-
PDF
- Split View
-
Views
-
Cite
Cite
Tiffany M Chapman, Kelsey L McAlister, Kristen N Moore, Wei-Lin Wang, Britni R Belcher, Screen time and allostatic load among youth: findings from the National Health and Nutrition Examination Survey, 2015-2018, Annals of Behavioral Medicine, Volume 59, Issue 1, 2025, kaaf031, https://doi.org/10.1093/abm/kaaf031
- Share Icon Share
Abstract
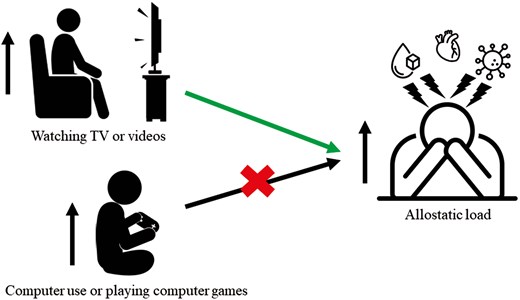
Lay Summary
Screen time (ST) behaviors, such as watching TV, using the computer, or playing video games, are common among youth. ST behaviors are linked to several health concerns, but it not clear how they affect physiological stress. This study examined how different types of ST affected allostatic load (AL), a summary measure of combined physiological stress across several body systems, among US youth aged 12-17 years. We found that more time spent watching TV or videos was associated with higher AL, suggesting that this behavior leads to higher overall stress-related wear and tear on the body. However, computer use or gaming did not show a relationship with AL. We also found that in older adolescents with a lot of TV or video ST, there was more stress in the cardiovascular system. These findings for the first time show that certain ST behaviors can influence physiological stress even in youth. More research is needed to understand the mechanisms beneath these relationships and how we should intervene to promote health and prevent disease.
Background
More screen time (ST) is associated with dysregulation of the individual biological systems (cardiovascular, immune, metabolic, and neuroendocrine) involved in the stress response in youth. However, its relationship with allostatic load (AL), a measure of the cumulative physiological stress response, is unclear in youth.
Purpose
To investigate the associations between ST types and AL outcomes in youth and to explore sociodemographic and behavioral moderators of these relationships.
Methods
Cross-sectional data were from 1053 US youth aged 12-17 years (Mage = 14.20; 54% male; 21% Hispanic) in the 2015-2018 National Health and Nutrition Examination Survey (NHANES). ST was assessed as watching TV/videos and computer use/playing computer games. AL was measured using 7 biomarkers across 3 systems: cardiovascular (systolic and diastolic blood pressure, heart rate), immune (C-reactive protein), and metabolic (body mass index, glycohemoglobin, and high-density lipoprotein). Weighted multivariable regression models assessed whether ST predicted AL composite and subsystem (cardiovascular, immune, and metabolic) scores. Moderation by age, sex, income, race/ethnicity, and physical activity was explored.
Results
A 1-hour/day increase in watching TV/videos was associated with a 4% increase in mean AL composite score (incident rate ratio = 1.040; 95% CI = 1.008, 1.073; P = .015), while computer use/gaming showed no significant associations (P’s > .05). Age moderated the TV/videos-AL cardiovascular association (P = .009), with older youth having higher AL cardiovascular scores.
Conclusions
More time spent watching TV/videos was associated with higher cumulative physiological stress in youth. Prospective studies are needed to determine causal pathways and potential intervention targets in youth.
Introduction
Stress is a risk factor for the leading causes of death in the United States, including type 2 diabetes, heart disease, and cancer.1–11 The physiological stress response, which involves a cascade of effects across multiple biological systems, is triggered during a stressful event to help the body modify or adapt to the stressor.7,12–14 This response has been implicated as a biological mechanism underlying the effect of exposure to stressors on chronic disease in adults.1–11 However, prior studies in youth are limited in that they have conceptualized “stress” as either a psychological construct or have relied on a limited number of biomarkers to capture biological measures of stress.13,15–19 Reliance on a single measure may not capture the whole-body effects of stress as the physiological stress response involves the activation of the hypothalamic-pituitary-adrenal and sympathetic-adrenal-medullary axes, which in turn increases the release of chemical messengers.11,12,20–25 These messengers are known as primary mediators (ie, neuroendocrine biomarkers) of the stress response that, when activated repeatedly over time, can lead to the dysregulation of secondary outcomes (ie, cardiovascular, immune, and metabolic biomarkers).11,12,20–25 This dysregulation can ultimately manifest in tertiary outcomes, such as type 2 diabetes, heart disease, cancer, and mortality.24,26,27 A common method for measuring this cumulative physiological stress response is allostatic load (AL), a sum of biological markers representing cardiovascular, immune, metabolic, and neuroendocrine systems.11,12,22–31 While most of the AL research has been conducted among adults,22,23,26–31 research is increasing in youth.24,25 Allostatic load has been shown to predict numerous poor health outcomes in youth24 and could be used to identify early signs of physiological dysregulation before the clinical manifestation of disease. Thus, understanding modifiable risk factors associated with AL during key developmental periods may prevent or delay poor health outcomes.
Sedentary behavior is a potentially modifiable health behavior that is highly prevalent in US youth.32–35 It is defined as any waking behavior characterized by low energy expenditure (≤1.5 metabolic equivalents) performed in a sitting or reclining position.36 This behavior encompasses a range of activities, including screen-based behaviors such as watching television (TV), using a computer or playing video games. Screen time (ST) is particularly concerning due to its high prevalence; youth spend an average of 6 to 9 hours daily on screen-based activities with the most time spent watching TV/videos, followed by gaming.37 This high level of engagement is concerning because sedentary behavior, particularly ST, is associated with poor cardiometabolic and mental health outcomes, and reduced life expectancy, independent of physical activity participation.17,38–42 Given its high prevalence and established associations with negative health outcomes, ST is a potential modifiable target for interventions to improve youth health.
Emerging evidence suggests that sedentary behavior may contribute to AL in youth. Although the literature on sedentary behavior and AL in youth is limited, studies suggest that more time spent engaging in sedentary behavior is associated with the dysregulation of each of the biological subsystems that comprise AL, including cardiovascular, immune, metabolic, and neuroendocrine systems in youth.34,43–49 However, to date, no prior studies in youth have assessed the relationship between ST and the composite score of AL. Assessing AL provides a comprehensive view of the whole-body effects of stress, which may not be apparent when examining individual biological systems. Thus, assessing this relationship may be an important step for designing targeted behavioral interventions to mitigate the effects of all biological systems impacted by stress rather than addressing each system separately.
Several theoretical and conceptual models suggest that ST may contribute to AL in youth through distinct pathways, impacting multiple physiological systems involved in the stress response. One possible explanation is ST may displace physical activity, reducing energy expenditure and contributing to metabolic and cardiovascular dysregulation, including elevated adiposity and lipid levels, which increase cardiometabolic stress.49–51 Additionally, ST, particularly watching TV, may promote suboptimal health behaviors, such as higher calorie consumption52–54 and disrupted sleep patterns,55–59 further exacerbating immune, metabolic, and neuroendocrine dysregulation. Psychological stressors may also play a role, as ST increases exposure to socially isolating or stress-inducing content, such as cyberbullying and disturbing or negative media, which can dysregulate neuroendocrine pathways, including overactivation of the hypothalamic-pituitary-adrenal axis leading to excess cortisol secretion.19,60,61 Understanding these pathways is critical for identifying modifiable behaviors that contribute to cumulative physiological stress in youth.
The health effects of sedentary behavior in youth vary by type (eg, TV/videos vs computer/video games) and context (eg, discretionary vs nondiscretionary use). Discretionary sedentary behavior, including leisure or recreational behaviors such as watching TV, is consistently associated with adverse physical and mental health outcomes, including body composition, lipid levels, and psychological distress, while nondiscretionary sedentary behavior, including required behaviors such as doing homework, appear to have null or favorable associations.34,41,48,62 Among discretionary activities, watching TV has shown stronger associations with negative health outcomes than other screen-based activities, potentially due to its mentally passive nature, compared to the cognitive engagement required by activities like computer use or gaming.34,41,62 These findings emphasize the importance of distinguishing between types of ST to better understand their differential contributions to stress-related physiological dysregulation, as captured by AL.
Sociodemographic and behavioral factors may also influence the relationship between ST and AL. For example, older youth tend to be more sedentary and have higher AL scores.37,43,63 Although sex differences are mixed, some studies suggest that boys engage in more ST than girls, potentially placing them at greater risk for negative health outcomes.37,43,63 Youth from lower-income families tend to have higher ST and experience higher cumulative stress.24,37 Racial and ethnic disparities have also been observed with non-Hispanic Black and Hispanic youth reporting higher levels of ST and having higher AL scores than their non-Hispanic White peers, likely reflecting systemic inequities and stressors such as discrimination.24,37,63 Finally, engaging in physical activity is negatively associated with ST and AL, suggesting it may mitigate their negative health effects.28,31,64–66 Examining these factors as moderators may help identify at-risk groups and inform strategies to reduce health disparities.
Despite the high prevalence of ST and its potential moderators, the relationship between ST and AL in youth is understudied. This study addresses this gap through 3 objectives. The primary aim of this study was to assess the relationship between ST and AL composite scores among a nationally representative sample of US youth. The secondary aim was to examine the relationship between ST and the relative importance of the individual biological systems that make up AL. The final aim explored whether sociodemographic or behavioral factors, including age, sex, poverty-to-income ratio, race/ethnicity, and physical activity, moderate these relationships. We hypothesized that higher self-reported time spent watching TV/videos and using the computer/playing computer games would be associated with higher objectively measured AL composite scores (H1) and higher objectively measured subsystem-specific AL scores (ie, cardiovascular, immune, and metabolic) (H2).
Methods
The Strengthening the Reporting of Observational Studies in Epidemiology Statement for cross-sectional studies was used to report the study methods and results.67
Participants
Data are from the 2015-2018 National Health and Nutrition Examination Survey (NHANES) cycles. NHANES is a nationally representative biannual cross-sectional study that monitors the health and nutritional status of the noninstitutionalized civilian US population.68 NHANES uses a stratified, 4-stage probability sampling procedure: (1) counties, (2) segments within counties, (3) households within segments, and finally (4) individuals within households.68 The 2015-2018 NHANES surveys oversampled subgroups based on age (under 11 or over 80 years old), income (at or below 185% of the federal poverty level), and race/ethnicity (Hispanic, non-Hispanic Black, and non-Hispanic, non-Black Asian).68 The NHANES data are collected via in-home questionnaires (eg, sociodemographic characteristics), in-person physical examinations (eg, height and weight), and laboratory tests (eg, blood specimens) at a mobile examination center.68 The NHANES protocols were approved by the National Center for Health Statistics Research Ethics Review Board (ERB #2011-17 and #2018-01). Written informed consent was obtained from all participants and their parents/legal guardians.
The 2015-2016 and 2017-2018 NHANES cycles were selected because they included the variables of interest and were the most recent publicly available data that met NHANES recommendations68 of combining adjacent cycles of at least 4 years of data with similar data collection methodology for all relevant variables included in the model. The sample only included youth aged 12-17 years (n = 1950) because blood samples were collected in this age group. To ensure AL scores were not skewed by health conditions, youth who reported a diagnosis of cancer or who were currently taking medications (ie, blood pressure, cholesterol, and/or diabetes) were excluded. Youth missing any of the 7 biomarkers used to measure AL or who had a biomarker that was not biologically plausible (ie, blood pressure of 0 mmHg) were also excluded. The final analytic sample included 1053 youth with complete data for all variables of interest (Supplementary Figure S1 presents missing data and exclusion details).
Measures
Screen time
Screen time was measured using items from the Physical Activity Questionnaire section of NHANES, which were adapted from the World Health Organization’s Global Physical Activity Questionnaire (GPAQ). The GPAQ is a validated tool used for physical activity and sedentary behavior surveillance69 that is administered by trained interviewers. Participants were asked to report, over the past 30 days, the average number of hours per day that were spent (1) sitting and watching TV or videos and (2) using a computer or playing computer games outside of school (eg, PlayStation, Nintendo DS, or other portable video games). Each question had 7 response options ranging from 0 to 5 or more hours/day (eg, 0 hours, less than 1 hour, 1 hour, 2 hours, etc.). Both were analyzed as continuous variables. These items have been used to assess ST in nationally representative samples of youth57,70–74 and are similar to questions used in other surveillance systems like the Youth Risk Behavior Surveillance System.75–77
Allostatic load
Allostatic load was measured using 7 biomarkers representing 3 biological systems: cardiovascular (systolic blood pressure, diastolic blood pressure, and heart rate), immune (C-reactive protein), and metabolic (body mass index, glycohemoglobin, and high-density lipoprotein). These biomarkers were selected because they are frequently used to create AL scores in youth,25 have been used in previous NHANES analyses of AL in youth,25,63,78–81 and were available for our sample in the NHANES dataset thereby allowing for comparison with prior studies.
Table 1 presents the cutoffs of the biomarkers used to create AL scores. Allostatic load was operationalized both as a composite and individual subsystem scores. Using the unweighted sample distribution, each biomarker above the 75th percentile was categorized as high risk (= 1), except high-density lipoprotein, where below the 25th percentile indicates high risk.63,78 The AL composite score was created by summing the number of high-risk biomarkers with higher scores indicating worse physiological functioning (range: 0-7). Additionally, each AL subsystem score (cardiovascular, immune, and metabolic) was created by summing the high-risk biomarkers within the respective system and dichotomizing them as binary variables. Subsystems were categorized as high-risk (= 1) if a participant had at least 1 high-risk biomarker, with possible scores ranging from 0 to 1 for each subsystem.
Quartile-based high-risk cutoffs for each biomarker used to create the allostatic load scores among youth aged 12-17 years who participated in the 2015-2018 National Health and Nutrition Examination Survey (NHANES) (analytic n = 1053).
Biomarkers . | High-risk categorya . |
---|---|
Cardiovascular | |
Systolic blood pressure (mmHg) | ≥114.00 |
Diastolic blood pressure (mmHg) | ≥67.33 |
Heart rate (BPM) | ≥86.00 |
Immune | |
C-reactive protein (mg/L) | ≥1.31 |
Metabolic | |
Body mass index (kg/m2) | ≥26.80 |
Glycohemoglobin (%) | ≥5.50 |
High-density lipoprotein (mg/dL) | ≤44.00 |
Biomarkers . | High-risk categorya . |
---|---|
Cardiovascular | |
Systolic blood pressure (mmHg) | ≥114.00 |
Diastolic blood pressure (mmHg) | ≥67.33 |
Heart rate (BPM) | ≥86.00 |
Immune | |
C-reactive protein (mg/L) | ≥1.31 |
Metabolic | |
Body mass index (kg/m2) | ≥26.80 |
Glycohemoglobin (%) | ≥5.50 |
High-density lipoprotein (mg/dL) | ≤44.00 |
BPM, beats per minute; kg/m2, kilogram per square meter; mg/dL, milligrams per deciliter; mg/L, milligrams per liter; mmHg, millimeters of mercury.
aHigh risk = 75th percentile (except high-density lipoprotein where high risk is the 25th percentile) was determined by the unweighted analytic sample.
Quartile-based high-risk cutoffs for each biomarker used to create the allostatic load scores among youth aged 12-17 years who participated in the 2015-2018 National Health and Nutrition Examination Survey (NHANES) (analytic n = 1053).
Biomarkers . | High-risk categorya . |
---|---|
Cardiovascular | |
Systolic blood pressure (mmHg) | ≥114.00 |
Diastolic blood pressure (mmHg) | ≥67.33 |
Heart rate (BPM) | ≥86.00 |
Immune | |
C-reactive protein (mg/L) | ≥1.31 |
Metabolic | |
Body mass index (kg/m2) | ≥26.80 |
Glycohemoglobin (%) | ≥5.50 |
High-density lipoprotein (mg/dL) | ≤44.00 |
Biomarkers . | High-risk categorya . |
---|---|
Cardiovascular | |
Systolic blood pressure (mmHg) | ≥114.00 |
Diastolic blood pressure (mmHg) | ≥67.33 |
Heart rate (BPM) | ≥86.00 |
Immune | |
C-reactive protein (mg/L) | ≥1.31 |
Metabolic | |
Body mass index (kg/m2) | ≥26.80 |
Glycohemoglobin (%) | ≥5.50 |
High-density lipoprotein (mg/dL) | ≤44.00 |
BPM, beats per minute; kg/m2, kilogram per square meter; mg/dL, milligrams per deciliter; mg/L, milligrams per liter; mmHg, millimeters of mercury.
aHigh risk = 75th percentile (except high-density lipoprotein where high risk is the 25th percentile) was determined by the unweighted analytic sample.
Covariates and moderators
A priori covariates included age (continuous; years), biological sex (dichotomous; male and female), race/ethnicity (categorical; Hispanic, non-Hispanic Asian, non-Hispanic Black, non-Hispanic White, and non-Hispanic other race/multiracial), parent’s education (categorical; less than high school degree, high school degree/some college, and college graduate or above), poverty-to-income ratio (continuous; ratio of family income to the US poverty threshold) and physical activity (continuous; number of days/week).24,28,31,37,43,63–66 For the physical activity question, participants were asked to report over the past 7 days, the average number of days per week spent being physically active for at least 60 minutes. This question had 7 response options ranging from 0 to 7 days/week.
Moderators included age (continuous), biological sex (dichotomous), race/ethnicity (dichotomous; non-Hispanic White and other), poverty-to-income ratio (continuous), and physical activity (dichotomous; meets and does not meet guidelines). The US Physical Activity Guidelines recommend that youth ages 6–17 years engage in at least 60 minutes of physical activity daily.82 Participants who reported engaging in at least 60 minutes of physical activity 7 days/week were classified as meeting physical activity guidelines.
Statistical analysis
For descriptive statistics, frequencies, percentages, means, standard deviations, and standard errors were calculated for the weighted and unweighted samples. Due to the AL composite score being an overdispersed count variable and the AL subsystem scores being high-prevalence (>10%) dichotomous variables, negative binomial regression was used to calculate incident rate ratio (IRR) and Poisson regression with robust standard errors was used to calculate adjusted prevalence ratio (APR), respectively. To assess aim 1, weighted negative binomial regression models were used to investigate the relationship between time spent watching TV/videos, computer use/gaming, and AL composite score after adjusting for covariates. To assess aim 2, separate Poisson regression models with robust standard errors were used to examine the relationship between time spent watching TV/videos, computer use/gaming, and each AL subsystem (ie, cardiovascular, immune, and metabolic) score after adjusting for covariates. To assess aim 3, interaction models were used to explore the moderating effects of sociodemographic and behavioral factors on the relationship between ST and AL outcomes. An interaction term was added to each regression model (eg, TV/videos × age, computer use/gaming × age, TV/videos × sex, computer use/gaming × sex, etc.) to test for moderation. All analyses were conducted in Stata version 18.0 with a significance level set at P < .05 and all regression estimates were weighted using sample weights and variance units to account for the NHANES complex survey design.
Results
Descriptive statistics
There were no statistically significant differences in biological sex, poverty-to-income ratio, or physical activity between the unweighted full and analytical samples (Supplementary Table S1 presents differences between excluded and included participants). However, these samples differed by age, race/ethnicity, and parent’s education. Those excluded were, on average, older than those included in the final analytic sample (14.83 ± 1.78 vs. 14.15 ± 1.57 years, P < .001). Also, a higher proportion of those excluded identified as non-Hispanic Asian (13% vs 10%, P = .04) and had parents with less than a high school degree (27% vs. 20%, P < .001). Additionally, a lower proportion of those excluded identified as non-Hispanic White (25% vs. 33%, P < .001) and had parents with a high school degree/some college (51% vs. 59%, P < .001). There were no statistically significant differences in the proportion of those identifying as Hispanic, non-Hispanic Black, or non-Hispanic other race/multiracial, nor in the proportion of those whose parents were college graduates or higher.
Table 2 presents sociodemographic, activity pattern, and AL characteristics in the unweighted and weighted samples. The unweighted sample (n = 1053) had a mean age of 14.15 years (SD = 1.57; range: 12-17) with 53% identifying as male and 46% identifying as non-Hispanic White. Similarly, the weighted sample (n = 15 425 985) had a mean age of 14.20 years (SE = 0.07) with the largest proportion of the sample identifying as male (54%) and non-Hispanic White (71%). Additionally, the weighted sample reported an average of 3.30 (SE = 0.09) and 3.29 (SE = 0.10) hours/day of watching TV/videos and computer use/gaming, respectively. The mean (SE) AL score for the weighted sample was 1.77 (0.07; range: 0-7) with a majority (87%) having an AL score of 3 or lower (Supplementary Figure S2 presents the percentage distribution of AL score). Additionally, 59%, 51%, and 24% of youth had at least 1 high-risk cardiovascular, metabolic, and immune biomarker, respectively.
Descriptive statistics of youth aged 12-17 years who participated in the 2015-2018 National Health and Nutrition Examination Survey (NHANES) (analytic n = 1053).
Variables . | Unweighted samplea F (%) or M (SD) . | Weighted sampleb % or M (SE) . |
---|---|---|
Sociodemographic characteristics | ||
Age (years; range: 12-17) | 14.15 (1.57) | 14.20 (0.07) |
Biological sex (male) | 555 (52.71) | 53.78 |
Poverty-to-income ratio (range: 0-5) | 2.15 (1.48) | 2.56 (0.09) |
Parent’s education | ||
Less than HS degree | 212 (20.13) | 16.74 |
HS degree/GED or some college/AA degree | 625 (59.35) | 57.55 |
College graduate or above | 216 (20.51) | 25.70 |
Ethnicity (Hispanic) | 298 (28.30) | 21.32 |
Race | ||
Non-Hispanic White | 345 (45.70) | 71.00 |
Non-Hispanic Black | 224 (29.67) | 15.44 |
Non-Hispanic Asian | 101 (13.38) | 5.21 |
Non-Hispanic other race or multiracial | 85 (11.26) | 8.23 |
Activity patterns | ||
Physical activity (at least 60 minutes 7 days/week) | 183 (17.38) | 17.76 |
Screen time | ||
TV/video (hours/day) | 3.35 (1.75) | 3.30 (0.09) |
Computer/gaming (hours/day) | 3.29 (2.05) | 3.29 (0.10) |
Allostatic load | ||
Composite (range: 0-7) | 1.85 (1.49) | 1.77 (0.07) |
Subsystems | ||
Cardiovascular (≥1 high-risk biomarker) | 607 (57.64) | 58.68 |
Metabolic (≥1 high-risk biomarker) | 581 (55.18) | 51.28 |
Immune (≥1 high-risk biomarker) | 264 (25.07) | 23.89 |
Variables . | Unweighted samplea F (%) or M (SD) . | Weighted sampleb % or M (SE) . |
---|---|---|
Sociodemographic characteristics | ||
Age (years; range: 12-17) | 14.15 (1.57) | 14.20 (0.07) |
Biological sex (male) | 555 (52.71) | 53.78 |
Poverty-to-income ratio (range: 0-5) | 2.15 (1.48) | 2.56 (0.09) |
Parent’s education | ||
Less than HS degree | 212 (20.13) | 16.74 |
HS degree/GED or some college/AA degree | 625 (59.35) | 57.55 |
College graduate or above | 216 (20.51) | 25.70 |
Ethnicity (Hispanic) | 298 (28.30) | 21.32 |
Race | ||
Non-Hispanic White | 345 (45.70) | 71.00 |
Non-Hispanic Black | 224 (29.67) | 15.44 |
Non-Hispanic Asian | 101 (13.38) | 5.21 |
Non-Hispanic other race or multiracial | 85 (11.26) | 8.23 |
Activity patterns | ||
Physical activity (at least 60 minutes 7 days/week) | 183 (17.38) | 17.76 |
Screen time | ||
TV/video (hours/day) | 3.35 (1.75) | 3.30 (0.09) |
Computer/gaming (hours/day) | 3.29 (2.05) | 3.29 (0.10) |
Allostatic load | ||
Composite (range: 0-7) | 1.85 (1.49) | 1.77 (0.07) |
Subsystems | ||
Cardiovascular (≥1 high-risk biomarker) | 607 (57.64) | 58.68 |
Metabolic (≥1 high-risk biomarker) | 581 (55.18) | 51.28 |
Immune (≥1 high-risk biomarker) | 264 (25.07) | 23.89 |
AA, associate in arts; F, frequency; GED, general education development; HS, high school; M, mean; SD, standard deviation; SE, standard error; TV, television.
an = 1053.
bn = 15 425 985.
Descriptive statistics of youth aged 12-17 years who participated in the 2015-2018 National Health and Nutrition Examination Survey (NHANES) (analytic n = 1053).
Variables . | Unweighted samplea F (%) or M (SD) . | Weighted sampleb % or M (SE) . |
---|---|---|
Sociodemographic characteristics | ||
Age (years; range: 12-17) | 14.15 (1.57) | 14.20 (0.07) |
Biological sex (male) | 555 (52.71) | 53.78 |
Poverty-to-income ratio (range: 0-5) | 2.15 (1.48) | 2.56 (0.09) |
Parent’s education | ||
Less than HS degree | 212 (20.13) | 16.74 |
HS degree/GED or some college/AA degree | 625 (59.35) | 57.55 |
College graduate or above | 216 (20.51) | 25.70 |
Ethnicity (Hispanic) | 298 (28.30) | 21.32 |
Race | ||
Non-Hispanic White | 345 (45.70) | 71.00 |
Non-Hispanic Black | 224 (29.67) | 15.44 |
Non-Hispanic Asian | 101 (13.38) | 5.21 |
Non-Hispanic other race or multiracial | 85 (11.26) | 8.23 |
Activity patterns | ||
Physical activity (at least 60 minutes 7 days/week) | 183 (17.38) | 17.76 |
Screen time | ||
TV/video (hours/day) | 3.35 (1.75) | 3.30 (0.09) |
Computer/gaming (hours/day) | 3.29 (2.05) | 3.29 (0.10) |
Allostatic load | ||
Composite (range: 0-7) | 1.85 (1.49) | 1.77 (0.07) |
Subsystems | ||
Cardiovascular (≥1 high-risk biomarker) | 607 (57.64) | 58.68 |
Metabolic (≥1 high-risk biomarker) | 581 (55.18) | 51.28 |
Immune (≥1 high-risk biomarker) | 264 (25.07) | 23.89 |
Variables . | Unweighted samplea F (%) or M (SD) . | Weighted sampleb % or M (SE) . |
---|---|---|
Sociodemographic characteristics | ||
Age (years; range: 12-17) | 14.15 (1.57) | 14.20 (0.07) |
Biological sex (male) | 555 (52.71) | 53.78 |
Poverty-to-income ratio (range: 0-5) | 2.15 (1.48) | 2.56 (0.09) |
Parent’s education | ||
Less than HS degree | 212 (20.13) | 16.74 |
HS degree/GED or some college/AA degree | 625 (59.35) | 57.55 |
College graduate or above | 216 (20.51) | 25.70 |
Ethnicity (Hispanic) | 298 (28.30) | 21.32 |
Race | ||
Non-Hispanic White | 345 (45.70) | 71.00 |
Non-Hispanic Black | 224 (29.67) | 15.44 |
Non-Hispanic Asian | 101 (13.38) | 5.21 |
Non-Hispanic other race or multiracial | 85 (11.26) | 8.23 |
Activity patterns | ||
Physical activity (at least 60 minutes 7 days/week) | 183 (17.38) | 17.76 |
Screen time | ||
TV/video (hours/day) | 3.35 (1.75) | 3.30 (0.09) |
Computer/gaming (hours/day) | 3.29 (2.05) | 3.29 (0.10) |
Allostatic load | ||
Composite (range: 0-7) | 1.85 (1.49) | 1.77 (0.07) |
Subsystems | ||
Cardiovascular (≥1 high-risk biomarker) | 607 (57.64) | 58.68 |
Metabolic (≥1 high-risk biomarker) | 581 (55.18) | 51.28 |
Immune (≥1 high-risk biomarker) | 264 (25.07) | 23.89 |
AA, associate in arts; F, frequency; GED, general education development; HS, high school; M, mean; SD, standard deviation; SE, standard error; TV, television.
an = 1053.
bn = 15 425 985.
Screen time and allostatic load
Table 3 presents the association between time spent watching TV/videos, computer use/gaming, and AL composite and subsystem scores using weighted regression models. There was a significant association between time spent watching TV/videos and AL composite score. A 1-hour/day increase in sitting and watching TV/videos was associated with a 4% increase in mean AL score, after adjusting for age, sex, race/ethnicity, poverty-to-income ratio, parent’s education, physical activity, and computer use/gaming (IRR = 1.040; 95% CI: 1.008, 1.073; P = .015). However, there were no statistically significant associations between watching TV/videos and the cardiovascular (APR = 1.009; 95% CI: 0.973, 1.046), immune (APR = 1.059; 95% CI: 0.976, 1.149), and metabolic (APR = 1.025; 95% CI: 0.972, 1.080) subsystems after adjusting for covariates. Additionally, there were no statistically significant associations between computer use/gaming, AL composite (IRR = 0.990; 95% CI: 0.962, 1.018), or individual subsystem (P’s > .05) scores after adjusting for covariates.
Weighted regression models assessing the association between screen time, allostatic load composite, and allostatic load subsystems among youth aged 12-17 years (National Health and Nutrition Examination Survey, 2015-2018).
Screen time . | Model 1a,b . | Model 2a,c . | ||
---|---|---|---|---|
AL composite . | AL cardiovascular . | AL immune . | AL metabolic . | |
IRR (95% CI) . | APR (95% CI) . | APR (95% CI) . | APR (95% CI) . | |
TV/video (hours/day) | 1.040 (1.008, 1.073)d | 1.009 (0.973, 1.046) | 1.059 (0.976, 1.149) | 1.025 (0.972, 1.080) |
Computer/gaming (hours/day) | 0.990 (0.962, 1.018) | 1.007 (0.964, 1.052) | 0.968 (0.915, 1.023) | 0.999 (0.963, 1.036) |
Screen time . | Model 1a,b . | Model 2a,c . | ||
---|---|---|---|---|
AL composite . | AL cardiovascular . | AL immune . | AL metabolic . | |
IRR (95% CI) . | APR (95% CI) . | APR (95% CI) . | APR (95% CI) . | |
TV/video (hours/day) | 1.040 (1.008, 1.073)d | 1.009 (0.973, 1.046) | 1.059 (0.976, 1.149) | 1.025 (0.972, 1.080) |
Computer/gaming (hours/day) | 0.990 (0.962, 1.018) | 1.007 (0.964, 1.052) | 0.968 (0.915, 1.023) | 0.999 (0.963, 1.036) |
AL, allostatic load; APR, adjusted prevalence ratio; CI, confidence interval; IRR, incidence rate ratio; TV, television.
aAll models: Adjusted for age, sex, race/ethnicity, poverty-to-income ratio, parent’s education, and physical activity.
bModel 1: Negative binomial regression.
cModel 2: Separate Poisson regression models with robust standard errors.
dP < .05.
Weighted regression models assessing the association between screen time, allostatic load composite, and allostatic load subsystems among youth aged 12-17 years (National Health and Nutrition Examination Survey, 2015-2018).
Screen time . | Model 1a,b . | Model 2a,c . | ||
---|---|---|---|---|
AL composite . | AL cardiovascular . | AL immune . | AL metabolic . | |
IRR (95% CI) . | APR (95% CI) . | APR (95% CI) . | APR (95% CI) . | |
TV/video (hours/day) | 1.040 (1.008, 1.073)d | 1.009 (0.973, 1.046) | 1.059 (0.976, 1.149) | 1.025 (0.972, 1.080) |
Computer/gaming (hours/day) | 0.990 (0.962, 1.018) | 1.007 (0.964, 1.052) | 0.968 (0.915, 1.023) | 0.999 (0.963, 1.036) |
Screen time . | Model 1a,b . | Model 2a,c . | ||
---|---|---|---|---|
AL composite . | AL cardiovascular . | AL immune . | AL metabolic . | |
IRR (95% CI) . | APR (95% CI) . | APR (95% CI) . | APR (95% CI) . | |
TV/video (hours/day) | 1.040 (1.008, 1.073)d | 1.009 (0.973, 1.046) | 1.059 (0.976, 1.149) | 1.025 (0.972, 1.080) |
Computer/gaming (hours/day) | 0.990 (0.962, 1.018) | 1.007 (0.964, 1.052) | 0.968 (0.915, 1.023) | 0.999 (0.963, 1.036) |
AL, allostatic load; APR, adjusted prevalence ratio; CI, confidence interval; IRR, incidence rate ratio; TV, television.
aAll models: Adjusted for age, sex, race/ethnicity, poverty-to-income ratio, parent’s education, and physical activity.
bModel 1: Negative binomial regression.
cModel 2: Separate Poisson regression models with robust standard errors.
dP < .05.
Moderating effects
Moderation analyses examined interactions between ST and sociodemographic and behavioral factors on AL outcomes. For AL composite scores, a significant interaction was found between computer use/gaming and sex (P = .025); however, stratified analyses showed no significant association within either males or females. No other significant interactions were observed for TV/videos or computer use/gaming with sociodemographic or behavioral factors (P’s > .05). For cardiovascular AL scores, significant interactions were found between TV/videos and age (P = .009), and between computer use/gaming and race/ethnicity (P = .025). Among older youth, every 1-hour/day increase in watching TV/videos was associated with higher cardiovascular AL scores compared to younger youth. However, stratified analyses for the interaction with race/ethnicity revealed no significant associations between computer use/gaming and cardiovascular AL scores by racial/ethnic group. There was no additional significant moderation observed with other factors (P’s > .05). For immune and metabolic AL scores, no significant interactions were observed for TV/videos or computer use/gaming with any sociodemographic or behavioral factors (P’s > .05).
Discussion
The primary aim of this study was to assess the relationship between ST and AL composite score, measured as a sum of 7 biomarkers representing 3 biological systems, in a nationally representative sample of US youth. The secondary aim examined the relationship between ST and the relative importance of the individual biological subsystems that make up AL (ie, cardiovascular, immune, and metabolic). The final aim explored whether sociodemographic or behavioral factors moderated these relationships. We found more time spent watching TV/videos, but not computer use/gaming, was associated with higher AL composite scores. Our results also showed neither time spent watching TV/videos nor computer use/gaming was significantly associated with any individual AL subsystem scores. Finally, moderation analyses showed that the association between watching TV/videos and cardiovascular AL was stronger among older youth. To our knowledge, this study is the first to provide preliminary evidence that AL is associated with sedentary behavior, specifically time spent watching TV/videos, and not computer use/gaming.
Similar to studies in adults that show more time spent engaging in sedentary behavior or physical inactivity is associated with higher AL scores,28,29,31 we found that youth who reported spending more time watching TV/videos had higher AL composite scores. This congruency could be explained by previous studies showing that time spent watching TV tracks from childhood and adolescence into adulthood83 and AL increases with age.31,63,84–86 This modest association (a 4% increase/hour/day) provides preliminary data on the association between TV/videos and AL in youth. Although the public health implications and clinical significance of this finding are limited, future studies should consider the widespread prevalence of watching TV/videos among youth33,37 and the cumulative impact of even small physiological changes over time could contribute to adverse health outcomes.24,26,27 Since AL is a cumulative measure of stress-induced physiological dysregulation,11,22,87 its increase over time may result in the early onset of chronic diseases.24,26,27 Prospective research in youth is needed to confirm these associations, explore how different types of ST influence health trajectories, and determine whether ST directly contributes to the physiological consequences of stress.
Despite reporting similar amounts of time spent watching TV/videos and using the computer/playing computer games, only watching TV/videos were significantly associated with AL composite scores. One explanation for this finding could be the differences in the cognitive demands of these activities. Watching TV/videos is often a mentally passive activity, whereas using the computer/playing computer games, typically requires more active engagement. Recent studies have shown that mentally passive, but not mentally active, sedentary behavior is associated with worse health outcomes.62,88–90 Future prospective studies should consider assessing whether the type of ST matters and if targeting specific types, such as watching TV/videos, may be a more effective strategy for interventions aimed at reducing AL and associated health risks.
Contrary to previous findings in youth, we did not find associations between ST and each AL subsystem. Prior studies show that sedentary behavior (typically assessed self-reported TV time) is associated with the cardiovascular, immune, and metabolic systems,34,43,46–49 however, we did not see this in our study. These contradictory findings may be due to how we conceptualized AL. We conceptualized AL as a latent construct representing stress-induced systemic physiological dysregulation as a sum of biological markers across multiple biological systems.12,22–31 A recent study found that AL may have a unidimensional factor structure in youth.91 After testing 5 hypothesized AL factor structures (ie, unidimensional factor, second order 2-, 3-, and 5-subfactor, and 5 correlated factors structures) among a representative sample of US youth, this study found a unidimensional model was the best measurement of AL.91 Allostatic load may need to be measured as a composite or total score rather than as separate biological subsystems in youth, as these biomarkers may have a common shared variance in younger populations. Thus, each biomarker comprising AL may directly load onto or is directly influenced by the construct of AL. In contrast to these findings, prior studies in adults provide support for a second-order factor structure92–94 in which each biomarker loads onto a specific biological system and then each biological system loads onto the AL construct. Thus, how we conceptualized AL may have contributed to our findings that time spent watching TV/videos was associated with AL measured as a composite and not each individual AL subsystem.
Finally, our moderation analyses showed the association between watching TV/videos and cardiovascular AL was stronger among older youth. There is evidence that adolescence is a critical period for cardiovascular development and that sedentary behaviors during this time may amplify age-related vulnerabilities.95 However, additional research is needed to determine if this subgroup is particularly vulnerable to the cardiovascular effects of ST. Although significant interactions were also observed between computer use/gaming and sex for AL composite scores, and between computer use/gaming and race/ethnicity for cardiovascular AL scores, stratified analyses revealed no significant associations within specific subgroups. This may suggest that these effects may be subtle or context-dependent. Future research should explore moderators to provide greater insight into the pathways linking ST with cumulative physiological stress. Larger and more diverse samples are necessary to better understand subgroup-specific vulnerabilities and inform targeted interventions aimed at mitigating the potential health risks of sedentary behaviors in youth.
Limitations
Study strengths included the investigation of whether ST and AL are associated in younger populations using multiple biomarkers to capture physiological stress in a large representative sample of US youth. However, there were some limitations. First, NHANES data are cross-sectional, thus we were unable to assess the direction of the ST-AL relationship. There is a possible bidirectional or reciprocal association as some studies suggest sedentary behavior is a stress-related coping mechanism in youth.96–98 Youth with higher AL may be more likely to engage in sedentary activities, such as watching TV, to manage stress. Future longitudinal studies are needed to establish temporality. Second, neuroendocrine biomarkers, which is a biological system included to measure AL, were not assessed. The exclusion of the neuroendocrine system may have physiological and/or theoretical implications as neuroendocrine biomarkers (eg, cortisol or dehydroepiandrosterone) are on the causal pathway between chronic stress and the development of high AL. Thus, we were unable to assess the relationship between ST and the neuroendocrine subsystem, which may be a driving factor in this relationship in youth as they are generally healthy and the neuroendocrine subsystem is the primary mediator of the stress response.11,12,20–25 However, numerous studies have used the same subsystems used in this analysis to measure AL22–25,30 and have shown this method is valid for predicting poor health outcomes. Future studies should assess the longitudinal relationship between ST and AL, including neuroendocrine biomarkers, to establish the temporality of this relationship and optimize the measurement of AL in youth. Third, the study findings may not be generalizable to all US youth, because compared to our final analytic sample, those excluded due to having missing data were slightly older with a lower proportion identifying as non-Hispanic White and having parents with a high school degree/some college. Fourth, self-reported ST may be susceptible to desirability or recall bias leading to underreporting and potentially influencing the strength of relationships. Furthermore, ST was measured as TV/video and computer use/gaming which does not encompass all types of sedentary behavior (eg, homework, reading, and transportation) that may have an association will AL. Additionally, the use of a 30-day recall to assess ST may have introduced measurement error, as youth may have difficulty recalling their behavior over such a long time frame. Although the ST items in NHANES were adapted from validated surveillance tools69 and administered by trained interviewers, they have not been validated against objective measures, such as accelerometry. This may partially explain the null findings for individual AL subsystems. Another limitation is that NHANES did not distinguish between discretionary (leisure) and nondiscretionary (academic) ST for TV/video viewing. While computer use/gaming was explicitly categorized as nonschool-related, the inability to separate academic and leisure screen use for watching TV limited our ability to assess context-specific effects. Finally, we were unable to control for sleep or sleep-related disturbances, which have been shown to increase stress reactivity56 as the data were not available for our study population.
Conclusion
This study is the first to provide preliminary evidence of an association between ST, specifically watching TV/videos, and AL in youth. These findings underscore the need for future research to confirm these associations by examining the relationship between specific ST types and AL, exploring potential moderators, investigating longitudinal mechanisms linking ST with AL, and assessing whether reducing or replacing TV time impacts the cumulative physiological consequences of stress.
Acknowledgments
The authors thank the participants and staff of the National Health and Nutrition Examination Survey (NHANES).
Author contributions
Tiffany M. Chapman (Conceptualization: Lead; Formal analysis: Lead; Methodology: Lead; Writing – original draft: Lead; Writing – review & editing: Lead), Kelsey L. McAlister (Formal analysis: Supporting; Writing – review & editing: Supporting), Kristen N. Moore (Formal analysis: Supporting; Writing – original draft: Supporting), Wei-Lin Wang (Formal analysis: Supporting; Writing – review & editing: Supporting), and Britni R. Belcher (Formal analysis: Supporting; Methodology: Supporting; Supervision: Lead; Writing – review & editing: Supporting)
Funding
None declared.
Conflict of interest
None declared.
Transparency statements
Study registration: This study was not formally registered.
Analytic plan pre-registration: The analysis plan was not formally pre-registered.
Data availability: This study used de-identified, publicly available data from the National Health and Nutrition Examination Survey(NHANES): https://wwwn.cdc.gov/nchs/nhanes/Default.aspx
Analytic code availability: There is no analytic code associated with this study.
Materials availability: This study used publicly available materials from the NHANES: https://wwwn.cdc.gov/nchs/nhanes/Default.aspx
References
Rideout V, Peebles A, Mann S, Robb MB. Common Sense census: Media use by tweens and teens, 2021. San Francisco, CA: Common Sense Media; 2022.