-
PDF
- Split View
-
Views
-
Cite
Cite
Zhongshuai Tian, Tao Hu, Edward C Holmes, Jingkai Ji, Weifeng Shi, Analysis of the genetic diversity in RNA-directed RNA polymerase sequences: implications for an automated RNA virus classification system, Virus Evolution, Volume 10, Issue 1, 2024, veae059, https://doi.org/10.1093/ve/veae059
- Share Icon Share
Abstract
RNA viruses are characterized by a broad host range and high levels of genetic diversity. Despite a recent expansion in the known virosphere following metagenomic sequencing, our knowledge of the species rank genetic diversity of RNA viruses, and how often they are misassigned and misclassified, is limited. We performed a clustering analysis of 7801 RNA-directed RNA polymerase (RdRp) sequences representing 1897 established RNA virus species. From this, we identified substantial genetic divergence within some virus species and inconsistency in RNA virus assignment between the GenBank database and The International Committee on Taxonomy of Viruses (ICTV). In particular, 27.57% virus species comprised multiple virus operational taxonomic units (vOTUs), including Alphainfluenzavirus influenzae, Mammarenavirus lassaense, Apple stem pitting virus, and Rotavirus A, with each having over 100 vOTUs. In addition, the distribution of average amino acid identity between vOTUs within single assigned species showed a relatively low threshold: <90% and sometimes <50%. However, when only exemplar sequences from virus species were analyzed, 1889 of the ICTV-designated RNA virus species (99.58%) were clustered into a single vOTU. Clustering of the RdRp sequences from different virus species also revealed that 17 vOTUs contained two distinct virus species. These potential misassignments were confirmed by phylogenetic analysis. A further analysis of average nucleotide identity (ANI) values ranging from 70% to 97.5% revealed that at an ANI of 82.5%, 1559 (82.18%) of the 1897 virus species could be correctly clustered into one single vOTU. However, at ANI values >82.5%, an increasing number of species were clustered into two or more vOTUs. In sum, we have identified some inconsistency and misassignment of the RNA virus species based on the analysis of RdRp sequences alone, which has important implications for the development of an automated RNA virus classification system.
1. Introduction
RNA viruses comprise a highly diverse group of infectious agents that are commonly found in eukaryotes and bacteria. They are able to rapidly evolve and adapt to new environments, which ultimately leads to infectious disease outbreaks and epidemics that have posed major challenges to human, animal, and plant health worldwide (Göertz et al. 2018, Nicastri et al. 2019, Hu et al. 2021).
RNA viruses are broadly classified according to their genome structure. They are divided into those with double-stranded RNA (dsRNA) and single-stranded RNA (ssRNA) genomes. The ssRNA viruses can be further grouped into those with genomes in positive-sense RNA (+ssRNA) or negative-sense RNA (−ssRNA) orientations. RNA viruses generate high levels of genetic variability through a combination of high mutation rates resulting from error-prone polymerases, large population sizes, rapid replication kinetics, and sometimes recombination and/or reassortment (Moya et al. 2004). This combination of processes means that the evolutionary (i.e. nucleotide substitution) rate of the RNA viruses is ∼104 to 106 times greater than that of the cellular organisms (Duffy et al. 2008, Sanjuán et al. 2010). The genome length of the RNA viruses also varies substantially, from ∼1.7 kb (Huang and Lo 2010) to 47.3 kb (Hou et al. 2023). Additionally, RNA virus particles exhibit remarkable diversity in terms of shape and size, with some displaying icosahedral or complex symmetries, while others adopt filamentous, rectangular, or bullet-shaped nucleocapsid structures (Fermin 2018).
Despite this variability, nearly all the members of the viral kingdom Orthornavirae depend on the activity of a virus-encoded RNA-directed RNA polymerase (RdRp) for the condensation of nucleotide triphosphates. The structure of RdRp resembles a cupped right hand, comprising three subdomains: thumb, palm, and fingers (te Velthuis 2014, Venkataraman et al. 2018). Within these subdomains, seven conserved motifs—denoted G, F1–3, A, B, C, D, and E—are arranged in an amino-terminal and carboxyl-terminal order (Gorbalenya et al. 2002, Bruenn 2003). Of particular significance, motifs A, B, and C are located in the palm subdomain and together form the catalytic core of RdRp, such that they are highly conserved (te Velthuis 2014, Charon et al. 2022). As a consequence of this conservation, which is greater than that seen in other RNA virus proteins, the RdRp domain is widely used for the large-scale phylogenetic analysis and classification of RNA viruses (Shi et al. 2016, 2018, Harvey and Holmes 2022).
As noted earlier, genetic diversity in RNA viruses primarily arises through high rates of mutation (Bordería et al. 2011, Waman et al. 2014, Stenglein et al. 2015), itself resulting from low fidelity of replication by the RdRp (Duffy et al. 2008). Importantly, that the extent of genetic diversity varies within different RNA virus species poses major challenges for understanding the fundamental mechanisms of RNA virus evolution as well as for accurately classifying RNA viruses (Wu et al. 2010, Groseth et al. 2017, Wang et al. 2019).
Herein, we sought to determine how the levels of genetic diversity vary among RNA virus species and how this might impact virus classification. Through a comprehensive analysis of the genetic diversity of RdRps, we determined how often RNA virus species officially ratified by The International Committee on Taxonomy of Viruses (ICTV) (i) clustered into multiple rather than single virus operational taxonomic units (vOTUs) and (ii) different virus species were grouped into the same vOTU. In doing so, we found inconsistency and misassignment in the nomenclature of RNA viruses that warrant clarification and correction.
2. Materials and methods
2.1 Retrieval and identification of viral RdRps
We downloaded all virus sequences (GenBank flat files) available from the National Center for Biotechnology Information (NCBI) GenBank (https://ftp.ncbi.nlm.nih.gov/genbank/; Release Number 249.0) (Sayers et al. 2019). The Python package ete3 (Huerta-Cepas et al. 2016) was used to screen the kingdom Orthornavirae, which includes the RNA viruses encoding an RdRp. We then used the Biopython SeqIO package (Cock et al. 2009) to parse the GenBank files to obtain the protein sequences from the coding sequences feature annotation. RdRp protein sequences were identified based on homology searches using the profile hidden Markov model (pHMM) of virus RdRp domains. To increase the detection of divergent RdRp protein sequences (te Velthuis 2014), pHMMs were generated and updated through two iterations. In the first iteration, we downloaded all the RdRp pHMMs from Pfam and used hmmsearch (HMMER v3.1b2) (Potter et al. 2018) to search for homologous sequences with an e-value cut-off of 1e-5 (Table 1). Only sequences longer than 300 amino acids were retained. In the second iteration, the RdRp sequences obtained from the first iteration were grouped at the rank of virus order based on the Virus Metadata Resource (VMR, VMR_MSL38_v3.xlsx) released by the ICTV. Every RdRp group was aligned using Clustal Omega (v1.2.4) (--auto option) (Sievers et al. 2011), and hmmbuild (HMMER v3.1b2) was then used to construct new pHMMs. Using this procedure, we identified a total of 470 637 putative RdRps (Fig. 1).
Pfam ID . | Name . | Model length . | Family (HMM) version . |
---|---|---|---|
PF06317 | Arenavirus RNA polymerase | 2048 | 14 |
PF00603 | Influenza RNA-dependent RNA polymerase subunit PA | 694 | 20 |
PF00604 | Influenza RNA-dependent RNA polymerase subunit PB2 | 754 | 20 |
PF05919 | Mitovirus RNA-dependent RNA polymerase | 498 | 14 |
PF00602 | Influenza RNA-dependent RNA polymerase subunit PB1 | 732 | 20 |
PF07925 | Reovirus RNA-dependent RNA polymerase lambda 3 | 1271 | 14 |
PF00998 | Viral RNA-dependent RNA polymerase | 486 | 26 |
PF00978 | RNA-dependent RNA polymerase | 440 | 24 |
PF04196 | Bunyavirus RNA-dependent RNA polymerase | 742 | 15 |
PF04197 | Birnavirus RNA-dependent RNA polymerase (VP1), palm domain | 531 | 15 |
PF00946 | Mononegavirales RNA-dependent RNA polymerase | 1068 | 22 |
PF00680 | Viral RNA-dependent RNA polymerase | 462 | 23 |
PF02123 | Viral RNA-directed RNA polymerase | 479 | 19 |
PF05788 | Orbivirus RNA-dependent RNA polymerase (VP1) | 1297 | 15 |
PF00972 | Flavivirus RdRp, fingers and palm domains | 451 | 23 |
PF08467 | Luteovirus RNA polymerase P1-P2/replicase | 339 | 13 |
PF06478 | Coronavirus RNA-dependent RNA polymerase, N-terminal | 350 | 16 |
PF03431 | RNA replicase, beta-chain | 540 | 16 |
PF12426 | RNA-dependent RNA polymerase | 41 | 11 |
Pfam ID . | Name . | Model length . | Family (HMM) version . |
---|---|---|---|
PF06317 | Arenavirus RNA polymerase | 2048 | 14 |
PF00603 | Influenza RNA-dependent RNA polymerase subunit PA | 694 | 20 |
PF00604 | Influenza RNA-dependent RNA polymerase subunit PB2 | 754 | 20 |
PF05919 | Mitovirus RNA-dependent RNA polymerase | 498 | 14 |
PF00602 | Influenza RNA-dependent RNA polymerase subunit PB1 | 732 | 20 |
PF07925 | Reovirus RNA-dependent RNA polymerase lambda 3 | 1271 | 14 |
PF00998 | Viral RNA-dependent RNA polymerase | 486 | 26 |
PF00978 | RNA-dependent RNA polymerase | 440 | 24 |
PF04196 | Bunyavirus RNA-dependent RNA polymerase | 742 | 15 |
PF04197 | Birnavirus RNA-dependent RNA polymerase (VP1), palm domain | 531 | 15 |
PF00946 | Mononegavirales RNA-dependent RNA polymerase | 1068 | 22 |
PF00680 | Viral RNA-dependent RNA polymerase | 462 | 23 |
PF02123 | Viral RNA-directed RNA polymerase | 479 | 19 |
PF05788 | Orbivirus RNA-dependent RNA polymerase (VP1) | 1297 | 15 |
PF00972 | Flavivirus RdRp, fingers and palm domains | 451 | 23 |
PF08467 | Luteovirus RNA polymerase P1-P2/replicase | 339 | 13 |
PF06478 | Coronavirus RNA-dependent RNA polymerase, N-terminal | 350 | 16 |
PF03431 | RNA replicase, beta-chain | 540 | 16 |
PF12426 | RNA-dependent RNA polymerase | 41 | 11 |
Pfam ID . | Name . | Model length . | Family (HMM) version . |
---|---|---|---|
PF06317 | Arenavirus RNA polymerase | 2048 | 14 |
PF00603 | Influenza RNA-dependent RNA polymerase subunit PA | 694 | 20 |
PF00604 | Influenza RNA-dependent RNA polymerase subunit PB2 | 754 | 20 |
PF05919 | Mitovirus RNA-dependent RNA polymerase | 498 | 14 |
PF00602 | Influenza RNA-dependent RNA polymerase subunit PB1 | 732 | 20 |
PF07925 | Reovirus RNA-dependent RNA polymerase lambda 3 | 1271 | 14 |
PF00998 | Viral RNA-dependent RNA polymerase | 486 | 26 |
PF00978 | RNA-dependent RNA polymerase | 440 | 24 |
PF04196 | Bunyavirus RNA-dependent RNA polymerase | 742 | 15 |
PF04197 | Birnavirus RNA-dependent RNA polymerase (VP1), palm domain | 531 | 15 |
PF00946 | Mononegavirales RNA-dependent RNA polymerase | 1068 | 22 |
PF00680 | Viral RNA-dependent RNA polymerase | 462 | 23 |
PF02123 | Viral RNA-directed RNA polymerase | 479 | 19 |
PF05788 | Orbivirus RNA-dependent RNA polymerase (VP1) | 1297 | 15 |
PF00972 | Flavivirus RdRp, fingers and palm domains | 451 | 23 |
PF08467 | Luteovirus RNA polymerase P1-P2/replicase | 339 | 13 |
PF06478 | Coronavirus RNA-dependent RNA polymerase, N-terminal | 350 | 16 |
PF03431 | RNA replicase, beta-chain | 540 | 16 |
PF12426 | RNA-dependent RNA polymerase | 41 | 11 |
Pfam ID . | Name . | Model length . | Family (HMM) version . |
---|---|---|---|
PF06317 | Arenavirus RNA polymerase | 2048 | 14 |
PF00603 | Influenza RNA-dependent RNA polymerase subunit PA | 694 | 20 |
PF00604 | Influenza RNA-dependent RNA polymerase subunit PB2 | 754 | 20 |
PF05919 | Mitovirus RNA-dependent RNA polymerase | 498 | 14 |
PF00602 | Influenza RNA-dependent RNA polymerase subunit PB1 | 732 | 20 |
PF07925 | Reovirus RNA-dependent RNA polymerase lambda 3 | 1271 | 14 |
PF00998 | Viral RNA-dependent RNA polymerase | 486 | 26 |
PF00978 | RNA-dependent RNA polymerase | 440 | 24 |
PF04196 | Bunyavirus RNA-dependent RNA polymerase | 742 | 15 |
PF04197 | Birnavirus RNA-dependent RNA polymerase (VP1), palm domain | 531 | 15 |
PF00946 | Mononegavirales RNA-dependent RNA polymerase | 1068 | 22 |
PF00680 | Viral RNA-dependent RNA polymerase | 462 | 23 |
PF02123 | Viral RNA-directed RNA polymerase | 479 | 19 |
PF05788 | Orbivirus RNA-dependent RNA polymerase (VP1) | 1297 | 15 |
PF00972 | Flavivirus RdRp, fingers and palm domains | 451 | 23 |
PF08467 | Luteovirus RNA polymerase P1-P2/replicase | 339 | 13 |
PF06478 | Coronavirus RNA-dependent RNA polymerase, N-terminal | 350 | 16 |
PF03431 | RNA replicase, beta-chain | 540 | 16 |
PF12426 | RNA-dependent RNA polymerase | 41 | 11 |

Schematic diagram of the bioinformatic analysis workflow. (1) Viral RdRp protein sequences were identified using the HMM search-and-update pipeline, which assisted the identification of highly divergent RdRp amino acid sequences. The dashed arrows represent the two iterations of this process. Subsequently, true-positive RdRps were obtained using PalmScan and WebLogo. (2) Taxonomic assignment of RdRps based on the ICTV classification. (3) RdRp nucleotide sequences were clustered using 95% ANI and 85% AF to obtain the vOTUs. (4) AAI distribution histograms were calculated using EzAAI. (5) Phylogenetic analysis was performed for those vOTUs that contained two virus species.
To evaluate the viral authenticity of the 470 637 putative RdRps, we identified true-positive RdRps containing a high confidence “palmprint”: these were delineated using the three essential motifs A–C by PalmScan (false discovery rate = 0.001) (Babaian and Edgar 2022). Notably, for Alphainfluenzavirus influenzae, the RdRp is composed of three subunits—PA, PB1, and PB2—with six of the seven canonical RdRp domains found in PB1 (te Velthuis 2014). We found that PalmScan lacks sensitivity in the detection of RdRp in the order Muvirales, which may be due to the presence of the IDD (Ile-Asp-Asp) motif instead of the canonical GDD (Gly-Asp-Asp) motif (Charon et al. 2021). To address this, we used Clustal Omega (v1.2.4) (--auto option) to align the sequences of viruses of Muvirales. Subsequently, we applied WebLogo (v3.7.9) (Crooks et al. 2004) to create sequence logos and obtained sequences containing the palmprint logo. In addition, to ensure the quality and integrity of RdRp, we removed sequences with ambiguous residues (i.e. “X”s) and those with annotations containing “partial” (Fig. 1).
2.2 Taxonomic assignment of RdRps
The organism names of the RdRp sequences were retrieved using the Biopython SeqIO package. They were compared with the “species,” “Virus name (s),” and the organism names obtained from the “Virus GENBANK Access” column of the ICTV VMR file. If a match was found, the species classification provided by ICTV was assigned to the corresponding RdRp sequence. This process resulted in a total of 56 136 RdRps with species information from the ICTV (Fig. 1).
2.3 Clustering RdRps into vOTUs
Removing highly similar RdRp protein sequences was performed using CD-HIT v4.8.1 (Fu et al. 2012), using a global identity threshold of 99% (-c 0.99). The corresponding RdRp nucleotide sequences were identified and extracted from the GenBank files using the Biopython SeqIO package. Notably, only the exemplar sequences (i.e. containing an RdRp) listed in the VMR file were included in the dataset: these effectively serve as the “anchors” for each virus species. This resulted in a dataset of 7801 RdRp nucleotide sequences, including 1897 exemplar sequences from different virus species (Fig. 1).
To determine within-species genetic diversity, all RdRp nucleotide sequences were grouped based on the species information obtained in the previous step. For each virus species, the sequences were clustered into vOTUs based on 95% average nucleotide identity (ANI) and 85% alignment fraction (AF), as recommended previously (Roux et al. 2019) (Fig. 1). To this end, the RdRp nucleotide sequences were first aligned in all-versus-all local alignments using blastn from the blast+ package v.2.9.0 (options: word_size = 11, max_target_seqs = 10 000, e-value = 0.05) (Camacho et al. 2009). Next, ANI and AF were calculated between all pairs of RdRp nucleotide sequences using an in-house script from the CheckV repository (Nayfach et al. 2021). Finally, clustering was performed using a UCLUST-like greedy, centroid-based algorithm. The clustering process involved the following steps: (i) sorting the RdRp nucleotide sequences by length, (ii) designating the longest genome as the centroid of a new cluster, and (iii) assigning all RdRp nucleotide sequences within 95% ANI and 85% AF to the cluster. Steps 2 and 3 were repeated until all RdRp nucleotide sequences were assigned to a cluster. However, we conjecture that the 95% ANI threshold for RdRp may be too high as it was initially proposed for the complete virus genome rather than the RdRp alone (Roux et al. 2019). To address this, we repeated this analysis, but with ANI thresholds ranging from 70% to 97.5%.
To investigate the potential misassignments between different RNA virus species, all RdRp nucleotide sequences were clustered into vOTUs at different ANI thresholds, ranging from 70% to 97.5% and 85% AF without pregrouping (Fig. 1). In this step, we excluded the assignment information when identifying different virus species within the same vOTU. In addition, to evaluate whether there are potential misclassifications of ICTV-designated RNA virus species, we used the same processing method but with all nonexemplar sequences removed. Subsequently, heatmaps were generated based on ANI values using the pheatmap package in R v4.2.2 and used to visualize the clustering results for different virus species within the same vOTU.
2.4 Comparison of vOTUs at the amino acid level
For those virus species harboring multiple vOTUs, we utilized EzAAI v1.2.2 (-p blastp) (Kim et al. 2021) to calculate the average amino acid identity (AAI) of the RdRp proteins between each vOTU within the virus species. Subsequently, we used the Matplotlib package in Python to generate frequency distribution histograms of AAI (Fig. 1). Histograms with multiple distinct peaks could represent the presence of different virus subtypes or lineages (Chiumenti et al. 2021). In addition, an AAI value of <90% might suggest a potential misassignment for RNA virus species (Babaian and Edgar 2022, Wang et al. 2023).
2.5 Phylogenetic analysis
To validate the potential misassignment between different species within the same vOTUs and to determine their evolutionary relationships, we performed phylogenetic analyses on the RdRp nucleotide sequences. Accordingly, the RdRps of vOTUs with different virus species assignments were extracted, as well as those of other representative species within the same virus genus. Additionally, exemplar sequences from the ICTV were included to serve as the anchor for these species. Multiple sequence alignment was then performed using MAFFT v7.520 (Katoh and Standley 2013) with the -auto mode. Ambiguously aligned regions were removed using trimAl v1.2.rev59 (Capella-Gutiérrez et al. 2009). Finally, maximum likelihood phylogenetic trees of each dataset were estimated using IQ-TREE v1.6.12 (Minh et al. 2020) with 1000 bootstrap replications, and the best-fit substitution model was determined with the “-m MFP” setting. All trees were rerooted using Dendroscope v3.5.10 (Huson and Scornavacca 2012) and visualized using FigTree v1.4.4.
3. Results
3.1 Intraspecies genetic diversity of RdRps
To assess the extent of intraspecies genetic diversity in RNA viruses, we clustered the RdRp nucleotide sequences from each species into vOTUs using the parameters 95% ANI and 85% AF [i.e. following the recommendations of Roux et al. (2019)]. As a result, a total of 7801 RdRp nucleotide sequences, representing 1897 species, were clustered into 4609 vOTUs (Supplementary Table S1). The number of vOTUs within each species ranged from 1 to 234 (Fig. 2a, Supplementary Table S1). Notably, the majority of the virus species (72.43%, n = 1374) contained a single vOTU, consistent with the current ICTV classification. However, 27.57% of the RNA virus species (n = 523) contained multiple vOTUs (Fig. 2b, Supplementary Table S1). Among these, A. influenzae had the highest number of vOTUs at 234, followed by Mammarenavirus lassaense (135), Apple stem pitting virus (129), and Rotavirus A (101) (Fig. 2c). The remaining virus species encompassed <100 vOTUs (Supplementary Table S1).
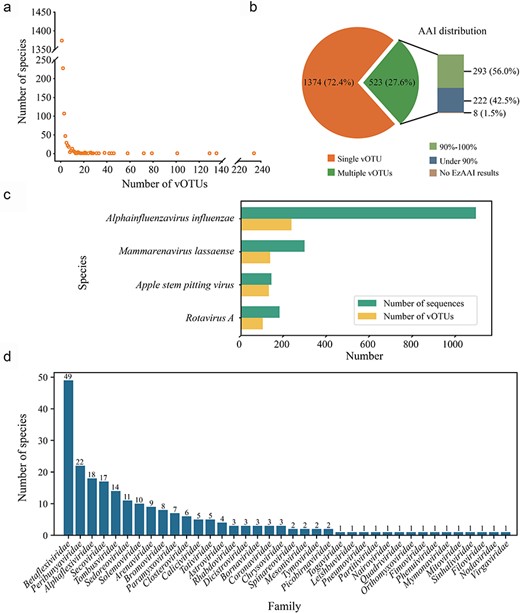
Species rank genetic diversity of ICTV-designated RNA viruses. (a) Summary of the vOTUs within each RNA virus species. The number of vOTUs per species (spanning from 1 to 234) is shown in a scatter plot. (b) The pie chart displays the proportion of virus species with single or multiple vOTUs. The bar chart illustrates the number of species with multiple vOTUs with different EzAAI results. (c) The numbers of sequences (in green) and vOTUs (in yellow) of the virus species with >100 vOTUs (detailed information can be found in Supplementary Table S1). (d) The distribution of the 222 virus species with an AAI value <90% across different virus families.
To further evaluate whether there are potential misclassifications of ICTV-designated RNA virus species, we removed all nonexemplar sequences from this dataset and repeated the analysis described earlier. This revealed that all exemplar sequences were clustered into 1893 vOTUs using 95% ANI and 85% AF without any prior grouping, and 1889 of the ICTV-designated RNA virus species (99.58%) were clustered into a single vOTU. However, four vOTUs each contained two distinct exemplar sequences: Phlebovirus leticiaense (accession no. HM566152) and P. napoliense (accession no. HM566167), Flock House virus (accession no. X77156) and Black beetle virus (accession no. X02396), Duamitovirus boci1 (accession no. EF580100) and D. opno3b (accession no. AM087550), and Botoulivirus zetabotrytidis (accession no. MN605481) and Sclerotinia botoulivirus 3 (accession no. MF444276).
3.2 Potential intraspecies misassignment
For the 523 RNA virus species with multiple vOTUs, the assessment of AAI values between vOTUs within each virus species revealed that 293 had an AAI threshold between 90% and 100% and 222 displayed an AAI threshold <90%, while eight did not have AAI results due to the high divergence between the vOTUs of each virus species (Fig. 2b, Supplementary Table S2). Notably, of the 222 virus species with an AAI threshold below 90%, the family Betaflexiviridae had the highest number of virus species (n = 49), followed by Peribunyaviridae (n = 22) and Alphaflexiviridae (n = 18) (Fig. 2d, Supplementary Table S2).
For virus species with many vOTUs, including A. influenzae, M. lassaense, Apple stem pitting virus, and Rotavirus A, the lowest AAI values were 75.53%, 73.15%, 82.69%, and 63.21%, respectively (Fig. 3a, Supplementary Table S2). Remarkably, the AAI distribution histograms for these four virus species exhibited multiple peaks (Fig. 3a). Alphainfluenzavirus influenzae demonstrated a predominant distribution of AAIs between 90% and 100%, with a smaller proportion falling between 75% and 80%. Interestingly, a single sequence isolated from great fruit bats (GenBank accession no. AXG50945.1) (Campos et al. 2019) resulted in this unique peak. This virus, belonging to subtype H18N11, displayed high similarity to subtype H17N10 (nucleotide identity of 93.7% in PB1), with both types related to classical influenza A viruses (subtypes H1–H16 and N1–N9) (Ciminski and Schwemmle 2021). Mammarenavirus lassaense had a high peak at 75% due to the relatively low AAI between most vOTUs. There were multiple peaks in Apple stem pitting virus, although the distribution is relatively concentrated between 85% and 95%. Rotavirus A exhibited a low AAI value of 63.21%, attributed to the presence of sequence accession QGR26305.1. This sequence had low sequence identity with other types of Rotavirus A. According to the classification criteria of a species within Rotavirus, it should be considered as a new species (Johne et al. 2019).

Pairwise AAI distribution of the virus species with multiple vOTUs. Histograms showing the pairwise AAI distribution of virus species with multiple vOTUs. The lowest AAI is highlighted with a red dashed line. (a) The AAI distribution histograms of the four virus species with >100 vOTUs. (b) The AAI distribution histograms of the eight virus species with the lowest AAI value.
Additionally, some virus species exhibited exceptionally low AAI values (<50%). This was largely due to the presence of certain distant sequences, such as Sapporo virus, Shallot latent virus, Aquareovirus C, Mamastrovirus 3, Beauveria bassiana victorivirus 1, and Yam virus X (Fig. 3b). Moreover, the AAI distribution histograms of Orthopicobirnavirus hominis and Norwalk virus showed multiple peaks (Fig. 3b), suggesting that there were several highly divergent sequences within these species.
3.3 Potential interspecies misassignment
To investigate the occurrence of interspecies misassignment, all RdRp nucleotide sequences from 1897 virus species were clustered into 4593 vOTUs using 95% ANI and 85% AF without any prior grouping. Of these, 4576 vOTUs (99.66%) belonged to a single virus species, thereby supporting established species classifications. Notably, however, 17 vOTUs were found to contain two virus species (Supplementary Table S3). Among these, four contained sequences of Orthobunyavirus ainoense, which clustered with O. shuniense, O. schmallenbergense, O. thimiriense, and O. akabaneense (Supplementary Table S3). Phylogenetic analysis revealed that members of O. ainoense did not form a single monophyletic group (Fig. 4a). Specifically, sequence accessions MH735095.1 and MH735098.1, assigned as O. ainoense, clustered with members of O. akabaneense and O. schmallenbergense. However, they did not cluster with sequence accession HE795087.1, an ICTV exemplar sequence of O. ainoense (Fig. 4a). In addition, sequence accession MH484297.1, which is assigned as O. shuniense, fell into the clade with sequence accession HE795087.1 (Fig. 4a), rather than with the ICTV exemplar sequence of O. shuniense (accession no. KF153118.1). In addition, we observed that sequence accessions MH735097.1 (O. ainoense) and MH735109.1 (O. thimiriense) were grouped together, with the ICTV exemplar sequence of O. thimiriense (accession no. MH484336.1) forming a distinct branch (Fig. 4a). Results from ANI were consistent with those from phylogenetic analysis. Sequence accessions MH735095.1 and MH484339.1 (O. akabaneense) exhibited the highest ANI of 98.24% (Supplementary Fig. S1A). Likewise, an ANI of 97.83% was observed between sequence accessions MH735098.1 and HE795090.1 (O. schmallenbergense) (Supplementary Fig. S1B). In addition, the ANI between sequence accessions MH484297.1 and HE795087.1 (95.21%) was greater than that between sequence accessionss MH484297.1 and KF153118.1 (84.69%) (Supplementary Fig. S1C). Sequence accessions MH735109.1 (O. thimiriense) and MH735097.1 (O. ainoense) exhibited the highest ANI with each other, reaching 97.67% (Supplementary Fig. S1D).
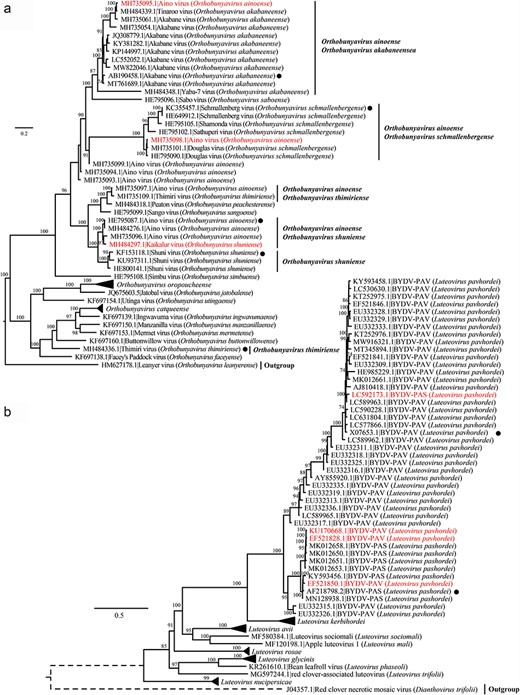
Phylogenetic analysis of representative virus strains and potentially misassigned viruses within the same vOTU. Phylogenetic analysis of RdRp nucleotide sequences from (a) O. ainoense, O. shuniense, O. schmallenbergense, O. thimiriense, and O. akabaneense and other representative species within the same virus genus; (b) L. pashordei and L. pavhordei and other representative species within the same virus genus. Multiple sequence alignment was performed using MAFFT, and the aligned sequences were used to infer maximum likelihood phylogenetic trees. Only bootstrap values >70% are shown. Potentially misassigned sequences were marked in red, and the ICTV exemplar sequences are highlighted with black dots.
Three of the 17 vOTUs were a mixture of Luteovirus pashordei and L. pavhordei (Supplementary Table S3). Phylogenetic analysis revealed that sequence accession LC592173.1 (L. pashordei) fell within a branch containing the sequence accession X07653.1, which is an ICTV exemplar sequence of L. pavhordei, and most sequences on this branch were L. pavhordei (Fig. 4b). Additionally, three members of L. pavhordei (sequence accessions KU170668.1, EF521828.1, and EF521850.1) were grouped with sequence accession AF218798.2—an ICTV exemplar sequence of L. pashordei (Fig. 4b). These phylogenetic patterns were confirmed in the ANI heatmaps (Supplementary Fig. S2). The ANIs between sequence accession LC592173.1 and multiple members of L. pavhordei, including sequence accession X07653.1, were very high (>95%), even reaching 97.81% (Supplementary Fig. S2A). Moreover, the ANI between sequence accessions KU170668.1 and AF218798.2 (93.25%) was higher than that between sequence accessions KU170668.1 and X07653.1 (79.65%) (Supplementary Fig. S2B). Similar results were observed for sequence accessions EF521828.1 and EF521850.1, which displayed higher ANIs with sequence AF218798.2 (93.29% and 97.54%) than with X07653.1 (79.90% and 80.69%) (Supplementary Fig. S2B, C).
For the other nine vOTUs (Supplementary Table S3), results from phylogenetic analyses and heatmaps also showed similar patterns to those described earlier (Supplementary Figs S3 and S4), such as in the cases of Respirovirus laryngotracheitidis and R. muris (Supplementary Figs S3A and S4A), as well as Coguvirus citri and C. eburi (Supplementary Figs S3D and S4B). In addition, we found cases in which two ICTV exemplar sequences from different virus species clustered together with high ANI, such as B. zetabotrytidis and S. botoulivirus 3 (Supplementary Figs S3B and S4I), Black beetle virus and Flock House virus (Supplementary Figs S3F and S4F), and D. boci1 and D. opno3b (Supplementary Figs S3G and S4G). Notably, the identity between the two exemplar sequences P. leticiaense (accession no. HM566152.1) and P. napoliense (accession no. HM566167.1) was 100%.
3.4 Clustering RdRps using different ANI thresholds
To further evaluate whether the RdRp sequences can be used for automated RNA virus classification, we tried ANI thresholds ranging from 70% to 97.5% (Fig. 5, Supplementary Table S4). This revealed that the proportion of accurately assigned species (i.e. clustering one ICTV-assigned species into a single vOTU) with all exemplar sequences and their GenBank relatives initially increased and then decreased with increasing ANI, reaching a maximum of 82.18% at 82.5% ANI. This suggests that using ANI values >82.5% results in an increasing number of virus species being classified into two or more vOTUs (Fig. 5). However, if the RdRp sequences from one virus species are allowed to be clustered into multiple vOTUs, then the proportion of “correctly assigned virus species” steadily increased with increasing ANI and reached 98.58% (n = 1870) at an ANI of 95% and 99.16% (n = 1881) at ANI of 97.5%, even if all the RdRp sequences of the 1897 ICTV-designated species available from GenBank are included in this analysis (Fig. 5).
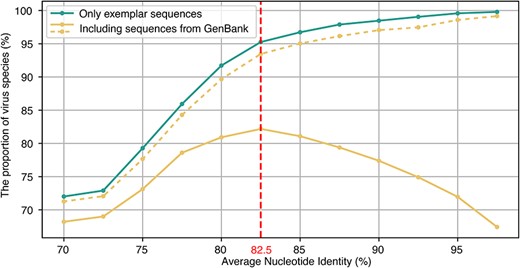
Comparison of the proportion of correctly assigned virus species under different scenarios. The x-axis represents different ANI thresholds from 70% to 97.5% with an interval of 2.5%. The y-axis represents the proportion of correctly assigned virus species (i.e. in which a single ICTV-assigned species is clustered into a single vOTU). The blue line represents the accurately assigned species (only using the 1897 exemplar sequences). The orange line represents the proportion of accurately assigned species if the RdRp sequences of the 1897 virus species available from GenBank are included. The dashed orange line represents the scenario if we allow RdRp sequences from one virus species to be clustered into multiple vOTUs and also believe that these virus species are correctly assigned.
4. Discussion
RNA viruses notoriously possess a high capacity for evolutionary change. Ultimately, this stems from a high background mutation rate, with recombination and/or segmental reassortment shuffling these mutations into new combinations (Sanjuán et al. 2010) and sometimes blurring the boundaries between different virus species. All these factors may lead to different subtypes or variants within the same virus species, potentially complicating virus classification and nomenclature and resulting in taxonomic misassignments (Wu et al. 2010, Groseth et al. 2017, Wang et al. 2019).
The RdRp, as the pivotal polymerase for RNA viruses, is highly conserved, which makes it routinely employed for the classification of RNA viruses. Herein, we investigated the within-species genetic diversity of the RNA virus using RdRp. We found that the majority of RNA virus species (72.43%) correctly possessed a single vOTU, while 27.57% had multiple vOTUs. For example, A. influenzae, M. lassaense, Apple stem pitting virus, and Rotavirus A each had >100 vOTUs. Such high “within-species” genetic diversity may be due to the fact that these virus species themselves have diverged into multiple distinct lineages (Wang et al. 2024). Similarly, the Lassa virus sequences in GenBank (species M. lassaense) can be divided into at least six different species (Radoshitzky and Radoshitzky et al. 2015). Interestingly, when we tried different ANIs, the number of vOTUs of M. lassaense increased with increasing ANI (Supplementary Table S4). Additionally, this high level of genetic diversity resulted in lower AAIs between vOTUs, and 222 RNA virus species had an AAI <90%, with some AAI values even <50%. Previous studies have used an RdRp AAI threshold of <90% for assigning different virus species (Babaian and Edgar 2022, Wang et al. 2023) and 70%–90% for virus genus rank assignment (Babaian and Edgar 2022). Overall, our analyses evidently revealed substantial genetic divergence within some RNA virus species that may pose a serious challenge to virus taxonomy and nomenclature.
We also investigated the potential interspecies inconsistency and misassignment in the nomenclature of RNA viruses based on vOTU (Roux et al. 2019) using different ANI thresholds, although species classification criteria vary from family to family and universal species demarcation criteria are yet to be accepted by the ICTV. We found that the great majority (99.58%) of the ICTV-designated RNA virus species can be correctly clustered into a single vOTU when all nonexemplar sequences are removed, suggesting that the ICTV classification is largely correct and that the RdRp alone may suffice to correctly assign most currently classified viruses to established species even if the 95% ANI and 85% AF cut-offs are applied. However, when incorporating other RdRp sequences of the 1897 ICTV-designated species available from GenBank, only 71.96% (n = 1365) can be correctly clustered into a single vOTU. Therefore, the RdRp exemplar sequences proposed by the ICTV fail to capture the genetic diversity of the remaining virus species.
Of particular note, if RdRp sequences from one virus species are allowed to be clustered into multiple vOTUs, then the proportion of “correctly assigned virus species” steadily increases with increasing ANI and reaches 99.16% at an ANI of 97.5%. Hence, if we assign more exemplar sequences for these virus species, 1870 of the 1897 virus species (98.58%) would be correctly classified with 95% ANI and 85% AF. However, the ANI threshold cannot be arbitrarily designated, and when ANI is >82.5%, an increasing number of virus species fall into two or more vOTUs. Our results indicate that if we select an appropriate ANI for different viruses, RNA virus classification can indeed be largely automated only using RdRp.
Overall, we found widespread misassignments in the nomenclature of RNA viruses available in GenBank. This may be due to a variety of reasons: (i) the assignment of viruses in GenBank has been done in various ways, such as serological cross-reactions, phylogenetic analysis, and distance-based methods (Simmonds 2015); (ii) rectifying these misassignments of sequences is not easy as only the submitters have the authority to modify these sequences; and (iii) the time lag between the implementation of the new ICTV taxonomy and NCBI updating their taxonomy assignments has exacerbated this issue.
There is currently no consensus nor consistency in the species rank classification of RNA viruses. As many viruses have recently been discovered following the deployment of metagenomic sequencing, and many more will be identified in the future, it is imperative that a coherent and rationale virus taxonomy and nomenclature should be established, which is robust to the misassignment documented here. To this end, utilization of the RdRp alone for classification may require a personalized and dynamic ANI threshold system for different RNA viruses and at different taxonomy ranks.
Supplementary data
Supplementary data is available at VEVOLU Journal online.
Conflict of interest:
None declared.
Funding
This study was supported by grants from the Academic Promotion Program of Shandong First Medical University (2019QL006), National Natural Science Foundation of China for Distinguished Young Scholars (32325003), and Natural Science Foundation of Shandong Province (ZR2021QH317). E.C.H. was supported by a National Health and Medical Research Council Investigator award and by AIR@InnoHK administered by the Innovation and Technology Commission, Hong Kong Special Administrative Region, China.
Data availability
The data incorporated in this work were gathered from the public NCBI/GenBank and ICTV resource.