-
PDF
- Split View
-
Views
-
Cite
Cite
Shiqiang Cheng, Bei Han, Miao Ding, Yan Wen, Mei Ma, Lu Zhang, Xin Qi, Bolun Cheng, Ping Li, Om Prakash Kafle, Xiao Liang, Li Liu, Yanan Du, Yan Zhao, Feng Zhang, Identifying psychiatric disorder-associated gut microbiota using microbiota-related gene set enrichment analysis, Briefings in Bioinformatics, Volume 21, Issue 3, May 2020, Pages 1016–1022, https://doi.org/10.1093/bib/bbz034
- Share Icon Share
Abstract
Psychiatric disorders are a group of complex psychological syndromes with high prevalence. It has been reported that gut microbiota has a dominant influence on the risks of psychiatric disorders through gut microbiota–brain axis. We extended the classic gene set enrichment analysis (GSEA) approach to detect the association between gut microbiota and complex diseases using published genome-wide association study (GWAS) and GWAS of gut microbiota summary data. We applied our approach to real GWAS data sets of five psychiatric disorders, including attention deficiency/hyperactive disorder (ADHD), autism spectrum disorder (AUT), bipolar disorder (BD), schizophrenia (SCZ) and major depressive disorder (MDD). To evaluate the performance of our approach, we also tested the genetic correlations of obesity and type 2 diabetes with gut microbiota. We identified several significant associations between psychiatric disorders and gut microbiota, such as ADHD and genus Desulfovibrio (P = 0.031), order Clostridiales (P = 0.034). For AUT, association signals were observed for genera Bacteroides (P = 0.012) and Desulfovibrio (P = 0.033). Genus Desulfovibrio (P = 0.005) appeared to be associated with BD. For MDD, association signals were observed for genus Desulfovibrio (P = 0.003), order Clostridiales (P = 0.004), family Lachnospiraceae (P = 0.007) and genus Bacteroides (P = 0.007). Genus Desulfovibrio (P = 0.012) and genus Bacteroides (P = 0.038) appeared to be associated with SCZ. Our study results provide novel clues for revealing the roles of gut microbiota in psychiatric disorders. This study also illustrated the good performance of GSEA approach for exploring the relationships between gut microbiota and complex diseases.
Introduction
Psychiatric disorders refer to a group of complex psychological syndromes, mainly characterized by clinically significant disturbance in cognition, emotion regulation or behavior [1]. The common psychiatric disorders include the attention deficiency/hyperactive disorder (ADHD), autism spectrum disorder (AUT), bipolar disorder (BD), schizophrenia (SCZ) and major depressive disorder (MDD). Psychiatric disorders have been found commonly, with over a third of people in most countries reporting sufficient criteria to be diagnosed at some points of their lives [2]. Epidemiological research has shown that about 3–18% of children suffered psychiatric disorders causing significant functional impairment [3]. Costello et al. [3] have proposed a median prevalence estimate of 12%.
The etiology of psychiatric disorders is still unclear now. The predominant view at present is that biological, psychological and environmental factors all contribute to the occurrence and development of psychiatric disorders [4]. For instance, multiple epidemiological [5–7] and molecular biological [8–11] studies have observed shared risk components among psychiatric disorders. Similar environmental risk factors, like physical abuse and neglect, have also been found to underlie a range of psychiatric disorders, for instance SCZ and depression [12]. However, the etiology and molecular mechanism of psychiatric disorders remain elusive now.
Humans have complex interactions with the bacteria that exist inside and on the surface of the body, referred as the microbiota [13]. The changes of composition and abundance of microbiota, particularly the intestinal microbiota, can greatly affect host health and play a vital role in many metabolite pathways related to human complex diseases, such as obesity [14, 15], Crohn's disease [16, 17], type 2 diabetes (T2D) [18] and rheumatoid arthritis [19]. Cryan et al. suggested that the gut microbiota had a dominant influence on signaling along the microbiota–gut–brain axis, a bidirectional communication network encompassing the gut microbiota and the nervous system, as well as neuroendocrine and neuroimmune pathways [20–22]. Remarkably, emerging evidence has suggested the role of gut microbiota in brain function and behavior [23–25]. In turn, intestinal dysbiosis has been linked to different neuropsychiatric syndromes [23–25].
The composition and abundances of human microbiota are influenced by many factors, such as the delivery method at birth [26], feeding modes during infancy [27] and diet [28]. Recent genome-wide association studies of gut microbiota (GWASGM) confirmed the important role of host genetics on determining the composition and functions of human gut microbiome, with some bacterial taxa having heritability estimates as high as 0.39 [29–31]. Host genetics and the gut microbiome can both influence metabolic phenotypes [30]. In an independent cohort study, Knights et al. [32] have reported that NOD2 risk allele-associated inflammatory bowel disease was significantly related to the increased abundance of Enterobacteriaceae. It would allow us to understand the characteristics and metabolism of these health-associated bacteria, eventually to exploit them to promote health once the interactions between host genetics and the microbiome are understood.
Gene set enrichment analysis (GSEA) is capable of identifying groups of genes that share common biological function or regulation for target diseases [33]. The goal of GSEA is to determine whether members of a gene set are correlated with the phenotypic class distinction [33]. By integrating the biological information of multiple genes, GSEA provides additional pathogenetic information that is undetectable for traditional single gene analysis [33]. The power of GSEA tools have led to their wide use in genomic research. For example, a growing body of researches has demonstrated the utility of GSEA to identify functional pathways using genetically defined systems [34–36]. Based on the advantage of GSEA, we developed a novel approach to explore the relationships between gut microbiota and complex diseases by utilizing publicly available genome-wide association study (GWAS) summary data. We applied our approach to real GWAS data sets of five major psychiatric disorders, as well as obesity and T2D.
Materials and methods
GWAS summary data of psychiatric disorders
The latest GWAS summary data of ADHD (19 099 cases and 34 194 controls), AUT (7387 cases and 8567 controls), BD (20 129 cases and 21 524 controls), SCZ (33 426 cases and 32 541 controls) and MDD (135 458 cases and 344 901 controls) were downloaded from the Psychiatric GWAS Consortium website (https://www.med.unc.edu/pgc/results-and-downloads) [37–40]. Briefly, all study subjects were European whites and diagnosed according to the criteria of the Diagnostic and Statistical Manual of Mental Disorders. Detailed description of sample characteristics, Single Nucleotide Polymorphisms (SNP) genotyping, imputation, association analysis, experimental design and statistical analysis can be found in the published studies (Supplementary Table S1).
GWAS summary data of BMI and T2D
Compared with psychiatric disorders, more efforts have been paid to explore the functional relevance of gut microbiota and with obesity and T2D. To illustrate the performance of our proposed approach, we applied it to detect the associations of gut microbiota with obesity and T2D. The GIANT GWAS summary data (including 339 224 subjects) of body mass index (BMI), waist circumference adjusted for BMI (WAISTadjBMI) and waist-to-hip ratio adjusted for BMI (WHRadjBMI) were downloaded (http://portals.broadinstitute.org/collaboration/giant/index.php/GIANT_consortium_data_files). The GWAS summary data of T2D contains 26 488 cases and 83 964 controls of European, East Asian, South Asian and Mexican and Mexican American ancestry [41].
Gut microbiota-related gene sets
Recent studies demonstrated the important impact of host genetics on the composition and abundances of human gut microbiome [29–31]. The gut microbiota-associated host genes were collected from recently published GWAS of gut microbiota (GWASGM) [30, 42–44]. Briefly, we conducted a manual literature search for significant genetic associations between host genes and gut microbiota composition (including kingdom, phylum, class, order, family, genus and species), detected by the published GWASGM [30, 42–44]. The genes significantly associated with gut microbiota composition were extracted and combined to generate the gene sets for each gut microbiota analyzed by this study. The detailed description of experimental design, sample characteristics, genotyping, imputation, association analysis and statistical analysis can be found in the published GWASGM studies (Supplementary Table S2).
Gut microbiota-related GSEA
The classic GWAS-based GSEA algorithm was used here [45]. Briefly, utilizing the generated gut microbiota-related gene sets, GSEA was applied to the GWAS summary data of psychiatric disorders to determine whether psychiatric disorder-associated genes were significantly enriched in a gut microbiota-related gene set. Let Lm denote the most significant GWAS statistic (for example, chi-square test statistic) of a gene. All genes are ranked by sorting Lm from maximum to minimum |$({L}_{m1}\ge {L}_{m2}\ge \dots .{L}_{mN})$|, denoted as |${L}_m=[{L}_{m1,}{L}_{m2},\dots .{L}_{mN}]$|. For a given gut microbiota-related gene set A with |${N}_{\mathrm{A}}$| genes, let |${G}_i$| denote the ith gene of gene set A. Let |${\mathrm{ES}}_m^{\mathrm{A}}$| denote the enrichment scores (ESs) of gene set A, which is calculated by weighted Kolmogorov–Smirnov-like running sum statistic, defined by
Type I error rates evaluation
To evaluate the type I error rates of our analysis approach, we also generate a random gene set annotation file. The Kyoto Encyclopedia of Genes and Genomes (KEGG) pathway annotation database was downloaded from GSEA Molecular Signatures Database (http://software.broadinstitute.org/gsea/msigdb/index.jsp) [33]. This downloaded KEGG pathway annotation data contains 185 annotated biological pathways. The random gene set annotation file was then generated by randomly shuffling the gene labels of the KEGG pathway annotation file. The randomly generated gene set annotation file was used for GSEA of five psychiatric disorders, BMI and T2D, instead of the real gut microbiota-related gene set annotation file. Figure 1 presents the study design and data analysis procedure of this study.
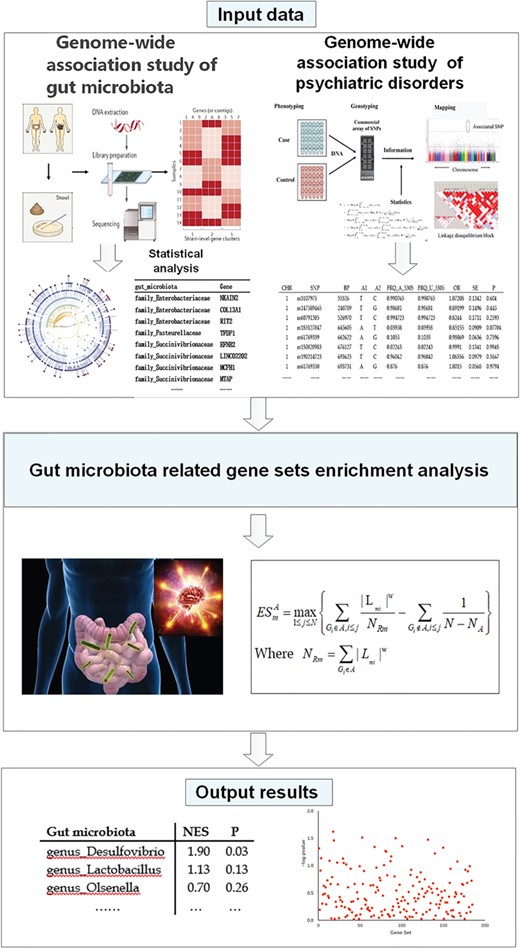
Results
Gut microbiota-related GSEA of psychiatric disorders
We detected several significant associations between gut microbiota and psychiatric disorders (permutated empirical P < 0.05). For ADHD, association signals were observed for genus Desulfovibrio (empirical P = 0.031) and order Clostridiales (empirical P = 0.034). For AUT, association signals were observed for genus Bacteroides (empirical P = 0.012) and Desulfovibrio (empirical P = 0.033). Genus Desulfovibrio (empirical P = 0.005) appeared to be associated with BD. For MDD, association signals were observed for genus Desulfovibrio (empirical P = 0.003), order Clostridiales (empirical P = 0.004), family Lachnospiraceae (empirical P = 0.007) and genus Bacteroides (empirical P = 0.007). Genera Desulfovibrio (empirical P = 0.012) and Bacteroides (empirical P = 0.038) appeared to be associated with SCZ (Table 1).
Association analysis results of gut microbiota and psychiatric disorders (empirical P < 0.05)
Gut microbiota . | Empirical P-values . | . | ||||
---|---|---|---|---|---|---|
. | ADHD . | AUT . | BD . | MDD . | SCZ . | |
genus_Desulfovibrio | 0.031 | 0.033 | 0.005 | 0.003 | 0.012 | |
genus_Lactobacillus | – | – | – | – | – | |
genus_Olsenella | – | – | – | – | – | |
k Bacteria_p Bacteroidetes_c Bacteroidia_o Bacteroidales | – | – | – | – | – | |
k Bacteria_p Bacteroidetes_c Bacteroidia_o Bacteroidales_f Rikenellaceae | – | – | – | – | – | |
k Bacteria_p Firmicutes_c Clostridia | – | – | – | – | – | |
k Bacteria_p Firmicutes_c Clostridia_o Clostridiales_f Veillonellaceae_g Megamonas | – | – | – | – | – | |
k Bacteria-p Bacteroidetes-c Bacteroidia-o Bacteroidales-f Bacteroidaceae-g Bacteroides | – | 0.012 | – | 0.007 | 0.038 | |
k Bacteria-p Firmicutes-c Clostridia-o Clostridiales | 0.034 | – | – | 0.004 | – | |
k Bacteria-p Firmicutes-c Clostridia-o Clostridiales-f Lachnospiraceae | – | – | – | 0.007 | – | |
k Bacteria-p Firmicutes-c Clostridia-o Clostridiales-f Ruminococcaceae | – | – | – | – | – | |
order_Rhizobiales | – | – | – | – | – |
Gut microbiota . | Empirical P-values . | . | ||||
---|---|---|---|---|---|---|
. | ADHD . | AUT . | BD . | MDD . | SCZ . | |
genus_Desulfovibrio | 0.031 | 0.033 | 0.005 | 0.003 | 0.012 | |
genus_Lactobacillus | – | – | – | – | – | |
genus_Olsenella | – | – | – | – | – | |
k Bacteria_p Bacteroidetes_c Bacteroidia_o Bacteroidales | – | – | – | – | – | |
k Bacteria_p Bacteroidetes_c Bacteroidia_o Bacteroidales_f Rikenellaceae | – | – | – | – | – | |
k Bacteria_p Firmicutes_c Clostridia | – | – | – | – | – | |
k Bacteria_p Firmicutes_c Clostridia_o Clostridiales_f Veillonellaceae_g Megamonas | – | – | – | – | – | |
k Bacteria-p Bacteroidetes-c Bacteroidia-o Bacteroidales-f Bacteroidaceae-g Bacteroides | – | 0.012 | – | 0.007 | 0.038 | |
k Bacteria-p Firmicutes-c Clostridia-o Clostridiales | 0.034 | – | – | 0.004 | – | |
k Bacteria-p Firmicutes-c Clostridia-o Clostridiales-f Lachnospiraceae | – | – | – | 0.007 | – | |
k Bacteria-p Firmicutes-c Clostridia-o Clostridiales-f Ruminococcaceae | – | – | – | – | – | |
order_Rhizobiales | – | – | – | – | – |
Note: attention deficiency/hyperactive disorder, ADHD; autism spectrum disorder, AUT; bipolar disorder, BD; schizophrenia, SCZ; major depressive disorder MDD.
Association analysis results of gut microbiota and psychiatric disorders (empirical P < 0.05)
Gut microbiota . | Empirical P-values . | . | ||||
---|---|---|---|---|---|---|
. | ADHD . | AUT . | BD . | MDD . | SCZ . | |
genus_Desulfovibrio | 0.031 | 0.033 | 0.005 | 0.003 | 0.012 | |
genus_Lactobacillus | – | – | – | – | – | |
genus_Olsenella | – | – | – | – | – | |
k Bacteria_p Bacteroidetes_c Bacteroidia_o Bacteroidales | – | – | – | – | – | |
k Bacteria_p Bacteroidetes_c Bacteroidia_o Bacteroidales_f Rikenellaceae | – | – | – | – | – | |
k Bacteria_p Firmicutes_c Clostridia | – | – | – | – | – | |
k Bacteria_p Firmicutes_c Clostridia_o Clostridiales_f Veillonellaceae_g Megamonas | – | – | – | – | – | |
k Bacteria-p Bacteroidetes-c Bacteroidia-o Bacteroidales-f Bacteroidaceae-g Bacteroides | – | 0.012 | – | 0.007 | 0.038 | |
k Bacteria-p Firmicutes-c Clostridia-o Clostridiales | 0.034 | – | – | 0.004 | – | |
k Bacteria-p Firmicutes-c Clostridia-o Clostridiales-f Lachnospiraceae | – | – | – | 0.007 | – | |
k Bacteria-p Firmicutes-c Clostridia-o Clostridiales-f Ruminococcaceae | – | – | – | – | – | |
order_Rhizobiales | – | – | – | – | – |
Gut microbiota . | Empirical P-values . | . | ||||
---|---|---|---|---|---|---|
. | ADHD . | AUT . | BD . | MDD . | SCZ . | |
genus_Desulfovibrio | 0.031 | 0.033 | 0.005 | 0.003 | 0.012 | |
genus_Lactobacillus | – | – | – | – | – | |
genus_Olsenella | – | – | – | – | – | |
k Bacteria_p Bacteroidetes_c Bacteroidia_o Bacteroidales | – | – | – | – | – | |
k Bacteria_p Bacteroidetes_c Bacteroidia_o Bacteroidales_f Rikenellaceae | – | – | – | – | – | |
k Bacteria_p Firmicutes_c Clostridia | – | – | – | – | – | |
k Bacteria_p Firmicutes_c Clostridia_o Clostridiales_f Veillonellaceae_g Megamonas | – | – | – | – | – | |
k Bacteria-p Bacteroidetes-c Bacteroidia-o Bacteroidales-f Bacteroidaceae-g Bacteroides | – | 0.012 | – | 0.007 | 0.038 | |
k Bacteria-p Firmicutes-c Clostridia-o Clostridiales | 0.034 | – | – | 0.004 | – | |
k Bacteria-p Firmicutes-c Clostridia-o Clostridiales-f Lachnospiraceae | – | – | – | 0.007 | – | |
k Bacteria-p Firmicutes-c Clostridia-o Clostridiales-f Ruminococcaceae | – | – | – | – | – | |
order_Rhizobiales | – | – | – | – | – |
Note: attention deficiency/hyperactive disorder, ADHD; autism spectrum disorder, AUT; bipolar disorder, BD; schizophrenia, SCZ; major depressive disorder MDD.
Gut microbiota-related gene sets enrichment analysis for obesity and T2D
As shown by Table 2, we observed significant associations (all permutated empirical P < 0.05) of gut microbiota with obesity and T2D-related phenotypes. BMI and WHRadjBMI appeared to be associated with order Clostridiales (empirical P = 0.001), family Ruminococcaceae (empirical P = 0.018), Lachnospiraceae (empirical P = 0.019), genera Bacteroides (empirical P = 0.025) and Lactobacillus (empirical P = 0.026). For WAISTadjBMI, association signals were observed for family Ruminococcaceae (empirical P = 0.013) and order Clostridiales (empirical P = 0.027).
Association analysis results of gut microbiota, obesity and T2D (empirical P < 0.05)
Gut microbiota . | Empirical P-values . | |||||
---|---|---|---|---|---|---|
BMI . | WAISTadjBMI . | WHRadjBMI . | FAST_female . | FAST_male . | T2D . | |
genus_Desulfovibrio | – | – | 0.075 | 0.018 | 0.012 | 0.015 |
genus_Lactobacillus | 0.026 | – | 0.026 | – | – | – |
genus_Olsenella | – | – | – | – | – | – |
k Bacteria_p Bacteroidetes_c Bacteroidia_o Bacteroidales | – | – | – | – | – | – |
k Bacteria_p Bacteroidetes_c Bacteroidia_o Bacteroidales_f Rikenellaceae | – | – | – | – | – | – |
k Bacteria_p Firmicutes_c Clostridia | – | – | – | 0.003 | – | – |
k Bacteria_p Firmicutes_c Clostridia_o Clostridiales_f Veillonellaceae_g Megamonas | – | – | – | – | – | – |
k Bacteria-p Bacteroidetes-c Bacteroidia-o Bacteroidales- f Bacteroidaceae g Bacteroides | 0.025 | – | 0.025 | – | 0.011 | – |
k Bacteria-p Firmicutes-c Clostridia-o Clostridiales | 0.001 | 0.027 | 0.001 | – | 0.003 | – |
k Bacteria-p Firmicutes-c Clostridia-o Clostridiales-f Lachnospiraceae | 0.019 | – | 0.019 | – | – | 0.005 |
k Bacteria-p Firmicutes-c Clostridia-o Clostridiales-f Ruminococcaceae | 0.018 | 0.013 | 0.018 | 0.044 | 0.002 | – |
order_Rhizobiales | – | – | – | – | – | – |
Gut microbiota . | Empirical P-values . | |||||
---|---|---|---|---|---|---|
BMI . | WAISTadjBMI . | WHRadjBMI . | FAST_female . | FAST_male . | T2D . | |
genus_Desulfovibrio | – | – | 0.075 | 0.018 | 0.012 | 0.015 |
genus_Lactobacillus | 0.026 | – | 0.026 | – | – | – |
genus_Olsenella | – | – | – | – | – | – |
k Bacteria_p Bacteroidetes_c Bacteroidia_o Bacteroidales | – | – | – | – | – | – |
k Bacteria_p Bacteroidetes_c Bacteroidia_o Bacteroidales_f Rikenellaceae | – | – | – | – | – | – |
k Bacteria_p Firmicutes_c Clostridia | – | – | – | 0.003 | – | – |
k Bacteria_p Firmicutes_c Clostridia_o Clostridiales_f Veillonellaceae_g Megamonas | – | – | – | – | – | – |
k Bacteria-p Bacteroidetes-c Bacteroidia-o Bacteroidales- f Bacteroidaceae g Bacteroides | 0.025 | – | 0.025 | – | 0.011 | – |
k Bacteria-p Firmicutes-c Clostridia-o Clostridiales | 0.001 | 0.027 | 0.001 | – | 0.003 | – |
k Bacteria-p Firmicutes-c Clostridia-o Clostridiales-f Lachnospiraceae | 0.019 | – | 0.019 | – | – | 0.005 |
k Bacteria-p Firmicutes-c Clostridia-o Clostridiales-f Ruminococcaceae | 0.018 | 0.013 | 0.018 | 0.044 | 0.002 | – |
order_Rhizobiales | – | – | – | – | – | – |
Note: body mass index, BMI; waist circumference adjusted for BMI, WAISTadjBMI; waist-to-hip ratio adjusted for BMI, WHRadjBMI; type 2 diabetes, T2D; male fasting glucose and fasting insulin, FAST_male; female fasting glucose and fasting insulin, FAST_female.
Association analysis results of gut microbiota, obesity and T2D (empirical P < 0.05)
Gut microbiota . | Empirical P-values . | |||||
---|---|---|---|---|---|---|
BMI . | WAISTadjBMI . | WHRadjBMI . | FAST_female . | FAST_male . | T2D . | |
genus_Desulfovibrio | – | – | 0.075 | 0.018 | 0.012 | 0.015 |
genus_Lactobacillus | 0.026 | – | 0.026 | – | – | – |
genus_Olsenella | – | – | – | – | – | – |
k Bacteria_p Bacteroidetes_c Bacteroidia_o Bacteroidales | – | – | – | – | – | – |
k Bacteria_p Bacteroidetes_c Bacteroidia_o Bacteroidales_f Rikenellaceae | – | – | – | – | – | – |
k Bacteria_p Firmicutes_c Clostridia | – | – | – | 0.003 | – | – |
k Bacteria_p Firmicutes_c Clostridia_o Clostridiales_f Veillonellaceae_g Megamonas | – | – | – | – | – | – |
k Bacteria-p Bacteroidetes-c Bacteroidia-o Bacteroidales- f Bacteroidaceae g Bacteroides | 0.025 | – | 0.025 | – | 0.011 | – |
k Bacteria-p Firmicutes-c Clostridia-o Clostridiales | 0.001 | 0.027 | 0.001 | – | 0.003 | – |
k Bacteria-p Firmicutes-c Clostridia-o Clostridiales-f Lachnospiraceae | 0.019 | – | 0.019 | – | – | 0.005 |
k Bacteria-p Firmicutes-c Clostridia-o Clostridiales-f Ruminococcaceae | 0.018 | 0.013 | 0.018 | 0.044 | 0.002 | – |
order_Rhizobiales | – | – | – | – | – | – |
Gut microbiota . | Empirical P-values . | |||||
---|---|---|---|---|---|---|
BMI . | WAISTadjBMI . | WHRadjBMI . | FAST_female . | FAST_male . | T2D . | |
genus_Desulfovibrio | – | – | 0.075 | 0.018 | 0.012 | 0.015 |
genus_Lactobacillus | 0.026 | – | 0.026 | – | – | – |
genus_Olsenella | – | – | – | – | – | – |
k Bacteria_p Bacteroidetes_c Bacteroidia_o Bacteroidales | – | – | – | – | – | – |
k Bacteria_p Bacteroidetes_c Bacteroidia_o Bacteroidales_f Rikenellaceae | – | – | – | – | – | – |
k Bacteria_p Firmicutes_c Clostridia | – | – | – | 0.003 | – | – |
k Bacteria_p Firmicutes_c Clostridia_o Clostridiales_f Veillonellaceae_g Megamonas | – | – | – | – | – | – |
k Bacteria-p Bacteroidetes-c Bacteroidia-o Bacteroidales- f Bacteroidaceae g Bacteroides | 0.025 | – | 0.025 | – | 0.011 | – |
k Bacteria-p Firmicutes-c Clostridia-o Clostridiales | 0.001 | 0.027 | 0.001 | – | 0.003 | – |
k Bacteria-p Firmicutes-c Clostridia-o Clostridiales-f Lachnospiraceae | 0.019 | – | 0.019 | – | – | 0.005 |
k Bacteria-p Firmicutes-c Clostridia-o Clostridiales-f Ruminococcaceae | 0.018 | 0.013 | 0.018 | 0.044 | 0.002 | – |
order_Rhizobiales | – | – | – | – | – | – |
Note: body mass index, BMI; waist circumference adjusted for BMI, WAISTadjBMI; waist-to-hip ratio adjusted for BMI, WHRadjBMI; type 2 diabetes, T2D; male fasting glucose and fasting insulin, FAST_male; female fasting glucose and fasting insulin, FAST_female.
Family Lachnospiraceae (empirical P = 0.005) and genus Desulfovibrio (empirical P = 0.015) appeared to be associated with T2D. Female fasting glucose and fasting insulin appeared to be associated with class Clostridia (empirical P = 0.003), family Ruminococcaceae (empirical P = 0.044) and genus Desulfovibrio (empirical P = 0.018). For male fasting glucose and fasting insulin, association signals were observed for family Ruminococcaceae (empirical P = 0.002), order Clostridiales (empirical P = 0.003), genus Bacteroides (empirical P = 0.011) and Desulfovibrio (empirical P = 0.012).
Type I error rates
Using the randomly generated gene set annotation data, we reconducted GSEA of five psychiatric disorders, obesity and T2D using the same analysis approach. As expected, no significant association (all empirical P > 0.05) was observed for five psychiatric disorders, obesity and T2D, indicating the good control of type I error rate of our statistical analysis approach in this study.
Discussion
In this study, we extended the classic GSEA approach to detect the associations between gut microbiota and complex diseases using published GWAS and GWASGM summary data. We applied our approach to real GWAS data sets and detect multiple gut bacteria associated with psychiatric disorders, providing new clues for revealing the roles of gut microbiota in the development of psychiatric disorders. Our study also illustrated the good performance of GSEA approach for exploring the relationships between gut microbiota and complex diseases using GWAS and GWASGM data.
One important finding of this study is genus Desulfovibrio, which appeared to be associated with ADHD, AUT, BD, MDD and SCZ in this study. Desulfovibrio is an anaerobic, gram-negative sulfate-reducing bacillus. Its physiology and metabolism can uniquely account for much of the pathophysiology seen in AUT. For instance, a recent study employing pyrosequencing technique showed that Desulfovibrio species were presented in significantly higher numbers in the stools of severely autistic children than in controls [47]. Tomova et al. [23] reported a significant increase of Desulfovibrio in the fecal microbiota of autistic children at Slovakia. Besides AUT, we also observed associations of Desulfovibrio with ADHD, BD, MDD and SCZ, which have not been reported by now. Given the overlapped etiology and mechanism of various psychiatric disorders [48, 49], it is reasonable to infer that psychiatric disorders may share common risk components at the gut microbiota level, for example Desulfovibrio.
Another interesting finding is phylum Bacteroidetes, which were associated with AUT, MDD and SCZ. It has been reported that the level of Bacteroidetes was strongly increased in the active-MDD and responded-MDD groups compared to healthy control group [24]. Compared to non-depressed control subjects, the fecal microbiota of depression patients had overrepresented Bacteroidales at the order level [50]. Our study results support the implication of order Bacteroidales in the development of MDD and suggest that Bacteroidales also contributed to the development of AUT and SCZ. Additionally, we also identified several novel candidate gut microbiota, such as genus Desulfovibrio for BD and genus Bacteroides for SCZ. Further biological studies are needed to confirm our finding and clarify the potential mechanism of identified gut microbiota implicated in the development of psychiatric disorders.
To evaluate the performance of our analysis approach, we also evaluated the correlations of obesity and T2D with gut microbiota. We identified some gut microbiota significantly associated with obesity and T2D-related phenotypes, such as Bacteroidetes, Firmicutes and Clostridia. By comparing genetically obese mice and their lean littermates, as well as those of obese and lean human volunteers, previous studies have reported that obesity is associated with changes in the relative abundance of the two dominant bacterial divisions, the Bacteroidetes and the Firmicutes [14, 15]. A T2D study showed that the proportion of the Firmicutes and the Clostridia in the gut of patients was significantly reduced [18, 51]. These study results support the accuracy of our analysis approach for detecting disease-related gut microbiota.
Our analysis approach has two advantages. Firstly, to the best of our knowledge, this study represents the 1st effort to explore the genetic relationships between gut microbiota and psychiatric disorders using GWAS and GWASGM data. Our approach only needs GWAS summary data, which are usually available for many complex disease and traits. We hope that our analysis approach provided a novel approach for screening candidate gut microbiota for subsequent functional and mechanism studies. Secondly, because of using DNA level GWAS and GWASGM data, our approach should be more robust to the confounding impact of environmental and dietetic factors, which can affect the composition of gut microbiota in humans.
Notably, two limitations of our approach should also be noted. Firstly, the gut microbiota-related gene sets were collected from previously published GWASGM. Due to the very limited GWASGM performed at different platforms, we can only analyze limited numbers of gut microbiota at different microbiota levels and may miss some gut microbiota, which are truly associated with the diseases analyzed by this study. Given the good performance of detecting disease-related gut microbiota using GWAS and GWASGM summary data, more GWASGM are necessary to define the interactions between host genetics and gut microbiota. Secondly, the GWAS and GWASGM data of this study are from European ancestry. Therefore, it should be careful to apply our study results to other ethnic groups.
In summary, we proposed an approach to evaluate the associations between gut microbiota and complex diseases utilizing publicly available GWAS and GWASGM summary data. We identified multiple gut bacteria showing associations with psychiatric disorders, BMI and T2D. We hope our study results provide useful information for the mechanism studies of gut microbiota implicated in the development of psychiatric disorders.
Funding
National Natural Scientific Foundation of China (81472925 and 81673112); Key Projects of International Cooperation among Governments in Scientific and Technological Innovation (2016YFE0119100); Natural Science Basic Research Plan in Shaanxi Province of China (2017JZ024); Fundamental Research Funds for the Central Universities.
Shiqiang Cheng is currently working as a postgraduate student at the Key Laboratory of Trace Elements and Endemic Diseases of National Health Commission of the People's Republic of China, School of Public Health, Health Science Center, Xi'an Jiaotong University.
Bei Han is currently working as an associate professor at the School of Public Health, Health Science Center, Xi'an Jiaotong University.
Miao Ding is currently working as a postgraduate student at the Key Laboratory of Trace Elements and Endemic Diseases of National Health Commission of the People's Republic of China, School of Public Health, Health Science Center, Xi'an Jiaotong University.
Yan Wen is currently working as a lecturer at the Key Laboratory of Trace Elements and Endemic Diseases of National Health Commission of the People's Republic of China, School of Public Health, Health Science Center, Xi'an Jiaotong University.
Mei Ma is currently working as a postgraduate student at the Key Laboratory of Trace Elements and Endemic Diseases of National Health Commission of the People's Republic of China, School of Public Health, Health Science Center, Xi'an Jiaotong University.
Lu Zhang is currently working as a postgraduate student at the Key Laboratory of Trace Elements and Endemic Diseases of National Health Commission of the People's Republic of China, School of Public Health, Health Science Center, Xi'an Jiaotong University.
Xin Qi is currently working as a postgraduate student at the Key Laboratory of Trace Elements and Endemic Diseases of National Health Commission of the People's Republic of China, School of Public Health, Health Science Center, Xi'an Jiaotong University.
Bolun Cheng is currently working as a doctor student at the Key Laboratory of Trace Elements and Endemic Diseases of National Health Commission of the People's Republic of China, School of Public Health, Health Science Center, Xi'an Jiaotong University.
Ping Li is currently working as a doctor student at the Key Laboratory of Trace Elements and Endemic Diseases of National Health Commission of the People's Republic of China, School of Public Health, Health Science Center, Xi'an Jiaotong University.
Om Prakash Kafle is currently working as a doctor student at the Key Laboratory of Trace Elements and Endemic Diseases of National Health Commission of the People's Republic of China, School of Public Health, Health Science Center, Xi'an Jiaotong University.
Xiao Liang is currently working as a postgraduate student at the Key Laboratory of Trace Elements and Endemic Diseases of National Health Commission of the People's Republic of China, School of Public Health, Health Science Center, Xi'an Jiaotong University.
Li Liu is currently working as a postgraduate student at the Key Laboratory of Trace Elements and Endemic Diseases of National Health Commission of the People's Republic of China, School of Public Health, Health Science Center, Xi'an Jiaotong University.
Yanan Du is currently working as a postgraduate student at the Key Laboratory of Trace Elements and Endemic Diseases of National Health Commission of the People's Republic of China, School of Public Health, Health Science Center, Xi'an Jiaotong University.
Yan Zhao is currently working as a postgraduate student at the Key Laboratory of Trace Elements and Endemic Diseases of National Health Commission of the People's Republic of China, School of Public Health, Health Science Center, Xi'an Jiaotong University.
Feng Zhang is currently working as a professor at the Key Laboratory of Trace Elements and Endemic Diseases of National Health Commission of the People's Republic of China, School of Public Health, Health Science Center, Xi'an Jiaotong University.
We proposed an approach to evaluate the associations between gut microbiota and complex diseases.
We identified multiple gut bacteria showing associations with complex diseases.
Our study results may provide useful information for the mechanism studies of gut microbiota implicated in complex diseases.
References
Author notes
Shiqiang Cheng and Bei Han contribute equally to this manuscript.