-
PDF
- Split View
-
Views
-
Cite
Cite
Zhaoqian Liu, Jingtong Feng, Bin Yu, Qin Ma, Bingqiang Liu, The functional determinants in the organization of bacterial genomes, Briefings in Bioinformatics, Volume 22, Issue 3, May 2021, bbaa172, https://doi.org/10.1093/bib/bbaa172
- Share Icon Share
Abstract
Bacterial genomes are now recognized as interacting intimately with cellular processes. Uncovering organizational mechanisms of bacterial genomes has been a primary focus of researchers to reveal the potential cellular activities. The advances in both experimental techniques and computational models provide a tremendous opportunity for understanding these mechanisms, and various studies have been proposed to explore the organization rules of bacterial genomes associated with functions recently. This review focuses mainly on the principles that shape the organization of bacterial genomes, both locally and globally. We first illustrate local structures as operons/transcription units for facilitating co-transcription and horizontal transfer of genes. We then clarify the constraints that globally shape bacterial genomes, such as metabolism, transcription and replication. Finally, we highlight challenges and opportunities to advance bacterial genomic studies and provide application perspectives of genome organization, including pathway hole assignment and genome assembly and understanding disease mechanisms.
Introduction
As essential life forms on earth, bacteria have been around for at least 3.5 billion years [1]. To date, bacteria have been found within various environments, such as mountaintops [2], marine and terrestrial subsurface [3,4], the guts of animals [5] and even the frozen rocks and ice [5]. Different from eukaryotic genomes, most bacterial genomes consist of a circular DNA molecule of smaller size ranging from 100 to 15 000 kbp [6]. One of the key reasons for bacteria with the small genome size is that most bacterial species have undergone genomic degradation by the deletion of superfluous sequences during evolution [7] and show a relatively small number of junk DNA [7]. Furthermore, a third of the annotated genes of bacteria overlap with others [8], greatly expanding the functional potential from a small set of genetic information. The property of a small size genome enables DNA transactions (e.g. replication and repair) at an appropriate time [9,10], making bacteria highly adaptable to diverse environments [11].
The insight into the plasticity of bacterial genomes introduces a fundamental question: are the genomes organized in arbitrary manners or following some rules during evolution? The initial understanding of genome structures was proposed in the 1960s. Jacob and Monod observed that genes involving in uptake and metabolism of lactose are arranged together in the genome (i.e. lac operon) [12,13], which are controlled by a single promoter and co-transcribe as a single unit [12]. This finding indicates that the genome arrangement is not arbitrary but follows certain principles with important functional implications [14]. Based on this landmark work, more effort has been made for a full understanding of underlying organization principles. Yet the progress was stalled by the lack of experimental data. Until 1995, the first two complete bacterial genome sequences were published [15–17]. Since then, the increasing availability of genome data has greatly improved the understanding of genome arrangement.
Compelling evidence suggests that genome structures typically have direct or indirect associations with significant cellular processes. For example, the existence of operon structure on genomes not only coordinate expression and regulation of the constituent genes [12] but also improve the persistence of genes by horizontal transfer within and among taxa (the selfish operon model) [18]. Specifically, due to the heterogeneity of natural selection on both temporal and spatial scales, the genes encoding unimportant or rarely employed functions are more likely to evolutionary extinction with genetic drift [18]. To escape such loss, genes with neighborly functions tend to form clusters for the increasing probabilities of horizontal transfer to novel genomes [18]. This model explicated the existence of gene clusters from a new perspective, providing mechanistic insights into the local structures of bacterial genomes. Furthermore, another study observed that the locations of operons are under strong constrains of metabolic pathways, where operons participating in frequently activated pathways are clustered more tightly along genomes [19].
Beyond the local gene arrangements, there are discernible patterns of global genome organization [20,21]. For example, there is a unique origin of replication for most bacteria. During replication, DNA will open up at this origin and form two Y-shaped structures (a.k.a., replication forks) [22]. The replication forks move in opposite directions until they arrive at the terminus [22]. Based on the directions of DNA chain elongation and fork movement, the strands can be classified as leading strands (the one in which the directions of these two are the same) and lagging strands (the one in which they are opposite) [22]. The general observation suggested that the majority of protein-encoding genes are located on the leading strands, ranging from 50% to 90% across different bacteria [23,24]. Intuitively this makes sense to avoid head-on collisions in terms of DNA replication and transcription [25]. Additionally, the spatial organization, i.e. chromosome, has gained much interest in genomic studies. A large number of approaches have been available to measure chromosome-wide patterns, such as Hi-C [26], fluorescent repressor operator system (FROS) [21] and chromatin immunoprecipitation sequencing (ChIP-seq) [21], offering a strong opportunity to understand the overall layout of genome organization. Globally, bacterial chromosomes are several orders of magnitude longer than the cells. They fold compactly to fit the volume of cells and form complex structures [22]. For example, the chromosome of Escherichia coli (E. coli) is folded into a series of consecutive loops, i.e. supercoils [27]. Imaging data indicated that the distribution of supercoils is uneven and variable under different physiological conditions. There are fewer supercoils of E. coli during the stationary phase, while there are more of those during the exponential growth phase [28]. Additionally, the highly expressed genes (HEGs) are distributed at the folding-domain boundaries [21]. These findings demonstrate that the locations of genes and chromosome conformations have important impacts on gene expression [29] and copy number [30]. On this basis, an interesting computational model was proposed by Ma et al. [31] to infer supercoil structure, and the predicted results showed high consistency with the experimentally verified DNA binding sites of the nucleoid-associated proteins (NAPs) that assist the structure. Furthermore, the folding chromosome was organized into large size domains, such as chromosome interaction domains (CIDs) and macrodomains (MDs) [32,33]. Thirty-one CIDs and four MDs of the E. coli genomes have been reported. Nevertheless, the understanding of functions associated with such domains is not sufficient, and more effort is being made for a deep insight into the underlying mechanisms.
Taken together, given the structures of bacterial genomes, one cannot simply understand them as an evolutionary accident. Instead, they are closely related to functional activities. Understanding the principle of the genome organization can contribute to functional insights into diverse bacteria, providing favorable strategies for the full design of a new-to-nature genome layout in synthetic biology. In this review, our focus is on the bacterial organization and current understanding, both experimentally and com putationally, of the essential constraints on the genomes.
Local organization as operons, transcription units and alternative transcription units facilitates gene co-transcription
In 1961, Jacob and Monod showed that one or multiple continuous genes in a bacterial genome that encode proteins with related biological functions were transcribed in a single polycistronic unit, and these genes shared the same regulation signals by common promoters and terminators [13]. Then, the concept ‘operon’ was proposed as a local organizational feature of bacterial genomes [12] (Figure 1). Such a structure facilitates efficient co-transcription [34] and increases the fitness of genes during evolution [22]. Operons have since then been extensively studied as the basic transcriptional and functional units of bacterial organisms, laying a foundation for a holistic viewpoint of genome organization.
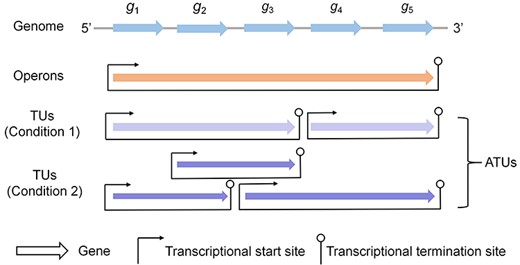
A diagram of operons, TUs and ATUs of the bacterial genome. The genome consists of five genes (blue arrows). These genes form an operon, i.e. (g1, g2, g3, g4, g5) (orange arrow). Actually, genes are dynamically co-transcribed with different gene sets, and each gene set is called a TU (all purple arrows under both two conditions). In some cases, such as under condition 1, TUs are nonoverlapping (light purple arrows). However, TUs are overlapped with each other under certain conditions, such as condition 2 (dark purple arrows). Given the different transcription structures under multiple conditions, the TUs under condition 1 and condition 2 are specifically called ATUs.
Initially, operons were assumed to be conserved across different genomes of a species and not overlapped with each other along the genome [35–37], which served as the basis for the identification of operons. A previous study used the conservation of gene pairs across genomes to identify operons, and its application in E. coli demonstrated the effectiveness of the proposed model [36]. Further, Dam et al. [38] integrated five available features of genomic sequences (the intergenic distance, the conserved gene neighborhood, the distances between adjacent genes’ phylogenetic profiles, the ratio between the lengths of two adjacent genes and the frequencies of specific DNA motifs in the intergenic regions) into a new model, which substantially improved the accuracy of operon prediction. These methods present exciting opportunities for understanding the local structures of various genomes. Subsequently, a large amount of computational predicted and experimentally validated operons have become available in public databases, such as RegulonDB [39], DBTBS [40], OperonDB [41], MicrobesOnline [42], ODB [43], ProOpDB [44] and DOOR [45]. These databases are different from each other in terms of reliability and emphases. Specifically, RegulonDB contains highly reliable operons of E. coli from both experiments and computationally prediction, while DBTBS contains experimentally characterized operons of Bacillus subtilis (B. subtilis) [39,40]. OperonDB and MicrobesOnline have computationally predicted operons for 550 genomes and 620 genomes, respectively [41,42]. Additionally, ODB provides both experimental and predicted operons for 203 genomes [43]. Of particular note, the predicted operons used in the above several databases are with relatively low accuracies (∼80%). Several databases were constructed thereafter, including ProOpDB and DOOR [44,45], and provided high-quality predicted operons (with accuracy more than 90%) besides those from experiments. Notably, the predicted operons of more than 2000 genomes are also available at DMINDA 2.0 in support of cis-regulatory motif discovery for prokaryote [46]. The information of operons serves as a basis of higher-level genome organization as well as underlying cellular functions [19], such as metabolic pathways and regulation systems [47].
Recently, researchers found that genes within the predicted single operon are not always co-transcribed but dynamically transcribed with different gene sets under different triggering conditions [48,49]. To cope with this discrepancy, the concept ‘transcription unit (TU)’ was specifically used to refer to the conditionally dependent co-transcribed gene sets (Figure 1). At least three TUs, including (pdhR, aceE, aceF, lpd), (aceE, aceF) and (ldp), of the pdhR-aceEF-lpd operon in E. coli have been found in different growth environments [48]. Additionally, a critical study suggested that TUs may overlap with each other by sharing the common genes, forming TU clusters [50]. It has been demonstrated that the TUs in a cluster are controlled by two different bacterial mechanisms for terminating transcription [51]: TUs that end inside the TU cluster tend to use Rho-dependent terminations, while the terminal TUs (i.e. those end at the last gene of TU cluster) more likely link with Rho-independent terminations [50].
Currently, TUs have been shown as a reliable structure of the bacterial transcriptional profiles that associate with genome organization [49]. Cho et al. [52] identified 4661 TUs of E. coli based on the organizational elements of TUs, such as transcription start and termination sites. A recent study by Yan et al. [49] applied SMRT-Cappable-seq to reidentify the TUs of E. coli under multiple growth conditions, which avoids information missing on the large transcriptional contexts and provides a more accurate visualization of genomic transcriptional profiles. Yet little is known about complete TU maps of various bacteria due to the time-consuming, laborious and costly experiment technologies. Fortunately, a variety of computational methods/tools based on available transcriptional data have been developed to advance the deep understanding of TUs, such as DOOR2 [53], BAC-BROWSER [54], SeqTU [55,56] and rSeqTU [57]. Among them, the first three are devoted to capturing the features of transcription intervals based on RNA-seq reads count signals [56–58] for TU identification of bacteria. rSeqTU applies random forest-based feature selection for TU inference [57], and it has improved into a comprehensive pipeline with high performance, from data quality control to TU visualization [57]. Overall, both experimental and computational techniques enhance the understanding of genome arrangement at the transcriptional level. Notably, due to the dynamic nature of gene co-transcription, TUs are of high diversity under different conditions. The different TU structures of a genome are specifically called alternative TUs (ATUs) (Figure 1) [57].
Metabolic pathways constrain operon locations on genomes
While local structures of genome arrangement have been well studied, researchers began to explore the rules that control the global arrangement of operons, not simply to push the limits of their specialties but rather to provide insights into cellular functions (e.g. metabolism and regulatory) at the genome scale [22,59]. By analogy with the operon organization within a regulon [60,61], it was originally speculated that operons working in the same metabolic pathway would cluster together along the genome. However, this speculation is generally not correct. A seminal study, focusing on both experimental [40,62] and computationally predicted [45] operons of E. coli K12 and B. subtilis str. 168, showed that more than 40% operons are involved in multiple pathways [19] (the definition of the pathway is based on three popular pathway databases, the SEED database [63], the Kyoto Encyclopedia of Genes and Genomes database [64] and the BioCyc database [65]). The component-sharing property among pathways makes each pathway, especially those encoded by multiple operons, tend to compose of a few clusters rather than one [66]. Inspired by this observation, researchers have put more effort into the studies about the potential mechanisms of global genomic locations.
The great advances of available genomic and transcriptomic data in the last decades have greatly improved the development of computational models. The first influential model was developed by Yin et al. [19], and they found that the position of an operon is determined by the relevant pathways in which it is involved. Particularly, the operons involved in pathways of higher activation frequencies (where the activation frequency is assessed based on the available microarray data) tend to form tighter clusters in a genome [19]. This finding motivates the development of the mathematical model, C value, to quantify the relationships between metabolic pathways and relative locations of operons in a bacterial genome [19]. In the proposed model, the C value of a genome is assessed by the compactness of operons in all pathways (|$C=\sum_{i=1}^N\sum_{j=1}^{M_i}{d}_{ij}$| where |$N$| indicates the number of pathways, |${M}_i$| indicates the number of operons of the ith pathway and |${d}_{ij}$| indicates the distance between the |${j}^{th}$| operon and the center operon in the ith pathway). We recalculated C values of the actual E. coli K12 genome and of a large number of reshuffled genomes (permuting the locations of operons in the genome in silico) based on the available genome data of E. coli K12. The result suggests that the actual genome typically has a smaller C value than most reshuffled genomes (Figure 2A), demonstrating that a bacterium keeps operons in individual pathways as compact as possible. Specifically, the operons that participate in multiple pathways will tend to minimize the overall compactness of their participating pathways, while operons involved in single pathway tend to minimize the compactness of the individual pathway.
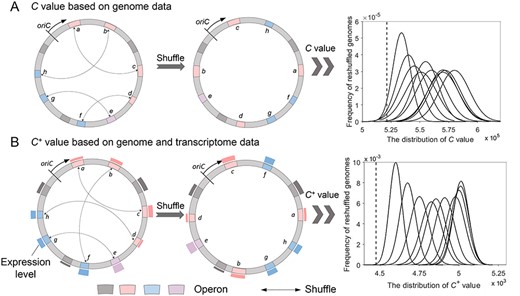
The calculation of C value and C+ value for the actual genome and reshuffled genomes. The blocks (pink, blue, gray and purple) represent operons. The two pathways that pink and blue operons participate in (the same color means those are involved in the same pathway) are used here to show the calculation of C and C+ value. Operon e participates in both these two pathways, and gray operons represent other operons on the genome. The bars outside the circle of B indicate the expression level of genes (operons), which can be assessed from transcriptomic data. oriC represents the origin of chromosomal replication. (A) The C value calculation of E. coli K12. (B) The C+ value calculation of E. coli K12. In each coordinate system, the x-axis represents the C or C+ value, and the y-axis represents the frequency of reshuffled genomes for a specific C or C+ value. We use 1 million permutations by randomly shuffling X% of operons (X = 10, 20, …, 100, respectively) and calculate the distribution of C or C+ value. In this way, 10 curves are generated corresponding to the specific X value from left to right. The dashed line represents the calculated C or C+ value of the actual E. coli K12 genome.
Despite much advance, the C value model neglects the transcriptional differences in pathways when quantifying the compactness of genomes, whereas the transcriptional frequency has been proven with a significant impact on global operon arrangement [19]. With this in mind, another extended model, C+ value was proposed to infer the locations of operons, where |${C}^{+}=\sum_{i=1}^N{f}_i\times \Big({t}_i+\alpha \sum_{j=1}^M{\omega}_{ij}\Big),$||$N$| is the number of pathways, |$M$| is the number of partitioned segments to be determined, |${f}_i$| is the transcriptional frequency of the ith pathway, |${t}_i$| is the number of partitioned segments containing operons of the ith pathway, |${\omega}_{ij}$| is the ratio of operons involved in the ith pathway but not covered by the jth partitioned segment and |$\alpha$| is a scaling factor. This model not only quantifies the effect of transcriptional frequencies but also assesses multiple clusters in each pathway (based on the hypothesis that a bacterial genome is partitioned to a set of genomic segments) [66]. By minimizing C+ value of a target genome, the operon locations and optimal partitions of a genome can be determined simultaneously with dynamic programming procedures [66]. Similar to the application of the C value model, C+ value was recalculated for both actual and reshuffled genomes of E. coli K12 to evaluate the performance of the model (both genome and transcriptome data were used here). Not surprisingly, the actual genome arrangement tends to minimize C+ value in comparison with those of alternatives (Figure 2B). The result showed that, compared with the previous C value model, the actual genome stands out more significantly from those artificially generated [66], suggesting a better performance of the improved C+ value model. The study substantially demonstrates that the pathway activation frequencies impose strong constraints on operon locations along the genome, contributing to a deep understanding of global arrangement of operons.
Note that both the C value and the C+ value are computational models based on the hypothesis from a large amount of data, and there is a lack of biological interpretation on the results. For example, what is the biological meaning of the predicted partitioned segments in the C+ value model? Fortunately, the subsequent study has proven that the predicted segments are consistent with the supercoiled structure of the folded chromosome (next section). Nevertheless, more work associated with biological explanations is required for a comprehensive viewpoint of genome organization.
Gene expression and replication affect chromosome structures
In bacteria, the double-strand DNA with a helical structure usually keeps a twisting state and folds compactly within cells [67,68], because if fully stretched out, the chromosome is approximately 1000 times longer than the length of the cell. Researchers have found that the folded conformations of chromosomes are highly variable under different conditions [69] (Figure 3A). More intriguingly, the varying conformations are not random but formed in a way that is compatible with multiple cellular processes [69]. Here, we discuss the functional factors (gene expression and DNA replication) that affect chromosome structures at different scales (supercoils, CIDs and MDs).
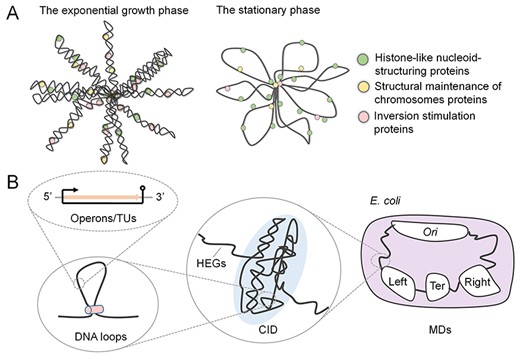
The folded chromosome in cells. The bacterial chromosomes are compactly organized into complex structures. (A) The chromosomal structures of E. coli are different in the exponential growth phase and the stationary phase. (B) The bacterial chromosome is organized at different scales. The operons/TUs along the genome will form DNA loops and large chromosome substructures (i.e. CIDs). Further, independently organized domains (MDs) can be observed.
Supercoils
Generally, the helix DNA strands undergo underwound or overwound conditions, resulting in turns of the entire DNA molecule and forming a tertiary structure, called supercoil [70]. Due to the distinct directions of twisting, supercoils are classified as positive supercoils and negative supercoils (twisting in right-handed and left-handed directions, respectively). Bacterial DNA strands are often found to be underwound and introduce more negative supercoils with the help of various topoisomerases [71,72]. It has been demonstrated that the variability of negative supercoils (in both number and structure [70]) is closely related to the implementation of cell functions and can contribute to localizing conformational changes (e.g. DNA breakage) [73]. For example, when the bacteria immunity system (clustered regularly interspaced short palindromic repeats associated system) is stimulated [74–80], the number of negative supercoils will increase to accelerate the R-loop formation between guide RNA and target DNA, thereby preventing foreign DNA transcription [81,82]. Additionally, the density of negative supercoils is associated with varying levels of gene expression and metabolism processes. Studies have found that negatively supercoiled genes are generally more efficiently transcribed than compact positively supercoiled genes [83,84]. Therefore, bacterial chromosomes in the exponential growth stage are highly negatively supercoiled, which has more looped domains compared to those in the stationary stage [83,84]. Notably, the density of negative and positive supercoils is changeable. However, overall supercoiling levels would be controlled to ensure primary bacterial life via topoisomerases due to the impact of supercoils on replication processes (accumulation of negative supercoils lead to the catenation of newly synthesized DNA strands and hinder chromosome segregation, while excess positive supercoils block the replisome progression) [85].
Based on such general understanding, more attention is given to the specific folded conformations of bacterial chromosomes. Considering the experimental data of chromosomal conformations are limited, several computational models are carried out aiming at a deep understanding of genome organization [86,87]. In view of the high correlation of chromosome conformations (the structure of supercoils) with bacterial transcription, Ma et al. inferred that the supercoiled structure would form in a way that facilitates the realization of transcription (i.e. keep energy consumption minimum for the folding and unfolding of supercoils during transcription) [31,88]. Based on this idea, a function called E value (|$E=\sum_{i=1}^N{\omega}_i{s}_i$|, where |${s}_i$| is the number of supercoils containing the operons of the |${i}^{th}$| pathway and |${\omega}_i$| is the transcriptional frequencies of the ith pathway) was developed to measure the energy consumption of transcription [31]. In this function, the energy is quantified proportional to the number of supercoils containing genes/operons of the pathway multiplied by its transcription frequency. It is noteworthy that the transcription frequency can be assessed by gene expression data [19], and a pathway is considered as activated if and only if at least a certain number of its operons/genes are activated [31]. Ten E. coli strains were used to validate the usefulness of the function [89,90]. Results showed that the inferred dynamic structures of supercoil folding domains are consistent with the experimental data that assist the folding of chromosomal domains [31]. Additionally, the analysis suggested that E value is highly correlated to the cell growth rate (with correlation coefficient −0.8) [89,90], which is intuitively reliable with the fact that less consumption accompanies faster transcription.
Chromosome interaction domains
The progress in experimental technologies, such as 3C [91], 4C [92], 5C [91], Hi-C [91] and ChIP-seq [21], has greatly improved the understanding of the conformations of bacterial chromosomes, in which 4C, 5C and Hi-C are advanced versions of 3C to capture chromosome structures [91], and ChIP-seq contributes to detecting focused protein binding on the wide genome [21] (Box 1). Based on these technologies, it has been found that the bacteria DNA conformation relies heavily on various NAPs [93], which have various structural effects on DNA. For example, the histone-like nucleoid-structuring proteins form filaments [94,95] and bridges between two DNA segments [21,96], while structural maintenance of chromosomes proteins extrudes DNA loops [97–103]. Additionally, the inversion stimulation proteins collaborated with heat-stable proteins which help DNA bending and twisting [104,105]. Through Hi-C contact maps, researchers observed that the small DNA loops by NAPs form larger chromosome substructures along the genomes (i.e. CIDs with sizes ranging from 30 to ~500kbp) [106,107] (Figure 3B). These CIDs are highly self-interacting genomic regions and relatively insulated from others [21,108]. Current studies have suggested that HEGs play a direct role in defining CID boundaries [109,110]. The E. coli chromosome is organized into 31 CIDs, in which 22 are consistent with locations of HEGs [111]. Moreover, HEGs are clustered to varying degrees under different conditions, contributing to the CID boundaries with distinct characteristics, such as sharpening boundaries while undergoing exponential growth and diminishing boundaries in the starvation state [67]. This phenomenon indicates that transcription is closely associated with genome organization at a higher level.
Experimental technologies used to study chromosome structures
3C [91]: 3C is a technology to capture the interaction between a paired genomic loci. After a series of restricted digesting, religating, reverse cross-linking and polymerase chain reaction (PCR), an interaction between two gene loci exists when ligation products exist. It is a ‘one-to-one’ approach and has enormous difficulties in designing quantified specified ligation products. 4C [92]: 4C is a technology to quantify the interactions between one locus and all other genomic loci. Different from 3C and 5C (see below), it does not need the prior knowledge of interacting chromosomal regions, and it involves a second ligation step to create self-circularized DNA fragments for inverse PCR. Inverse PCR allows the known sequence to be used to amplify the unknown sequence ligated to it. 5C [91]: 5C is another improvement of 3C to capture interactions between all fragments within a given region. It is a ‘many-to-many’ approach and can be performed by ligating universal primers to all fragments. Hi-C [91]: Hi-C represents the technology to analyze the structure of genomes. The most distinguishing feature is that Hi-C is an ‘all-to-all’ approach. It can offer a complete spatial view of the DNA information. Even genomic elements far away from each other, like HEGs and regulatory factors, can find the interactions among them thanks to the huge library. ChIP-seq [21]: ChIP-seq is a widely used technology and is convenient in identifying the binding sequence combined with PCR. It is a technology that combines Hi-C with chromatin immunoprecipitation by using a specific antibody. It is a ‘many-to-many’ approach. The advantage is the capability of identifying a wide range of interactions even if it is rare, while the disadvantage is the difficulties in interpreting data. FROS [21]: FROS is a single-cell technology offering the dynamics of chromosomes. Specific loci can be tagged and tracked in live cells to define the spatial position and interrelationship of positions, providing the dynamics of interesting loci. However, it is limited in its throughput.
Macrodomains
More advances in genome technologies, such as FROS [21] (Box 1), enable researchers to determine the positions of genomic loci and track the dynamics of specific loci, improving the understanding of genome organization at the macro scale. By visualizing the locations of up to 100 genomic loci, the origin and termination sites of bacterial replication were revealed not located randomly but in specific positions [112]. This fundamental feature of chromatin organization has been found associated with the macroscale domains of bacterial chromosomes [33]. Specifically, E. coli has the well-defined structure with four MDs (Ori MD, Ter MD, Right and Left MDs) (Figure 3B) and two nonstructured (NS) regions based on 3C and DNA–DNA interaction studies [113,114], in which Ori MD contains the origin site of DNA replication [115], while Ter MD contains the terminators of the replication process. Further studies suggested that the positions of loci on the chromosome are not stable. The loci in NS regions are under fewer constraints than loci in MDs [114,115]. Loci in Ter MD are the least mobile, while mobility increases along the chromosomal arms towards Ori MD [116]. Nevertheless, the mechanisms of the positions of genomic loci and chromosome separate processes are far from clear. Although several proteins related to the organization of MDs, such as the gene product named MaoP and MatP, have been studied [117], more attention is required to explore the structural relations with function.
Collectively, the organization of chromosomes is not random but associated with critical cellular functions, from supercoils to CIDs and MDs. Further studies are expected to deepen the understanding of how cellular processes shape the chromosomes, which will, in turn, help reveal the mechanisms of complex cellular processes.
DNA replication, transcription and gene products direct gene strand bias
In addition to the organization of operons and chromosomal conformation, another significant pattern within bacterial genomes is the preferential location on the leading strand. It has been observed that the majority of bacterial genes, with percentages ranging from 50% to 90% across different species, are preferably located on the leading strand in a genome [23,24]. This observation is well known as strand-biased gene distribution (SGD) [118]. Currently, a large number of studies have been performed, aiming at a mechanistic explanation of this observation. A key finding revealed in these studies is that the collisions between replication and transcription result in SGD. Since replication and transcription occur simultaneously based on the same DNA template but proceed at different rates, collisions are inevitable between them (head-on collisions occur if the gene is in the lagging strand, while co-oriented collisions occur if the gene is in the leading strand) [119]. However, the collisions are deleterious, such as leading to abortive transcription or stalling replication [118]. Recent experimental results suggested that head-on collisions are more deleterious than co-oriented collisions, making the genes more prone to exert negative mutations when performing specific functions [118]. Therefore, the genes with essential functions (essentiality-driven hypotheses [119]) or high expression (expression-driven hypotheses [120]) are preferably located on leading strands [22].
Despite these insights, neither essentiality-driven hypotheses nor expression-driven hypotheses can explain SGD thoroughly. By removing highly expressed genes and essential genes from multiple bacterial species, the phenomena of SGD were decreased in varying degrees of different bacteria but not completely removed [118]. For a more comprehensive understanding of SGD, Mao et al. [121] explored other factors that may affect the strand bias in a bacterium. They found that genes with specific functions prefer different strands and consequently cause SGD, such as genes of ribosome prefer to locate on the leading strands, while genes of transcription factor prefer the lagging strands [121]. Additionally, Gao et al. [118] proposed the energy efficiency model, and they argued that the locations of genes tend to under strong selection for efficient energy usage. Since lagging-strand genes encode more expensive protein products (the proteins whose synthesis requires cost more energy) than leading-strand ones, genes prefer to located on the leading strand [118], especially those highly expressed. Nevertheless, the understanding of SGD is not comprehensive yet. If more sophisticated analyses, including the living environments and the survivability requirements of bacteria, could be considered, the quantitative models that integrated more parameters will be established, contributing to a comprehensive insight into the factors that drive SGD [121].
Conclusions and discussions
Recent advances in genomic studies have revealed the bacterial genomes are highly organized with functional implications. Here, we overviewed the well-studied organization during bacterial evolution, including operons, TUs, supercoils, CIDs, MDs and SGD. Meanwhile, there are several other structures, such as overlapping genes, playing important roles in improving genome compaction and being associated with translational coupling [122], which will not be extended into more details here.
The summarized knowledge in this manuscript will contribute to the studies of functional activities of bacteria [123]. For example, there are some missing enzymes to catalyze reactions in a pathway (i.e. pathway holes) [124], and we can apply the understanding of global gene arrangement to fill the holes. By identifying all the metabolic pathways that involve an unassigned enzyme based on its EC number and its substrates and products, we can predict the supercoils containing operons for each of the involved pathways. In this way, the candidate gene would lie in a supercoil covered by all these pathways, i.e. within a narrow genomic range, for researchers to detect.
Additionally, the understanding of genome organization improves the genome assembly process, which in turn benefits the genomic studies. Advances in next-generation sequencing offer a large number of raw datasets consisting of short reads, which make genome assembly necessary [125]. Most current approaches depend heavily on phylogenetic relationships among species to select close-related reference genome for the separate contigs ordering during assembly [126–128]. However, due to the intensive rearrangement during bacterial evolution, the same genome organization may present in remotely related strains rather than closely related strains [11,129], which would lead to systemic errors when assembly contigs based on the closely related reference. Fortunately, focusing on the genome organization, the high-order conservation of core genes along the genome is generalized [130]. Therefore, one can envision that ordering the contigs, by extracting the order-conserved genes from known complete genomes [131], provides a reliable draft genome for a full understanding of bacteria at both taxonomic and functional levels.
More importantly, the study of bacterial genomes allows the understanding of diseases in a new perspective. The explosion of meta-omics data of human microbiomes provides an unprecedented opportunity to study the relations between microbes and diseases in species levels [132,133]. Microbes have been demonstrated with significant associations with diseases [134]. Based on the well-studied genome organization, we can explore markers of diseases that go beyond species classification, such as the operon/TU structure [135,136]. These information will provide deep insights into the occurrence of specific diseases, such as tuberculosis and gastric cancer [135,136].
Notably, current studies remain to be improved for a system-level understanding of bacterial genomes. Firstly, the findings based solely on data from informatics analyses or predictions can be misleading. Extensive experimental validation of genome structures, from operons to chromosomes, will be significant to iteratively construct, test and improve models for understanding genome organization. Secondly, the computational model should be developed to keep up with advanced experimental data, such as the increasing data from chromosome capture technologies. One can apply these data to study the mechanisms of genome organization and validate the effectiveness of proposed models. We expect the field of the genomic organization of bacteria, more broadly of all microbes, would be active and exciting in the coming years.
Bacterial genomes interact intimately with multiple cellular processes. These interactions impose constraints on genome organization.
The genome organization of bacteria, such as the local structure as operons, transcription units and alternative transcription units, and global chromosome architecture as supercoils, chromosome interaction domains and macrodomains, has close associations with functional activities (e.g. metabolism, transcription and replication). Additionally, gene strand bias is another significant pattern within bacterial genomes, which can be explained by multiple cellular processes.
Bacterial genome organization contributes to the studies of functional activities of bacteria. One can apply the insights of genome organization to multiple contexts, such as pathway holes, genome assembly and the mechanistic understanding of human diseases.
Despite much progress, current studies of bacterial genome organization remain to be improved from both experimental and computational perspectives.
Authors’ contributions
Q.M. and B.L. conceived the project. Z.L., J.F. and B.Y. collected materials. J.F. drafted the chromosome and gene strand bias sections, as well as data analysis of C value and C+ value. Z.L. drafted other sections and prepared all figures. All the authors revised the manuscript. The authors declare that they have no competing interests.
Acknowledgments
The authors would like to thank Xianquan Wei and Yu Gan from Shandong University for their effort in collecting literature. The authors want to thank Anjun Ma from the Ohio State University for his valuable suggestions on figures.
Funding
This work was supported by the National Nature Science Foundation of China (61772313, 11931008), Interdisciplinary Science Innovation Group Project of Shandong University (2019) and the Innovation Method Fund of China (Ministry of Science and Technology of China) (2018IM020200).
Conflict of interest
None.
Zhaoqian Liu is a PhD student in School of Mathematics, Shandong University, and now she is a visiting scholar at the Ohio State University. Her research interest is microbiome studies.
Jingtong Feng is a master student in School of Mathematics, Shandong University. Her research interest is the bacterial structure.
Bin Yu, PhD, is an associate professor in College of Mathematics and Physics, Qingdao University of Science and Technology. His primary research interests are artificial intelligence, data mining and biomedical image processing.
Qin Ma, PhD, is an associate professor in the Department of Biomedical Informatics, the Ohio State University. Dr Ma has over 10 years of research experience in studying how functional machinery encoded in a (meta-)genome.
Bingqiang Liu, PhD, is a professor in School of Mathematics, Shandong University. His primary research strength is microbial regulatory network construction.