-
PDF
- Split View
-
Views
-
Cite
Cite
Danica Dillion, Curtis Puryear, Longjiao Li, Andre Chiquito, Kurt Gray, National politics ignites more talk of morality and power than local politics, PNAS Nexus, Volume 3, Issue 9, September 2024, pgae345, https://doi.org/10.1093/pnasnexus/pgae345
- Share Icon Share
Abstract
Politics and the media in the United States are increasingly nationalized, and this changes how we talk about politics. Instead of reading the local news and discussing local events, people are more often consuming national media and discussing national issues. Unlike local politics, which can rely on shared concrete knowledge about the region, national politics must coordinate large groups of people with little in common. To provide this coordination, we find that national-level political discussions rely upon different themes than local-level discussions, using more abstract, moralized, and power-centric language. The higher prevalence of abstract, moralized, and power-centric language in national vs. local politics was found in political speeches, politician Tweets, and Reddit discussions. These national-level linguistic features lead to broader engagement with political messages, but they also foster more anger and negativity. These findings suggest that the nationalization of politics and the media may contribute to rising partisan animosity.
American politics and the media are becoming more nationalized. We show how this shift may change political discourse and fuel animosity. Across three unique contexts—political speeches, Tweets from politicians, and Reddit discussions among everyday users—we find that national vs. local political language is consistently more abstract, power-centric, and moralized. These findings reveal how the increasingly nationalized focus of American politics may be fueling partisan animosity, and also inform theories about how language drives engagement with politics. More broadly, the present work advances understanding of how people coordinate in large societies.
Introduction
Political animosity has reached historic highs in the United States, and many point to the degeneration of political discourse to explain this trend (1, 2). The majority of Americans report that current-day political discussions are charged with moral outrage and lack substance and specifics (2). Scholars blame multiple factors for this worrying shift in discourse, including the design of social media algorithms and the monetization of news platforms. However, the rise of this polarized discussion may be a linguistic adaptation to another challenge in modern politics: how to coordinate an increasingly large and diverse population.
Politics in the United States has shifted sharply towards national issues, and political conversations regularly involve partisans from different regions with different experiences (3–12). This nationalization of politics strips away the shared, concrete, place-based knowledge that often provides a common frame of reference for local discussions. Without shared local knowledge, it becomes harder to coordinate people, and so discussants may try to solve this problem by adapting their speech (13, 14). People may find themselves speaking differently when talking about national vs. local issues. Specifically, political speakers may rely more on abstract, power-centric, and moralized language—all features which are widely understood (15–18). This language has the power to better coordinate action but may also divide and polarize society.
Language evolved partly to help people coordinate their behavior (19). By bridging gaps in knowledge between people, it helped groups work together to gather food, maintain shelter, and care for each other. As humans formed larger and more complex societies, language-based coordination became especially crucial. Modern scholars argue that language helped people create political systems by allowing them to share information and form coalitions (20), and Aristotle argued that language about politics—political discourse—was essential to citizenship in nation states (21).
Historically, these coalitions and nation states were smaller than modern nations, making even national issues relatively local. Even as modern nations grew, politics often maintained its focus on local issues, but in recent years, politics and media have shifted away from local concerns. In the United States, political discourse is increasingly grounded in national news outlets like Fox News and CNN over local media (3, 4). Alarmingly, over half of US counties lack sufficient access to local news outlets (5). Many local newspapers have folded as people have lost interest in local political issues and shifted their subscriptions to national papers like the New York Times (6–8). Even local news channels have begun broadcasting stories that cater to a national audience, like national policies and presidential scandals (4, 9, 10), and state-level political rhetoric has become more aligned with national rhetoric (11, 12).
This shift towards national news has been further amplified by social media, with algorithms that highlight the most attention-grabbing controversies from across the country (22). As social media platforms have drawn political discussants online, community spaces for local political discourse have dwindled, pushing many political conversations online (23). Reflecting these changes, modern Americans are more versed in national politics than in the political matters of their state or local community (24).
The nationalization of political discourse poses a challenge for political coordination, which thrives when people share common knowledge and goals, especially when bound to concrete common spaces and shared physical traditions (13, 14). Coordination is difficult enough when trying to mobilize a town or county, but even harder when addressing a heterogeneous audience of 300 million people, with different identities, worldviews, and experiences. The lack of concrete shared experiences creates a need for language that appeals to large audiences across a wide range of backgrounds and perspectives.
The larger audiences of national politics may contribute to a distinct language profile in other ways as well. When audiences are larger, there is also likely to be more competition over political power. Leaders and activists must leverage all available tools to compete for national attention, making it essential to use maximally engaging language. Supporting this idea, past work has found that politicians adopt language that is more emotively engaging and simpler to understand when addressing larger audiences (25, 26). The demands of national political discourse likely cause people to adapt their language both consciously and unconsciously. Political discourse may inevitably shift to more abstract language as societies expand (13, 14), especially language that focuses on two universal concerns: power and morality.
Power is one of the most effective signals for helping people choose sides and coordinate (27). Siding with the group who holds the most social power reduces risks to one's safety and provides more access to resources. As a result, people are highly attuned to social power and possess an innate understanding of its dynamics from infanthood (15, 16). In modern political discourse, power-centric language amplifies political messages because people preferentially attend to the powerful (28, 29). However, coordinating solely on the basis of power also has limitations. Sometimes powerful and corrupt leaders can steer societies towards destruction (30). People also choose sides and elect leaders based on another signal: morality.
Morality is a universally understood coordination signal (17,18). Moral language is at the heart of revolutions throughout history, as it provides a vital tool for forming coalitions against powerful and oppressive leaders. Leaders can leverage moral language to frame their side as morally righteous, creating a moral obligation for people to back their cause (31, 32). Not only is moral language broadly relevant, but it is also highly engaging (33–36). Political messages with moral content are widely shared on social media, demonstrating their potency to boost political engagement (17, 37, 38). Negative moral language especially amplifies engagement for politicians of both major parties, acting as an attention-grabbing societal alarm against wrongdoings (38, 39). This suggests that negative moral sentiment like outrage may be a particularly useful tool for national politics to unite people around common goals. However, while these language tools can engage a large number of people, they are often leveraged by competing sides as they fight each other. As moral language mobilizes members of coalitions on both sides, it may also entrench divisions.
Morality and power are two key forms of language that capture attention (40), but they also tend to be divisive, meaning that their use in political dialogue may create harmful feedback loops of outrage. Moralized language can incite outrage and animosity (41–44). Studies have shown that moralized language has unique impacts on animosity, separate from those of strong negative emotions and partisanship (45). The moralization of political beliefs increases bias and hostility towards opponents, hindering conflict resolution (41, 45). Similarly, power-centric language can escalate group rivalries and conflicts (46, 47). Because these forms of language position groups against each other—competing values and competing for power—they may exacerbate political polarization. The dual nature of these abstract language forms as both unifiers and dividers might provide insight into today's deepening political rifts.
Current research
In the present research, we examine the language of local and national politics. We test whether national-level discussions of political issues use more abstract, moralized, and power-centric language; whether these linguistic differences predict more engagement; and whether they predict further divisive language. We predict that (i) national-level discussions of political issues include more abstract, moralized, negative moral, and power-centric language compared to local-level discussions, (ii) the use of abstract, moralized, negative moral, and power-centric language in political discussions predicts higher levels of public engagement, and (iii) the use of abstract, moralized, negative moral, and power-centric language in political discussions predicts higher levels of subsequent divisive language.
We explore three arenas of political discourse. First, we examine political speeches from city mayors (local) vs. presidents (national). Political speeches showcase the rhetoric politicians use to appeal to voters and rally them behind issues. Comparing speeches between local and national politicians can reveal whether politicians use different language strategies depending on the scope of their audience. Additionally, to account for potential differences between politicians, we conducted within-politician analyses, comparing speeches of senators given before vs. after they were elected into national office. Second, we analyzed the Twitter feeds of mayors (local) vs. federal senators (national). Twitter (recently rebranded as X) is a prominent discourse arena, providing a rich data source to analyze political messaging and public reactions via replies, retweets, and likes. Third, we examined discourse on Reddit, analyzing conversations about the politicized issue of COVID-19 in either local city subreddits or national news subreddits. We also conducted within-person analyses of Reddit comments from the same users on national vs. local subreddits to see whether users adopted different language strategies in national contexts. Reddit is a hub for political discussion among everyday people, and has a forum structure that shows interactive conversations among users. Reddit users also remain anonymous, better allowing them to avoid self-presentational concerns—and better allowing us to examine people's unfiltered speech. Examining Reddit enabled us to test whether the predicted differences in language existed for both strategic political communication (i.e. messages from political leaders) and everyday people.
Results
We present results in two parts. In Part 1, we investigate the frequencies of abstract, moral, and power-centric language in local and national political discussion. We hypothesize that national discourse employs language tailored to engage a diverse audience from varied backgrounds and regions, resulting in more abstract, moral, and power-centric language in national than local politics. We also expect specifically negative moral language to be more prevalent in national contexts than local because of its attention-grabbing properties. We investigate the frequencies of these language forms among politicians and everyday people.
In Part 2, we test whether abstract, moral, and power-centric language in political posts fuels engagement and animosity in responses. We predict that political messages with more abstract, moral, negatively moral, and power-centric language will spread more widely because they are universally engaging. Additionally, because moralized and power-centric language can incite animosity and intensify group conflict, we expect that political dialogue with higher rates of moralized and power-centric language will generate more divisive discussion with greater anger and negativity.
Part 1: the language profile of national vs. local politics
We first tested our key predictions that national political discussion uses more abstract, moralized, and power-centric language than local politics. As we outlined above, we compared national vs. local political language across three contexts: political speeches (between mayors and presidents: n = 101; and among federal senators before vs. after holding federal office positions: n = 110), Tweets (n = 112,899), and Reddit comments (between-users: n = 412,778; within-users: n = 39,223). We also tested whether national politics includes more negative moral language specifically (e.g. condemnation, outrage, and disgust) because people often weigh negatively valanced information more heavily (48).
We analyzed moral language using the extended Moral Foundations Dictionary (eMFD (49)), calculating the morality score for each text as the average probability that each word in the text indicates the text's moral relevance, summed across five moral foundations. We analyzed power-centric language using the Linguistic Inquiry and Word Count summary algorithm (50), which estimates the percentage of words in a given text related to power. Finally, we analyzed abstractness using Brysbaert et al.'s lexicon (51). These dictionaries demonstrated conceptual and internal validity during development (49–51), and have exhibited convergent validity with manually annotated ratings of analogous concepts in relevant studies (52–54). In addition to the text dictionary analyses, we replicated the main results from the between-person Twitter and Reddit analyses using the large language model GPT-3.5-turbo, which demonstrates high alignment with manual annotators at rating psychological constructs (55) (see Supplementary Material Section 2). We also validated our measures with a manually annotated sample of codes (see Supplementary Material Section 3). The full details of the procedure are described in the Methods section. See Table S41 for examples of data rated high and low in language features.
Between-person results of national vs. local politics
We regressed moral, power-centric, abstract, and negative moral language upon local vs. national context in four separate linear regressions. We fit these four models for each of the three between-person datasets: speeches from city mayors vs. presidents, Tweets from mayors vs. federal senators, and local city subreddits vs. national news subreddits.
We found that each language feature (moral, power-centric, abstract, and negative moral language) was significantly more common in national than local contexts (with the exception of negative moral language in between-politician speeches; see Table 1 for full results). For instance, federal senator Tweets contained approximately 2.5 times as many power-related words as city mayor Tweets. Our primary results in Table 1 report standardized effect sizes for our continuous measures of moral and abstract language. To aid with the interpretability of these effect sizes, we also dichotomized these continuous scores using logistic regression models that we originally trained to validate our measures. This approach revealed that 35% of Tweets written by federal senators were classified as moral in nature compared to 5% of Tweets written by city mayors, and 53% of Tweets written by federal senators were classified as abstract in nature compared to 30% of Tweets written by city mayors.
Language feature . | b (SE) . | β . | t . | df . | P . | 95% CIs . | d . |
---|---|---|---|---|---|---|---|
Abstract language | |||||||
0.10 (0.002) | 0.43 | 42.11 | 97,060 | < 0.001 | 0.10,0.11 | 0.43 | |
Political speeches (between) | 0.07 (0.01) | 0.96 | 5.44 | 98 | <0.001 | 0.04,0.09 | 1.10 |
Political speeches (within) | 0.03 (0.02) | 0.40 | 2.07 | 47.28 | 0.04 | 0.001,0.06 | 0.44 |
Reddit (between) | 0.07 (0.001) | 0.20 | 63.14 | 420,387 | <0.001 | 0.07,0.07 | 0.20 |
Reddit (within) | 0.05 (0.004) | 0.14 | 11.46 | 3,197 | <0.001 | 0.04,0.05 | 0.09 |
Moral language | |||||||
0.04 (0.001) | 0.47 | 45.79 | 96,033 | <0.001 | 0.04,0.05 | 0.47 | |
Political speeches (between) | 0.03 (0.004) | 1.21 | 7.58 | 98 | <0.001 | 0.02,0.04 | 1.53 |
Political speeches (within) | 0.03 (0.01) | 0.70 | 3.09 | 24.33 | 0.005 | 0.01,0.04 | 0.73 |
Reddit (between) | 0.03 (<0.001) | 0.26 | 79.22 | 398,156 | <0.001 | 0.03,0.03 | 0.26 |
Reddit (within) | 0.02 (0.001) | 0.16 | 12.03 | 2,350 | <0.001 | 0.01,0.02 | 0.18 |
Negative moral languagea | |||||||
−0.23 (0.006) | −0.40 | −38.55 | 96,033 | <0.001 | −0.24, −0.22 | −0.40 | |
Political speeches (between) | −0.01 (0.02) | −0.13 | −0.65 | 98 | 0.51 | −0.06,0.03 | −0.13 |
Political speeches (within) | −0.13 (0.05) | −0.54 | −2.43 | 19.92 | 0.02 | −0.23, −0.02 | −0.56 |
Reddit (between) | −0.15 (0.002) | −0.22 | −65.30 | 398,156 | <0.001 | −0.15, −0.15 | −0.22 |
Reddit (within) | −0.10 (0.01) | −0.16 | −11.93 | 2,288 | <0.001 | −0.12, −0.09 | −0.14 |
Power-centric language | |||||||
2.01 (0.04) | 0.52 | 51.84 | 97,115 | <0.001 | 1.94, 2.09 | 0.53 | |
Political speeches (between) | 0.94 (0.19) | 0.89 | 4.90 | 96 | <0.001 | 0.56, 1.32 | 1.00 |
Political speeches (within) | 1.69 (0.44) | 0.73 | 3.82 | 39.34 | <0.001 | 0.81, 2.55 | 0.78 |
Reddit (between) | 0.76 (0.01) | 0.20 | 60.90 | 423,822 | <0.001 | 0.74,0.79 | 0.20 |
Reddit (within) | 0.54 (0.05) | 0.14 | 11.40 | 2,099 | <0.001 | 0.45,0.63 | 0.16 |
Language feature . | b (SE) . | β . | t . | df . | P . | 95% CIs . | d . |
---|---|---|---|---|---|---|---|
Abstract language | |||||||
0.10 (0.002) | 0.43 | 42.11 | 97,060 | < 0.001 | 0.10,0.11 | 0.43 | |
Political speeches (between) | 0.07 (0.01) | 0.96 | 5.44 | 98 | <0.001 | 0.04,0.09 | 1.10 |
Political speeches (within) | 0.03 (0.02) | 0.40 | 2.07 | 47.28 | 0.04 | 0.001,0.06 | 0.44 |
Reddit (between) | 0.07 (0.001) | 0.20 | 63.14 | 420,387 | <0.001 | 0.07,0.07 | 0.20 |
Reddit (within) | 0.05 (0.004) | 0.14 | 11.46 | 3,197 | <0.001 | 0.04,0.05 | 0.09 |
Moral language | |||||||
0.04 (0.001) | 0.47 | 45.79 | 96,033 | <0.001 | 0.04,0.05 | 0.47 | |
Political speeches (between) | 0.03 (0.004) | 1.21 | 7.58 | 98 | <0.001 | 0.02,0.04 | 1.53 |
Political speeches (within) | 0.03 (0.01) | 0.70 | 3.09 | 24.33 | 0.005 | 0.01,0.04 | 0.73 |
Reddit (between) | 0.03 (<0.001) | 0.26 | 79.22 | 398,156 | <0.001 | 0.03,0.03 | 0.26 |
Reddit (within) | 0.02 (0.001) | 0.16 | 12.03 | 2,350 | <0.001 | 0.01,0.02 | 0.18 |
Negative moral languagea | |||||||
−0.23 (0.006) | −0.40 | −38.55 | 96,033 | <0.001 | −0.24, −0.22 | −0.40 | |
Political speeches (between) | −0.01 (0.02) | −0.13 | −0.65 | 98 | 0.51 | −0.06,0.03 | −0.13 |
Political speeches (within) | −0.13 (0.05) | −0.54 | −2.43 | 19.92 | 0.02 | −0.23, −0.02 | −0.56 |
Reddit (between) | −0.15 (0.002) | −0.22 | −65.30 | 398,156 | <0.001 | −0.15, −0.15 | −0.22 |
Reddit (within) | −0.10 (0.01) | −0.16 | −11.93 | 2,288 | <0.001 | −0.12, −0.09 | −0.14 |
Power-centric language | |||||||
2.01 (0.04) | 0.52 | 51.84 | 97,115 | <0.001 | 1.94, 2.09 | 0.53 | |
Political speeches (between) | 0.94 (0.19) | 0.89 | 4.90 | 96 | <0.001 | 0.56, 1.32 | 1.00 |
Political speeches (within) | 1.69 (0.44) | 0.73 | 3.82 | 39.34 | <0.001 | 0.81, 2.55 | 0.78 |
Reddit (between) | 0.76 (0.01) | 0.20 | 60.90 | 423,822 | <0.001 | 0.74,0.79 | 0.20 |
Reddit (within) | 0.54 (0.05) | 0.14 | 11.40 | 2,099 | <0.001 | 0.45,0.63 | 0.16 |
aNegative morality is reverse coded such that negative coefficients indicate higher rates of negative morality.
Language feature . | b (SE) . | β . | t . | df . | P . | 95% CIs . | d . |
---|---|---|---|---|---|---|---|
Abstract language | |||||||
0.10 (0.002) | 0.43 | 42.11 | 97,060 | < 0.001 | 0.10,0.11 | 0.43 | |
Political speeches (between) | 0.07 (0.01) | 0.96 | 5.44 | 98 | <0.001 | 0.04,0.09 | 1.10 |
Political speeches (within) | 0.03 (0.02) | 0.40 | 2.07 | 47.28 | 0.04 | 0.001,0.06 | 0.44 |
Reddit (between) | 0.07 (0.001) | 0.20 | 63.14 | 420,387 | <0.001 | 0.07,0.07 | 0.20 |
Reddit (within) | 0.05 (0.004) | 0.14 | 11.46 | 3,197 | <0.001 | 0.04,0.05 | 0.09 |
Moral language | |||||||
0.04 (0.001) | 0.47 | 45.79 | 96,033 | <0.001 | 0.04,0.05 | 0.47 | |
Political speeches (between) | 0.03 (0.004) | 1.21 | 7.58 | 98 | <0.001 | 0.02,0.04 | 1.53 |
Political speeches (within) | 0.03 (0.01) | 0.70 | 3.09 | 24.33 | 0.005 | 0.01,0.04 | 0.73 |
Reddit (between) | 0.03 (<0.001) | 0.26 | 79.22 | 398,156 | <0.001 | 0.03,0.03 | 0.26 |
Reddit (within) | 0.02 (0.001) | 0.16 | 12.03 | 2,350 | <0.001 | 0.01,0.02 | 0.18 |
Negative moral languagea | |||||||
−0.23 (0.006) | −0.40 | −38.55 | 96,033 | <0.001 | −0.24, −0.22 | −0.40 | |
Political speeches (between) | −0.01 (0.02) | −0.13 | −0.65 | 98 | 0.51 | −0.06,0.03 | −0.13 |
Political speeches (within) | −0.13 (0.05) | −0.54 | −2.43 | 19.92 | 0.02 | −0.23, −0.02 | −0.56 |
Reddit (between) | −0.15 (0.002) | −0.22 | −65.30 | 398,156 | <0.001 | −0.15, −0.15 | −0.22 |
Reddit (within) | −0.10 (0.01) | −0.16 | −11.93 | 2,288 | <0.001 | −0.12, −0.09 | −0.14 |
Power-centric language | |||||||
2.01 (0.04) | 0.52 | 51.84 | 97,115 | <0.001 | 1.94, 2.09 | 0.53 | |
Political speeches (between) | 0.94 (0.19) | 0.89 | 4.90 | 96 | <0.001 | 0.56, 1.32 | 1.00 |
Political speeches (within) | 1.69 (0.44) | 0.73 | 3.82 | 39.34 | <0.001 | 0.81, 2.55 | 0.78 |
Reddit (between) | 0.76 (0.01) | 0.20 | 60.90 | 423,822 | <0.001 | 0.74,0.79 | 0.20 |
Reddit (within) | 0.54 (0.05) | 0.14 | 11.40 | 2,099 | <0.001 | 0.45,0.63 | 0.16 |
Language feature . | b (SE) . | β . | t . | df . | P . | 95% CIs . | d . |
---|---|---|---|---|---|---|---|
Abstract language | |||||||
0.10 (0.002) | 0.43 | 42.11 | 97,060 | < 0.001 | 0.10,0.11 | 0.43 | |
Political speeches (between) | 0.07 (0.01) | 0.96 | 5.44 | 98 | <0.001 | 0.04,0.09 | 1.10 |
Political speeches (within) | 0.03 (0.02) | 0.40 | 2.07 | 47.28 | 0.04 | 0.001,0.06 | 0.44 |
Reddit (between) | 0.07 (0.001) | 0.20 | 63.14 | 420,387 | <0.001 | 0.07,0.07 | 0.20 |
Reddit (within) | 0.05 (0.004) | 0.14 | 11.46 | 3,197 | <0.001 | 0.04,0.05 | 0.09 |
Moral language | |||||||
0.04 (0.001) | 0.47 | 45.79 | 96,033 | <0.001 | 0.04,0.05 | 0.47 | |
Political speeches (between) | 0.03 (0.004) | 1.21 | 7.58 | 98 | <0.001 | 0.02,0.04 | 1.53 |
Political speeches (within) | 0.03 (0.01) | 0.70 | 3.09 | 24.33 | 0.005 | 0.01,0.04 | 0.73 |
Reddit (between) | 0.03 (<0.001) | 0.26 | 79.22 | 398,156 | <0.001 | 0.03,0.03 | 0.26 |
Reddit (within) | 0.02 (0.001) | 0.16 | 12.03 | 2,350 | <0.001 | 0.01,0.02 | 0.18 |
Negative moral languagea | |||||||
−0.23 (0.006) | −0.40 | −38.55 | 96,033 | <0.001 | −0.24, −0.22 | −0.40 | |
Political speeches (between) | −0.01 (0.02) | −0.13 | −0.65 | 98 | 0.51 | −0.06,0.03 | −0.13 |
Political speeches (within) | −0.13 (0.05) | −0.54 | −2.43 | 19.92 | 0.02 | −0.23, −0.02 | −0.56 |
Reddit (between) | −0.15 (0.002) | −0.22 | −65.30 | 398,156 | <0.001 | −0.15, −0.15 | −0.22 |
Reddit (within) | −0.10 (0.01) | −0.16 | −11.93 | 2,288 | <0.001 | −0.12, −0.09 | −0.14 |
Power-centric language | |||||||
2.01 (0.04) | 0.52 | 51.84 | 97,115 | <0.001 | 1.94, 2.09 | 0.53 | |
Political speeches (between) | 0.94 (0.19) | 0.89 | 4.90 | 96 | <0.001 | 0.56, 1.32 | 1.00 |
Political speeches (within) | 1.69 (0.44) | 0.73 | 3.82 | 39.34 | <0.001 | 0.81, 2.55 | 0.78 |
Reddit (between) | 0.76 (0.01) | 0.20 | 60.90 | 423,822 | <0.001 | 0.74,0.79 | 0.20 |
Reddit (within) | 0.54 (0.05) | 0.14 | 11.40 | 2,099 | <0.001 | 0.45,0.63 | 0.16 |
aNegative morality is reverse coded such that negative coefficients indicate higher rates of negative morality.
As a robustness check, we replicated the findings from the single-predictor linear regression models in logistic regression models with three of the language variables (moral, power-centric, and abstract language) entered simultaneously as predictors of national vs. local contexts (negative moral language was entered into identical models in place of moral language). These analyses tested whether abstract, moral, and power-centric language each uniquely distinguish national from local contexts. In the Twitter robustness analyses, we controlled for politicians’ political parties and the year they took office to account for potential differences between parties and levels of political experience. Across the robustness models for each dataset, the four language features of interest were almost always more common in national contexts,a suggesting that they are unique features differentiating national from local political discourse (see Supplementary Material Section 1 for full coefficient tables) (Figure 1).
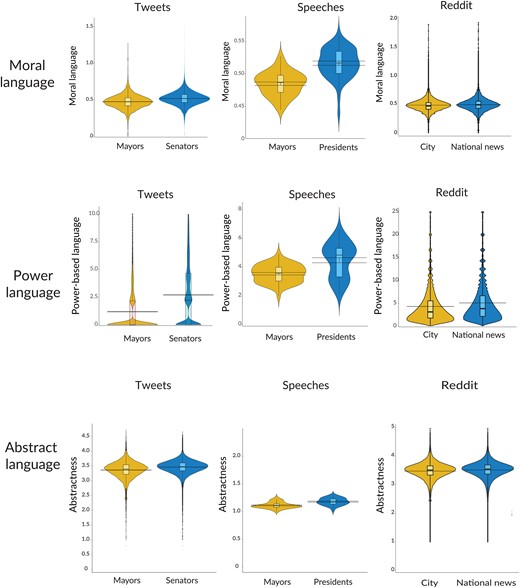
Features of national vs. local political discourse in between-person datasets. Frequencies of abstract, moral, and power-centric language across Tweets, between-politician speeches, and Reddit posts. The violin plots on the right sides of the graphs represent national contexts, and the violin plots on the left sides of the graphs represent local contexts. Confidence intervals represent standard errors. All statistical tests are two-tailed.
We found that politicians and laypeople use more abstract, moral, negatively moral, and power-centric language in national contexts compared to local ones. However, part of the reason for these distinct language profiles could be that differences in context between local and national settings lead people to discuss less impactful topics in local politics. To address the possibility that differences in conversation topics explain the present findings, we used topic modeling to control for the topic of discussion in the Twitter data. After controlling for topics, federal senators still used more abstract, moral, negatively moral, and power-centric language than local mayors. This suggests that even when discussing the same topics, national-level discussions employ a distinct language profile (see Tables S31–S34 for results; see Table S40 for list of topics).b
Although we found large differences between mayoral vs. presidential political speeches in abstract, moral, and power-centric language (Cohen's ds of 1.10, 1.53, and 1.00), we did not find a significant difference in negative moral language between mayor vs. president speeches. This may be because the United States presidential speeches were inauguration and state of the union speeches, which tend to be more positive in nature. In other words, though national politicians’ language was consistently more moralized, whether this moral language is more negatively valanced may depend on context.
The Twitter and political speech datasets indicated distinct language profiles between national and local politicians. The same language differences were present among laypeople (i.e. Reddit users) discussing the political issue of COVID-19. City subreddits used significantly less abstract, moralized, power-centric, and negatively moralized language than national news subreddits. This has two major implications. First, it suggests that the differences between national and local discourse among political elites may bleed into national political discussions among ordinary Americans. Second, it suggests that these linguistic differences do not simply reflect national politics focusing on more inherently divisive issues, but rather how people discuss the same issues in national vs. local contexts.
After finding that abstract, moralized, negative moral, and power-centric language distinguished local from national political discussions, we wanted to test the extent to which they were the defining features of national political dialogue. To do so, we conducted an exploratory analysis comparing the effects of our four variables of interest to 91 additional language factors from the LIWC-22 dictionary (see Figure S2). In contrast to the 91 other language factors, power-centric, moral, and abstract language were three of the strongest effects distinguishing national from local political dialogue in political speeches, Twitter, and Reddit.
Together, these findings suggest that national dialogue leverages language features that appeal to a wider base: morality, power, and abstract language (see Figure 1). In contrast, local dialogue employed more concrete language, consistent with the idea that local politics can highlight the shared knowledge among community members.
Within-person results of national vs. local politics
We also examined how the same people changed their language when delivering political messages in local vs. national contexts. Two of the datasets afforded these within-person analyses: the longitudinal speeches data (in which we obtained speeches from 22 US senators before and after they entered national politics, n = 110 speeches) and the Reddit data (in which 4,863 users had comments in both local and national subreddits; n = 39,223). These analyses provided a stronger test of whether differences in political language across national and local contexts were due to the scope of the political environments rather than differences in the types of people creating messages in each environment.
We tested the within-person effects of national vs. local context via mixed effects models. In four separate models for each of the two datasets, we regressed moral, power, abstract, and negative moral language upon national vs. local context while including random intercepts and random slopes for each person.c We controlled for the word count in the within-person speeches. In both the longitudinal speeches and the Reddit dataset, we found significant within-person effects upon all four language variables (see Table 1 for full results). As depicted in Figure 2, the language of 22 elected US senators became significantly more moralized, power-centric, and abstract (on average) after they entered national politics (i.e. speeches given as local or state politicians vs. speeches given as US senators; see Figure 2). We observed the same changes in Reddit users’ language as they moved back and forth between local and national subreddit communities to discuss COVID-19. These results suggest that national contexts evoke more moralized, power-centric, and abstract messages about political topics.
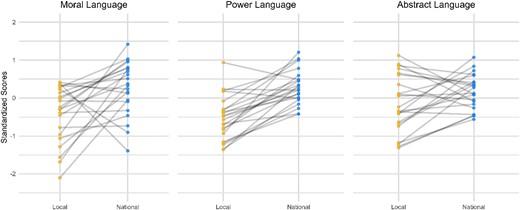
Within-politician differences in national vs. local political discourse. Frequencies of abstract, moral, and power-centric language in speeches given by United States federal senators while in national office vs. prior to their national political positions. Dots on the right sides of the graphs represent national contexts and dots on the left sides of the graphs represent local contexts. Each line connects a federal senator's local degree of language use to their national level.
Part 2: the language of national politics fuels engagement and animosity
After finding that national political dialogue includes more abstract, moral, and power-centric language, we next investigated the potential of these features to garner broad engagement. We theorized that the language inherent to national politics helps leaders attract attention—a necessary first step to mobilizing coalitions. In the Twitter and Reddit datasets, we evaluated the impact of moral, power-centric, negative moral, and abstract language on engagement metrics, including replies, retweets, and likes in response to online posts. The aim of these analyses was to test whether the linguistic features that were associated with national politics each uniquely predicted the spread of political messages.
At the same time, nationalized political language may also have a darker consequence: inciting animosity. Moralized content can incite outrage on social media, and power-centric language can stir group conflict (39, 41–44, 46). To investigate whether these language features sparked outrage in responses to political Tweets and Reddit comments, we tested whether abstract, moral, and power-centric language—the features found to characterize national political language—were associated with anger and negative moral language in replies.
We also conducted an exploratory analysis testing whether abstract, moral, and power-centric language were more strongly associated with engagement in national than local discourse. Though a robust literature suggests that moral language broadly increases engagement on social media (57), it is unclear whether the effectiveness of moral and power-centric language varies depending on the context of political dialogue (e.g. local vs. national discourse). We expected moral and power-centric language to be broadly engaging across political contexts, but we also wondered whether they may be particularly effective in national discourse, given that national politics cannot rely on shared concrete context to the same degree as local politics. We therefore tested whether the power-centric and moralized language of national discourse is more effective in national vs. local contexts.
Engagement
We tested whether the themes found to differentiate national from local politics—moral, power-centric, negative moral, and abstract language—allowed politicians and laypeople to garner more engagement with their messages, measured as the number of retweets, replies, and likes they received in response to online posts.d We fit mixed effect models to account for nesting in the data (see Supplementary Material Section 1 for full coefficient tables). Abstract, power-centric, and moral (or negative moral) language were entered as simultaneous predictors of engagement (measured as retweets for Twitter and replies for Reddit). Tweets were nested within politicians, and Reddit comments were nested within Reddit users. We log-transformed the number of replies and retweets (58). As in Part 1, we replicated the results controlling for politicians’ political parties and the year they took office in the Twitter analyses (see Supplementary Material Section 1 for full coefficient tables).
We tested whether abstract, moral, negative moral, and power-centric language in politician Tweets predicted the number of retweets that they received. As predicted, abstract (b = 0.17, β = 0.02, SE = 0.01, t(95,900) = 11.29, P < 0.001, CIs [0.14,0.20]), moral (b = 1.25, β = 0.06, SE = 0.04, t(95,890) = 33.09, P < 0.001, CIs [1.17, 1.32]), negative moral (b = −0.27, β = −0.08, SE = 0.006, t(95,890) = −44.26, P < 0.001, CIs [−0.28, −0.25]), and power-centric (b = 0.05, β = 0.10, SE = 0.001, t(95,890) = 52.19, P < 0.001, CIs [0.05,0.05]) language were all uniquely associated with increased engagement. These significant relationships remained after controlling for politician party and the year they were elected into office (see Supplementary Material Section 1).
We next conducted exploratory analyses to test whether the impact of abstract, moral, negative moral, and power-centric language on engagement was stronger for national politicians than local politicians. We thought that the language of national politics might be especially likely to fuel engagement in national discussions. On the other hand, because abstract, moral, and power-centric language are generally captivating, they may drive engagement regardless of political scale. We explored the interactions between political scale (national vs. local) and each feature of national political language and found significant interactions whereby moral (b = 0.77, β = 0.04, SE = 0.12, t(95,900) = 6.39, P < 0.001, CIs [0.53, 1.00]), negative moral (b = −0.16, β = −0.05, SE = 0.02, t(95,900) = −8.98, P < 0.001, CIs [−0.20, −0.13]), abstract (b = 0.14, β = 0.02, SE = 0.04, t(95,910) = 3.31, P = 0.001, CIs [0.06,0.22]), and power-centric (b = 0.04, β = 0.08, SE = 0.004, t(95,910) = 9.59, P < 0.001, CIs [0.03,0.05]) language were associated with higher retweet counts for national politicians than local politicians. Together these results suggest that moral, abstract, and power-centric language are particularly effective at engaging audiences for national politicians.
After finding that the language features common in national politics drive engagement for politicians, we next tested whether the same pattern would emerge among everyday people online. We analyzed whether Reddit users receive more replies to their posts when using language features typical of national politics. As predicted, abstract (b = 0.06, β = 0.04, SE = 0.005, t(161,200) = 13.41, P < 0.001, 95% CI = [0.05,0.07]), moral (b = 0.11, β = 0.03, SE = 0.01, t(161,100) = 9.65, P < 0.001, 95% CI = [0.09,0.13]), negative moral (b = −0.03, β = −0.04, SE = 0.002, t(161,100) = −15.40, P < 0.001, 95% CI = [−0.04, −0.03]), and power-centric (b = 0.001, β = 0.009, SE = 0.0004, t(161,000) = 3.03, P = 0.002, 95% CI = [0.0004,0.002]) language were all uniquely associated with increased engagement.
We next explored whether the impact of these language features on engagement was stronger for national subreddits than city subreddits, as was found among politicians on Twitter. We were unsure whether we would observe differences in the effectiveness of these language forms based on local vs. national contexts among everyday Reddit users, rather than figureheads of political groups trying to attract support for their side. Contrary to our findings among politicians on Twitter, there were significant interactions whereby abstract (b = −0.05, β = −0.03, SE = 0.009, t(161,200) = −5.61, P < 0.001, CIs [−0.07, −0.03]), moral (b = −0.18, β = −0.04, SE = 0.02, t(161,000) = −7.25, P < 0.001, CIs [−0.22, −0.13]), power-centric (b = . −0.004, β = −0.03, SE = 0.001, t(160,700) = −5.27, P < 0.001, CIs [−0.006, −0.003]), and negative moral (b = 0.03, β = −0.06, SE = 0.004, t(161,000) = 7.62, P < 0.001, CIs [0.02,0.04]) language were associated with fewer replies for national news subreddits than city subreddits. The conflicting patterns on Reddit vs. Twitter may be due to the relative rarity of power-centric and moralized language in local contexts, causing these language features to garner more attention in city subreddits. However, the reasons why abstract, power-centric, and moralized language proved more effective at engaging audiences for city subreddits despite their rarity in city subreddits remain uncertain and present an interesting question for future research. Nevertheless, abstract, moral, power-centric, and negative moral language were each uniquely associated with engagement, supporting our main predictions.
Political animosity
Consistent with past work, we found that the language profile of national politics boosts engagement with political messages. But this heightened engagement may have a cost: the same language that drives engagement may also drive animosity. We next tested whether the language features found to differentiate national from local politics generated more divisive conversations (i.e. received replies marked by more anger and negative moral language). We again fit mixed effect models to account for nesting in the data (see Supplementary Material Section 1 for full coefficient tables). Abstract, power-centric, and moral (or negative moral) language were entered as simultaneous predictors of anger and negative moral language. Tweets were nested within politicians, and Reddit comments were nested within Reddit users. We replicated the results controlling for politicians’ political parties and the year they took office in the Twitter analyses (see Supplementary Material Section 1 for full coefficient tables).
We first examined the overall differences between replies in national and local contexts (e.g. comparing all Twitter replies to federal politicians to all Twitter replies to local politicians). We found that national political messages incited more negativity and anger in replies than did local political messages. People replied to Tweets written by national politicians with more negative moral language (b = −0.03, β = −0.16, SE = 0.002, t(285,374) = −13.55, P < 0.001, CIs [−0.03, −0.02]) and anger (b = 0.38, β = 0.11, SE = 0.04, t(285,395) = 9.70, P < 0.001, CIs [0.30,0.45]) than they did to tweets written by local politicians. Similarly, replies to national news subreddit posts contained more negative moral language (b = −0.16, β = −0.23, SE = 0.002, t(383,836) = −66.70, P < 0.001, CIs [−0.17, −0.16]) and more anger (b = 0.03, β = 0.02, SE = 0.004, t(412,438) = 7.24, P < 0.001, CIs [0.02,0.04]) than replies to city subreddit posts. The animosity that national political messages provoke could partly be due to their divisive linguistic tools. Here we test whether the language features found to distinguish national from local politics—abstract, moral, and power-centric language—were associated with anger and negative moral language in replies.
We tested whether the four language features found to differentiate national from local politics were associated with anger and negative moral language in replies. As predicted, abstract (b = 0.11, β = 0.01, SE = 0.03, t(274,300) = 4.02, P < 0.001, CIs [0.06,0.17]), moral (b = 0.73, β = 0.02, SE = 0.07, t(277,700) = 10.35, P < 0.001, CIs [0.59,0.87]), negative moral (b = −0.13, β = −0.02, SE = 0.01, t(276,400) = −11.37, P < 0.001, CIs [−0.15, −0.11]), and power-centric (b = 0.02, β = 0.02, SE = 0.002, t(278,200) = 11.03, P < 0.001, CIs [0.01,0.02]) language were all uniquely associated with more anger in replies.
Additionally, abstract (b = −0.01, β = −0.01, SE = 0.002, t(279,800) = −4.85, P < 0.001, CIs [−0.01, −0.004]), moral (b = −0.06, β = −0.03, SE = 0.004, t(281,200) = −15.97, P < 0.001, CIs [−0.07, −0.05]), negative moral (b = 0.03, β = 0.09, SE = 0.001, t(279,900) = 43.84, P < 0.001, CIs [0.02,0.03]), and power-centric (b = −0.001, β = −0.03, SE < 0.001, t(281,100) = −17.19, P < 0.001, CIs [−0.002, −0.001]) language were all uniquely associated with more negative moral language in replies. These significant relationships remained after controlling for politician party and the year they were elected into office (see Supplementary Material Section 1). These findings suggest that when politicians use moral, power-centric, and abstract language, they may incite anger and negative moral language in response.
We found that the language features of national politics predict more divisive language in response to politicians, but what about ordinary people discussing a single political issue? As predicted, moral (b = 0.16, β = 0.01, SE = 0.02, t(124,500) = 8.84, P < 0.001, CIs [0.13,0.20]), negative moral (b = −0.02, β = −0.01, SE = 0.003, t(154,200) = −6.19, P < 0.001, CIs [−0.03, −0.01]), and power-centric (b = 0.002, β = 0.01, SE = 0.001, t(132,700) = 3.02, P = 0.003, CIs [0.001,0.003]) language were all uniquely associated with more anger in replies. Abstract language was not significantly associated with anger in replies, b = 0.003, β = 0.001, SE = 0.007, t(134,700) = 0.35, P = 0.73, CIs [−0.01,0.02], potentially suggesting that abstract language alone is a less robust predictor of animosity. However, abstract (b = −0.07, β = −0.03, SE = 0.005, t(170,000) = −15.69, P < 0.001, CIs [−0.08, −0.07]), moral (b = −0.30, β = −0.05, SE = 0.01, t(164,100) = −25.32, P < 0.001, CIs [−0.32, −0.27]), negative moral (b = 0.17, β = 0.14, SE = 0.002, t(170,900) = 81.12, P < 0.001, CIs [.16,0.17]), and power-centric (b = −0.004, β = −0.02, SE < 0.001, t(161,900) = −11.40, P < 0.001, CIs [−0.005, −0.004]) language were all uniquely associated with more negative moral language in replies. These findings suggest that laypeople may also generate more divisive conversations when using the language features common in national politics.
General discussion
Modern political discourse in the United States has grown more divisive and less substantive than before (1, 2, 59). At the same time, politics has become more dominated by national over local issues (3–5). We explored whether these changes may be related. National politics differs from local politics in that it lacks the concrete common ground that comes from shared place-based knowledge (13, 14). This national focus creates challenges for political coordination because the millions of people across the United States have different backgrounds and perspectives. We propose that specific kinds of language in political discourse help to solve this coordination challenge: moral, power-centric, and abstract language. These themes are universally understood, allowing politicians, commentators, and everyday people to capture the attention of other people and coordinate their opinions and behaviors on broad national issues. These language features may not only harness attention but may also contribute to increased political animosity.
Our studies support the hypothesis that political language styles vary between local and national levels of discourse. Across three communication mediums—politician speeches, Twitter, and Reddit—national vs. local political discussions used more moral, power-centric, and abstract language. In national settings, people were more likely to share moral messages like “Everyone involved in this corrupt, fraudulent, and defamatory scheme must be held accountable,” and power-centric messages like “Strong borders = strong America.” US presidents used more moral, power-centric, and abstract language in their speeches than city mayors; federal senators’ Tweets included more of these themes than Tweets from city mayors; and national news subreddits contained higher rates of these features than local city subreddits.
Differences between national vs. local discourse existed not only across different people, but also within the same person, depending on their position. The same politicians adopted more moralized, power-centric, and abstract language when they were senators compared to when they were mayors. The same Reddit users used these language features more in national news subreddits than in local city subreddits. These results suggest that moral, power-centric, and abstract language are defining features of national political discourse among both politicians and the general public.
The themes found to define national politics fostered widespread engagement with political discussions among both politicians and everyday people. Tweets and Reddit comments that included more moral, power-centric, and abstract language received higher levels of engagement. These results are consistent with past work showing that people are highly attuned to moral and power-centric themes (15, 16, 18, 28, 29, 33–38). They also support recent models of social media engagement, such as Brady and colleagues’ PRIME model, which posits that information relevant to prestige, in-group identity, morality, and emotion is particularly likely to capture attention (37). Two key features of national politics—moral and power-centric language—align with the predictions of this model.
These language features captured attention but also cultivated anger and negativity. People responded with greater animosity to Tweets and Reddit comments that had higher rates of moral, power-centric, and abstract language. These findings are consistent with research showing that moralized and power-centric themes can fuel animosity and escalate conflict (41–47). While the moralized and power-centric language of nationalized politics effectively engages people, its role in coordinating side-taking may also contribute to us-vs.-them political animosity.
Proposed mechanisms and integration with past work
Like many social phenomena, the unique language profile of national politics likely emerges from multiple factors. Historically, allegiances have often been forged based on power dynamics and moral standings, which continue to be strong coordination signals (17, 18, 27). Our findings suggest that people are especially likely to leverage these strategies when coordinating across broad audiences. The need to appeal to a broader, more heterogeneous audience on the national stage creates a demand for widely relevant themes like morality and power. This aligns with cognitive science findings that broad, distant concepts are best understood through abstract representation (13, 14), and that moral perceptions become more abstract as social networks expand (60). Moreover, the competitive nature of national politics likely encourages the use of attention-grabbing language. National politicians may strategically use language that elicits anger to bolster support among partisan voters. Previous research has found that national politicians incite anger during key campaign periods because outgroup anger enhances in-group party loyalty (61). Thus, the moralized, power-centric, and abstract language of national politics may aid politicians in garnering support through both its attention-grabbing and anger-inciting nature.
The present work builds upon existing research from political science on the relationships between national and local political rhetoric. Using topic modeling, this literature has found that state political agendas have grown more homogenous and similar to national agendas (11, 12), but that local politicians use political speech with substantial differences in topics and less polarizing content than national politicians (62, 63). This work has revealed trends of nationalization in state-level politics and shown that political speech at the local community level is distinct from national political speech. Building on these insights, the present work identifies a core set of language that robustly emerges more frequently in national contexts. Moreover, the within-person analyses in the present work demonstrate that the same people use a more nationalized language profile when moving from local to national contexts.
Open questions and future directions
We have proposed that the nationalization of politics is accompanied by changes in political language that fuel both engagement and division. However, it is also possible that the engaging language of national politics has contributed to this nationalization. Although there is not yet consensus on why politics has become increasingly nationalized in the United States, some leading ideas suggest that nationalization is demand-based. Past research indicates that the shifting focus toward national issues is driven by a decline in interest in local politics and a rise in interest in national politics (7, 8). One possible reason for this increased interest in national politics is that new technologies have connected Americans with more people across the country than ever before, leading them to care more about national events. Another likely reason is that the same language the present work has found to capture attention in the competitive national political arena also makes national politics more interesting, thereby accelerating the shift to national politics. This suggests that the nationalization of politics may be self-reinforcing: the shift to national politics may lead people to use more moralized and powerful language to drive engagement, and this highly engaging language may further accelerate the shift to national politics.
Another question is whether political discourse targeting larger, national audiences will always rely upon more abstract, moralized, and power-centric language. We theorize that coordinating around common causes is difficult in national US politics because of the many different experiences, identities, and beliefs that need to be addressed. This raises the question of whether the same effects would be present in societies that are more homogeneous than the United States, where people share more common experiences, identities, and beliefs. In more homogeneous societies, we predict that the effects observed in the present work may be attenuated, but it is still more difficult to rally a large group of people behind a cause than it is a smaller group of people. For these reasons, replicating the present findings in other cultural contexts is an exciting path for future work.
A strength of the present work is that we leverage naturalistic data across three unique contexts. However, naturalistic studies also carry limitations. One alternative explanation for our findings is that national dialogues tend to use more power-centric and moral language because national issues inherently address more abstract and easily moralized issues. For example, national healthcare discussions naturally involve more moral language than discussions about pothole repairs. This potential confound motivated our Reddit analyses (which examined a single issue, COVID-19) and the topic modeling in our Twitter analyses. Results from these controlled analyses suggested that differences in the topics of discussion alone could not explain the differences in language across national and local discussions. Nonetheless, it is still possible that differences in local vs. national contexts may have led people to discuss different aspects of the same topics. When people discuss COVID-19 in local contexts, they may spend more time talking about things like the specific locations where vaccines are available, a more concrete and practical aspect of COVID-19 discussions that may be more relevant locally. But this is arguably an important part of our theorized mechanism: it is easier to talk about more concrete and practical aspects of the same topic in local contexts where people have greater shared knowledge.
In light of our findings that national political discourse sparks more anger and negativity than local political discourse, future research may further explore whether the nationalization of politics drives political polarization. A longitudinal analysis on political nationalization and polarization over time would provide valuable insight into how the nationalizing political landscape may contribute to political tensions. Future research should also further examine the overall effectiveness of abstract, moralized, and power-centric language in local vs. national contexts. While we predict that such language generally promotes engagement, it could also have drawbacks in certain contexts. For example, it may seem inappropriate to moralize certain local political discussions that are focused on concrete problems, like city park maintenance. Our preliminary analyses comparing the use of abstract, moral, and power-centric language at local and national levels showed mixed results. In local contexts, using these language features increased engagement, suggesting that they are advantageous in local discussions even if less common. As expected, federal senators gained more engagement for using these language features than city mayors. However, an unexpected finding was that local city subreddits (vs. national news subreddits) received greater boosts in engagement from using the language features found to be more typical of national politics. This inconsistency could simply reflect contrasting norms between news-focused vs. regional subreddits, or it might highlight differences based on whether messages come from politicians vs. the general public. Future research could clarify whether the costs to using these language features vary by context.
Conclusion
In the modern arena of nationalized politics, moralized, power-centric, and abstract language provide a unifying and attention-grabbing framework for broad audiences. These linguistic strategies have several benefits: they unite people around universal concerns and encourage political participation. However, this abstract, moralized, and power-centric political dialogue may steer attention away from important local issues where everyday people can have more political impact. Instead, it focuses people on contentious national issues in a manner that creates animosity. For those seeking to ascend the political ladder, it may pay to use more abstract, moral, and power-centric language. However, as our social worlds increase in scope and complexity, it is important to both remember the importance of local issues, and to discover ways for large and diverse groups of people to effectively coordinate without stoking conflict.
Methods
Language measures
Power-centric language
We measured language reflecting power using the Linguistic Inquiry and Word Count summary algorithm, which estimates the percentage of words in a given text related to power (50). Examples of ‘power’ language include the words “own,” “order,” “allow,” and “power.”
Moral language
We measured moral language using the extended Moral Foundations Dictionary (eMFD (49)), which provides crowd sourced probabilities that the presence of a given word indicates a passage is morally relevant to one of five moral domains. This dictionary contains a large vocabulary of both morally relevant and irrelevant words. We summed the five probabilities for each word (i.e. its probability for each of five moral domains) to estimate the general moral relevance of each word in the dictionary. Following Hopp and colleagues (49), we then looked up the probability of moral relevance for each word in a given passage (e.g. a speech) and averaged them to measure the moral relevance of a passage.
Negative moral language
We calculated the moral sentiment of each passage using the VADER lexicon, which contains estimates of whether words reflect negative or positive sentiment. We then weighted the eMFD moral language score by the sentiment score. We applied the same method we used to calculate general moral language to calculate moral sentiment (i.e. looking up the moral sentiment estimate for each word in a passage and averaging them). This created a single measure of moral sentiment, in which higher scores indicate more positive moral language and lower scores indicate more negative moral language.
Abstract language
To measure abstract language, we used Brysbaert et al.'s concreteness dictionary (51). This dictionary is a validated lexicon of 40 thousand English words rated on their relevance to experiences involving all senses and motor responses. We measured abstractness by averaging the concreteness scores for each word in a given text based on this dictionary.
GPT-3.5 text analysis replication
We replicated the main results of the between-person Twitter and Reddit analyses using the large language model GPT-3.5-turbo (55) (see Supplementary Material Section 2). We used the OpenAI API to obtain ratings from GPT-3.5-turbo of each language feature of interest. We used code adapted from Rathje et al. (55) to prompt GPT-3.5-turbo. The temperature was set to 0 to capture the model's highest probability outcomes. See Table S35 for prompts.
Manually annotated reliability check
Two of the present work's authors manually coded a random subset of 250 Tweets for abstract, moral, and power-centric language to validate the dictionary and GPT-3.5-turbo text analysis methods. See Supplementary Material Section 3 for full methods and results.
Datasets
Within-politician speeches
We collected speech transcripts from United States federal senators from before they were elected into national office (n = 43) and during their time as federal senators (n = 67) to test whether politicians adopt different language strategies after taking the national stage. We included multiple speeches from 22 federal senators (Democrats = 10, Republicans = 10, and Independents = 2). 12 senators were men and 10 were women. 17 senators were White, 2 were Black, and 3 were Latino/a. The speeches were collected from a variety of online sources, including CSPAN and YouTube. We excluded campaign speeches from the local speeches, because the primary purpose of these were appealing to national voters. Otherwise, the local and national speeches were given on a variety of topics.
Mayor vs. president speeches
We collected speech transcripts from United States city mayors (n = 51) and presidents (n = 50) to analyze the language profiles of local vs. national political speeches. We included multiple speeches from each United States president since Richard Nixon. For both mayoral and presidential speeches, we focused on obtaining state of the union and inaugural speeches to standardize speech purpose. Mayor speeches were selected to represent a variety of geographic regions spanning the United States. 35 mayors were men and 16 were women. 33 mayors were White, 11 were Black, 4 were Latino/a, 1 was Asian, and 3 were multiracial. Mayors in the Democratic party were overrepresented (Democrats = 32, Republicans = 15, and Independents = 4) due to difficulty finding transcripts of speeches given by Republican mayors. The speeches were obtained from a variety of online sources, including nonprofit databases, news organizations, and YouTube.
Mayor vs. senator Tweets
We collected Tweets posted by city mayors (n = 13,538) and US senators (n = 99,293) in the United States. A list of active senators was gathered from the website https://www.senate.gov/senators/ and senators with a Twitter account were included in the dataset. City mayors were selected from the largest city from each state with populations under 300,000 people. The rationale for mayor selection was to strike a balance between accounts that would have a decent amount of activity, but to exclude mayors of massive cities that might more closely resemble national politicians (like the mayor of New York City). Tweets were collected using the Twitter API from January 2021 to December 2021, and all replies to the Tweets were collected. To measure engagement with Tweets, we collected the number of times each Tweet was retweeted, replied to, and liked. Retweet, reply, and like counts were log-transformed to account for the exponential distributions of the variables.
City vs. national news subreddits
Reddit is a valuable source of data on how people communicate about important political issues in various contexts. We collected Reddit comments from subreddits for cities, states, national news, and world news between March 2020 and October 2021. We focused on comparisons between city (n = 148,369) and national news (n = 275,399) subreddits to best reflect local vs national differences. We narrowed our search to comments about COVID-19 to control for topic and test whether an identical issue would be discussed differently in a local vs national context, limiting the scope to an issue that would be highly salient to most users. To conduct this search, we used the Pushshift API to collect every submission with the words “coronavirus OR covid* OR pandemic” in the title (see Table S39 for list of included subreddits). In addition to examining differences between city and national news subreddits, we assessed within-user differences among 4,863 users who had comments in both city and national news subreddits (n = 39,223; see Tables S5–S8 for full model results).
Notes
All four language features were significantly more common in national contexts in the Twitter and Reddit data, but there were two exceptions in the between-person speeches data when the language features were entered as simultaneous predictors. When entered as a simultaneous predictor of mayoral versus presidential speeches along with moral and abstract language, power-centric language was not significantly different between local and national speeches. However, power-centric language was significantly more common in presidential than mayoral speeches when entered as a simultaneous predictor along with negative moral language and abstract language. Additionally, negative moral language was not significantly different between presidential and mayoral speeches when entered as a simultaneous predictor along with power-centric language and abstract language. All four language features were consistently more common at the national level when entered in separate models, with the exception of negative moral language in the between-person speeches data (see Table 1).
To control for topic of discussion, we extracted topics from our Twitter data using BERTopic and fit mixed effects models for each language outcome, including random slopes and random intercepts for the topic. See Supplementary material for details.
Following recommendations to include the maximal (and justified) random effects structure (56), we included both random slopes and random intercepts. However, two of these models returned singular fits: models predicting power and abstract language in the longitudinal speech data. These models returned estimates of random effects variance equal to zero when random slopes were included. The reported within-person effects for these outcomes included random intercepts but not random slopes. Results did not substantively differ in models with random slopes and intercepts versus random intercept only models.
We present results on the number of retweets posts received in the main manuscript, but we examined two additional metrics of engagement on Twitter: replies and likes (See Supplementary Material Section 5). Our predictions were further supported in analyses for the number of replies and likes that Tweets received.
Supplementary Material
Supplementary material is available at PNAS Nexus online.
Funding
This research was supported by the Templeton World Charity Foundation under the grant TWCF-2022-31340 “Testing the ‘Morality and (Mis)Perception Model of Polarization’ Across Cultures and Contexts,” StandTogether through The Center for the Science of Moral Understanding, and The New Pluralists.
Data Availability
The data and code used in this paper are available at https://osf.io/gk46p/.
References
Author notes
Competing Interest: The authors declare no competing interest.