-
PDF
- Split View
-
Views
-
Cite
Cite
Yong Huang, Meiqi Shang, Tingting Liu, Kejian Wang, High-throughput methods for genome editing: the more the better, Plant Physiology, Volume 188, Issue 4, April 2022, Pages 1731–1745, https://doi.org/10.1093/plphys/kiac017
- Share Icon Share
Abstract
During the last decade, targeted genome-editing technologies, especially clustered regularly interspaced short palindromic repeat (CRISPR)/CRISPR-associated protein (Cas) technologies, have permitted efficient targeting of genomes, thereby modifying these genomes to offer tremendous opportunities for deciphering gene function and engineering beneficial traits in many biological systems. As a powerful genome-editing tool, the CRISPR/Cas systems, combined with the development of next-generation sequencing and many other high-throughput techniques, have thus been quickly developed into a high-throughput engineering strategy in animals and plants. Therefore, here, we review recent advances in using high-throughput genome-editing technologies in animals and plants, such as the high-throughput design of targeted guide RNA (gRNA), construction of large-scale pooled gRNA, and high-throughput genome-editing libraries, high-throughput detection of editing events, and high-throughput supervision of genome-editing products. Moreover, we outline perspectives for future applications, ranging from medication using gene therapy to crop improvement using high-throughput genome-editing technologies.
Introduction
In 2012, Emmanuelle Charpentier and Jennifer Doudna discovered one of gene technology’s sharpest tools: clustered regularly interspaced short palindromic repeats (CRISPR)/CRISPR-associated (Cas) genetic scissors (Jinek et al., 2012). In less than a decade afterward, CRISPR/Cas-based techniques have been successfully used as powerful and efficient tools for genome-editing in various species because of their simplicity, efficiency, and versatility (Glass et al., 2018; Chen et al., 2019; Manghwar et al., 2019; Wang et al., 2019a, 2019b). In addition to indel mutations induced by the CRISPR/Cas nuclease, several CRISPR/Cas-derived editors have been designed as well that can perform precise genome manipulations, such as the base and prime editors (Kim et al., 2017; Koblan et al., 2018; Anzalone et al., 2019; Richter et al., 2020). These game-changing tools, therefore, offer tremendous opportunities to decipher gene function and engineer beneficial traits through gene knockout, knockin, replacement, point mutations, fine-tuning of gene regulation, and other modifications at any gene locus in many biological systems (Barrangou and Doudna, 2016; Knott and Doudna, 2018). However, such manipulations are conducted in a low-throughput; gene-by-gene manner. Hence, it has been proposed that since the spacer sequence is the sole determinant of programmable gene-editing regions, CRISPR/Cas-based platforms can easily be scaled up to use guide RNA (gRNA) pools (Wang et al., 2014; Zhu et al., 2020). The idea is, by introducing large-scale pooled gRNA libraries that can target all the genes of a specific organism, the construction of high-throughput mutant libraries in bacteria (Garst et al., 2017), yeasts (Bao et al., 2018), and mammalian cell lines (Shalem et al., 2014) would be enabled. Moreover, by using deactivated Cas9 protein (dCas), high-throughput transcriptional activation (CRISPRa; Konermann et al., 2015; Bester et al., 2018), and interference (CRISPRi; Liu et al., 2017a, 2017b; Wang et al., 2018) methods in various hosts, studies have demonstrated this possibility. Furthermore, with the construction of genome-wide mutant libraries and the development of large-scale genetic screening techniques, CRISPR-based editing systems have recently transitioned to the high-throughput era (Shalem et al., 2015; Lu et al., 2017; Meng et al., 2017). However, in contrast to small-scale gene-editing experiments, high-throughput genome-editing methods that can be used to create libraries of genetic variants, covering all possible genes in a single experiment, provide a promising strategy for high-throughput functional genomics (Hsu et al., 2014; Shalem et al., 2015; Lian et al., 2018). High-throughput genome-editing technologies have thus been widely used during various biological screens in animal cell systems. For example, drug or toxin-resistance screens (Koike-Yusa et al., 2014; Zhou et al., 2014; Virreira Winter et al., 2016), drug target discovery (Shalem et al., 2015; Behan et al., 2019), screens related to viral infectivity (Zhang et al., 2016; Park et al., 2017a, 2017b), screens for essential genes in fitness (Wang et al., 2015, 2017a, 2017b), or T-cell mediated cancer immunotherapy screens (Manguso et al., 2017; Patel et al., 2017; Pan et al., 2018). However, compared to animal cell systems, the application of high-throughput genome-editing technologies in plants is still in its infancy, as only a few pooled CRISPR mutant collections have been reported in rice (Oryza sativa L.; Lu et al., 2017; Meng et al., 2017), tomato (Solanum lycopersicum; Jacobs et al., 2017), soybean (Glycine max (L.) Merr.; Bai et al., 2020), and maize (Zea mays; Liu et al., 2020a, 2020b). Additionally, saturation mutagenesis of crucial functional genes through CRISPR-mediated base editors has driven the implementation of directed evolution and the development of high-throughput protein engineering (Butt et al., 2019; Kuang et al., 2020; Kweon et al., 2020; Li et al., 2020; Liu et al., 2020a, 2020b). Therefore, we anticipate that high-throughput genome-editing technologies will greatly expand our understanding of gene function, with wide applications ranging from improved medications using gene therapy to crop improvement in the coming years.
Hence, on the basis of the various developments highlighted, we describe the recent research progress in using high-throughput genome-editing technologies in animals and plants in this review, including high-throughput design of targeted gRNA, construction of large-scale pooled gRNA and high-throughput genome-editing libraries, high-throughput detection of editing events, and high-throughput supervision of genome-editing products.
High-throughput designs for targeting gRNA
The targeting effect of the CRISPR/Cas system is mainly realized through Cas endonucleases and a short sequence known as a protospacer-adjacent motif (PAM) near the target DNA site. The Cas protein is directed to its genomic destination using a single gRNA, which is the key for CRISPR/Cas systems to recognize the target site in the genome, and its complementary sequence at the target site. Therefore, the specific PAM sequence limits the choice of target site, whose sequence characteristics vary depending on the Cas nuclease. Nevertheless, the universality of specific Cas nuclease target sites in the genome depends on the distribution of PAM sequences.
The design of targeted gRNA is one of the key steps for successful gene-editing using the CRISPR/Cas system. Mostly, the design of gRNA is mainly based on scanning the genome sequence to determine whether a suitable PAM sequence near the target site exists. However, since thousands of gRNAs need to be designed during high-throughput gene-editing, the quality of gRNA designs directly influences the quality of the CRISPR/Cas libraries and subsequent editing efficiency. Thus, as the number of gRNAs increases, the size of the necessary population also increases accordingly. Nevertheless, having more active gRNAs improves the efficiency of high-throughput editing and reduces the size of the required population. During high-throughput gene-editing, in order to generate loss-of-function mutations efficiently, two to the three gRNAs per gene should be designed and synthesized, and gRNA target site should be selected in exons near the beginning of ORFs just downstream of start codons (Gaillochet et al., 2021; Liu et al., 2020a, 2020b; Lu et al., 2017). In addition to the number and position, the design of gRNAs should also consider the following factors.
Rules of gRNA design
Based on mammalian cell systems, David E. Root’s laboratory successively proposed gRNA design rules:
In Rule Set 1, Doench et al. examined the activity of all possible target sites (1,841 gRNAs) for 6 mice and three human genes to determine sequence features within and surrounding their target sites that improved activity, thereby constructing a predictive on-target efficacy model for gRNAs (Rule Set 1). The efficiency score for Rule Set 1 was in the range of 0–1, where the higher-scoring gRNAs are more effective (Doench et al., 2014). Immediately afterward, by doubling the size of the gRNA activity data set and uncovering several factors contributing to gRNA activity and specificity, such as counts of position-independent nucleotide, the location of target sites within the protein, and melting temperature, Doench et al. updated and improved gRNA design rules on their original version (Rule Set 1), and developed Rule Set 2, which gave a demonstrably improved performance versus the Rule Set 1 (Doench et al., 2016). Nevertheless, the two gRNA design rules are both aimed at refining gRNA designs by maximizing the on-target activity and minimizing off-target effects of gRNA.
Selecting appropriate gRNA design tools for different systems
Following the establishment of the gRNA design rules, many algorithm models and calculation tools have been developed for the gRNA design rules to serve in the research and application of high-throughput genome editing (Figure 1). To date, more than 30 web-based design tools for gRNA exist (Graham and Root, 2015; Chuai et al., 2017; Cui et al., 2018; Hanna and Doench, 2020). Several of the more widely used web tools (Table 1), such as gRNA-designer (Doench et al., 2016), CHOPCHOP version 2 (Labun et al., 2016), CRISPOR version 2 (Haeussler et al., 2016), E-CRISP (Heigwer et al., 2014), CRISPR Library Designer (Heigwer et al., 2016), GuideScan (Perez et al., 2017), Guide Picker (Hough et al., 2017), and GUIDES (Meier et al., 2017), have been recommended for highly efficient gRNA design with optimal prediction performances. However, it is worth highlighting that nearly all gRNA activity predictive tools reported thus far were based on data from experiments in mammalian cell systems, thereby providing a great predictive accuracy for gRNA design in animals but not in plants and other systems. Recently, Naim et al. examined the predictive uniformity and performance of eight different online gRNA-site tools in plants, and observed little consensus or predictive accuracy (Naim et al., 2020). This finding suggested that the performance of predictive highly active gRNAs using mammalian cell systems is not fully applicable to other experimental systems. Hence, key factors affecting gRNA activity and specificity need to be uncovered and incorporated into gRNA prediction tools to increase their predictive power. When conducting genome-editing experiments on a specific species system, appropriate gRNA design tools should be selected first. Several gRNA design tools suitable for specific species have therefore been developed (Table 1), such as CRISPR-P version 2.0 for plant (Liu et al., 2017a, 2017b), flyCRISPR for Drosophila (Gratz et al., 2014), and EuPaGDT for pathogens (Peng and Tarleton, 2015). However, these species-specific tools are far from satisfactory. Thus, continuing efforts need to be made to optimize gRNA design. Machine and deep learning processes, which have greatly promoted the efficiency of gRNA designs for mammalian cell systems (Moreno-Mateos et al., 2015; Wong et al., 2015; Doench et al., 2016; Chari et al., 2017; Rahman and Rahman, 2017; Chuai et al., 2018; Listgarten et al., 2018; Kim et al., 2019a, 2019b; Wang et al., 2019; Arbab et al., 2020; Kim et al., 2020; Muhammad Rafid et al., 2020; Xiang et al., 2021), have also been used to improve the predictability of gRNA designs for other systems. Additionally, for species without reference genomes, CRISPR-local provides the possibilities for designing high-throughput gRNAs (Sun et al., 2019; Table 1).
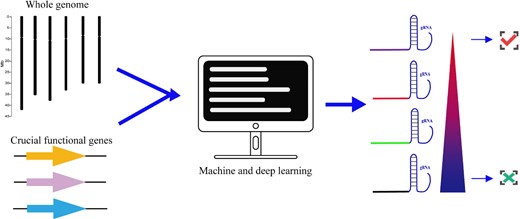
High-throughput design of gRNAs. Machine and deep learning methods are used for high-throughput design of gRNA for the whole genome or crucial functional genes. The gRNA at the apex of the pyramid is the best and is selected, whereas the gRNA at the root of the pyramid is the worst and is therefore discarded.
Predicting off-target effects of gRNA
Off-target activity is a perennial problem in gene-editing, which is proposed to cause severe problems for host organisms, potentially limiting the widespread application of genome-editing for therapeutic purposes. It is therefore vital to design an optimum gRNA with little or no off-target effects to achieve high on-targeting. To date, no tool exists that predicts off-target sites with high accuracy. The possible reasons are: (1) off-target genome-editing training data compared to that of the on-target benchmark is scarce and (2) the use of sequence alignments with mismatch counts to exhaustively search for off-targets is tedious (Chuai et al., 2017; Cui et al., 2018). Therefore, to improve off-target prediction accuracy, many promising approaches are being developed to reduce possible off-target activities, such as using the high fidelity Cas9 variant, increasing the specificity of nuclease-mediated target site cleavage, and decreasing the duration of nuclease expression to minimize the opportunity of accumulating off-target mutations (Tsai and Joung, 2016). Based on these strategies, various algorithms/tools have been developed to find potential off-target regions of a given gRNA sequence (Table 1), such as the CRISPRitz (Cancellieri et al., 2020), CRISPR-PLANT version 2 (Minkenberg et al., 2019), PEM-seq (Yin et al., 2019a, 2019b), GuideScan (Perez et al., 2017), CRISPOR version 2 (Labun et al., 2016), CRISPRscan (Moreno-Mateos et al., 2015), CCTop (Stemmer et al., 2015), CROP-IT (Singh et al., 2015), CASPER (Mendoza and Trinh, 2018), and CT-Finder (ZHu et al., 2016). Using these tools, off-target mutations can be reduced by selecting specific gRNAs whose predicted off-target probability is negligible. However, continuing efforts are still needed to further increase the on-target specificity and mitigate the off-target effects. It has also been revealed that off-target prediction and algorithm models in these tools have instructive values in the subsequent gRNA optimization design.
Construction of large-scale gRNA libraries
Choice of editing tool
In less than a decade, an increasing number of precise and versatile tools have been engineered and evolved for genome-editing, such as Cas9 and Cas12 nuclease variants, which have broadened targeting scope and specificity, base editor that precisely installs point mutations without requiring double-stranded DNA breaks or donor DNA templates, and prime editors that directly copy edited sequences into target DNA sites in a manner that replaces the original DNA sequence (Pickar-Oliver and Gersbach, 2019; Anzalone et al., 2020). The optimal choice of CRISPR/Cas tools and the overall editing strategy, however, depend on the intended application. In addition, editing efficiency is also a key factor that should be considered for large-scale gene editing. It determines the size of the genome-editing libraries. Thus, when targeting most genomes for simple knockouts, although the recognition of the PAM 5′-NGG limits the availability of SpCas9 in the genome, SpCas9 remains the most widely used genome-editing tool (Pickar-Oliver and Gersbach, 2019). Yet, in many cases, a single or few nucleotide mutations in the genome can cause human genetic diseases and crop agronomic traits. Therefore, when correcting disease-driving mutations, saturating key sites, or key amino acids of important genes, base editors, and prime editors are proposed to be the best options (Komor et al., 2016; Gaudelli et al., 2017; Anzalone et al., 2019; Xu et al., 2021). In addition to modifying genomic DNA sequences, CRISPR can be used as well to regulate the expression of a target gene (Gilbert et al., 2014; Konermann et al., 2015). Nevertheless, in the case of transcriptional modulation screens using CRISPRa or CRISPRi, the CRISPR/Cas toolbox that fused dCas9 with diverse effectors, such as transcription repressors or activators is a better choice (Manghwar et al., 2019; Pickar-Oliver and Gersbach, 2019).
Construction of gRNA libraries
The construction of larger-scaled gRNA libraries begins by following the design and selection of highly active gRNAs (Figure 2). To begin, gRNAs are synthesized into a pool of DNA oligonucleotides. For the past few decades, the array-based large-scale oligo-pool synthesis technology, which is synthesized in parallel on the surface of silica, has gradually developed into a higher throughput method, thereby making this process increasingly popular as a cheap source of designed oligos. Hence, it is the primary choice for most commercial oligo-synthesizers (Kosuri and Church, 2014; Song et al., 2021). These oligo-pools generated through array-based synthesis are the least expensive per gRNA, with cost per nucleotide being between $0.00001 and $0.001 (Kosuri and Church, 2014). Synthetic gRNAs have therefore been appended with common adaptor sequences and amplified through polymerase chain reaction (PCR) to generate sufficient clone-starting materials, which are then cloned into expression vectors using several methods, such as restriction enzyme (RE) ligation, Gibson’s assembly, or homologous recombination. After cloning, the quality of the library needs to be evaluated. The high uniformity, coverage, and accuracy of gRNAs are three key factors for the success of library construction. Hence, to quantify gRNA’s abundance in the cloned library, since only gRNA sequences differ between vectors, primers from flanking sequences are used to amplify these gRNA sequences for next-generation sequencing (NGS) to evaluate the relative presence of different gRNAs. The distribution of gRNAs in a library is assessed through the analysis of the relative abundance of each gRNA using a histogram (Joung et al., 2017). gRNAs should normally be distributed rather than over-enriched in individual gRNA libraries (Doench et al., 2014; Gaillochet et al., 2021), all target genes should be represented by at least one gRNA in the gRNA libraries. Furthermore, in addition to checking whether the gRNAs are highly covered and evenly mixed, it is also necessary to examine the accuracy of the synthesized gRNA sequence. Subsequently, after all quality control measures have been met, the gRNA libraries are ready to be transformed into cells or tissues.
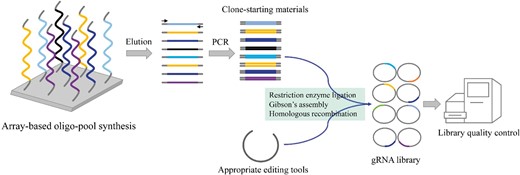
Construction of high-throughput gRNA libraries. gRNAs are synthesized via the array-based oligo-pool synthesis technology. Synthetic gRNAs need to be eluted, and amplified by PCR to generate sufficient clone-starting materials, which are then cloned into appropriate editing vectors by several methods, such as RE ligation, Gibson’s assembly, or homologous recombination. The constructed gRNA library requires quality control to evaluate the uniformity and coverage of gRNA.
Construction of high-throughput genome-editing libraries
Generation of high-throughput editing collections of animals
The generation of animal high-throughput editing libraries requires the use of lentiviral vectors as a medium (Figure 3). Recently, lentiviral vectors have been widely used to deliver CRISPR into target cells of interest (Zuckermann et al., 2015; O'Rourke et al., 2017; Walter et al., 2017; Rogers et al., 2018). The reason for using the lentiviral vector is that lentiviral vectors enable the stable expression of Cas9 and gRNA and can therefore enable efficient gene-editing in transduced cells in vivo (Rogers et al., 2017; Roper et al., 2017; Yin et al., 2019a, 2019b). After the construction of these gRNA libraries is completed, they are packaged together in a lentiviral vector, and further packaged into a lentivirus mixture. This lentivirus mixture is subsequently used to transfect cell lines that can stably express the Cas9 protein. By adjusting the ratio of cell and lentivirus titration, one lentivirus molecule can theoretically infect one cell. Next, after the lentivirus has accurately transfected the cell, it can be integrated into the genome of cell lines. At this point, an individual cell in the cell population can be transfected with different gRNAs. Then, when each cell carries only one gRNA, a cell collection containing all gRNA libraries can be generated. The transfected cells can therefore grow steadily for a certain time, allowing gRNAs and Cas9 proteins in each cell to do their job of editing the corresponding genes. Afterward, the whole population would comprise cells with different edited genes. On this basis, positive or negative selection approaches are used to screen cells of interest. Both positive and negative screening exerts certain screening pressure on the integrated gRNA library. The difference lies in the fact that with positive screening, only a few cells with target phenotypes can survive and achieve the purpose of gene enrichment. Enriched genes can then be applied to the screening of drug resistance and therapeutic targets (Dong et al., 2019; Hinze et al., 2019; Ye et al., 2019), whereas cells that survive during negative screening become unsuitable candidates for target phenotype cells. It is therefore necessary to compare the abundance of gRNA at different periods to obtain the missing gRNAs, and determine key genes, which can then be used to study cell growth and search for tumor-related genes (Chen et al., 2015; Beuter et al., 2018). Methods used to create selective pressure can be alternative culturing conditions (Rotem et al., 2015; Jain et al., 2016; Doench, 2018), small molecules (Johannessen et al., 2013; Vecchione et al., 2016; Wang et al., 2017a, 2017b), or infectious agents (Ma et al., 2015; Marceau et al., 2016; Orchard et al., 2016; Zhang et al., 2016; Doench, 2018).
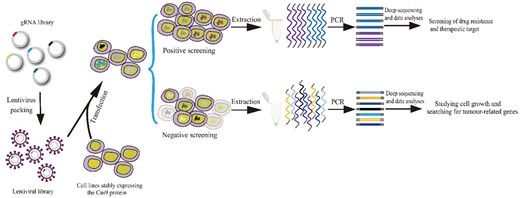
Construction of high-throughput genome-editing libraries of animals. The constructed gRNA libraries are packaged together into the lentiviral vectors, forming a lentiviral library. The lentivirus library is subsequently used to transfect cell lines that can stably express the Cas9 protein. On this basis, positive or negative selection approaches are used to screen cells of interest. With positive screening, only a few cells with target phenotypes can survive and achieve the purpose of gene enrichment. Enriched gRNA-coding fragments are PCR-amplified, and they are subsequently decoded through deep sequencing and data analysis to screen the drug resistance and therapeutic targets. Whereas cells that survive in the negative screening are not the target phenotype cells. It is therefore necessary to compare the abundance of gRNA at different periods to obtain the missing gRNAs by deep sequencing and data analysis, which can then be used to study cell growth and search for tumor-related genes.
Generation of high-throughput mutant libraries of plants
In contrast to mammalian cell systems, various transformation options are available for delivering pooled gRNA libraries into plant cells (Figure 4), such as electroporation, biolistic, and polyethylene glycol- or Agrobacterium-mediated transformation, of which the Agrobacterium-mediated transformation is thus far the most used. The main advantages of this approach are that they have (1) relatively simple operations; (2) high transformation frequencies; (3) more stable inheritance patterns, most of which conform to the laws of Mendelian’s inheritance; and (4) low copy number of imported genes, which ranges from only one or a few transfer DNAs (T-DNAs) in an individual plant cell in most cases (Depicker et al., 1985). Therefore, if pooled gRNA libraries are transformed into plant cells, theoretically, roughly 80% of transgenic events will contain one T-DNA, while the rest will contain two, three, or more different T-DNAs (Gaillochet et al., 2021). This pattern was observed in several reports (Jacobs et al., 2017; Bai et al., 2020; Kuang et al., 2020; Gaillochet et al., 2021). Additionally, even without T-DNA integration, there will be some unintended editing events owing to the presence of transiently expressed gRNAs, which increases the mutagenesis rate within the population (Chen et al., 2021; Gaillochet et al., 2021; Jacobs et al., 2017). To date, only a few pooled CRISPR mutant collections via Agrobacterium-mediated transformation have been reported in rice (Chen et al., 2021; Kuang et al., 2020; Li et al., 2020; Lu et al., 2017; Meng et al., 2017), tomato (Jacobs et al., 2017), soybean (Bai et al., 2020), and maize (Liu et al., 2020a, 2020b). These applications of large-scale CRISPR screens in plants mainly focus on generating knockout mutant collections to test gene functions, and saturation mutagenesis of crucial functional genes to drive the implementation of directed evolution and the development of high-throughput protein engineering. In addition, producing large numbers of transgenic plants is crucial when constructing high-throughput mutant libraries of plants. Several studies have shown that the minimum number of T0 plants is determined to be two to four times the number of target genes to achieve almost complete coverage of the candidate genes (Lu et al., 2017; Liu et al., 2020a, 2020b; Gaillochet et al., 2021). For the species highly amenable to transformation, such as rice or tomato, it is not difficult to produce enough transgenic plants (Jacobs et al., 2017; Lu et al., 2017; Meng et al., 2017). However, for wheat (Triticum aestivum), maize, or other species recalcitrant to transformation and tissue cultures, insufficient capacity exists to generate large transgenic collections. Therefore, there are only a few related reports in addition to a tour de force study in which over 4,000 independent transgenic maize events were generated (Liu et al., 2020a, 2020b). Thus, the improvement of plant genetic transformation or regeneration capability can allow CRISPR editing populations to be scaled up.

Construction of high-throughput genome-editing libraries of plants. In plants, various transformation options are available for delivering pooled gRNA libraries into plant cell nucleus, such as electroporation, particle bombardment, Agrobacterium- or nanoparticle-mediated transformation, the gene-editing components subsequently target and edit the genome in the nucleus. As a result, large numbers of transgenic plants are generated in high-throughput genome-editing libraries.
High-throughput detection of editing events
CRISPR/Cas-based techniques have been successfully used as efficient tools for genome-editing in various species because of their simplicity, efficiency, and versatility (Anzalone et al., 2020; Zhu et al., 2020). Detecting CRISPR/Cas-mediated genome-editing effects, including targeting efficiency and off-target effect, is therefore key in evaluating the success of genome-editing experiments (Figure 5). For high-throughput detection, although many analytical tools developed so far via NGS have achieved on-target and off-target detection, there are still shortcomings, and it is still necessary to develop simpler and more efficient detection methods and tools in the future.
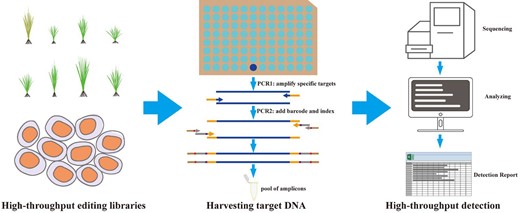
Schematic illustration of the workflow for high-throughput genome-editing detection. First, the samples are extracted from the high-throughput genome-editing library. Second, the specific targets are amplified using site-specific primers. The first-round PCR products are used in the second-round PCR in combination with barcode and index primer pairs. Amplicons are pooled and sequenced by NGS. Third, sequencing results are analyzed with high-throughput methods or tools.
On-target detection
Several traditional methods have been undertaken for genome-editing detection, including the PCR/RE (Shan et al., 2013, 2014), T7 endonuclease I (T7EI; Li et al., 2013; Xie and Yang, 2013), Surveyor nuclease (Cong et al., 2013), polyacrylamide gel electrophoresis (PAGE)-based genotyping (Zhu et al., 2014), and high-resolution melting (HRM) analysis-based assays (Montgomery et al., 2007), annealing at critical temperature PCR (ACT-PCR; Hua et al., 2017) and directly analyzing the Sanger sequencing results of PCR-amplified target regions (Brinkman et al., 2014; Xie et al., 2017), to name a few. However, some drawbacks exist, including the limited RE sites available near the target sequences for PCR/RE assays, the low detection sensitivity of T7EI and Surveyor nuclease assays, the false-positive results that occur during the detection of single-nucleotide polymorphisms or allelic mutations for PAGE-based method, the expensive equipment required for HRM analysis-based approaches, the harsh annealing temperature needed for ACT-PCR assays, and tedious decoding processes for Sanger sequencing. Moreover, during genotyping large-scale screening events like cell transfection, the above conventional methods are time- and labor-consuming, expensive, and low-throughput. Nevertheless, in recent decades, NGS technologies have developed rapidly in terms of speed, cost, and throughput, thereby bringing biology to a new era (Mardis, 2011; Goodwin et al., 2016). With NGS, millions of individual sequencing reactions can be conducted simultaneously in parallel, which generates large quantities of valuable sequence information (Metzker, 2010; Goodwin et al., 2016). Therefore, many analysis tools have been developed so far via NGS to track mutation patterns induced through genome-editing (Table 1). For example, CRISPR genome analyzer (CRISPR-GA) (Güell et al., 2014), CRISPR-AnalyzeR for pooled screens (caRpools) (Winter et al., 2016), CRISPResso (Pinello et al., 2016), Cas-Analyser (Park et al., 2017a, 2017b), CrispRVariants (Lindsay et al., 2016), MAGeCK-VISPR (Li et al., 2015), platform-independent analysis of pooled screens using python (PinAPL-Py) (Spahn et al., 2017), CRISPRCloud2 (Jeong et al., 2019), CRISPRAnalyzeR (Winter et al., 2017), and analysis of genome editing by sequencing (AGEseq) (Xue and Tsai, 2015), in addition to uni-directional targeted sequencing (UDiTaS) (Giannoukos et al., 2018), enables the analysis of multiple target sites comprising few samples. However, when analyzing large numbers of samples, these tools are proposed to be inefficient. Nevertheless, the advent of high-throughput tracking of mutations (Hi-TOM) and CRIS.py tools solves this dilemma (Table 1). Hi-TOM is a user-friendly web tool, which is used to track various mutations with precise percentage and high sensitivity for multiple samples and multiple target sites, thereby facilitating the application of NGS in tracking all types of mutations induced by CRISPR/Cas systems (Liu et al., 2019). Likewise, CRIS.py has a similar function to Hi-TOM (Connelly and Pruett-Miller, 2019).
Off-target detection
Although designed for site-specific cleavage, Cas nuclease-mediated cleavage is proposed to occur at other genomic sites, known as off-target sites. However, since off-target cleavage processes can produce undesired effects that confuse the analysis of the phenotype of interest, it remains necessary to screen for unintended changes in the genome, even after confirming on-target mutations. Off-target cleavage processes occur at sites of sequence homology to the on-target site. The simplest detection method is to amplify preselected potential off-target sites, followed by sequencing of the PCR products using Sanger or NGS procedures (Zischewski et al., 2017). The greatest drawback of amplification and sequencing of preselected off-target sites is that not every algorithm searches for every off-target, as some potential off-target sites can be ignored, which is termed biased nature. Therefore, while the unbiased detection of off-target mutations requires whole-genome sequencing, which is expensive and can only be used in relatively few samples, the whole-genome sequencing approach can still be used to screen for off-target mutations induced by genome-editing in various organisms (Ghorbal et al., 2014; Yang et al., 2014; Zhang et al., 2014; Iyer et al., 2015; Li et al., 2019a, 2019b; Wang et al., 2021). The confinement of whole-genome sequences to few samples also implies that most low-frequency off-target events are missed (Zischewski et al., 2017). Other high-throughput methods or tools have thus been developed specifically to detect off-target mutations (Table 1), which include GUIDE-seq (Tsai et al., 2015), LAM-HTGTS (Hu et al., 2016), Digenome-seq (Kim et al., 2015), DISCOVER-Seq (Wienert et al., 2019), OffScan (Cui et al., 2020), CRISPRitz (Cancellieri et al., 2020). However, in gene therapy and other applications that require absolute fidelity, existing off-target detection methods are still not sensitive enough, thereby requiring the development of more sensitive off-target detection methods.
High-throughput supervision of genome-editing products
During genome editing, DNA cassettes encoding editing components are delivered and integrated into the cells of host genomes. After the genetic cargo enters the target cells, target sequences will be modified to ultimately produce genome-editing products (Cong et al., 2013; Zhang et al., 2018). The integration of these exogenous components is random and can produce undesirable genetic changes. Even if DNA cassettes are degraded, resulting fragments are also proposed to be integrated and have adverse effects (Kim et al., 2014). Moreover, all unwanted introductions of foreign DNA into genomes raise safety problems and regulatory concerns for genome-edited organisms (Carroll et al., 2016; Li et al., 2019a, 2019b), such as genome-edited hornless calves’ event (Young et al., 2020). Therefore, with the widespread application of genome-editing technologies, how to supervise genome-editing products has become an urgent issue. Countries worldwide have not yet formed a unified consensus on the supervision of genome-editing products as well (Waltz, 2016; Callaway, 2018; Metje-Sprink et al., 2018; Halford, 2019; Schmidt et al., 2020). However, the prerequisite for the safe supervision of genome-editing products is to establish identification and accurate quantitative detection technologies for monitoring genome-editing products. Therefore, although many methods have been developed so far to detect the integration of foreign elements in genome-editing products, among which PCR or PCR-based methods are the most widely used for detecting these foreign elements (Dörries et al., 2010; Perez et al., 2013), specific primers need to be designed on the given exogenous elements for detection by PCR. PCR-based strategies have also been proposed to give false negatives if exogenous elements are fragmented or mutated (Skryabin et al., 2020). Hence, to improve the sensitivity and accuracy of detection, several tools for detecting integrated foreign elements within the host genome via whole-genome sequencing have been developed (Table 1). Notably, both Liu et al. (2021) and Tay et al. (2021) elucidated that foreign DNA sequences can be identified with great sensitivity and accuracy by using data derived from whole-genome sequencing, thereby allowing for enhanced monitoring of emerging biosecurity threats. Thus, with the use of foreign element detection tools, genome-editing technology will have broader application prospects in the future.
Conclusions and prospects
During the last decade, the precipitous development in CRISPR/Cas-based genome-editing techniques has been spectacular, due to the versatile, precise, and high-throughput refinement of genome-engineering tools. These high-throughput-based versatile tools have revolutionized the life sciences, which enable advances in basic and applied research of many biological systems. Progress has therefore been made in creating high-throughput genome-editing libraries and saturation mutagenesis tools of crucial functional genes that correspond to high-throughput detection and supervision. However, several challenges remain.
First, specificity is a major concern in complex eukaryotic organisms. For clinical applications, specificity is a key safety consideration, as off-target mutagenesis poses a risk of oncogenic transformation in genetically engineered cells (Gupta et al., 2019). As described in this review, many laboratories have contributed to the development of algorithms/tools to profile potential genome-wide CRISPR off-target sites. However, although all off-target predictive algorithms/tools reported thus far have been based on animal cell models, their predictive accuracy in plants is still unclear (Gaillochet et al., 2021). Additionally, high-throughput sequencing error rates limit the sensitivity of off-target detection, which is in the range of 0.01%–1% (Kim et al., 2019a, 2019b). Therefore, current off-target detection algorithms/tools are insufficient to identify off-target sites with extremely low mutation frequencies, which show the need for further improvements in off-target detection methods.
Second, although high-throughput genome-editing technologies have shown immense potential in targeted genome-editing, these tools are still a long way from being applied therapeutically. Hence, while CRISPR/Cas has been applied to mice models, which are an excellent model for studying potential therapies, it has low efficacy and is prone to complications (Long et al., 2016; El Refaey et al., 2017). Furthermore, apart from its off-target effect, the delivery technology remains a bottleneck that is awaiting major breakthroughs. Therefore, various strategies employed to deliver gene-editing plasmid constructs can mainly be categorized as viral and nonviral delivery methods. Notably, vehicles used for therapeutic approaches are virus-mediated (Gupta et al., 2019). Lentivirus is currently among the most widely used delivery vehicles for gene therapy, but it also has shortcomings, the most serious of which is the uncertainty of safety. Thus, since lentiviral vectors cannot insert transgene into specific sites, nonspecific and adverse effects are proposed to occur (Zheng et al., 2018). Nevertheless, continuous efforts to improve and revolutionize the delivery vehicle allow for safe and efficient transport to target cells, which advances their capabilities to edit. These advances will therefore ensure effective CRISPR/Cas systems for many therapeutic applications in the near future.
Third, as for directly transforming some exogenous DNA cassettes into plants, which are proposed to be problematic, especially for wheat, maize, or other species recalcitrant to transformation and tissue culture, developing additional novel delivery methods would be desirable. Hence, protoplast-mediated transfection has attracted much attention in plants due to the possibility of easily providing a large source of cells without cell walls from several plant species. Nevertheless, plant protoplast systems are frequently used in genome-editing transient assays (Bargmann et al., 2013; Bahariah et al., 2021). Yet, no reports on high-throughput genome-editing currently exist using plant protoplast systems. Plant cell suspension cultures provide an alternative that has similar characteristics to the lentivirus delivery in mammalian cell cultures (Moscatiello et al., 2013; Santos et al., 2016). Furthermore, cell suspensions can be transformed stably with Agrobacterium, allowing pooled vector libraries to be transformed, propagated, and potentially selected. However, nanoparticle-mediated delivery vehicles are promising because they can diffuse into walled plant cells without mechanical aid and without causing tissue damage (Demirer et al., 2019; Kwak et al., 2019; Zhang et al., 2019; Santana et al., 2020; Zhu et al., 2020). Thus, ongoing research focused on delivering systems should make high-throughput gene-editing technology better applications in plants.
All in all, the CRISPR/Cas technology, combined with developing NGS tools and many other high-throughput techniques, has undoubtedly revolutionized, and will continue to revolutionize life science.
CRISPR/Cas-based genome editing techniques have revolutionized the biotechnology field and offered tremendous opportunities to decipher gene function and engineer beneficial traits in a wide range of biological systems.
CRISPR/Cas systems combined with the development of NGS and many other high-throughput technologies have brought gene editing into a high-throughput era.
Significant advances have been made in the creation of high-throughput genome editing libraries and saturation mutagenesis of crucial functional genes, and corresponding high-throughput detection and supervision.
Compared to animal cell systems, the application of high-throughput genome editing technologies in plants is still in its infancy; however, advances in animal cell systems can guide the application of high-throughput genome editing technologies in plants.
How can we further refine gRNA design by maximizing on-target activity and minimizing off-target effects of gRNA?
What are the intrinsic off-target mechanisms, and how can we develop more sensitive algorithms/tools of off-target prediction and detection for gene therapy and other applications that require absolute fidelity?
How can we improve and revolutionize the delivery vehicle allowing for safe, efficient transport to the target cells, and advancing their capabilities to edit in species recalcitrant to transformation and tissue culture?
What is the best way to use and implement high-throughput gene-editing technologies in a research program?
K.W. conceived and designed this manuscript. Y.H. wrote the initial manuscript. M.S. and T.L. assisted with drawing the figures. K.W. revised the manuscript, and all authors read and approved the manuscript.
The author responsible for distribution of materials integral to the findings presented in this article in accordance with the policy described in the Instructions for Authors (https://dbpia.nl.go.kr/plphys/pages/general-instructions) is Kejian Wang ([email protected]).
Acknowledgments
We thank all members of the Wang lab for discussions. We apologize to colleagues whose work could not be included due to space limitations.
Funding
This work was supported by grants from the National Natural Science Foundation of China (32025028, U20A2030, and 32188102), Central Public-interest Scientific Institution Basal Research Fund (Y2020XK17), the Agricultural Science and Technology Innovation Program (CAAS-ZDRW202001), the Fundamental Research Funds for Central Nonprofit Scientific Institution (Y2020YJ12), China National Rice Research Institute Key Research and Development Project (CNRRI-2020-01), and the earmarked fund for China Agriculture Research System.
Conflict of interest statement. The authors declare no conflict of interest.
References
Author notes
Senior author