-
PDF
- Split View
-
Views
-
Cite
Cite
Chenyan Jia, Angela Yuson Lee, Ryan C Moore, Cid Halsey-Steve Decatur, Sunny Xun Liu, Jeffrey T Hancock, Collaboration, crowdsourcing, and misinformation, PNAS Nexus, Volume 3, Issue 10, October 2024, pgae434, https://doi.org/10.1093/pnasnexus/pgae434
- Share Icon Share
Abstract
One of humanity's greatest strengths lies in our ability to collaborate to achieve more than we can alone. Just as collaboration can be an important strength, humankind's inability to detect deception is one of our greatest weaknesses. Recently, our struggles with deception detection have been the subject of scholarly and public attention with the rise and spread of misinformation online, which threatens public health and civic society. Fortunately, prior work indicates that going beyond the individual can ameliorate weaknesses in deception detection by promoting active discussion or by harnessing the “wisdom of crowds.” Can group collaboration similarly enhance our ability to recognize online misinformation? We conducted a lab experiment where participants assessed the veracity of credible news and misinformation on social media either as an actively collaborating group or while working alone. Our results suggest that collaborative groups were more accurate than individuals at detecting false posts, but not more accurate than a majority-based simulated group, suggesting that “wisdom of crowds” is the more efficient method for identifying misinformation. Our findings reorient research and policy from focusing on the individual to approaches that rely on crowdsourcing or potentially on collaboration in addressing the problem of misinformation.
Introduction
One of humankind's strengths lies in our ability to collaborate. Under optimal conditions, people can often take on bigger, more challenging problems when they work together than they can on their own (1). Studies show that groups can outperform individuals at learning new skills (2), remembering important facts and events (3), working with technology (4), and making rational decisions (5).
At the same time, one weakness humans face is a vulnerability to deception. People often fail to detect deceptive messages because of a well-documented psychological tendency to presume communication is true (i.e. the truth bias see 6). Worries over susceptibility to deception have intensified with the spread of misinformation on social media, which undermines the democratic process (7) and threatens personal and public health (8).
Can collaboration ameliorate our weaknesses in detecting misinformation? A small body of research indicates that groups are better than individuals at detecting lies in interpersonal communication (e.g. about personal likes/dislikes, (9)). However, meta-analytic evidence suggests that detecting online misinformation about news and current events is meaningfully different from detecting lies about personal facts in everyday conversation (10), limiting the ability to extrapolate findings on interpersonal lie detection to the context of online misinformation. Furthermore, research on process losses indicates that the potential benefits of teamwork are not always realized in small groups (1), especially when people exert less effort as part of a group (e.g. (11)). While studies on the effects of collaboration on deception detection reveal positive results, whether group engagement will enhance or detract from misinformation detection is unclear.
To establish whether active collaboration contributes to performance gains above and beyond the effort and attention of multiple individuals’ judgments, small group researchers compare collaboration outcomes against “wisdom of the crowd” outcomes by statistically aggregating individual judgments (1). In the context of misinformation research, aggregating laypersons’ judgments of news veracity can approximate the performance of expert fact-checkers (for a review, see (12)). Individuals in these studies make judgments in isolation rather than via active collaboration and discussion. While the “wisdom of crowds” appears effective in identifying misinformation, it remains an open question as to how groups who can actively work together and collaborate on misinformation judgments might perform. Some contexts may lend themselves to groups of people collaborating actively to discern misinformation (e.g. discussing news shared on a private messaging platform), while other contexts lend themselves to aggregating individual judgments of veracity (e.g. “X”/Twitter's Community Notes).
To examine how collaboration influences misinformation detection, we conducted a lab experiment that asked people to evaluate the veracity of social media posts containing credible news or misinformation as part of a group of 3 (group condition; ngroups = 40) or alone (individual condition; nindividuals = 124). We compared the collaborating groups against 2 simulations of statistically aggregated individuals: (ⅰ) majority-based simulated groups in which the majority vote of the simulated group determined accuracy (i.e. wisdom of the crowds) and (ⅱ) consensus-based simulated groups in which all 3 votes were required to answer correctly to determine accuracy (i.e. matching the requirements of the collaborating groups). Small group research tends to use majority vote as a method to assess the contribution of collaboration that is above and beyond individual contributions (1).
The results reveal that collaborating groups were more accurate than individuals working alone at identifying posts containing misinformation, but they did not outperform the majority-based simulated groups. This pattern suggests that while collaborating groups outperformed individuals, the improvement was not above and beyond the collective effort of individual contributions (i.e. the wisdom of crowds). Nonetheless, the collaborating groups outperformed consensus-based simulated groups, indicating that there may be some potential for collaborative approaches to improve misinformation detection.
Scholars, social media platforms, and governments have invested considerable resources into developing interventions to combat misinformation (e.g. media literacy education, flagging content), but existing approaches focus overwhelmingly on individuals. Consistent with prior research on the value of collaboration in human problem-solving (5), our study is one of the first to test an alternative approach that leverages collaboration and active group discussion to build resilience against misinformation. Our findings bolster prior work on the power of the wisdom of crowds to successfully identify and flag misinformation via asynchronous judgments (12). Furthermore, we demonstrate that collaborative groups, who can communicate and reason together, outperformed consensus-based simulated groups, suggesting a promising collaborative approach to combat misinformation that requires further research.
Results
We fit mixed-effects regression models predicting post veracity detection accuracy as a function of whether participants judged social media posts (see Materials and methods for stimuli details) as individuals or as part of a collaborating group of 3, with both posts and participants modeled as random effects. Groups were significantly more likely to accurately identify false posts (M = 89.50% of judgments were accurate, SD = 13.58%) than individuals (M = 82.10%, SD = 18.88%) (β = 0.75, SE = 0.32, P = 0.02, Cohen's d = 0.43), but not more likely to accurately identify true posts (β = 0.07, SE = 0.24, P = 0.78, d = 0.05) (see Fig. 1). We also observed a positive and significant interaction (β = 0.78, SE = 0.33, P = 0.02, d = 0.43) between the experimental condition (1 = group, 0 = individual) and post veracity (1 = false, 0 = true) in a model predicting the likelihood that people rate posts “false” (1 = post rated as “false”, 0 = post rated as “true”), suggesting that groups were better able to discern true from false posts than individuals [e.g. (13)]a. Finally, groups were significantly less likely to report intentions to share false social media posts than individuals (β = −0.29, SE = 0.14, P = 0.04, d = 0.38).
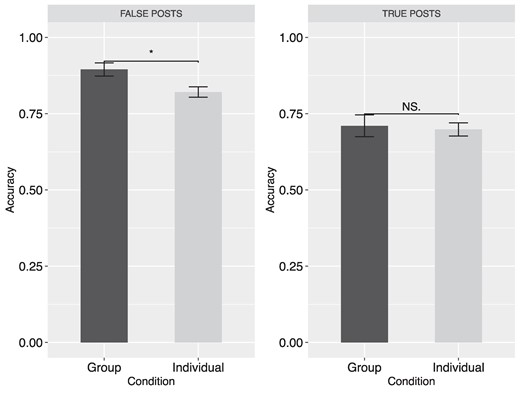
Accuracy rate of detecting false (left) and true (right) social media posts. The error bar indicates mean ± SE.
To assess whether the accuracy advantage for groups over individuals came from active collaboration versus the mere statistical aggregation of individual judgments, we performed simulations that sampled 1,000 randomly selected triads within each stimulus set. We simulated the “wisdom of crowds” by calculating the majority vote of these statistically aggregated individuals (e.g. (12)). Due to unequal variance between the 2 types of groups, a Welch's t test found that collaborative groups did not outperform the majority-based simulated groups, t(39.22) = 1.75, P = 0.09 (see Table 1). Specifically, there was no statistical difference between collaborative groups and majority-based simulated groups for detecting either false t(39.03) = 0.26, P = 0.80, or true posts, t(39.02) = 1.81, P = 0.08. The collaborating groups were as effective but not better than the “wisdom of crowds,” suggesting that the process of active collaboration did not contribute above and beyond the additional effort of multiple individuals making judgments.
Group type . | Overall accuracy . | False posts . | True posts . |
---|---|---|---|
Collaborative groups (n = 40) | 80.25% (SD = 12.71%) | 89.50% (SD = 13.58%) | 71.00% (SD = 22.62%) |
Individuals (n = 124) | 75.97% (SD = 13.79%) | 82.10% (SD = 18.88%) | 69.84% (SD = 24.13%) |
Simulations | |||
Majority-based simulated groups (n = 1,000) | 83.77% (SD = 6.46%) | 90.06% (SD = 1.37%) | 77.49% (SD = 1.63%) |
Consensus-based simulated groups (n = 1,000) | 45.74% (SD = 1.15%) | 57.12% (SD = 1.47%) | 34.37% (SD = 1.79%) |
Group type . | Overall accuracy . | False posts . | True posts . |
---|---|---|---|
Collaborative groups (n = 40) | 80.25% (SD = 12.71%) | 89.50% (SD = 13.58%) | 71.00% (SD = 22.62%) |
Individuals (n = 124) | 75.97% (SD = 13.79%) | 82.10% (SD = 18.88%) | 69.84% (SD = 24.13%) |
Simulations | |||
Majority-based simulated groups (n = 1,000) | 83.77% (SD = 6.46%) | 90.06% (SD = 1.37%) | 77.49% (SD = 1.63%) |
Consensus-based simulated groups (n = 1,000) | 45.74% (SD = 1.15%) | 57.12% (SD = 1.47%) | 34.37% (SD = 1.79%) |
Group type . | Overall accuracy . | False posts . | True posts . |
---|---|---|---|
Collaborative groups (n = 40) | 80.25% (SD = 12.71%) | 89.50% (SD = 13.58%) | 71.00% (SD = 22.62%) |
Individuals (n = 124) | 75.97% (SD = 13.79%) | 82.10% (SD = 18.88%) | 69.84% (SD = 24.13%) |
Simulations | |||
Majority-based simulated groups (n = 1,000) | 83.77% (SD = 6.46%) | 90.06% (SD = 1.37%) | 77.49% (SD = 1.63%) |
Consensus-based simulated groups (n = 1,000) | 45.74% (SD = 1.15%) | 57.12% (SD = 1.47%) | 34.37% (SD = 1.79%) |
Group type . | Overall accuracy . | False posts . | True posts . |
---|---|---|---|
Collaborative groups (n = 40) | 80.25% (SD = 12.71%) | 89.50% (SD = 13.58%) | 71.00% (SD = 22.62%) |
Individuals (n = 124) | 75.97% (SD = 13.79%) | 82.10% (SD = 18.88%) | 69.84% (SD = 24.13%) |
Simulations | |||
Majority-based simulated groups (n = 1,000) | 83.77% (SD = 6.46%) | 90.06% (SD = 1.37%) | 77.49% (SD = 1.63%) |
Consensus-based simulated groups (n = 1,000) | 45.74% (SD = 1.15%) | 57.12% (SD = 1.47%) | 34.37% (SD = 1.79%) |
Given that collaborating groups were required to come to a consensus in their decisions about the social media posts rather than majority vote, we also calculated consensus-based simulation. Collaborative groups were significantly more accurate than consensus-based simulated groups in overall accuracy, t(39.03) = 17.17, P < 0.001, being better at detecting both false, t(39.02) = 10.24, P < 0.001, and true, t(39.04) = 15.08, P < 0.001, social media posts. The results suggest that the collective judgment of collaborative groups was more accurate than the consensus of statistically aggregated individual judgments.
Discussion
Our study reveals that both collaborating groups and majority-based simulated groups outperform individuals in the detection of misinformation. The results confirm the effectiveness of the “wisdom of crowds” approach in detecting misinformation, consistent with other recent studies (12). These results have important policy implications, for example, that social media platforms and technology companies should harness crowd-sourced approaches as an effective and efficient way to combat misinformation at scale (14).
Our findings also highlight the potential of active collaboration as a novel strategy to mitigating the spread of online misinformation. Collaborating groups that synchronously evaluated the veracity of claims outperformed individuals at correctly identifying false news, consistent with prior work on the benefits of collaboratively detecting lies told interpersonally (e.g. (9)). While this approach did not outperform the standard majority-based “wisdom of crowds” approach, the collaborating groups detected misinformation more accurately than consensus-based simulated groups.
To advance research on collaboration in misinformation detection, future research should consider asking collaborating participants to make private individual decisions, rather than come to consensus, to allow for cleaner comparisons to the majority vote wisdom of crowds approach. Future work also needs to consider stimuli detection rates. In our study, participants discerned fake from real news at substantially higher than chance rates, which creates statistical artifacts when calculating majority vote decisions. We also acknowledge that our study is limited to a student sample who may have greater misinformation detection abilities or digital literacy than the general public, which might lead to the statistical artifacts of the simulated groups (which may also explain the high baseline accuracy). Despite these limitations, our study sheds light on the importance of understanding the role of collaboration in the context of misinformation.
While our study is the first to our knowledge to assess collaborative groups’ ability to identify misinformation, isolating the precise aspects of group collaboration (e.g. knowledge shared during discussion, additional motivation and effort, social norms) that led to the benefits to misinformation detection observed in this study via explicit manipulation of those factors is an important priority for future research to better understand the mechanisms that underlie gains from group collaboration. With the emergence of generative AI, we also suggest testing how human–AI collaboration may improve the detection of online misinformation as a future direction.
Taken together, these findings suggest a reorientation of research and policy from focusing on the individual to leveraging more collaborative, social, and crowd-sourced approaches to address the problem of misinformation.
Materials and methods
This research involved human subjects who provided informed consent. All procedures were approved by the Stanford University Institutional Review Board under the protocol “Collaborative Study on Detecting Misinformation.” Participants were randomly assigned to judge the veracity of social media posts as part of a group of 3, or on their own. All were students from Stanford University's research pool. We selected 40 apolitical social media posts (half true and half false) fact-checked by major organizations such as Snopes.com and PolitiFact or large health organizations such as the CDC. Stimuli topics ranged from health, civics, and science. We created 4 different sequences of 10 posts each. Each participant, whether in the group or individual condition, read and judged one sequence consisting of 10 social media posts in a lab setting. Following Klein and Epley (9), participants in the group conditions were instructed to verbally discuss each post and come to a collective judgment on its veracity; see Fig. 2 for detailed experimental procedures.
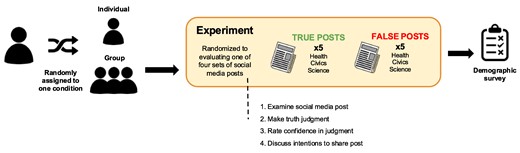
Notes
Acknowledgments
The authors would like to thank the research assistants Tori Qiu, JiAnne Kang, Josie Rose Gross-Whitaker, and Shane Patrick Griffith who helped the data collection. In addition, the authors thank the members of the Stanford Social Media Lab for providing their feedback on this piece. The authors extend their appreciation to the participants who made this research possible and the reviewers whose suggestions strengthened our work.
Supplementary Material
Supplementary material is available at PNAS Nexus online.
Funding
This research was supported by the Stanford Social Impact Lab Test Solutions Funding and the National Science Foundation, United States CHS 1901151. A.Y.L. and R.C.M. were supported by a Stanford Interdisciplinary Graduate Fellowship.
Author Contributions
C.J.: conceptualization, methodology, formal analysis, data curation, writing—original draft preparation, visualization; A.Y.L.: conceptualization, methodology, data curation, writing—original draft preparation; R.C.M.: methodology, formal analysis, data curation, writing—original draft preparation; C.H.-S.D.: data curation, writing—original draft preparation; S.X.L.: methodology, writing—review and editing, project administration, funding acquisition; J.T.H.: conceptualization, methodology, writing—review and editing, supervision, project administration, funding acquisition.
Data Availability
The study was pre-registered on the Open Science Frameworkb. The data and code for the main analyses are available on the Open Science Framework repository https://osf.io/zkbn6/.
References
Author notes
C.J. and A.Y.L. contributed equally to this work.
Competing Interest: The authors declare no competing interests.