-
PDF
- Split View
-
Views
-
Cite
Cite
Anne Löffler, Patrick Haggard, Stefan Bode, Decoding Changes of Mind in Voluntary Action—Dynamics of Intentional Choice Representations, Cerebral Cortex, Volume 30, Issue 3, March 2020, Pages 1199–1212, https://doi.org/10.1093/cercor/bhz160
- Share Icon Share
Abstract
Voluntary actions rely on appropriate flexibility of intentions. Usually, we should pursue our goals, but sometimes we should change goals if they become too costly to achieve. Using functional magnetic resonance imaging, we investigated the neural dynamics underlying the capacity to change one’s mind based on new information after action onset. Multivariate pattern analyses revealed that in visual areas, neural representations of intentional choice between 2 visual stimuli were unchanged by additional decision-relevant information. However, in fronto-parietal cortex, representations changed dynamically as decisions evolved. Precuneus, angular gyrus, and dorsolateral prefrontal cortex encoded new externally cued rewards/costs that guided subsequent changes of mind. Activity in medial frontal cortex predicted changes of mind when participants detached from externally cued evidence, suggesting a role in endogenous decision updates. Finally, trials with changes of mind were associated with an increase in functional connectivity between fronto-parietal areas, allowing for integration of various endogenous and exogenous decision components to generate a distributed consensus about whether to pursue or abandon an initial intention. In conclusion, local and global dynamics of choice representations in fronto-parietal cortex allow agents to maintain the balance between adapting to changing environments versus pursuing internal goals.
Introduction
Adaptive behavior in a complex world requires continuous and dynamic decision-making. “Changes of mind” (CoM) are a striking example of our ability to flexibly reverse decisions and adjust our actions accordingly. While we are constantly reacting to our environment, another crucial feature of being human is that we experience being able to act freely and to follow internal goals. Surprisingly, however, previous studies investigating CoM have predominantly focused on perceptual decisions, which are driven by external evidence (e.g., Resulaj et al. 2009). Conversely, despite its utmost importance for everyday life, little is known about the neurocognitive mechanisms underlying CoM in voluntary action.
Voluntary actions are guided by internal (endogenous) decisions based on one’s own goals, preferences, or memory (Passingham 1987; Haggard 2008; Fried et al. 2017). However, external (exogenous) context can provide important information about actions and their respective values, such as their anticipated rewards and temporal/effort costs (McClure et al. 2004; Rudebeck et al. 2006; Rushworth 2008). Consequently, people may change an initial endogenous decision due to changes in the external environment (Wisniewski 2018). For example, after having invaded Russia, Napoleon decided to retreat from Moscow in 1812 when facing a harsh winter and burnt-down deserted cities. Even in everyday life, actions are often initiated based on incomplete or uncertain external information or circumstances might change before a decision is fully carried out. For instance, one might initially choose to walk to a shop to buy organic fruits, in line with internal goals, but if it begins to rain heavily, may decide to divert to a closer shop with non-organic produce. Voluntary actions typically have several counterfactual alternatives, linked to the feeling that one “could have done otherwise” (Kulakova et al. 2017; Rens et al. 2018)—and when action contexts change, one may in fact “do otherwise”.
Internally versus externally guided actions have previously been attributed to distinct medial versus lateral pathways of action selection, respectively (Passingham 1987). More specifically, voluntary intentions may be generated in media frontal cortex (MFC) and precuneus (PCUN) (Fried et al. 1991; Soon et al. 2008; Bode et al. 2011; Fried et al. 2011; Krieghoff et al. 2011; Bode et al. 2013; Soon et al. 2013; Zapparoli et al. 2018). MFC forms an “intentional hub” that integrates various components of voluntary decisions about what to do, when to do it, and whether to do it at all, whereas the role of parietal cortex appears to be more specific to voluntary “what” decisions (Zapparoli et al. 2018). PCUN may contribute to these decisions by resolving conflict when choice options appear to be equal, hence preventing behavioral stalemate (Bode et al. 2013). Lateral fronto-parietal areas, e.g., dorsolateral prefrontal cortex (dlPFC), might be important to represent counterfactual choice options after an initial voluntary decision has been made (Rens et al. 2018). Furthermore, a recent study showed that dlPFC can rapidly update value information of competing choice options (Voigt et al. 2019), which may then inform switches to an alternative choice option later on. Finally, angular gyrus (AG) has been implicated in shaping the conscious experience of voluntary actions by monitoring the implementation of intentions into motor actions and generating a predictive model of the upcoming movement (Sirigu et al. 2004; Chambon et al. 2012). AG might also contribute to monitoring discrepancies between intended and actual action outcomes upon action completion (Farrer et al. 2008) and therefore is involved at various stages of voluntary action.
However, previous studies investigating the role of fronto-parietal areas in volition have focused exclusively either on processes prior to action initiation to investigate how and when conscious voluntary intentions emerge (e.g., Soon et al. 2008; Chambon et al. 2012) or on processes that evaluate actions after they have already been completed (e.g., Farrer et al. 2008). By contrast, most previous studies have neglected processes that shape voluntary actions as they evolve. Consequently, little is known about how changes in external contextual information may lead to dynamic updating of evolving voluntary actions—in particular with regard to changes in higher-order endogenous intentions. This is surprising given that other aspects of action updates, such as mere motor reprogramming of reaching movements, have been studied in great detail (e.g., Wise and Mauritz 1985; Buch et al. 2010; Pastor-Bernier et al. 2012; Saberi-Moghadam et al. 2016). Additionally, updating processes have been central to understanding the neuro-cognitive mechanisms underlying other forms of decision-making. For example, for perceptual decisions, it has been shown that visual areas provide continuous updates of perceptual information (Tong et al. 1998; Polonsky et al. 2000), and additionally, posterior MFC gradually tracks sensory evidence after an initial decision has been made to signal the potential need for action updates (Fleming et al. 2018). For value-based decisions, several areas in prefrontal cortex, including MFC (Boorman et al. 2013; Kolling et al. 2016; Shenhav et al. 2016) and frontopolar cortex (Boorman et al. 2009), have been shown to continuously track the values of competing choice options and indicate the need to switch to an alternative option. Finally, switching between alternative choice options presumably relies on the engagement of cognitive control mechanisms in lateral and medial frontal cortex that are recruited based on anticipated rewards and costs (Dixon and Christoff 2012; Botvinick and Braver 2015).
Updates in value-based decisions are particularly relevant when values of competing choice options are uncertain or change dynamically, for example, in probabilistic reversal learning (Izquierdo et al. 2017) or foraging in dynamic environments where agents commonly face decisions between exploitation of a previous choice versus exploration of alternative choice options (Daw et al. 2006; Badre et al. 2012; Rushworth et al. 2012). However, choice reversals in these paradigms often occur across several trials or even blocks—and hence, are the result of relatively slow updates that rely on learning (Lee et al. 2014). Yet, choice values can be updated on a much faster time scale as decisions evolve (Voigt et al. 2019). Here, we applied an experimental approach that made use of such dynamic, value-based decision processes in order to study how initially endogenous intentions are updated when new externally cued value-based information needs to be integrated to guide ongoing actions as they evolve. Specifically, in our task, initial voluntary intentions were generated in the absence of external perceptual or value-based evidence. However, actions later evolved in a dynamic external environment that provided new information about trade-offs between temporal costs and rewards associated with the initial intention. This allowed us to address the yet unresolved questions of whether and how neural representations of voluntary decisions, which are at the core of our experience of being free agents, change as new contextual information becomes available and needs to be integrated.
Multivariate pattern analysis (MVPA) for functional magnetic resonance imaging (fMRI) data provided an optimized tool for this because, based on activity patterns in various decision-related brain areas, we could directly decode 1) the transition from initial endogenous decisions to later decision updates in a new external context and 2) the specific contextual information that was integrated to inform final decisions. By using cross-classification analyses, we further show in which brain regions neural representations of decisions remained stable despite changes in external context, or instead, changed when integrating new contextual evidence. Finally, functional connectivity analyses revealed how updated information represented in distinct brain areas may be integrated to guide dynamic action updates. In our task, participants first made endogenous decisions about arbitrary action goals (i.e., moving toward a face or a house stimulus), but then received new external information that changed the costs and rewards associated with achieving the endogenous goal (i.e., the remaining distance to the chosen stimulus vs. the reward for reaching it)—sometimes prompting a change of mind. Our results show that fronto-parietal areas not only generate endogenous intentions prior to action, but additionally, update and revise voluntary intentions continuously throughout an ongoing action by integrating new decision- and action-relevant information.
Materials and Methods
Participants
The study was approved by the Melbourne University Research Ethics Committee, and all participants provided written informed consent prior to the study. Participants were right-handed, healthy, with no history of psychiatric or neurological illness, and no contraindication for MRI. Initially, 37 participants were recruited for a behavioral training session (26 female, age: M = 23.3 yr, SD = 4.8). Based on performance criteria (see below), 30 participants were re-invited for the fMRI session. Of those, 1 participant withdrew after training, 1 participant was excluded due to excessive movement, and 3 participants were excluded because they did not meet the performance criteria in the fMRI session (see below). Hence, 25 participants (16 female, age: M = 23.4 yr, SD = 5.2) were included in the final fMRI data set. Participants received $10 for the behavioral session and $45 for the fMRI session, plus a small performance-based reward they could win in each session (max $5).
Apparatus and Stimuli
The experiment was programmed in Matlab R2014a and the Psychophysics Toolbox (Brainard 1997). Grayscale images of faces and houses (170 × 170 pixel) of equal luminance and spatial frequency were presented on a black background, at a viewing distance of 50 cm (training session) or 90 cm (fMRI session). Participants indicated their choices on a HHSC 2×2 button box, using their left and right index and middle fingers, which corresponded to 4 possible target positions (top/bottom left/right).
Double-Step Choice Task
Participants performed a computer-based decision-making task (Fig. 1), in which they could win points. For each 50 points they won, they received an additional 10 cents at the end of the study. At the beginning of each trial, 2 images of a face and a house were presented centrally either left/right or above/below a fixation cross for 1–2 s and participants had to make an endogenous decision between the 2 images (initial decision period). After a jittered delay of 3–9 s, the images were presented in 2 of 4 possible target locations. Target locations were assigned randomly (counter-balanced within blocks) with the restriction that the 2 images always appeared in neighboring locations (e.g., house in top-left and face in top-right location). Participants then had to press a response key that corresponded to the location of their initially chosen option, causing a manikin to move toward the chosen image at a constant and slow velocity of 60 pixel/s (2.2 cm/s). Only a single key press was required to initiate the movement of the manikin. Once the manikin reached the image, participants won 10 points.
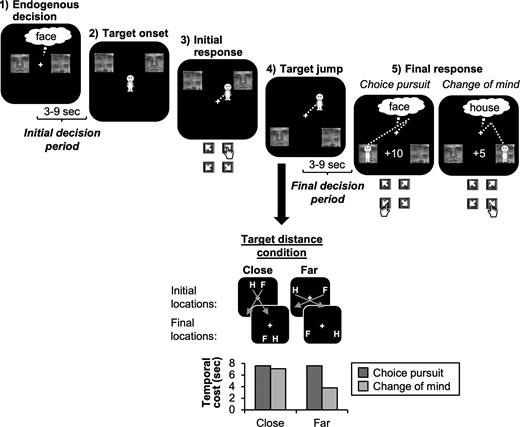
Double-step choice task (diagonal trials). Participants made an endogenous face/house decision (initial decision period) and then had to navigate a manikin to a target location by pressing the key corresponding to the location of the chosen image. On some trials, the images jumped to new locations after action onset and participants then had to decide whether to pursue their initial choice or change their mind and switch to the alternative option (final decision period). Final decisions depended on a trade-off between rewards and temporal costs: in diagonal trials, images jumped to the diagonally opposite location, and hence, participants could save time by switching to the alternative choice option for 5 points, instead of pursuing their initial choice for 10 points. Differences in temporal costs were particularly pronounced in the far-distance condition where temporal costs associated with choice pursuit were twice as large as costs associated with CoM (bottom). By contrast, in the close-target condition, costs were roughly equal for choice pursuit and CoM.
In the majority of trials, both images jumped to a new location once the manikin had traveled half the distance (~2.5 s) toward the initially chosen image. Participants then had to press a second response key to redirect the manikin to one of the new target locations. Crucially, target relocation did not simply require motor re-programming of actions, but additionally, required a second choice with regard to the image identity, and hence, a re-evaluation of the initial endogenous intention. Specifically, participants could freely choose to either stick with their initial choice (e.g., face for initial face choice) yielding 10 points or switch to the alternative option (e.g., house for initial face choice) for 5 points. Participants were told that they had exactly 1 h for the experiment in which they should win as many points as possible and that switching to the other option might sometimes allow them to save time and get to the next trial more quickly (and hence, complete more trials in the allocated time). By contrast, staying with the initially chosen option would win them more points on a given trial. Hence, final decisions depended on a trade-off between rewards and temporal costs. To allow for analyzing brain activity related to participants’ final decisions, the manikin stopped moving when the images changed their location, and participants had to make their final decisions within a jittered delay period of 3–9 s (final decision period). After the delay, the manikin turned green, which served as a go signal for participants to indicate their final decision.
In the main condition of interest (16/28 trials per run), the images jumped to the diagonally opposite locations (diagonal trials), causing the initially chosen option to be further away from the current position of the manikin than the alternative option. Hence, participants could save time by switching to the other image (CoM) instead of pursuing their initial choice. In order to induce CoM on some but not all trials with diagonal jumps, we additionally manipulated the distance between the images to vary cost-reward trade-offs. The distance of the targets to each other was either close (50% of trials) or far (50%). Importantly, the images were always equally far away from the center of the screen, but the difference in distance between them caused differences in the relative temporal cost associated with each image after target jump. In the far-target condition, the temporal cost of the originally chosen image was roughly twice as large (~7.6 s) as for the alternative image (~3.6 s). Hence, participants should be more likely to change their mind. In the close-target condition, the difference in temporal cost was negligible (~7.6 s for choice pursuit vs. ~ 7.2 s for CoM), and hence, participants should be more likely to stick with their initial choice given the higher reward for intention pursuit. The precise distances were adjusted slightly according to each individual’s cost-reward trade-off during practice (see below). Finally, in 2 control conditions, the images jumped in a parallel manner (4/28 trials) or did not jump at all (4/28 trials). These trials served to prevent participants from using stereotypical choice strategies and anticipating if and how the location of the choice options would change. Following a parallel jump, the initial choice option was always closer, regardless of target distance. Consequently, participants should always pursue their initial choice in this condition.
Since we aimed to measure brain activity related to the (abstract) initial choice before target locations were revealed, it was essential that participants indeed chose between the face and house at trial start (frame 1, Fig. 1), rather than waiting for target onset (frame 2, Fig. 1). In the instructions, we stressed that participants should always make a choice between the face and the house as soon as the 2 images were presented on the screen, and participants were reminded of this instruction between runs. We further used randomly inserted short trials (4/28 per run) to ensure compliance with this task instruction. In short trials, the fixation cross turned green and participants then had to respond to the location of their chosen image as fast and accurately as possible to win 50 points. The choice options were only presented very briefly (200 ms) and appeared in diagonally opposite locations, making it more difficult for participants to select a correct response. Participants were instructed that, to be fast and accurate, it would help them to make a choice at the very beginning of every trial and then focus their attention on the choice they made. The point in time at which the fixation cross turned green randomly varied between 0.5–9 s to ensure that participants focused on their decision throughout the entire initial choice period. The reaction time (RT) cut-off for short trials was adjusted based on each individual’s RT during training, such that each participant responded fast enough in ~75% of all short trials. In all other trial conditions, participants did not have to respond quickly, but were asked to be as accurate as possible.
Behavioral Training Session
Before the fMRI session, all participants were invited for a behavioral training session to 1) familiarize participants with the task and 2) pre-select participants who followed task instructions and showed roughly balanced numbers of CoM and no-CoM trials in the diagonal-jump condition. The training session consisted of a short practice block followed by 3 blocks of 28 trials each. In the first 2 blocks, participants had to verbally indicate their choice at trial start, which helped participants to get used to making their choice at the very start of each trial and enabled us to provide feedback when participants pressed a wrong key (which resulted in a loss of 10 points). In the last block, participants were instructed to “say the choice in their head” (as they would in the fMRI session). For participants who showed a strong preference for either CoM or no-CoM choices, image distances were adjusted after each practice block in order to exaggerate differences in costs, and hence, motivate participants to stay or switch more often, respectively. However, for all participants who were selected for the fMRI session, highly similar image distances resulted (for a given participant, far targets were either 280 or 290 pixels apart, whereas close targets were 120 or 93 pixels apart, respectively). Participants were invited for the fMRI session if they reached the following performance criteria by block 3: 1) 6–10 CoM trials per run (37.5–62.5%) in the diagonal jump condition, 2) roughly balanced trial numbers for initial face/house choices across all trial conditions (37.5–62.5% for each choice category), 3) less than 10% errors across all trial conditions, and 4) less than 10% early responses during the final decision period (before the manikin turned green).
fMRI Session
In the fMRI session (2–7 days after training), participants were given ~1 h to complete as many trials as possible. Although participants were instructed that they had exactly 1 h to complete the task, unknown to them, this time limit was not strictly imposed. Instead, we prioritized implementing the optimal number of trials per experimental block (5 × 28 trials in total). Most participants finished the task in slightly less than 1 h, after which they were informed that they had completed the maximum number of trials. One participant only completed 4 blocks within the 1 h due to technical issues. All other participants were able to complete all 5 runs of 28 trials each. Because participants were not required to indicate their choices verbally, no error feedback could be provided. Instead, participants were asked to correct themselves when they made an error (which, however, only happened on 0.6% of trials). At the end of the fMRI session, participants were asked 1) on what percentage of trials they made a choice at trial start (rather than delaying it to target onset) and 2) what strategy they used to decide whether or not to switch to the alternative option when the images changed their location. All participants reported that they made initial choices in at least 90% of trials (M = 97.5%, SD = 3.5%) and that they used information about target distance to make CoM decisions.
fMRI Data Acquisition
Functional MRI volumes of the whole brain were acquired using a Siemens Skyra 3T scanner. A 20-channel head coil was used and volumes were acquired using gradient-echo echo-planar imaging (38 axial slices, repetition time TR = 2200 ms, voxel size: 3×3×3 mm without gap; echo time TE = 30 ms; flip angle 90 degrees). At the end of the fMRI session, high-resolution (1×1×1 mm) structural T1 scans were obtained for spatial normalization.
fMRI Analyses
The first 4 volumes of each run were discarded by default to avoid magnetic saturation effects. All remaining images were pre-processed using standard routines as implemented in SPM 12 (http://www.fil.ion.ucl.ac.uk/spm/), including slice-timing correction and correcting for head motion by realigning to the first image of the first run. No spatial normalization or smoothing was conducted at this stage to preserve the original information structure in each individual’s data. General linear models (GLMs) were then estimated for each individual participant using SPM 12, and motion correction parameters were included as regressors-of-no-interest. For each analysis, a separate first-level GLM analysis was performed where trials were labeled according to the conditions of interest: either 1) face/house choice, 2) left/right target location, 3) close/far target distance, or 4) CoM/no-CoM decision. For a given GLM, 1 beta map with parameter estimates for every voxel was created for each condition (e.g., face vs. house choice), separately for each of the 5 runs. The resulting run-wise regressors, rather than single trial regressors, were used for the following MVPA analyses to increase the signal-to-noise ratio, as it is standard in the field (e.g., Mourao-Miranda et al. 2006; Soon et al. 2008; Kruschwitz et al. 2018). Prior to decoding, estimates of each condition were z-standardized to rule out that differences in average signal strength between conditions might have been the underlying reason for pattern differences picked up by MVPA.
The following a priori defined regions of interest (ROIs) were included in the analyses: 1) inferior occipital gyrus (IOG) and 2) fusiform gyrus (FG), because choice behavior typically correlates with information processing in visual cortex and visual fixations on choice options (Krajbich et al. 2010; Voigt et al. 2019), 3) the PCUN (Soon et al. 2008; Bode et al. 2013), 4) AG (Farrer et al. 2008), 5) MFC; Soon et al. 2008; Bode et al. 2013; Soon et al. 2013; Zapparoli et al. 2018), and 6) dlPFC (Rens et al. 2018), given their consistent demonstrated involvement in most types of decision-making, including voluntary decisions. ROI masks were compiled using the Automated Anatomical Labelling atlas library (Tzourio-Mazoyer et al. 2002) in MarsBaR (Brett et al. 2002). Bilateral ROIs were generated by combining unilateral ROIs into a single mask. Given that some ROIs, especially PCUN, MFC, and dlPFC, were relatively large, the main analyses were repeated splitting these ROIs into dorsal and ventral sub-regions, which did not change the overall pattern of results (see Supplementary Material S1). Run-averaged beta estimates were extracted for all voxels within each ROI and served as inputs for separate MVPA analyses.
The Decoding Toolbox (Hebart et al. 2015) was used for MVPA. A linear support vector machine (SVM) with cost parameter C = 1 was trained to classify patterns of brain activity from all ROIs according to the categories of interest (e.g., face vs. house choices). A 5-fold cross-validation procedure was used in which each of the runs served as test run once with the remaining 4 runs serving as training samples. Decoding accuracy was then averaged across all runs for each subject. Finally, mean decoding accuracies were compared to accuracies obtained by permuting the labels (which resulted in roughly 50% decoding accuracy). One-tailed t-tests comparing actual decoding accuracies to the permutation tests are reported after Bonferroni correction for the number of ROIs in each analysis.
The main analyses focused on brain activity measured during the initial or final decision period. Event-related activity locked to stimulus onset (frame 1, Fig. 1) and target jump (frame 4, Fig. 1) was analyzed, respectively. For decoding analyses on initial decisions, all trial conditions were included, given that the initial decision period was identical across conditions. For analyses of final choices, only diagonal trials were included, given that this was the only condition that required reward-cost trade-offs to guide final decisions. Trials with erroneous responses during final choice (i.e., responses that did not match either of the 2 final image locations) were excluded (M = 0.5%, SD = 1.0%). Additionally, trials with responses that occurred too early, i.e., during the delay period were excluded (M = 4.1%, SD = 6.2%) in order to avoid capturing any motor-related activity during this time interval.
Connectivity Analysis
Functional connectivity between fronto-parietal ROIs was investigated in a psycho-physiological interaction (PPI) analysis (Friston et al. 1997) using the gPPI Toolbox for SPM (McLaren et al. 2012). Functional images were pre-processed in the same way as for the MVPA analysis, but additionally, spatial normalization and smoothing were performed. To investigate whether connectivity between dlPFC and PCUN/AG/MFC during the final decision period was different for CoM versus no-CoM trials, the dlPFC was defined as a seed region. First, a standard first-level analysis was performed to extract the time course of BOLD signal from dlPFC for trials with versus without CoM. The interaction between dlPFC x CoM was then included as a regressor in a second GLM, together with the main effect of dlPFC activity as well as the main effect of CoM and motion correction parameters as regressors-of-no-interest. A second-level analysis was then performed and parameter estimates were extracted for PCUN, AG, and MFC using MarsBaR. Both the contrasts CoM > no-CoM and no-CoM > CoM were analyzed.
Results
Behavioral
All participants made roughly balanced face/house choices during the initial decision period (face: M = 54.0%, SD = 4.2%, Min = 41.7%, Max = 61.4%). Furthermore, during the final decision period, participants had highly balanced numbers of CoM and no-CoM trials in the diagonal jump condition (M = 50.3%, SD = 4.6%, Min = 38.8%, Max = 62.5%). As expected, CoM was very frequent in the far-target condition (M = 95.9%, SD = 7.0%) where CoM allowed participants to save time. By contrast, in the close-target condition CoM was relatively rare (M = 4.7%, SD = 7.2%) given that differences in costs were negligible. Furthermore, in parallel trials, only 2 participants erroneously switched to the alternative option in 1–2 trials, respectively, while none of the other participants showed any CoM in parallel trials. This suggests that overall, participants paid attention to the identity of the chosen images and remembered them correctly throughout the course of a trial.
Decoding Decisions
fMRI analyses focused on investigating the specific information about the decision process that could be decoded from patterns of brain activity in decision-related regions using MVPA (Fig. 2A). Initial decisions could be decoded significantly above chance from all ROIs (Fig. 2B, top row; IOG: M = 72.6%, SD = 17.2%, t(24) = 6.61, P < 0.001, d = 1.32; FG: M = 73.5%, SD = 20.1%, t(24) = 5.85, P < 0.001, d = 1.17; PCUN: M = 63.7%, SD = 18.9%, t(24) = 3.64, P < 0.001, d = 0.73; AG: M = 62.5%, SD = 17.1%, t(24) = 3.66, P < 0.001, d = 0.73; MFC: M = 65.0%, SD = 14.6%, t(24) = 5.15, P < 0.001, d = 1.03; dlPFC: M = 59.2%, SD = 15.0%, t(24) = 3.07, P = 0.003, d = 0.61).
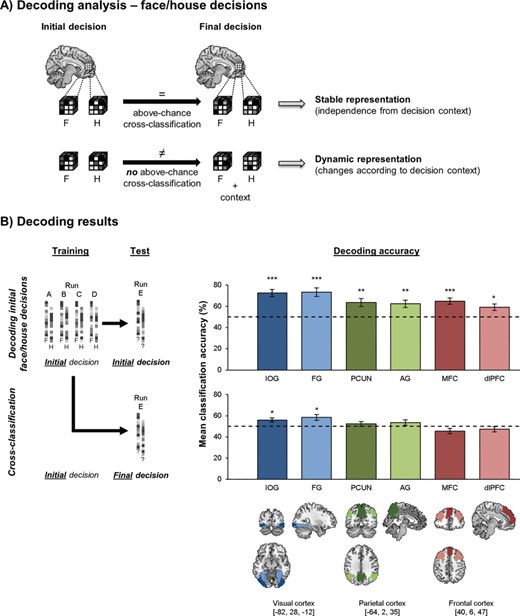
Decoding face/house decisions. (A) Decoding approach for initial and final face/house (F/H) decisions. Cross-classification across both decision stages allows for investigation of the stability of choice representations. Areas that represent choice options regardless of changes in decision context should support cross-classification, whereas areas that integrate novel information during the course of decision making would not support cross-classification. (B) Decoding results: mean decoding accuracies (±1 SEM) for initial face/house choices (top row) and cross-classification from initial to final face/house choices (bottom row). Chance level (dashed line) is 50%. *P < 0.05/6, **P < 0.01/6, ***P < 0.001/6. Permutation tests were used for statistical testing. MNI coordinates refer to the coordinates of the slices presented in this figure, providing a reference for the approximate location of each ROI.
We then tested for each ROI to what extent neural activity patterns for the initial decisions remained stable during the transition to the final decision period despite the fact that new reward/cost information was now available and participants were already executing the action corresponding to their initial choice. Areas that represent choice features independently of changes in the external decision context would use similar representations for face/house decisions across both decision stages (Fig. 2A). By contrast, areas that adjust choice representations to the current decision context would dynamically change neural patterns, e.g., in order to integrate decision information from both phases, rather than simply reflecting face/house decisions per se. Cross-classification decoding analyses (Bode et al. 2013; Kaplan et al. 2015) were used for which a decoder was trained on neural patterns of initial face/house choices, but was tested on final face/house choices (Fig. 2B, bottom row). Hence, training samples for this analysis were identical to the ones used for decoding of initial decisions reported above. Importantly, for final decisions, face/house choices were included regardless of whether a CoM occurred to shed light on how choices were generally represented when contextual information changed, independent of whether or not this required a CoM. Note that cross-classification accuracies were expected to be lower than for within-phase decoding, given that training and test patterns were recorded at different time periods. More importantly, if decoding accuracies for cross-classification are above chance, neural patterns for each choice option are, at least to some extent, similar across the 2 decision stages—indicating stability of neural choice representations. By contrast, substantial differences in neural patterns—e.g., because different information was represented during initial versus final decisions—would cause cross-classification to be at chance. Thus, cross-classification accuracy served as a binary indicator that allowed us to qualitatively compare neural representations of initial and final face/house choices, and hence, assess their stability across different decision contexts. This approach is in line with previous studies using above-chance cross-classification as an indicator of generalizability of neural representations across different contexts (for a review and discussion, see Kaplan et al. 2015; Hebart and Baker 2018; Wisniewski 2018).
In IOG and FG, cross-classification was above chance, indicating stable representations of choice options across the different decision stages (Fig. 2B, bottom row; IOG: M = 56.0%, SD = 10.0%, t(24) = 2.95, P = 0.004, d = 0.59; FG: M = 58.5%, SD = 13.5%, t(24) = 3.26, P = 0.002, d = 0.65). By contrast, cross-classification was not significantly above chance in any of the fronto-parietal ROIs (PCUN: M = 52.3%, SD = 11.4%, t(24) = 1.13, P = 0.135, d = 0.23; AG: M = 53.6%, SD = 13.2%, t(24) = 1.21, P = 0.120, d = 0.24; MFC: M = 45.6%, SD = 12.3%, t(24) = −1.85, P = 0.038, d = −0.37; dlPFC: M = 47.3%, SD = 12.5%, t(24) = 1.14, P = 0.132, d = −0.23). Hence, in all fronto-parietal ROIs, neural choice representations changed from the initial to the final decision phase. This suggests that during final decisions, neural patterns in these regions did not encode pure face/house decisions any longer, but might potentially predict the external information that had to be integrated. In line with this, decoding face/house choices from the final decision stage alone was not significant in fronto-parietal regions (all P > 0.100; data not shown). However, note that this could reflect lack of power for the face/house decoding analysis when focusing on final decisions alone. In this analysis, only trials with diagonal jumps (16 trials/run) could be included to train and test the decoder. By contrast, for initial decoding and cross-classification analyses, all 28 trials/run were included as training samples, rendering the classifier more reliable. Hence, it is possible that some information about face/house decisions was still present in fronto-parietal cortex during the final decision period, but was too weak/noisy to be detected by the decoder that only included final decisions. In line with this, we found that final decisions could not be decoded from the 2 visual ROIs either (both P > 0.100). This was true even though both visual areas showed successful cross-classification of decisions, suggesting that some face/house representation was indeed preserved across task phases in these areas. Hence, interpretation of final face/house decoding per se may have been limited by lower trial numbers. Thus, we focused on cross-classification analyses for face/house choices, given that training samples were identical to the initial decoding analyses, hence, ruling out that differences in the signal-to-noise ratio in training samples can account for our findings. Next, we investigated whether fronto-parietal areas indeed incorporated representation about external decision context during final choices.
Fronto-Parietal Areas Integrate New Information
One plausible reason for changes in fronto-parietal neural patterns is that new action- and decision-relevant information was available for making final decisions, whereas initial decisions were abstract and endogenous. More specifically, during final decisions, the external context provided information about 1) target locations, which was required to successfully transform the final decision into an appropriate motor action and 2) target distance, which guided re-evaluation of the initial choice based on cost-reward trade-offs. In order to investigate to what extent neural patterns in fronto-parietal areas incorporated these new pieces of external information, we performed separate decoding analyses for the location of the chosen image (left/right) and the distance between the target locations (close/far; Fig. 3A).
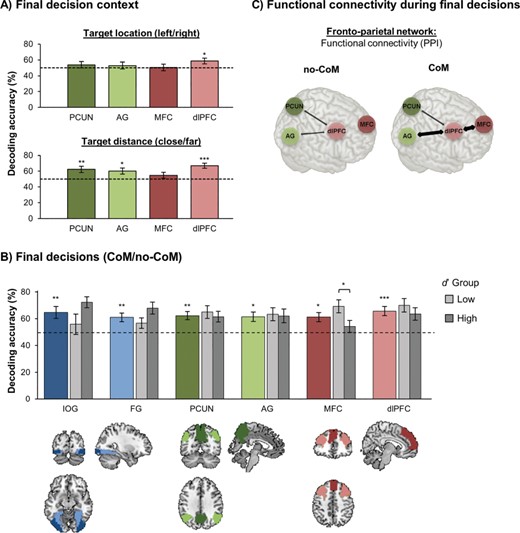
Decoding results and functional connectivity during final decision period. (A) Decoding decision context during final decisions in fronto-parietal ROIs. Mean decoding accuracies (±1 SEM) for location of the chosen target (top row) and target distance condition (bottom row). *P < 0.05/4, **P < 0.01/4, ***P < 0.001/4. (B) Mean decoding accuracies (±1 SEM) for CoM/no-CoM decisions. *P < 0.05/6, **P < 0.01/6, ***P < 0.001/6. (C) Functional connectivity during final decisions. Schematic illustrating differences in connectivity between fronto-parietal ROIs for CoM/no-CoM trials.
The only fronto-parietal ROI found to be predictive of location of the chosen image was dlPFC (t(24) = 2.45, P = 0.011, d = 0.49; all other ROIs: P > 0.170). This suggests that dlPFC had access to information that was required to successfully transform final decisions into motor actions. Target distance could be decoded from PCUN, AG, and dlPFC (PCUN: t(24) = 3.13, P = 0.002, d = 0.63; AG: t(24) = 2.72, P = 0.006, d = 0.54; dlPFC: t(24) = 5.68, P < 0.001, d = 1.14), but not from MFC (t(24) = 1.30, P = 0.104, d = 0.26). Note that target location and distance could also be decoded from both ROIs in visual cortex (all P < 0.001). However, IOG and FG presumably represented these pieces of information in terms of their low-level visual features. Given that cross-classification of face/house choices was above chance in visual areas, representations of target location and distance did not seem to substantially change, or interfere with, representations about the choice options themselves. By contrast, in fronto-parietal areas, integration of information about target distance and location toward a decision may explain changes in neural patterns during the final decision phase.
As the distance between the images was constant within a given trial, information about target distance condition was already available as soon as the images first appeared in their respective locations (target onset; frame 2 Fig. 1), immediately after the initial decision period. AG did not encode target distance at this early point (M = 50.8%, SD = 18.9%, t(24) = 0.21, P = 0.417, d = 0.04), but instead, only integrated target distance information when it became relevant for the decision-making process—i.e., during the final decision period when participants decided to stay/switch. By contrast, PCUN and dlPFC already encoded distance as soon as the targets appeared (PCUN: M = 58.9%, SD = 17.6%, t(24) = 2.53, P = 0.009, d = 0.51, dlPFC: M = 58.7%, SD = 17.7%, t(24) = 2.46, P = 0.011, d = 0.49). This suggests some functional separation within the fronto-parietal network in tracking information that is currently relevant for the decision process (AG) versus information that is currently available, but may only become relevant later on (PCUN and dlPFC)—possibly in anticipation of a potential future decision reversal.
Decoding CoM
The new decision context provided during final decisions was systematically tailored to trigger CoMs in trials in which the distance to the originally chosen object was substantially higher. Decisions for CoM (no-CoM/CoM; irrespective of face/house) could be decoded significantly above chance from all 6 ROIs (Fig. 3B; IOG: t(24) = 3.31, P = 0.001, d = 0.66; FG: t(24) = 3.35, P = 0.001, d = 0.67; PCUN: t(24) = 3.97, P < 0.001, d = 0.79; AG: t(24) = 3.22, P = 0.002, d = 0.64; MFC: t(24) = 3.22, P = 0.002, d = 0.64; dlPFC: t(24) = 4.61, P < 0.001, d = 0.92). In both visual areas, neural patterns associated with face/house choices could be cross-classified from initial to final decisions, suggesting an ongoing tracking of the visual stimulus properties—and hence, a dynamic reversal of patterns in CoM trials. Given that distance was the criterion for most CoM decisions, and target distance could indeed be decoded from PCUN, AG, and dlPFC during the same time period, it is highly likely that these areas integrated initial decision information with target distance information to prepare CoM decisions. Interestingly, MFC was the only ROI that did not allow for decoding of target distance, even though decoding of CoM decisions was significantly above chance in this area. This finding suggests that processing of target distance and CoM were not perfectly correlated at the behavioral and neural level, but should be regarded as separate processes. Hence, we next investigated whether the neural representations of CoM in MFC could be explained by some CoM decisions not being based on target distance, but instead, being prepared endogenously.
Endogenous CoM Decisions
Although overall, CoM decisions were largely based on external information about distance, some participants occasionally decided to change their mind (or refrain from doing so) independently of target distance. For example, during debriefing, some participants reported that they sometimes “particularly liked one of the two images”, thought that “the little man should go into the house”, or just “randomly wanted to mix it up from time to time”. These decisions were not related to the external task cues, but instead, were generated internally. Hence, decoding CoM from MFC, which did not explicitly represent target distance, might have been driven by those participants who based their CoM decisions less strongly on target distance and instead more strongly on endogenous factors. To quantify the extent to which each participant’s CoM decisions were based on target distance, a sensitivity parameter d’ was calculated according to signal detection theory (Green and Swets 1966):
d’ = z (hit rate) − z (false alarm rate),
where hits correspond to CoM in the far-target condition, whereas false alarms correspond to CoM in the close-target condition. For participants with hit/false alarm rates of 100%/0%, values were adjusted by −/+ 1% to allow for computation of d’ (Stanislaw and Todorov 1999). Within the context of the current task, d’ does not strictly reflect perceptual sensitivity, but rather should be interpreted as an indicator of a participant’s decision-making strategy—i.e., how strongly decisions were informed by external information about target distance. Higher d’ reflects higher sensitivity of CoM decisions to target distance, while lower d’ reflects higher influence of endogenous factors on CoM.
A median split analysis was conducted to check whether interindividual differences in d’ were related to differences in CoM decoding accuracy. We chose a median split rather than a regression approach, because d’ values were strongly skewed. In particular, 9 participants with high d’ had exclusively based their CoM decisions on target distance information. This limited between-subject variance in d’ values. Hence, the sample was split into a high-d’ group (d’: Mdn = 4.65, IQR = [4.38, 4.65]) and a low-d’ group (d’: Mdn = 3.08, IQR = [2.79, 3.61]). Note that d’ was high in both groups, reflecting that overall, image distance was the most important driving factor for CoM (as intended). However, importantly, the difference in d’ was significant between groups (Mann–Whitney U test for non-normal data: Z(22) = 4.27, P < 0.001), indicating that the division into high and low-d’ groups was meaningful. MFC decoding performance for CoM decisions was only above chance in the low-d’ group (Fig. 3B; t(11) = 4.24, P = 0.001, d = 1.23), but not in the high-d’ group (t(11) = 0.86, P = 0.408, d = 0.25), and the group difference in decoding accuracies was significant (t(22) = 2.27, P = 0.034, d = 0.93). Furthermore, when we excluded trials in which CoM decisions did not follow target distance, CoM could not be decoded anymore from MFC in the low-d’ group (M = 57.5%, SD = 22.2%, t(11) = 1.17, P = 0.267, d = 0.33). Note that this is most likely not due to lower trial numbers in this analysis as all other fronto-parietal ROIs still showed above-chance decoding of CoM decisions in the low d’ group when CoM/no-CoM trials that did not follow target distance were excluded (PCUN: M = 62.3%, SD = 16.2%, t(11) = 3.20, P = 0.008, d = 0.75; dlPFC: M = 65.2%, SD = 17.3%, t(11) = 3.84, P = 0.003, d = 0.89; marginal trend in AG: M = 61.3%, SD = 16.5%, t(11) = 2.00, P = 0.071, d = 0.68). Hence, together these results suggest that decoding of CoM from MFC was indeed driven by trials in which endogenous decision criteria, rather than target distance, were the crucial drivers.
In order to explore whether any of the other ROIs showed differences between the high- and low-d’ group, an analysis of variance (ANOVA) with the factors ROI (IOG/FG/PCUN/AG/dlPFC) and d’ group (low/high) was conducted. No significant main effect of ROI or group was found (both F < 1), but the interaction of ROI x group was significant (F(4,88) = 2.87, P = 0.037, η2 = 0.115). This was driven by the visual ROIs, which showed a non-significant (after Bonferroni correction) trend in the opposite direction of the effect observed in MFC (stronger effects for a stronger influence of target distance; IOG: t(22), = −1.89, P = 0.072, d = 0.77, FG: t(22) = −1.88, P = 0.074, d = 0.77). In all other ROIs, decoding accuracies for CoM decisions were comparable between the two groups (all P > 0.350). Importantly, both d’ groups had similar trial numbers for CoM/no-CoM decisions (% CoM in low-d’ group: M = 50.3%, SD = 6.7%; high-d’ group: M = 50.1%, SD = 0.6%; t(24) = 0.92, P = 0.916, d = 0.04), and the groups did also not differ in the precise target-distance values (t(22) = 0.405, P = 0.689, d = 0.17), meaning that these factors cannot explain the group differences in decoding accuracies.
Functional Connectivity during CoM Decisions
After establishing that the fronto-parietal ROIs differed in what decision-related information they represented—and presumably contributed to final decisions—we analyzed the functional connectivity between them. This might provide additional insights into how these areas communicated to exchange information to update the unfolding decision process. We focused on dlPFC, which constituted a critical cortical node because it encoded both target location and distance during the final decision period, and hence, represented all information relevant to implement a CoM as actions unfolded. A PPI analysis (Friston et al. 1997) was conducted with dlPFC as a seed region. The aim of this analysis was to measure whether functional connectivity between dlPFC and PCUN/AG/MFC differed for CoM versus no-CoM trials during the final decision period.
First, we established whether fronto-parietal regions showed any functional connectivity during final decisions by analyzing the main effect of dlPFC BOLD signal on PCUN, AG, and MFC activity. A significant effect was found in PCUN (b = 0.61, t(24) = 4.51, P < 0.001) and AG (b = 0.65, t(24) = 5.36, P < 0.001), and a trend was observed in MFC (b = 0.24, t(24) = 1.44, P = 0.082). This indicates that time courses of activity in these areas were correlated, suggesting that these areas were functionally connected. More importantly, the PPI effect was analyzed to check whether the strength of connectivity varied as a function of CoM (Fig. 3C). A significant effect (corrected α = 0.05/3) was found for dlPFC-MFC connectivity, revealing increased connectivity for CoM compared to no-CoM trials (b = 0.50, t(24) = 2.80, P = 0.005). In fact, dlPFC and MFC only showed significant functional connectivity in CoM trials (b = 0.49, t(24) = 2.64, P = 0.007), but not in no-CoM trials (b = −0.01, t(24) = −0.08, P = 0.531). A similar trend for increased connectivity during CoM was found for dlPFC-AG connectivity (b = 0.33, t(24) = 2.15, P = 0.021), although this effect was only marginally significant after correction for multiple comparisons. No effect of CoM on dlPFC-PCUN connectivity was observed (b = 0.17, t(24) = 0.89, P = 0.292).
Given that CoM decoding from MFC depended on how strongly decisions were based on endogenous factors, we also checked whether modulation of dlPFC-MFC connectivity was driven by participants with low d’. As expected, increases in dlPFC-MFC connectivity in CoM compared to no-CoM trials were only observed in the low-d’ group (b = 0.74, t(11) = 2.73, P = 0.010), but not in the high-d’ group (b = 0.28, t(11) = 1.07, P = 0.155). Hence, CoM may be implemented through functional connectivity of dlPFC with both AG and MFC, allowing for integration of externally cued information encoded in lateral fronto-parietal areas with endogenous information from MFC.
Discussion
The current study investigated the neural dynamics that enable agents to integrate new information about choice options as endogenous decisions evolve. First, participants were required to generate an arbitrary endogenous decision for a visually presented face or house stimulus without any choice outcome being associated with rewards at this stage. We derived ROIs from previous studies and confirmed that initial voluntary decisions were encoded in MFC, PCUN, dlPFC, and AG (Brass and Haggard 2008; Soon et al. 2008; Bode et al. 2011; Krieghoff et al. 2011; Bode et al. 2013; Soon et al. 2013; Zapparoli et al. 2018). Additionally, decisions could be decoded from visual cortex, presumably due to visual fixation of the chosen image, and attention to visual features of the chosen image (Krajbich et al. 2010; Rens et al. 2018; Voigt et al. 2019). More importantly, we then analyzed whether and how neural patterns associated with initial endogenous decisions changed during the integration of exogenous factors as on some trials the locations of the choice options changed, and participants had to re-evaluate their original intention based on new information about costs and rewards associated with pursuing or changing the decision.
Our results revealed that neural representations of choice options in visual cortex were similar during the initial and final decision period, as indicated by above-chance cross-classification between decision stages. Hence, low-level perceptual features of choice options were represented in a stable manner that was, at least to some extent, independent from changes in location and changes in decision-relevant information that became available. Consequently, although sensory areas provide continuous updates of dynamic percepts (Tong et al. 1998; Polonsky et al. 2000), the underlying neural code appears to be relatively static. By contrast, in fronto-parietal cortex, decisions could not be cross-classified between task stages. This cannot simply be explained by initial decoding accuracies being slightly lower in fronto-parietal areas compared to visual areas. First, decoding accuracies cannot be compared across regions because of potentially different underlying coding schemes (Bhandari et al. 2018). Second, even relatively low initial decoding accuracies would allow for significant cross-classification if patterns were sufficiently stable across decision stages (for examples of successful cross-classification despite low initial decoding accuracies, see Gallivan et al. 2013; Coutanche and Thompson-Schill 2014; Christophel et al. 2015). Instead, our findings showed that neural codes in fronto-parietal regions changed dynamically between decision phases and represented new decision-relevant information, suggesting that integration of novel exogenous information occurred during endogenous decision-making.
While previous studies have provided evidence for dynamic coding of decisions in fronto-parietal cortex, these studies have largely focused on tasks where actions were fully instructed by external stimuli (Toth and Assad 2002; Woolgar et al. 2011; Stokes et al. 2013; Schuck et al. 2015), rather than involving endogenous choice. Furthermore, previous studies have focused on post-decision processes that continuously evaluate a single source of evidence—either purely perceptual (Fleming et al. 2018) or value-based information (Boorman et al. 2009)—whereas our study shows how dynamic coding in fronto-parietal cortex could contribute to integration of several internal and external sources of evidence. This may be particularly relevant for voluntary decisions, which need to integrate internally generated intentions with contextual information about alternative courses of action and their respective costs and rewards.
Across fronto-parietal areas, we observed differences in what specific information was encoded and when it became available. Both parietal ROIs, PCUN and AG, represented information about target distance, which in turn informed cost-reward trade-offs guiding CoM decisions. Interestingly, AG did not encode distance information immediately when it was available, but only during the final decision period when it became relevant for the decision-making process. Hence, changes in the external environment may have triggered a re-computation of current intentions in AG (Sirigu et al. 2004; Chambon et al. 2012), taking into account new information about target distance. By contrast, PCUN started to encode distance information as soon as it was available, regardless of whether it was immediately relevant for the current decision. There are 2 possible explanations for this finding. First, given its proximity to dorsomedial occipital lobe, PCUN may have represented purely visual information about distance (Pitzalis et al. 2006), which was available as soon as the choice options first appeared in their respective locations. Alternatively, in line with its role in prospective memory (Burgess et al. 2011), PCUN may have started to extract decision-relevant evidence early on in order to anticipate future re-evaluation of the current intention. This is likely given how frequent trials with diagonal image relocation were, allowing participants to predict to some extent whether and how actions may need to be changed. This explanation would further be in line with other studies showing early decision encoding in PCUN (e.g., Soon et al. 2008, 2013). Finally, both PCUN and AG specifically represented the distance between images, regardless of their actual locations, indicating that both areas encoded abstract features reflecting decision-relevant information about externally cued rewards and costs associated with each choice option. This suggests that PCUN and AG were not simply involved in representing low-level sensorimotor information to transform decisions into movements, but instead, provided information guiding the decision process itself.
The dlPFC was the only area that encoded both target distance and location, and hence, represented decision- as well as action-relevant information. Previous studies have shown that dlPFC represents alternative choice options (Rens et al. 2018) and is involved in rapid value updates of competing choice options (Voigt et al. 2019). Here we show that these representations can be used to guide switches to an alternative choice option when values change. The dlPFC may have directly integrated value-based updates with action representations in order to guide changes in action selection. In support of this, it has previously been proposed that information about decisions and their implementation into motor actions can be represented in a common neural space (Cisek 2007; Thura and Cisek 2014; Yoo and Hayden 2018). Such concurrent representations might allow dlPFC to compute action updates in a fast and efficient manner. Additionally, similarly to PCUN, dlPFC already encoded distance information at target onset, suggesting a role in integrating currently available information to prepare future decision reversals and their implementation into flexible changes in action (MacDonald et al. 2000; Koechlin and Summerfield 2007).
Interestingly, PCUN, AG, and dlPFC switched from encoding internal decisions to encoding externally cued information during final decisions. This suggests that neural substrates underlying endogenous and exogenous decision making may not follow clear neuroanatomical boundaries, nor clear dissociations into medial versus lateral regions. Instead, different modes of decision-making may be implemented through different functional codes (Yoo and Hayden 2018), where a given brain area can dynamically change neural representations to encode internal or external information, depending on the evidence that is currently available. This idea is in line with previous accounts proposing a unifying framework for externally versus internally directed cognition (Dixon et al. 2014). Previous studies have reported response costs when people need to switch between internal and external modes of action selection (Obhi and Haggard 2004). Based on our findings, an intriguing hypothesis to be tested in future research is that costs incurred by switches in decision modes may be caused by the need to change representations within a given neural structure, rather than switching between different neural structures.
Nevertheless, in line with the idea that a medial frontal pathway is of particular importance for endogenous action selection (Passingham 1987; Brass and Haggard 2008; Soon et al. 2008; Bode et al. 2013; Fried et al. 2017; Zapparoli et al. 2018), we found that MFC was the only area that represented endogenous components of both initial decisions and later decision reversals. More specifically, MFC encoded CoM in participants who more strongly considered endogenous information, but not in those who exclusively relied on external cues, i.e., target distance. This shows that the same choice can result from different sources of information, or different decision strategies, and the underlying processes in turn recruit partially distinct neural circuits. Although this finding was based on a post-hoc group split, it could not be accounted for by other between-subject differences, such as overall frequency of CoM. Furthermore, it is unlikely that engagement of MFC simply reflected detection of erroneous (no-)CoM decisions (Ridderinkhof et al. 2004) since in that case, information about target distance itself should still be present in MFC. Instead, our findings suggest that some participants occasionally “detached” the decision process from external cues and made endogenous CoM decisions. In that sense, the observed dissociation in the pattern of MFC activity provides a powerful test of the hypothesis that MFC plays a distinct and crucial role in endogenous choice because it isolates the specific aspect of the design where endogenous decisions were exposed to exogenous cues that were in principle highly informative. Overcoming, or actively going against, such exogenous cues may represent a particularly strong aspect of volitional control (Ach 1935). While it is not clear why and how exactly some participants occasionally used these internal strategies for decision reversals, our study is the first to capture CoMs that are not exclusively guided by externally cued perceptual or value-based information. In this case, just as initial decisions, later decision reversals can be generated endogenously, which appears to rely on continuous involvement of MFC.
Finally, we tested whether endogenous and exogenous components of decisions may be integrated via changes in functional connectivity between regions that encode different sources of information (O’Reilly et al. 2012). We found that when participants changed their mind, dlPFC showed increased connectivity with both AG and MFC, compared to trials without decision reversals. dlPFC-MFC connectivity was only increased in participants who also showed endogenous CoM decisions, suggesting that connectivity between the medial action pathway and dlPFC is required to incorporate internally guided decision updates. Although PPI analyses do not reveal causality or directionality of connectivity (O’Reilly et al. 2012), it seems plausible that AG and MFC provided dlPFC with information about external and internal decision updates, respectively, enabling dlPFC to integrate both sources of information in order to guide changes in the ongoing decision-making process. Hence, dlPFC may serve as a critical node that allows for convergence of external and internal decision updates and their implementation into flexible action (Ridderinkhof et al. 2004).
This flexibility enables agents to adjust their behavior to the current context. Impairments in the processes underlying intention updates may result in escalation of commitment (Staw 1981) or rigidity of behavior, such as in obsessive compulsive disorder (Gruner and Pittenger 2017). However, in some situations, intentions need to remain stable despite external changes. In fact, goal attainment often requires continuous pursuit of an initial intention in the face of challenges or costs (Gollwitzer and Oettingen 2012). Hence, the balance between flexibility and stability of intentions is crucial for adaptive behavior (Goschke 2014; Wisniewski 2018). In our study, participants maintained their intentions when costs of intention pursuit were only slightly larger than for CoM. Such optimal behavior may be achieved through the interplay of several fronto-parietal areas that weigh different sources of evidence to generate a “distributed consensus” (Cisek 2012; Yoo and Hayden 2018) about whether or not the original intention should be changed.
An interesting question that remains to be addressed by future research is whether new information can completely override initially encoded intentions or whether the fronto-parietal network gradually incorporates external changes while maintaining initial decisions to some degree. In the current study, we were not able to decode final face-house decisions from fronto-parietal areas per se. This may have been caused by a lack of statistical power due to fewer training samples when focusing on final decisions alone (Pereira et al. 2009). Hence, it is possible that some residual face-house representation was still encoded in fronto-parietal areas, but was too weak to be picked up by the decoder that was only trained on final decisions. In line with this, our behavioral results strongly suggested that all participants made appropriate face-house decisions during the final decision phase since otherwise, we would have observed erroneous CoM/no-CoM decisions in parallel trials (control condition). As a result, we can rule out that the lack of decoding for final face-house decisions was caused by participants ignoring face-house information during final decisions. Note that we did not identify any other regions outside of the ROIs that encoded final face-house decisions (see whole-brain searchlight analyses in Supplementary Material S2). Hence, it seems likely that the same fronto-parietal regions encoding the initial decision continued to maintain face-house representation to some extent. However, given that new decision-relevant information about target distance and its associated costs and rewards were encoded during final decisions, these face-house representations may have been weaker than during initial decisions, which were generated in the absence of additional information, and hence, were purely endogenous. The ability of fronto-parietal areas to flexibly adjust neural representations of endogenous and exogenous choice features may be a crucial aspect of voluntary actions, which are guided by a variety of endogenous factors and externally cued value-based and perceptual information (Brass and Haggard, 2008; Rushworth, 2008), and hence, rely on appropriate weighting of information that is currently most relevant. In line with this, we could decode externally cued information about target location and distance during final decisions despite lower trial numbers, suggesting that these pieces of information were now weighted more strongly than face-house representations per se.
In conclusion, voluntary actions require continuous evaluation, and integration, of dynamically changing external and internal information—creating the opportunity, and sometimes the need, to change one’s own intention after an action has already been initiated. Our study provides important insights into the fundamental processes underlying volition by establishing a direct link between an initial intention and the subsequent processes that shape actions as they evolve. Our results show that voluntary actions are the result of 1) local dynamics within fronto-parietal regions allowing for flexible updates of decision-relevant information and 2) the global dynamics across regions enabling integration of information from various sources. These insights into the neurocognitive mechanisms that determine the stability versus flexibility of voluntary intentions are essential for our understanding of the mechanisms underlying adaptive behavior in a complex and dynamic world.
Funding
Australian Research Council grant (ARC DP160103353) to S.B.; ERC Advanced Grant HUMVOL (323943 to P.H. and A.L.); and by a study visit award from the Experimental Psychology Society, UK, to A.L.
Notes
The authors thank Richard McIntyre for support with MRI data acquisition and Daniel Feuerriegel, Milan Andrejevic, and Will Turner for helpful discussions.
Author Contributions
All authors contributed to the study conception and design. A.L. performed data acquisition and analysis under the supervision of S.B. All authors contributed to the interpretation of the results. A.L. drafted the manuscript and S.B. and P.H. provided critical revisions. All authors approved the final version of the paper.
Declaration of Interests
The authors declare no competing interests.