connectome prediction framework. Functional connectivity maps (FCs) were extracted by power-264 atlas from preprocessed rs-fMRI data. We conducted 10-fold cross-validation procedures to predict changes of delay discounting rates during pandemic. (C) Consensus functional connectivity for visualization. Consensus FCs were defined as overlapping FCs among folds. (D) Applying binarized consensus functional connectivity to external data sets for clinical extension and increased external validity.
To validate and generalize our results and to explore their clinical relevance, 3 additional samples (secondary data sources) were obtained. The first one was the REST-meta-MDD Consortium Data Sharing project (Yan et al. 2019), which contained processed neuroimaging data of 1300 depressed patients and 1128 normal controls from 25 research groups. The second one was shared by the Center for Biomedical Research Excellence (COBRE), which contained original resting state and structural imaging data from 72 patients with schizophrenia and 75 healthy controls (Mayer et al. 2013). The last one was OpenNeuro Dataset ds001461 (Liao and Fan 2020), containing original resting-state imaging data from 47 patients with schizophrenia and 34 healthy controls. The characteristics of the 4 samples are given in Table 1.
Datasets . | Data collection period . | N . | Male . | Age (years) . | |
---|---|---|---|---|---|
Mean . | SD . | ||||
First pandemic survey | 22–28 February 2020 | 901 | 273 | 18.91 | 1.13 |
Second pandemic survey | 24 April–1 May 2020 | 824 | 239 | 18.94 | 1.02 |
MRI data | September–December 2019 | 498 | 139 | 18.98 | 0.96 |
REST-meta-MDD Consortium (Yan et al. 2019) | |||||
Depressed group | Before pandemic | 1225 | 449 | 36.15 | 14.73 |
Normal control group | 1068 | 444 | 36.16 | 15.82 | |
COBRE (Mayer et al. 2013) | |||||
Schizophrenia group | Before pandemic | 43 | 36 | 34.49 | 13.48 |
Normal control group | 60 | 41 | 34.90 | 11.54 | |
OpenNeuro Dataset ds001461 (Liao and Fan 2020) | |||||
Schizophrenia group | Before pandemic | 40 | 25 | 31.13 | 9.59 |
Normal control group | 30 | 21 | 35.17 | 9.67 |
Datasets . | Data collection period . | N . | Male . | Age (years) . | |
---|---|---|---|---|---|
Mean . | SD . | ||||
First pandemic survey | 22–28 February 2020 | 901 | 273 | 18.91 | 1.13 |
Second pandemic survey | 24 April–1 May 2020 | 824 | 239 | 18.94 | 1.02 |
MRI data | September–December 2019 | 498 | 139 | 18.98 | 0.96 |
REST-meta-MDD Consortium (Yan et al. 2019) | |||||
Depressed group | Before pandemic | 1225 | 449 | 36.15 | 14.73 |
Normal control group | 1068 | 444 | 36.16 | 15.82 | |
COBRE (Mayer et al. 2013) | |||||
Schizophrenia group | Before pandemic | 43 | 36 | 34.49 | 13.48 |
Normal control group | 60 | 41 | 34.90 | 11.54 | |
OpenNeuro Dataset ds001461 (Liao and Fan 2020) | |||||
Schizophrenia group | Before pandemic | 40 | 25 | 31.13 | 9.59 |
Normal control group | 30 | 21 | 35.17 | 9.67 |
Datasets . | Data collection period . | N . | Male . | Age (years) . | |
---|---|---|---|---|---|
Mean . | SD . | ||||
First pandemic survey | 22–28 February 2020 | 901 | 273 | 18.91 | 1.13 |
Second pandemic survey | 24 April–1 May 2020 | 824 | 239 | 18.94 | 1.02 |
MRI data | September–December 2019 | 498 | 139 | 18.98 | 0.96 |
REST-meta-MDD Consortium (Yan et al. 2019) | |||||
Depressed group | Before pandemic | 1225 | 449 | 36.15 | 14.73 |
Normal control group | 1068 | 444 | 36.16 | 15.82 | |
COBRE (Mayer et al. 2013) | |||||
Schizophrenia group | Before pandemic | 43 | 36 | 34.49 | 13.48 |
Normal control group | 60 | 41 | 34.90 | 11.54 | |
OpenNeuro Dataset ds001461 (Liao and Fan 2020) | |||||
Schizophrenia group | Before pandemic | 40 | 25 | 31.13 | 9.59 |
Normal control group | 30 | 21 | 35.17 | 9.67 |
Datasets . | Data collection period . | N . | Male . | Age (years) . | |
---|---|---|---|---|---|
Mean . | SD . | ||||
First pandemic survey | 22–28 February 2020 | 901 | 273 | 18.91 | 1.13 |
Second pandemic survey | 24 April–1 May 2020 | 824 | 239 | 18.94 | 1.02 |
MRI data | September–December 2019 | 498 | 139 | 18.98 | 0.96 |
REST-meta-MDD Consortium (Yan et al. 2019) | |||||
Depressed group | Before pandemic | 1225 | 449 | 36.15 | 14.73 |
Normal control group | 1068 | 444 | 36.16 | 15.82 | |
COBRE (Mayer et al. 2013) | |||||
Schizophrenia group | Before pandemic | 43 | 36 | 34.49 | 13.48 |
Normal control group | 60 | 41 | 34.90 | 11.54 | |
OpenNeuro Dataset ds001461 (Liao and Fan 2020) | |||||
Schizophrenia group | Before pandemic | 40 | 25 | 31.13 | 9.59 |
Normal control group | 30 | 21 | 35.17 | 9.67 |
Delay Discounting Measurement
The 27 items Monetary Choice Questionnaire (MCQ-27) (Kirby et al. 1999) was used to measure delay discounting. It is easy and feasible to implement in a survey running during the COVID-19 pandemic. Each MCQ-27 item asked participants to choose between a smaller immediate reward (e.g., ¥25 today) and a larger delayed reward (e.g., ¥30 in 80 days). The monetary rewards in choices ranged from ¥11 to ¥85. They were divided into 3 sizes: S = small, from 25 to 35; M = medium, from 50 to 60; and L = Large, from 75 to 85 according to the sizes of delayed rewards. The delays ranged from 1 week to 186 days (Kirby et al. 1999). We simply replaced the USD ($) with Yuan (¥) because the World Bank data (https://data.worldbank.org) shows that in 2018, the per capita GNI of China and the USA were comparable (60 011 Yuan vs. 60 524 USD, respectively) (constant LCU). The delay discounting rate (k value) was calculated using the hyperbolic temporal discounting functions (Mazur 1987): V = A/(1 + kD) where V is the present value of the delayed reward A at delay D. We used a spreadsheet application (Kaplan et al. 2014) to compute k value and choice consistency. Consistency represents the consistency of choices. For example, participants choosing 25 RMB today over 60 RMB in 2 weeks in one trial, but choosing 30 RMB in 80 days over 25 RMB today in another trial will impair the consistency indicator. Participants with choice consistency lower than 0.80 (Gray et al. 2016) in either survey of any monetary size were excluded from further analysis (n = 52, about 6.9%) to increase the accuracy of k estimation. The geometric mean of k value (geometric mean of 3 sizes) was calculated as the index of participants’ delay discounting rate. The k difference (Δk) between the second survey (k2) and the first survey (k1) was calculated as an indicator of delayed discounting changes during the pandemic. In order to get accurate Δk, the participants whose k exceed the measurement range (0.00016–0.25) in either survey were excluded from further analysis on Δk.
Functional MRI Data Acquisition
The prepandemic participants underwent an 8-minute rs-fMRI scanning using a 3T SIEMENS PRISMA scanner (Erlangen, Germany) at Southwest University Brain Imaging Center. Participants were asked to keep their eyes open, but not to sleep or think of anything specific, and to keep their head still as much as possible. A total of 240 functional volumes were obtained with the following scanning parameters: repetition time [TR]/echo time [TE] = 2000/30 ms, field of view [FOV] = 224 × 224 mm2, and flip angle = 90°. A cushioned head fixation device was used to minimize head movements.
Image Preprocessing
Data preprocessing was accomplished with the tool Data Processing Assistant for resting-state fMRI (Yan et al. 2016). The first 10 images were discarded to allow the equilibration of the signal. Slice timing and realignment were then performed. The resulted realigned images were normalized using Montreal Neurological Institute echo-planar imaging template, resampled into 3 × 3 × 3 mm3 resolution, and smoothed with 4 mm FWHM Gaussian kernel. The nuisance signals (i.e., WM, CFS, Friston 24 parameters) were regressed to control for potential artifacts. The temporal band-pass filter (0.01–0.08 Hz) and linear detrending were also applied to the image. A scrubbing method was used to remove bad volume caused by head motion (Power et al. 2012).
Brain Network Construction
The preprocessed functional MRI data were parcellated using a large meta-analysis of whole-brain functional connectivity (FC) maps (Power-264) (Power et al. 2011), resulting in 264 regions of interests (ROIs, nodes) for calculating FC coefficients (see Fig. 1B). Time series within each node was obtained for everyone by averaging fMRI time series over all voxels in each of the 264 ROIs. Then, we calculated Pearson correlation coefficients of each pair of time series and transformed to Fisher’s Z score. For the fact that matrices are symmetric, after removing 264 diagonal elements, the lower triangle of elements of the FC matrix was retained for the following prediction. That is, a total of (264 × 263)/2 = 34 716 edges (FC) were prepared as whole-brain features for each participants.
Predictive Analysis
We performed elastic-net regression to evaluate whether the prepandemic functional connectome predicted changes in delay discounting rates (Δk) between the second and first surveys in males. Elastic-net regression is a linear sparse kernel multivariate regression method, essentially a combination of LASSO regression and ridge regression (Zou and Hastie 2005). Using elastic-net regression in FC modeling is common, because it reduces the effect of multicollinearity on feature selection (Trzepacz et al. 2014; Teipel et al. 2015; de Vos et al. 2016; Schouten et al. 2016). The MATALB built-in LASSO algorithm with 2 main parameters was implemented in this study. The regularization coefficients lambda (λ) was optimized by nested 10-fold cross-validation (CV) with minimal mean-squared error within a training set. The identical optimal lambda was applied to the testing sets. The parameter alpha (α) that controls the weight of LASSO (L1) versus ridge (L2) optimization is a positive scalar value in the interval [0, 1]. It was chosen from 11 values, that is, [0.1−7 0.1 0.2 0.3… 1] outside of the CV loop. When alpha value equals 1, the process is equivalent to a LASSO regression. When the value is close to 0, the process resembles a ridge regression.
The procedure mainly includes the following steps (He et al. 2021): (1) divide participants into training and testing sets, (2) feature selection, (3) model building, (4) assessment of prediction performance, and (5) permutation test (see Fig. 1B). First, participants were divided into 10 subsets by using 10-fold CV. Nine-folds were randomly selected to build the predictive model (training) and 1-fold to evaluate the predictive power (testing). The process was repeated 9 more times to complete the CV procedure. Features were selected in training set by the statistical significance of correlation coefficients between FCs and Δk (P values: 0.05, 0.01, 0.005, and 0.001. P values were defined out of the CV loop). Confound variables (age, head motion) were controlled for by partial correlation during feature selection. The elastic-net regression model was built to fit the selected features and Δk. The test sets were fed into the model to get predicted Δk. After the CV was completed, the predicted score for each participant was obtained. The CV procedure was repeated 100 times for stability. We then calculated the mean of all the predicted scores. Pearson correlation coefficients between true and predicted Δk were calculated to evaluate the prediction performance. Lastly, 2000 times permutation tests were conducted to evaluate the significance of the correlation coefficients. Permutation test calculated possible models and statistical values by randomly rearranging the labels (Δk) for the data (FCs). The significance of the permutation test (ppt) was calculated as the proportion of sampled permutations that are greater or equal to the true predicted correlation. To further validate, we also tried to replace Δk with a series of random numbers, and see if the model will report a false positive result.
Internal Validation with Connectome-Based Predictive Modeling
Connectome-based predictive modeling (CPM) is a data-driven protocol based on linear relationships typically with a slope and an intercept (i.e., y = mx + b) for brain-behavior prediction (Shen et al. 2017). CPM may not be optimal for capturing brain–behavior relationships but is advantageous in that it provides a more interpretable model and improves the rejection rate of false positives (Shen et al. 2017). Features were selected by significance of correlation coefficients (r) between brain and behavior. They were split into positive (r > 0) and negative (r < 0) features. Then the selected features were summed to fit the model separately. Other steps followed the abovementioned CV process. We also conducted CPM based on the commonly used shen-268 atlas (Shen et al. 2017), to increase the reliability of prediction results. We also tried to predict k1 and k2 separately, to explore differences and communalities between Δk and k.
Clinical Relevance
Consensus FC among folds were selected for the clinical extension of our study. Consensus FC would have small changes due to different splits of folds. We repeated the CV process 1000 times (10 folds × 100 repetitions) to get more stable results. The 95% overlapping FCs in repetitions were binarized as feature selection masks that applied to external clinical rs-fMRI data. The REST-meta-MDD Consortium Data contains processed ROI signals based on Power-264 atlas. We compared the consensus features of normal controls and MDD patients on the data share by REST-meta-MDD (Yan et al. 2019). The consensus masks were also applied to the COBRE schizophrenia data (Mayer et al. 2013) and external data provided by Liao (Liao and Fan 2020), to compare normal controls and schizophrenia patients (see Fig. 1D). These 2 secondary datasets were processed in the same pipeline as the BBP data we collected.
Code and Data Availability
The MATALB built-in LASSO algorithm was used to implement elastic-net regression. The connectome predictive model scripts was used to implement linear-based prediction model (Shen et al. 2017). Feature visualization uses NeuroMArVL (https://immersive.erc.monash.edu/neuromarvl/). The REST-meta-MDD Consortium Data Sharing project (Yan et al. 2019), COBRE (Mayer et al. 2013), and OpenNeuro Dataset ds001461 (Liao and Fan 2020) are available online. The code used in this study is available on github (https://github.com/gbShaw/DDTconnectome), and the behavioral data and ROI signals data are available from the authors upon request.
RESULTS
Behavioral Data
From the invitees, 683 participants (190 males) completed both surveys with valid geometric k values (consistency > 0.80). A mixed-design ANOVA (2 × 2) was used to examine differences in delay discounting rate (natural log transformed geometric k; view the distributions in Fig. 2), with time of assessment (t1 vs. t2) as repeated-measures variable, and sex (male vs. female) as a between-subjects variable. There was a significant time × sex interaction (F(1, 681) = 3.956, P = 0.047, partial η2 = 0.006, 95% CI = [3.82 × 10−5 0.02], see Fig. 2C). The main effects of sex (F(1, 681) = 1.071, P = 0.302) and time (F(1, 681) = 3.127, P = 0.077) were nonsignificant. Simple main effect analysis revealed that males’ delay discounting rate increased during the pandemic (F(1,681) = 4.889, P = 0.027, partial η2 = 0.007, 95% CI = [4.14 × 10−4 0.02]), but females’ did not (F(1,681) = 0.044, P = 0.834, partial η2 = 0.000, 95% CI = [0 2.80 × 10−3]). ANOVA conducted on samples in the predictive analysis (n = 335; 88 males) showed the same result (Supplementary Table S1). These finding indicated increased delay discounting rates in males during the pandemic; males increased their preference for small immediate rewards compared with larger delayed rewards. The results can be interpreted as showing that males responded to the stress-induced by the pandemic differently than females did.
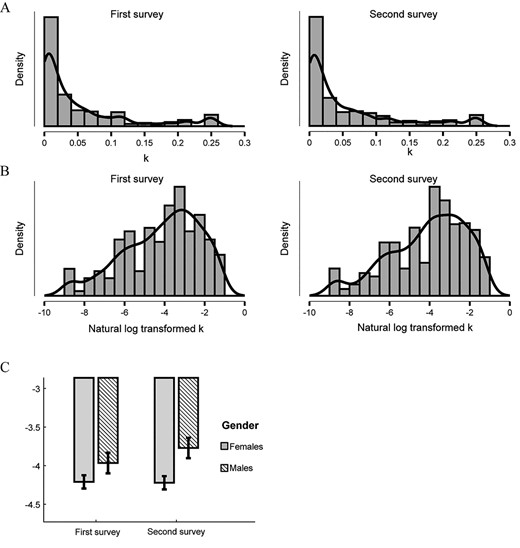
(A) Distributions of discounting rates of first survey and second survey. (B) Distributions of natural log transformed k. (C) Interaction between time of assessment and sexes. The plot and the error bar show mean ± 95% CI of log transformed k.
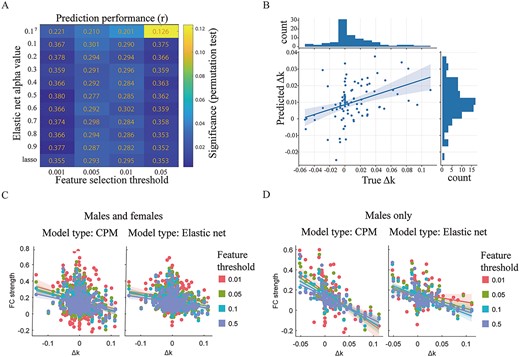
(A) Prediction performance of elastic regression. Numeric labels over the heat map indicate the correlation coefficients between predicted Δk and true Δk. Colors indicates significance (2000 times permutation test) of correlation coefficients. X-axis indicates feature selection threshold. Y-axis indicates value of parameter α in elastic regression. (B) Correlation between predicted Δk and true Δk (optimal prediction performance). (C) Correlation coefficients between summed FC strength within consensus connectivity and Δk for both sexes (n = 335). (D) Correlation coefficients between summed FC strength within consensus connectivity and Δk in males (n = 88).
Prediction of Male’s Increased Discounting Rates
The prepandemic functional connectome predicted increased discounting rate in males from the first survey to the second survey (88 males) using elastic-net regression (optimal prediction performance: r = 0.380, ppt < 0.01, α = 0.5. See Fig. 3B and Supplementary Table S2 for details) after controlling for head motion and age. The parameter alpha (α) that controls the weight of LASSO (L1) versus ridge (L2) optimization slightly impacted prediction performance (see Fig. 3A for details). This result has been verified with additional method (CPM: r = 0.234, ppt < 0.01, optimal performance). Consensus features extracted by CPM (see Fig. 4) and elastic-net were almost the same (Supplementary Fig. S1). The summed strength of selected consensus features showed significant negative correlation with increased discounting (Δk) in males (highest correlation: r = −0.719, P < 0.001, feature selection threshold = 0.01. See Fig. 3C and D and Supplementary Table S3 for details). In other words, decreased overall strength of the network was related to the increase in delayed discounting. Except for the 2 connections within the DMN, the other connections are internetwork connections (Fig. 4). All of these internetwork connections showed consistent negative correlation with Δk (Supplementary Table S5). The CPM results mainly based on negative correlated features, except for when feature selection is set by 0.001 (Supplementary Table S2). The CPM methods conducted with shen-268 atlas largely supported this view, it showed slight prediction power (r = 0.154, ppt = 0.054). The FCs that predicted Δk showed slight predictive performance on k1 (optimal performance: r = 0.148, ppt = 0.024) and k2 (r = 0.137, ppt = 0.031), but was not stable across feature selection thresholds (Supplementary Table S4). The whole-brain analysis showed better performance on k1 (optimal performance: r = 0.182, ppt = 0.030) and k2 (r = 0.158, ppt = 0.080) across thresholds (Supplementary Table S2). The FCs that predicted k and Δk involve many of the same networks. However, they are very different at the node level (Supplementary Fig. S2). Randomly generated behavioral changes could not be predicted by the whole-brain (r = −0.02 ± 0.11 SD, 1000 iterations) and the discovered connectome (r = −0.10 ± 0.13 SD, 1000 iterations).
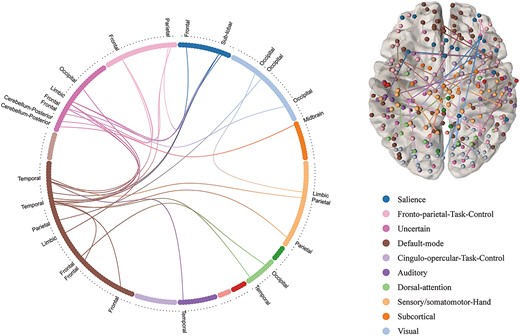
Consensus function connectivity pattern extracted by CPM. Features were selected by correlation coefficients which under selection threshold (P < 0.01) and valence of correlation (negative). Functional connectivity points to overlapping functional connections in iterations (10 × 100 iterations; 95% occurrence).
Clinical Extension
Due to missing power264 atlas ROI signals, only 2293 imaging records (1225 patients and 1068 normal controls) in the MDD dataset (2428 in total) were included in our analysis. The MDD patients showed significantly lower FC strength within the consensus feature mask compared with healthy controls (t = 2.007, P < 0.05, 95% CI = [0.000 0.013], Cohen's d = 0.084, feature selection thresh = 0.01). The feature selection threshold slightly affected the result (see Fig. 5A and Supplementary Table S6 for details).
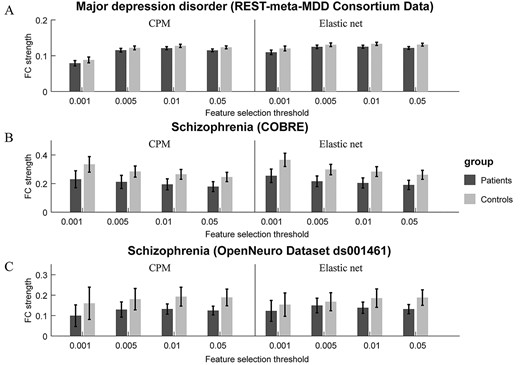
(A) the differences of summed FCs strength within consensus features between MDD patients and normal controls in the REST-meta-MDD Consortium data set. (B) Differences between schizophrenia patients and normal controls in the COBRE dataset. (C) Differences between schizophrenia patients and normal controls in the OpenNeuro Dataset ds001461. The plot and the error bar show mean ± 95% CI.
The COBRE dataset had relatively large head motions. It resulted in including 103 participants after exclusion by head motion (i.e., >2 mm) and scrubbing (i.e., >30% of the acquired volumes, 45 volumes were scrubbed). The schizophrenia patients (n = 43) in the remaining COBRE imaging records showed significantly lower FC strength than normal controls (n = 60) within the consensus feature mask (t = 2.678, P < 0.01, 95% CI = [0.018 0.132], Cohen's d = 0.535, feature selection thresh = 0.01; see Fig. 5B and Supplementary Table S7). The patients (n = 40) in Liao’s study dataset also showed significantly lower FC strength (t = 2.509, P < 0.05, 95% CI = [0.012 0.109], Cohen's d = 0.606; see Fig. 5C and Supplementary Table S8) than normal controls (n = 30).
Discussion
In this longitudinal study, we first, found sex-based difference in changes in delay discounting during COVID-19. Second, we conducted a rs-fMRI analysis to reveal the FC patterns that underlie increased delay discounting rates in males during the pandemic (i.e., in response to stress). Third, we increased the validity and the potential practical implications of our findings by showing that the observed FC issues that underlie maladaptive delay discounting responses also explain differences between healthy samples and people with psychiatric disorders that involve elevated delayed discounting, specifically, major depression disorder and schizophrenia. To obtain these results, we implemented elastic-net regression to predict increased discounting rate in males with prepandemic FC. Further, to gain an intuitive understanding of the connectivity pattern, we illustrated a linear relationship between FC and increased delay discounting rate by CPM. We found robust predictive patterns under distinct parameters: increased discounting rates of males were positively correlated with the decrease of FC strength within the consensus features. The major depression disorder patients and schizophrenia patients also showed deficit in the strength of FC within the consensus features. These findings ultimately suggest that the delay discounting of males was susceptible to increases during the COVID-19 pandemic and point to neural markers that can explain individual differences in such responses to presumed stress in males. The observed neural markers of maladaptive delay discounting responses also showed clinical relevance and generalizability beyond our study and beyond healthy populations.
Delay Discounting of Males Increased during the Pandemic
Delay discounting of males increased between the two surveys during COVID-19 pandemic, whereas females’ delay discounting did not. Delay discounting has been associated with stress (Malesza 2019), including during the pandemic, (Craft et al. 2021; DeAngelis et al. 2021), which has been a source of stress (Charles et al. 2021; Liu et al. 2021). To illustrate, participants’ anxiety increased from the first survey period (February 2019) to the second survey period (April 2019) during the pandemic (He et al. 2021), even though the pandemic was under more control in China during the second survey. Lifting restrictions may be assumed to reduce pressure. However, we have found various studies demonstrating that the progress of the pandemic and its detrimental psychological consequence are not synchronized (i.e., there is often a lag). Detrimental psychological consequences of the COVID-19 pandemic persisted for several months after the end of the lockdown measures in Austria (Pieh et al. 2021). People in Italy showed higher stress levels at the end of lockdown compared with the start of the lockdown (Roma et al. 2020). Anxiety, depression, and stress levels were higher during remission than during lockdown in Spain (Planchuelo-Gómez et al. 2020). This continuous impact may be caused by complex reasons. In China mainland, researchers found stress, anxiety, and depression growth in the postlockdown period (Li et al. 2020). Many studies have shown the delayed effects of the pandemic or lockdown on psychological distress (Gan et al. 2020; Muehlschlegel et al. 2021). A systematic review found that the pandemic or lockdown have persistent impacts on symptoms of depression, anxiety, and trauma and stressor related disorders even 1–6 months after the end of lockdown. Psychological distress, alcohol abuse/dependence, and financial concerns have been described to persist over 6 months after relief in pandemic pressures (Muehlschlegel et al. 2021). This can be problematic, as individuals are inclined to engage in impulsive and sometimes risky behaviors in order to reduce stress or because they lack self-control resources that have been consumed by the ongoing stress coping (Lempert et al. 2012). This is in line with our findings that show changes in delay discounting that reflect greater impulsivity at t2 in males, compared with t1.
Further in line with our findings, patients with COVID-19 also showed significant sex-based differences in cortisol levels (Etoga et al. 2021), which is consistent with previous results that cortisol responses to stress were higher in males compared with females (Kirschbaum et al. 1992; Kajantie and Phillips 2006). The sex-based differences in stress response can explain why males and not females experienced maladaptive changes in delay discounting during the pandemic. In turn, changes in delay discounting in males can explain the increase in risky and deviant male-dominant behaviors during the pandemic, for example, some violent crimes, though the nuances of this association require further research. This idea is conceptually supported, though, by findings showing that exposure to a stressor and exogenously induced psychosocial stress significantly increase risk-aversion in males (Cahlíková and Cingl 2017).
Importantly, prior research typically used experiments to induce stress and to establish the association between preferences for immediate rewards and risk (Mishra and Lalumière 2017; Johnson et al. 2020). We extend this idea to a real-world setting by focusing on a natural stressful event (i.e., COVID-19) that resulted in population-level behavioral changes in impulsive and risky behaviors (Håkansson 2020; Rmandic et al. 2020; Zhang 2020; Sutherland et al. 2021).
There is a tradition in some strands of stress research to use only male participants as a way to avoid female confounds such as hormonal contraceptives and menstrual cycle (Kirschbaum et al. 1999). Our findings suggest that this practice is unhealthy, beyond its inclusivity ramifications, as it can lead to an incomplete and inaccurate picture. This is because our findings point to sex-based differences in responses to stress in form of changes in delay discounting under stress. The inclusion of both sexes in such studies is especially important due to the robust association between delay discounting and many psychiatric disorders (Amlung et al. 2019).
Prepandemic Connectome Predicted Changes of Discounting Rates
The prepandemic FC predicted the increased delay discounting in males via multiple methods: elastic-net regression, LASSO regression, and CPM. The linear relationship between FC and increased delay discounting were clarified by CPM, which suggested that the increased delay discounting may be related to the declined strength of the consensus FC network. The CPM result was also largely validated by the shen-268 atlas. These findings suggest that the intrinsic functional connectivity serves as a neural marker of discounting preference changes under stressful events. The decision impulsivity under stressful events came from a highly integrated brain connections involving multiple internal networks, including salience (SN), default-mode (DMN), frontal parietal (FPN), dorsal attention (DAN), somato-motor (SMN), and visual network (VN). The pattern showed commonalities and differences compared with the pattern of delay discounting (Cai et al. 2020). We found the 5 of the 6 networks are shared: SN, DMN, DAN, SMN, and VN. The FPN is unique to stress-related discounting changes as it mediates cognitive control (Martín-Signes et al. 2021; Nee 2021), which was considered to be an important factor affecting delay discounting (Kim et al. 2012; Steinbeis and Crone 2016) and was found as an important factor affecting COVID-19-related stress (Khoo et al. 2021) and anxiety (Demlrtas 2021). The subcortical network was not found to be significant in our study. Because our results are based on Power-264 atlas which did not contain enough nodes within the subcortical network, conclusions about the subcortical network, for example, that it is unique to delay discounting, should be interpreted with caution.
Although the functional connectome we found as a neuromarker may be helpful for diagnosis and regulation (Yang and Zuo 2015), machine learning models have limited interpretability. However, the CPM method is based on a unary linear model, which simply sums strength of all selected connectivities. It therefore provides a way to explore the linear relationship between the strength of the connectome and the behavioral change (Shen et al. 2017). We found that negatively correlated features can accurately predict behavior under various thresholds, but only a small number of positively correlated connectivities can predict behavior changes. This means that maladaptive behavior changes are related to the reduction of a wide range of functional connections, except for a small number of connections that are enhanced. Many studies have found association between higher discounting and diminished engagement of prefrontal cortex related connectivity (Peper et al. 2012; Stanger et al. 2013; Zhang et al. 2021). However, our results point to the reduction of a more extensive functional connectivity that drives increased discounting. Moreover, there is another important feature of this pattern. Except for the 2 connections within the DMN, the other connections are internetwork connections (Fig. 4). That is, the weakened communication between networks is an important reason for this maladaptive behavior. This is in addition to the existing evidence of impulse-related change in connectivity between networks (Zhu et al. 2017; Chen et al. 2018).We used multiple thresholds to extract features, which is useful for in-depth understanding of connectome features. We found that from a broader perspective, the FCs are all reduced in maladaptive DDT, but only when the focus is small, some enhanced connections can be found in maladaptive DDT. This prompts us to be cautious in choosing a specific threshold and interpreting the results. Specifically, the large-scale decline in this comprehensive internetwork communication is importantly related to the increase in delay discounting during the pandemic. We also found that, in addition to changes in delay discounting, discounting can also be predicted by brain connectome. They involve many common networks, but are still different at the node level. These results indicated that the change of k may have unique brain mechanisms, beyond those that explain k.
Our Observed Neuromarkers Distinguish Psychiatric Patients from Controls
We extended the neural pattern to external clinical data, including major depression and schizophrenia patients and healthy controls. The results found that the patient’s functional connectivity strength, as identified in our study to be consistent with elevated delay discounting changes, showed deficit within the consensus functional connectivity. These results suggested that, in addition to the reported association between delay discounting and psychiatric disorders at the behavioral level (Amlung et al. 2019), there could be some shared intrinsic neural substrates between increased and maladaptive delay discounting under stressful events and psychiatric disorders (at least in major depression and schizophrenia). The consensus features contained elements of the default-mode network, which is typically deficient in major depression patients (Yan et al. 2019). Disrupted activity and connectivity of the default-mode network also characterizes schizophrenia patients (Hu et al. 2017). It is worth noting that patterns in our study are almost all internetwork connections (Fig. 4), and this is different from the research on the internal strength of a single or multiple networks. The connectivity strength between networks in the connectome declined. This points to possible alteration in the connectivity between networks in patients (Lancaster and Hall 2015; Li et al. 2017), and it also supplements the whole-brain connectome perspective. Thus, our findings can serve as a basis for studying the ability of our neural markers to detect the risk for MDD and/or schizophrenia. These findings further show that the link between the identified connectome issues and maladaptive delay discounting changes likely extend from normal and healthy populations, to populations with psychiatric disorders.
Limitation and Direction
Several limitations of this study are noteworthy. First, delay discounting shows changes along the lifespan (Blakemore and Robbins 2012; Anandakumar et al. 2018). Here, we collected data from undergraduate students, with limited age variability. Thus, our results do not capture the developmental characteristics of delay discounting. Further work should employ samples with larger variation in ages. Second, although we employed multiple machine learning methods to uncover the neural substrates of delay discounting, further studies can explore a classifier that can provide early warning for steep discounting rates to provide a deeper understanding of potential health risk caused by steep discounting rates.
Conclusion
This longitudinal study demonstrates that there is a difference between the sexes in maladaptive changes in delay discounting under stressful events. Our findings further show that the prepandemic functional connectome predicts increased delay discounting in males in response to stressful events. In addition, we show that this functional connectivity could effectively separate major depression disorder and schizophrenia patients from healthy controls. These finding contribute to understanding the sex-based differences and neural substrates of maladaptive changes in delay discounting under stressful events. They can explain the association between delay discounting and psychiatric disorders, and the population-level increase in various male-dominant impulsive and risky behaviors during the pandemic. As such, our findings have important implications for society, healthcare providers, criminologists, psychologists, and psychiatrists.
Notes
Conflict of Interest: None declared.
Funding
The National Natural Science Foundation of China (31972906), Natural Science Foundation of Chongqing (cstc2020jcyj-msxmX0215), and the High-end Foreign Expert Introduction Program (G20190022029). Major depression disorder data were provided by the members of REST-meta-MDD Consortium (Yan et al. 2019).
Authors’ Contributions
XL, JQ, TF, HC, OT, and QH developed the study concept. All authors contributed to the study design. Testing and data collection were performed by ZX and QH. ZX performed the data analysis and interpretation under the supervision of QH. ZX and QH drafted the manuscript, and QW, AB and OT provided critical revisions. All authors approved the final version of the manuscript for submission.
References
He L, Wei D, Yang F, Zhang J, Cheng W, Feng J, Yang W, Zhuang K, Chen Q, Ren Z, et al.