
Contents
-
-
-
-
-
-
-
-
Introduction Introduction
-
Placebos as a viable means for sustained relief from chronic pain Placebos as a viable means for sustained relief from chronic pain
-
Is a placebo predictable? And what are the clinical implications? Is a placebo predictable? And what are the clinical implications?
-
Genetic predictors of placebo responses Genetic predictors of placebo responses
-
Brain and personality predictors of placebo responses Brain and personality predictors of placebo responses
-
Predicting placebo responses through the study of semantics and meaning Predicting placebo responses through the study of semantics and meaning
-
Concluding remarks Concluding remarks
-
References References
-
-
-
-
-
-
-
-
-
-
-
-
5.1 Placebo responses and effects: Processes, potential, and ethical considerations in clinical care
-
-
-
-
-
-
-
-
2.1 C2.1Predictability of placebo responses and their clinical implications
-
Published:October 2023
Cite
Abstract
The bulk of research on the mechanisms underlying placebo effects has been conducted for painful stimuli in laboratory settings. Such studies identify a long list of learning mechanisms that can explain observed effects, as well as provide evidence that placebo analgesia is associated with reproducible patterns of brain activity. These studies also show that placebo effects are unstable across contexts, and the magnitude of a participant’s response in one setting does not generalize following subtle manipulations of the procedure, suggesting that placebo effects are primarily driven by immediate, context-specific expectations and, thus, remain essentially unpredictable. Recent studies challenge this notion by finding that clinical placebo effects in chronic pain patients can be predicted by genetics, brain properties and language use. If replicated, latter results suggest that placebo effects can be understood and harnessed in clinical treatment of chronic pain conditions and beyond.
C2.1S1Introduction
C2.1P1An extensive body of literature establishes the mechanisms that may underlie placebo effects at several levels of analysis, including psychological (e.g., expectations and learning) and brain (e.g., frontal-brainstem pathways, opioids, and other neurochemicals). Multiple learning mechanisms have been demonstrated to underlie placebo effects, and an extended brain circuitry associated with the phenomenon. Excellent reviews cover these topics,1 and we will not address them here. The bulk of this research has been and continues to be performed in healthy subjects and in artificial, but well-controlled, laboratory settings. However, in the field of pain, placebo procedures remain most relevant in clinical trials. The great strength of randomized clinical trial (RCT) designs is that, if properly conducted, a significant active treatment vs. control effect (e.g., drug vs. placebo) generally allows one to infer a causal relationship between the successful treatment and the condition under study. From this viewpoint, it is not surprising that most clinical trials for treating acute and chronic pain continue to fail. Placebo treatment arms are almost universally used in such studies, and overall responses driven by placebos (“placebo responses”) are often several times larger than the incremental active treatment effect. Two opposing interpretations can be derived from such observations: (1) the study is not well controlled, and various nuisance variables drive placebo responses and thus obscure the real biological treatment effects, or (2) the active arm has no, or minimal, causal benefit on the condition being studied.
C2.1P2Over the last decade, pain trialists have championed the concept that if one could minimize placebo responses, then RCTs would yield more significant active treatment effects. This interpretation essentially blames placebo responses magnitude for negative clinical trials. In this same period, improvements in placebo arms have grown larger, while drug effects remain stable, resulting in a shrinking drug versus placebo difference.2 This increase parallels improvements in the quality of RCTs, the amount of time spent with patients, and the number of study visits. These observations give further impetus to the concept that placebo responses need to be controlled to increase the chances of positive RCTs. These issues are particularly relevant to chronic pain, where pharmacological treatments have not made any serious progress over the last 20 years or so, although preclinical studies continue to identify many potential druggable targets.
C2.1P3There is irrefutable evidence that chronic pain is a large unmet medical need urgently requiring novel nonaddictive therapies, as it diminishes quality of everyday life in about 20% of the world population, with a staggering annual healthcare cost of more than $600 billion just in the United States.3 Often, chronic pain persists for a lifetime and commonly leads to depression,4 insomnia, depressed immune function, changes in eating patterns,5,6 substance abuse,4 impaired cognitive function,7 and costs to families and caregivers.8
C2.1P4Chronic pain patients are commonly treated with opioids, and thus remain a primary contributor to the ongoing opioid epidemic—particularly in the United States9—which has only worsened in the Covid-19 pandemic era. Available treatments for chronic pain do not cure the condition, and the majority of patients remain dissatisfied. For example, although nonsteroidal anti-inflammatory drugs (NSAIDs) are widely prescribed and used by the public, a Cochrane review of 65 clinical trials (11,237 total patients) found they are only modestly better than placebos for chronic and acute back pain. Updated Cochrane reviews reach the same conclusion. 10,11 NSAIDs were statistically comparable to other widely used treatments, including physiotherapy, spinal manipulation, paracetamol, opioids, and muscle relaxants.12,13 Thus, there is a lack of effective treatments for chronic pain, and new nonopioidergic treatments remain urgently needed. The pharmaceutical industry has spent billions of dollars searching for novel drugs for chronic pain unsuccessfully.
C2.1P5In this chapter, we present the latest evidence that a substantial component of placebo effects can be predicted by biological and behavioral parameters: genetic biomarkers, brain anatomy and function, personality characteristics, and language usage. Moreover, we briefly review the growing evidence that in well-controlled placebo studies (under neutral conditions, hidden conditions, or in properly explained and openly administered conditions) the evidence points to the possibility of large and sustained pain relief from placebo treatments. The combination of predictability and persistent analgesia imply that a placebo itself may be viewed as a viable treatment for chronic pain. Its basic psychological mechanisms—positive but appropriately calibrated expectation, hope, interpersonal care and connection, and learning processes shaped by selective attention to positive outcomes—overlap with psychological and behavioral treatments that can be delivered in a nondeceptive fashion.14 Thus, we conclude the chapter by a call for a concerted effort to demonstrate the viability of treating chronic pain using optimized placebo protocols.
C2.1S2Placebos as a viable means for sustained relief from chronic pain
C2.1P6Overall placebo responses in clinical trials are large, but in part, are due to natural history (improvement that would have occurred without the placebo), regression to the mean, and other statistical artifacts related to selective attrition.15 Estimating causal effects of a placebo requires comparing placebo groups with natural history controls, which tends to be rare in clinical trials but has been used in some studies.16 The latter data show prevalence of placebo analgesia across multiple types of chronic pain. Placebo treatments can cause sustained effectiveness rivaling in magnitude to that of active drug treatments.17 For example, meta-analyses of expectancy effects18 and placebo effects in clinical samples19 have found effect sizes in the Cohen’s d = 0.5 range, and in more advantageous combinations of suggestion and psychosocial support, they can be quite large.20,21,22,23,24 Note also that a recent meta-analysis of placebo effect sizes relative to no treatment in chronic back pain (CBP)25 suggests smaller effects overall, but shows that most studies are limited in quality and duration.
C2.1P7Placebo treatments engage multiple brain circuits implicated in supraspinal sensitization, as well as in pain and nociception related circuitry,26 descending modulation of the spinal cord,27,28,29 and spinal cord activity.30 Thus, placebo manipulations seem able to control spinal cord nociceptive inputs, their supraspinal projections, and the brain circuitry’s ability to modulate the transformation of nociception to pain perception. Within the circuitry involved in placebo responses, the ventromedial prefrontal cortex (VMPFC) and dorsolateral prefrontal cortex (DLPFC) seem to play chief roles, including via their ability to modulate subcortical affective and motivational circuitry. The VMPFC projects to the hippocampus, nucleus accumbens, amygdala, hypothalamus, and brainstem (dorsal raphe, periaqueductal gray [PAG]) to provide contextual meaning-based control over affect and physiology.31,32,33 It is consistently involved in placebo effects, including responding to placebos believed to be more expensive (a suggestion effect),34 placebo-induced opioid release,35 and placebo effects in social rejection.36 Moreover, these circuits are further involved in depression,37 posttraumatic stress disorder (PTSD),38,39 drug craving and relapse,40 social anxiety disorder,41 chronic pain,42,43 and others. A reason for this broad involvement in psychopathology may be its role in conceptualizing the self in a situational context, which is related to multiple forms of thought, including spontaneous and self-referential thought, future-oriented, prospective thought,44,45 theory of mind,46 and cognitive maps of conceptual relationships.47
C2.1P8Recent theoretical advances provide a framework for why context can exert such powerful effects,36 and how learning-related signals affect prediction errors to shape learning,46,48,49 resulting in placebo effects that do not diminish over time.48 Predictive processing theories emphasize that the brain actively constructs a model of the underlying causes of sensory and interoceptive signals. Perceptions are viewed as inferences about the underlying state of the world and self, including pain. Predictive learning depends strongly on the underlying meaning of the cues as interpreted by the organism.50 This provides an explanation for why verbal suggestions can be powerful in humans, even eliciting robust hormonal responses in some cases,51 and provides a common pathway for conditioning, treatment history, suggestion, interpersonal (e.g., doctor-patient) interactions, and effects of physical context (e.g., the place in which one is treated; the look and feel of an injection) based on their meaning to the patient. This meaning is conceptual and constructed based on integration of multiple aspects of the external and internal (body state) context. The semantic category labels we apply to objects influence the nature of the emotional and brain responses they elicit.52 For example, labeling an experience as belonging to one category or another influences how we generalize across pain-predictive cues, shaping pain and autonomic activity.53,54,55 A key aspect of predictive processing is that it drives learning, providing an explanation for why placebo effects can persist and even grow over time. It is not simply the presence of a pain-predictive cue that drives learning, but awareness that the cue is linked to pain, and attribution of unique signal value to the cue.
C2.1S3Is a placebo predictable? And what are the clinical implications?
C2.1P9As reviewed above, placebo effects hinge on concrete brain circuitry and behavioral phenomena that can be experimentally studied and manipulated. Thus, a key question is whether we can take advantage of these biological and behavior properties to predict who is likely to respond to a placebo. Being able to predict placebo responders—who will respond strongly to a placebo and who will not—is highly desirable and has nontrivial implications for both clinical practice and clinical trial designs. First, knowing who is likely to benefit from a placebo minimizes ethical concerns related to prescribing placebos to the wrong patients. Recent encouraging findings on open-label placebos56—placebos given without deception, with the patients’ full knowledge that they are receiving a placebo—suggests that this could be a viable pathway if the right individuals and conditions can be identified. Second, it could be used in replacement of other treatments, even if temporary, to reduce the burden of harmful side effects of many pharmacological treatments.57 And third, the ability to predict placebo responses would provide a stronger, more controlled way to prescreen patients in the context of randomized controlled trials. We now turn to some promising new research that is paving the path forward in predicting placebo responders using genetic, brain, personality, and language features.
C2.1S4Genetic predictors of placebo responses
C2.1P10Determining whether genes influence the neurological pathways engaged during response to placebo treatment and, therefore, are predictive of placebo responders, is an area of active investigation. However, placebo genomics, like the related field of pharmacogenomics (i.e., the study of how genes influence response to active treatments), is stymied by the related challenges of low statistical power and lack of reproducibility. With tens of thousands of genes and even more possible polymorphisms, a large amount of data is necessary to begin to assess which genes might affect placebo responsiveness. Currently, for example, the LDhub repository in the field of genetics accepts a sample size of, at minimum, 5,000 for genome-wide association findings, and some studies now include over 1 million participants. One way to tackle the power problem is to use candidate gene analysis, a process of identifying individual genes that influence outcomes in the placebo arms of clinical trials. Still, candidate gene analyses often fail to replicate, because of the very small underlying effects of any single polymorphism and large numbers of tests.58
C2.1P11The search for placebo genes, or the placebome, is made more difficult because clinical trials are not often replicated, and the potential for gene-drug interactions can often mask important effects that influence placebo outcomes. This potential for placebo response pathways to be modified by drugs raises an interesting question that faces many clinicians, researchers, and drug manufacturers: do the effects of drugs and placebos interact or do they operate via independent mechanisms? The common “belief” is that drug and placebo effects may be additive in some cases.59 But there is growing evidence that drugs like naloxone can abrogate response to placebo,60 implying interdependence of placebo and opioid responses; gene-drug-placebo interactions have been demonstrated in other cases as well (Figure 2.1.1).62,63,64,65 Hence, it is important to examine what happens if there are differential effects in the drug and placebo arms, and, further, if these effects vary by genotype. This topic is explored in further detail in Chapter 2.2.
C2.1F1 Genetic predictors for placebo effects.
(A) IBS outcomes (IBS-SSS) by COMT genotype and varying placebo treatments.65 (B) Major cardiovascular disease outcomes by COMT genotype in the WHS.62 (C) Placebo and aspirin response in cardiovascular disease prevention by COMT genotype in the WHS. (D) Placebo and vitamin E response in cardiovascular disease prevention by COMT genotype in the WHS.62
C2.1P12It is difficult to disentangle the attributions of placebos in the context of genetic effects, but use of the pharmacogenomics approach to identify populations that might differentially respond to a placebo or drugs are warranted to add a deeper understanding to and more accurate interpretation of clinical trial results. Further, with the low cost and ease of genetic testing, identification of genes that might predict placebo responsiveness would aid in assembling appropriate sample sizes and ensuring appropriate treatment for individual patients in clinical care and, therefore, deserves serious further investigation.
C2.1S5Brain and personality predictors of placebo responses
C2.1P13Only few studies have so far examined the neurobiology of placebo responses in chronic pain patients within the settings of RCTs. In a series of two RCTs, Apkarian lab tested the proposition that biological and psychological factors predetermine placebo effects in CBP patients.23,24 A first RCT was designed to discover the brain regions and the personality traits involved in placebo effects, and a second one was designed to validate these discoveries. The study was implemented sequentially, meaning that CBP patients from the validation RCT were randomly assigned based on their expression of a placebo signature derived in the discovery RCT. Importantly, both RCTs included a no-treatment arm.
C2.1P14In the discovery RCT, the patients visited the lab on six occasions over 8 weeks and underwent identical scanning protocols on four of these visits. A battery of questionnaires was collected at each visit to capture patients’ psychological profile and current emotional and pain states. The 43 patients in the placebo treatment group were exposed to multiple treatment periods and stratified into responders and nonresponders based on their pain ratings. The stratified patients were also compared to 20 patients randomly assigned to a no-treatment group that underwent the same protocol (Figure 2.1.2A). Brain networks constructed from resting state functional connectivity show that placebo responders, at baseline, displayed stronger functional connectivity between both ventral (VLPFC) and dorsolateral prefrontal cortex (DLPFC) and the sensorimotor cortex, as well as weaker connectivity between the dorsolateral prefrontal cortex and the PAG (frontal-PAG in Figure 2.1.2B). Personality questionnaires measuring emotion regulation and interoceptive awareness further dissociated placebo responders and nonresponders.24 A logistic regression model was built based on data from the discovery RCT, combining the brain and questionnaire parameters,23 and this model—a placebo biosignature—was used to predict placebo responders a priori in the validation RCT.23
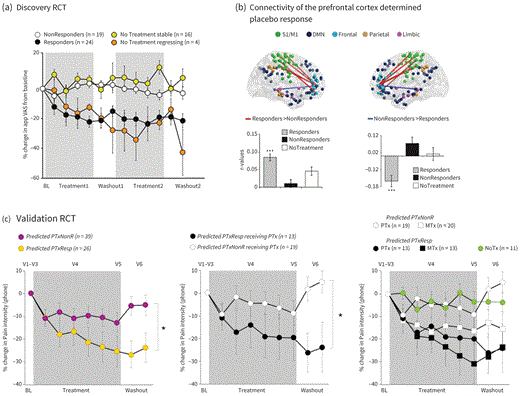
C2.1F2 Neurobiological determinants that predict placebo effects in randomized controlled trials. (A) In the discovery RCT, significant changes in pain intensity were most frequent in CBP receiving placebo pills (56% response rate) than in CBP patients receiving no treatment (20% response rate). (B) Edges between the DLPFC/VLPFC and the sensorimotor cortex (positive weight) or the PAG (negative weight) determined placebo responses. (C) Predicted placebo responses were determined by applying the derived biosignature in a new group of CBP patients randomized in a validation RCT. Changes in pain intensity after the stratification between predicted placebo responders and nonresponders irrespectively of treatment (left), only in the placebo group (middle), and across both treatment arms with the no-treatment arm altogether (right).
C2.1P15In the validation RCT, a new group of 77 patients with CBP were classified as placebo responders or nonresponders based on the aforementioned.23 The predicted placebo responders and nonresponders were identified prior to the randomization and were then allocated to placebo treatment, naproxen treatment, or no-treatment arms. The use of three treatment arms permitted researchers to disentangle the effect of pure placebo responses from the one embedded with the response to the drug (Figure 2.1.2C). The results demonstrate that placebo effects are is predictable at the group level, as within the placebo arm, patients a priori predicted as placebo responders show significant changes in pain—improvement of 21% from baseline pain—in contrast to no minimal pain relief in those predicted as nonresponders.23 Within the naproxen treatment arm, patients predicted as no-responders showed 15% pain relief from baseline, but pain relief in those predicted as placebo responders doubled to 32%, suggesting that pain relief could be partitioned into pure placebo and pure drug placebo analgesia of equivalent magnitudes (~10%–15%), and that these effects were approximately additive.23 Altogether, the approach demonstrates the potential placebo predictability in a validation cohort, that the biosignature was able to isolate pure active treatment effects, and that placebo and pure treatment showed additivity of pain relief.
C2.1S6Predicting placebo responses through the study of semantics and meaning
C2.1P16Genetic, brain, and personality contributions to placebo prediction have one aspect in common: they represent traits that are relatively static in time, which may in turn reflect perception and behavior. There is, however, an increased appreciation that placebo responses are highly context-dependent,66,67 and perhaps predicting behavior from static traits only taps into one of the potential sources of variation in behavior: the built-in machinery isolated from its context. The reality is, of course, more complicated. In the context of chronic pain, each patient carries their own pain story—How were they treated in the past and how successful were previous treatments? And how does the patient cope with their pain? While qualitatively it makes sense to simply interview patients to gather this information, quantifying it in an objective manner remains a challenge in psychological research, and to harness the predictive power of each person’s experiences with pain, we must turn to novel methodologies.
C2.1P17One of the distinguishing features of humans is our ability to communicate our thoughts and feelings through language. Language provides a wealth of information about the subjects’ self and is even popularly regarded as “a window to the soul.”68,69 In this context, natural language processing (NLP) emerged as a popular technique to extract language features out of text or speech, which allows researchers and clinicians alike to quantify latent topics being addressed by the patient during a normal conversation or clinical interview.70 With NLP, we can examine all the words a subject uses to respond to any given question and quantify the prevalence of words that map into a given semantic category or topic of interest (e.g., in chronic pain patients, are the words the patient is using related to themes of anxiety or depression? And what are common descriptors for their pain? Do patients use words more related to emotion or physical descriptors?). This allows us to capture and quantify important psychological constructs without addressing them explicitly, yet still in an objective and impartial way.
C2.1P18Berger et al.70 tested whether this technique can be used to predict placebo responses in CBP patients. The premise here is that as patients speak about their life, in particular, how they cope with pain and their previous experiences in the medical system, we could gather enough information that could predict, in an ecological manner, who is likely to respond to an inert placebo pill versus who will not. If successful, it could also give us clearer insight into the psychological dimensions that subtend placebo responsiveness. In parallel to the brain and behavior study reported above, we looked at language use in the two separate studies described above. The first study was designed to identify and generate a placebo prediction model. The second was designed to validate the prior model in an independent dataset and establish generalizability (see Figure 2.1.3. for an overview of the methods and language features).
C2.1F3 Language use predictive of placebo effects.
Methodology and natural language processing results are shown. In an interview participants are asked questions pertaining to their personality, mood, and pain. The answers are recorded, transcribed, and several language features are extracted, see. These parameters are then fed to a machine-learning algorithm that can predict who is a placebo responder and who is not, based on patterns of language use. In the first study (upper left), patients were divided into two arms: a set of patients with chronic back pain who received a placebo for two 2-week periods with a 1-week washout period in between, and a set of patients received no treatment (i.e., control condition). Patients rated their pain twice a day for the duration of the study and were determined to be placebo responders or not based on whether they had a significant pain reduction, compared to preintervention pain ratings. Study 2 (upper right) was conducted in an independent sample of patients with chronic back pain with similar characteristics to those in study 1, but the interview was performed before the start of the treatment and is, therefore, unbiased. Further, to test the specificity of prediction, the model was also tested in an arm of patients receiving Naproxen (NPX). Patients who do not respond to placebo (PTxNonR) used language more closely related to the terms force and stigma, whereas patients who respond to the placebo (PTxResp) used words more closely related to magnify (data from69). Below each topic is an example of patient speech related to each semantic topic, for each of the studies.
C2.1P19In Berger et al. study a set of language parameters collected at the end of the treatment phase did indeed identify placebo responders (i.e., patients who received significant analgesia from the placebo pill) with 79% accuracy.70 Patients who responded to the placebo described their pain experiences using words semantically proximal to afraid, fear, loss, awareness, identity, magnify, drives, and achievements. Patients who did not respond to the placebo used words more proximal to force (i.e., physical forces), stigma, and leisure. Through these parameters, a predictive model was generated including four key orthogonal parameters: semantic similarity to stigma, force, and magnify; and number of words related to achievement. Representative examples of patients’ speech as well as group differences for the semantic distance features are depicted in Figure 2.1.3. A second study was then conducted to validate and test the generalizability of the predictive language model. In this study, patients were instead interviewed before the treatment to assess the predictability of the model, and were randomized to either receive a placebo pill, or a naproxen pill. They were asked to describe themselves, to narrate a recent event, to describe their pain, and their past experiences in medical settings regarding their pain condition. Patients and researchers were blinded to which pill the patient received. By applying the predictive model from study 1 to study 2, the model predicted placebo responders with good accuracy (AUC = .71), but not drug responders (AUC = .52). This model could not predict spontaneous recovery in the no-treatment arms of study 1 and study 2 (AUC = .55), thus showing the model is predicting specific analgesic effects caused by a placebo and not attributable to, for example, regression to the mean effects. In fact, patients predicted to be placebo responders showed larger analgesic effects to the placebo compared to patients who were predicted to be nonresponders (30% vs. 3% change in pain, respectively).
C2.1P20In summary, how the subjects speak about themselves, their pain, and their medical expectations can provide important cues as to whether a patient will respond to a placebo or not. It is likely these language parameters reflect the subjects’ state at the time, considering a multiplicity of psychological factors, which further enhances its predictive ability—even beyond that shown by a large set of psychological questionnaires which instead measure more stable traits.71 This approach has strong ecological validity because it follows what would happen in a clinical context, where based on clinical interview, one must decide how to treat a patient. Although these studies remain mostly preliminary, advancing this technology can provide new opportunities for scientists and clinicians alike.
C2.1S7Concluding remarks
C2.1P21Historically, decades of research on predicting placebo responders has yielded a mixed picture, with findings of personality predictors in some studies failing to replicate or be consistent in others.72 In addition, small changes in context—including the name of the placebo “drug”—have been found to substantially alter who responds to a placebo, resulting in low correlations in placebo responses across contexts73 that interact with people’s predispositions in some cases.74 Similarly, placebo responses have been found to be uncorrelated across different outcomes. This variability fits within a pattern evidenced by personality research more broadly: Responses in a situation are driven by person x situation interactions.75 For example, rather than “anxiety” being a trait characteristic that predicts responses across situations, a person may be high-anxiety in one situation (e.g., at a party) but low-anxiety in another (e.g., parachuting) or vice versa. Placebo responses may be similar: A “responder” to a cream may not be a “responder” to soothing verbal suggestions and vice versa. To the extent that these effects are large and vary across individuals, stable genetic, brain, and personality traits that are not influenced by these variables must have small predictive effects.
C2.1P22The current studies present a counterpoint to this picture, suggesting that placebo effects may be predictable based on genetics or pretreatment brain structure or activity. They build on earlier studies finding that larger placebo analgesia and similar context-based pain modulation is predicted by trait optimism;76 low trait anxiety and fear of pain, for example,77 and high trait engagement and behavioral activation.78
C2.1P23What does this mean for the ability to predict the magnitude of an individual’s placebo responses, and how can these findings coexist? Larger-scale, preregistered studies are needed to test the effects found in earlier smaller-scale studies, particularly in genetics and neuroimaging, where large numbers of variables and tests can lead to selection biases. Alternatively, stable characteristics may indeed predict placebo responses. Such responses may apply only to narrow contexts. For example, the COMT gene may predict placebo responses only with a particular outcome measure in a particular population, and it will work if other factors—the interpersonal setting, prior associations, and perceived characteristics of the placebo—are tightly controlled and uniform across patients. On the other hand, they may predict placebo responses more broadly, perhaps as part of a general disposition or ability to engage in positive expectations and attend to potential treatment gains. Further research is needed to adjudicate between these possibilities. A productive avenue would be replication of observed placebo prediction effects in prior studies with a “near-context” replication, controlling and reproducing the original context as tightly as possible, as well as other “far-transfer” tests. In sum, the results of recent studies are promising, but we have a lot of work to do.
C2.1S8References
C2.1P241. Wager TD, Atlas LY.
C2.1P252. Tuttle AH, Tohyama S, Ramsay T, et al.
C2.1P263. Dahlhamer J, Lucas J, Zelaya, C, et al.
C2.1P274. Manchikanti L, Giordano J, Boswell MV, Fellows B, Manchukonda SR, Pampati V.
C2.1P285. Geha P, deAraujo I, Green B, Small DM.
C2.1P296. Lin Y, De Araujo I, Stanley G, Small D, Geha P.
C2.1P307. Apkarian VA, Sosa Y, Krauss BR, et al.
C2.1P318. Flor H, Turk DC, Berndt Scholz O.
C2.1P329. Jalal H, Buchanich JM, Roberts MS, Balmert LC, Zhang K, Burke DS.
C2.1P3310. Enthoven WT, Roelofs PD, Deyo RA, van Tulder MW, Koes BW. Non-steroidal anti-inflammatory drugs for chronic low back pain. Cochrane Back and Neck Group, ed. Cochrane Database of Systematic Reviews.
C2.1P3411. van der Gaag WH, Roelofs PD, Enthoven WT, van Tulder MW, Koes BW. In: Cochrane Back and Neck Group, eds. Non-steroidal anti-inflammatory drugs for acute low back pain.
C2.1P3512. Chou R, Deyo R, Friedly J, et al.
C2.1P3613. Ferreira ML, Ferreira PH, Latimer J, Herbert R, Maher CG.
C2.1P3714. Ashar YK, Chang LJ, Wager TD.
C2.1P3815. Kienle GS, Kiene H.
C2.1P3916. Hróbjartsson A, Gøtzsche PC. Placebo interventions for all clinical conditions. Cochrane Database of Systematic Reviews.
C2.1P4017. Chappell AS, Desaiah D, Liu-Seifert H, et al.
C2.1P4118. Peerdeman KJ, van Laarhoven AI, Peters ML, Evers AW.
C2.1P4219. Lennep J, Han PA, Trossèl F, Perez RSGM, et al.
C2.1P4320. Haake M.
C2.1P4421. Kaptchuk TJ, Kelley JM, Conboy LA, et al.
C2.1P4522. Tétreault P, Mansour A, Vachon-Presseau E, Schnitzer TJ, Apkarian AV, Baliki MN.
C2.1P4623. Vachon-Presseau E, Abdullah TB, Berger SE, Huang L, Griffith JW, Schnitzer TJ, Apkarian AV.
C2.1P4724. Vachon-Presseau E, Berger SE, Abdullah TB, et al.
C2.1P4825. Strijkers RHW, Schreijenberg M, Gerger H, Koes BW, Chiarotto A.
C2.1P4926. Zunhammer M, Bingel U, Wager TD;
C2.1P5027. Grahl A, Onat S, Büchel C.
C2.1P5128. Wager TD, Rilling JK, Smith EE, et al.
C2.1P5229. Zubieta JK.
C2.1P5330. Eippert F, Finsterbusch J, Bingel U, Büchel C.
C2.1P5431. Price JL, Drevets WC.
C2.1P5532. Roy M, Shohamy D, Wager TD.
C2.1P5633. Zhang L, Losin EAR, Ashar YK, Koban L, Wager TD.
C2.1P5734. Geuter S, Eippert F, Hindi Attar C, Büchel C.
C2.1P5835. Wager TD, Scott DJ, Zubieta JK. Placebo effects on human μ-opioid activity during pain.
C2.1P5936. Koban L, Kross E, Woo CW, Ruzic L, Wager TD.
C2.1P6037. Schmaal L, Yücel M, Ellis R, et al.
C2.1P6138. Etkin A, Wager TD.
C2.1P6239. Weaver SS, Kroska EB, Ross MC, et al.
C2.1P6340. Goldstein RZ, Volkow ND.
C2.1P6441. Hahn A, Stein P, Windischberger C, et al.
C2.1P6542. Nieminen LK, Pyysalo LM, Kankaanpää MJ.
C2.1P6643. Smallwood RF, Laird AR, Ramage AE, et al.
C2.1P6744. Bąbel P.
C2.1P6845. Satterthwaite TD, Green L, Myerson J, Parker J, Ramaratnam M, Buckner RL.
C2.1P6946. Benedetti F, Carlino E, Pollo A.
C2.1P7047. Constantinescu AO, O’Reilly JX, Behrens TEJ.
C2.1P7148. Jepma M, Koban L, van Doorn J, Jones M, Wager TD.
C2.1P7249. Jepma M, Wager TD.
C2.1P7350. Ploghaus A, Tracey I, Clare S, Gati JS, Rawlins JNP, Matthews PM.
C2.1P7451. Benedetti F.
C2.1P7552. Satpute AB, Lindquist KA.
C2.1P7653. Koban L, Jepma M, Geuter S, Wager TD.
C2.1P7754. Lebois LAM, Hertzog C, Slavich GM, Barrett LF, Barsalou LW.
C2.1P7855. Lieberman MD, Eisenberger NI, Crockett MJ, Tom SM, Pfeifer JH, Way BM.
C2.1P7956. Kaptchuk TJ, Friedlander E, Kelley JM, et al.
C2.1P8057. Schedlowski M, Enck P, Rief W, Bingel U.
C2.1P8158. Duncan LE, Pollastri AR, Smoller JW.
C2.1P8259. Atlas LY, Whittington RA, Lindquist MA, Wielgosz J, Sonty N, Wager TD.
C2.1P8360. Amanzio M, Benedetti F.
C2.1P8461. Schenk LA, Sprenger C, Geuter S, Büchel C.
C2.1P8562. Hall KT, Nelson CP, Davis RB, et al. Polymorphisms in catechol-O-methyltransferase modify treatment effects of aspirin on risk of cardiovascular disease.
C2.1P8663. Hall KT, Buring JE, Mukamal KJ, et al.
C2.1P8764. Hall KT, Kossowsky J, Oberlander TF, et al.
C2.1P8865. Hall KT, Lembo AJ, Kirsch I, et al.
C2.1P8966. Atlas LY.
C2.1P9067. Horing B, Weimer K, Muth ER, Enck P.
C2.1P9168. Pennebaker JW, Mehl MR, Niederhoffer KG.
C2.1P9269. Tausczik YR, Pennebaker JW.
C2.1P9370. Berger SE, Branco P, Vachon-Presseau E, Abdullah TB, Cecchi G, Apkarian AV.
C2.1P9471. Vachon-Presseau E, Berger SE, Abdullah TB, Griffith JW, Schnitzer TJ, Apkarian AV.
C2.1P9572. Kern A, Kramm C, Witt CM, Barth J.
C2.1P9673. Whalley B, Hyland ME, Kirsch I.
C2.1P9774. Hyland ME.
C2.1P9875. Lyby PS, Aslaksen PM, Flaten MA.
C2.1P9976. Geers AL, Wellman JA, Fowler SL, Rasinski HM, Helfer SG.
C2.1P10077. Koban L, Wager TD.
C2.1P10178. Schweinhardt P, Seminowicz DA, Jaeger E, Duncan GH, Bushnell MC.
Month: | Total Views: |
---|---|
October 2023 | 16 |
November 2023 | 41 |
December 2023 | 11 |
January 2024 | 9 |
February 2024 | 13 |
March 2024 | 9 |
April 2024 | 12 |
May 2024 | 8 |
June 2024 | 29 |
July 2024 | 12 |
August 2024 | 12 |
September 2024 | 19 |
October 2024 | 16 |
November 2024 | 17 |
December 2024 | 5 |
January 2025 | 4 |
February 2025 | 17 |
March 2025 | 14 |
April 2025 | 15 |
May 2025 | 4 |