-
PDF
- Split View
-
Views
-
Cite
Cite
Liying Zhou, Qing Li, Jingshen Xu, Shuaikang Wang, Zhiqiang Song, Xinyi Chen, Yan Ma, Zhiguang Lin, Bobin Chen, He Huang, Cerebrospinal fluid metabolic markers predict prognosis behavior of primary central nervous system lymphoma with high-dose methotrexate-based chemotherapeutic treatment, Neuro-Oncology Advances, Volume 5, Issue 1, January-December 2023, vdac181, https://doi.org/10.1093/noajnl/vdac181
- Share Icon Share
Abstract
Primary central nervous system lymphoma (PCNSL) is a highly aggressive non-Hodgkin’s B-cell lymphoma which normally treated by high-dose methotrexate (HD-MTX)-based chemotherapy. However, such treatment cannot always guarantee a good prognosis (GP) outcome while suffering several side effects. Thus, biomarkers or biomarker-based models that can predict PCNSL patient prognosis would be beneficial.
We first collected 48 patients with PCNSL and applied HPLC-MS/MS-based metabolomic analysis on such retrospective PCNSL patient samples. We then selected the highly dysregulated metabolites to build a logical regression model that can distinguish the survival time length by a scoring standard. Finally, we validated the logical regression model on a 33-patient prospective PCNSL cohort.
Six metabolic features were selected from the cerebrospinal fluid (CSF) that can form a logical regression model to distinguish the patients with relatively GP (Z score ≤0.06) from the discovery cohort. We applied the metabolic marker-based model to a prospective recruited PCNSL patient cohort for further validation, and the model preformed nicely on such a validation cohort (AUC = 0.745).
We developed a logical regression model based on metabolic markers in CSF that can effectively predict PCNSL patient prognosis before the HD-MTX-based chemotherapy treatments.
Cerebrospinal fluid metabolites are associated with the prognostic outcome of PCNSL patients who received HD-MTX-based chemotherapy.
The prospective study validates the metabolic marker-based model for the prognostic outcome prediction before the HD-MTX-based treatment.
Our results provide an applicable model that predicts the prognosis of HD-MTX-based treatment using a scoring standard.
Primary central nervous system lymphoma (PCNSL) is a highly aggressive non-Hodgkin’s B-cell lymphoma that accounts for 3%–5% of all primary brain tumors. High-dose methotrexate (HD-MTX)-based chemotherapy serving as the first-line treatment causes strong adverse effects in patients. Although several clinical and basic types of research have investigated the way to predict the prognostic outcome of PCNSL patients before receiving the HD-MTX-based treatment, none of the biomarkers or prediction models were evaluated by a prospective PCNSL cohort due to the rare incidence rate of such disease. In this study, we applied metabolomics technique to discover a metabolic feature-based prediction model from a retrospective PCNSL cohort, and validated our model using a prospective PCNSL cohort. Our results indicated the prognosis of PCNSL patients with HD-MTX-based treatment would associate with CSF metabolism disorders.
Primary central nervous system lymphoma (PCNSL) is a highly aggressive non-Hodgkin’s B-cell lymphoma type with a median overall survival (OS) of around 4 years after diagnosis.1 It accounts for 3%–5% of all primary brain tumors2 and 4%–6% of all extranodal lymphomas.3 More than 90% of PCNSLs are diffuse large B-cell lymphomas (DLBCL), while the remaining ones are T-cell, Burkitt, lymphoblastic, and marginal zone lymphomas.2 The incidence of PCNSL has increased in recent years, particularly in patients over the age of 60 years, and is now at an overall rate of 0.5 per 100 000.3
There is no consensus on the optimal treatment regimen for PCNSL, but high-dose methotrexate-based chemotherapy is widely used. High-dose chemotherapy (HDC) followed by autologous stem cell transplantation (ASCT) is the most recent option for consolidation therapy.4 Despite advances in initial treatment, up to half of patients relapse, and 10% to 15% have primary refractory disease.5 The median overall survival has improved significantly from 2.5 months to 26 months over the past few decades,6 but the 5-year survival rate of PCNSL patients is still very low, especially in the elderly and those who cannot tolerate high-dose chemotherapy. Considering the strong toxicity of MTX, the high-dose methotrexate (HD-MTX)-based chemotherapy readily results in adverse effects in patients, including hepatorenal complications and hematological toxicities.7 Thus, finding of biomarkers and clinical indexes that can predict the prognostic outcome of HD-MTX-based treated patients would be beneficial.
Several clinical prognostic models were developed, including the International Extranodal Lymphoma Study Group (IELSG) score,8 the Nottingham-Barcelona score,9 and the Memorial Sloan-Kettering Cancer Center (MSKCC) classification of PCNSL.10 Although a variety of prognostic biomarkers of PCNSL have been reported by previous studies,1,11–18 it is difficult to comprehensively reflect the overall characteristics of prognosis with only a single or a few biomarkers. The anti-cancer target of MTX is dihydrofolate reductase (DHFR) which can convert dihydrofolate to tetrahydrofolate. The inhibition of DHFR also perturbs DNA synthesis and one-carbon metabolism.19,20 It is natural to hypothesize that metabolite alterations may be associated with HD-MTX-based chemotherapy outcomes, and metabolic molecular markers can be a type of promising biomarker to predict the efficacy of HD-MTX-based chemotherapy in PCNSL patients.
In this work, we first performed a retrospective cohort study to identify global changes in metabolites and screen biomarkers for prognosis prediction, followed by a prospective cohort study to validate our findings. By integrating clinical characteristics, therapeutic regimens, and metabolic markers, we identified a molecular model with a prognostic value that is helpful for risk stratification and management of PCNSL patients.
Methods
Patients
The discovery cohort consisted of 48 patients with PCNSL diagnosed from 2018 to 2020 at our institution. Diagnosis of all participants was confirmed based on the WHO classification of tumors of hematopoietic and lymphoid tissues. Patients would be excluded if they had systemic lymphoma and immunodeficiency disease. The validation cohort included 35 patients prospectively recruited from 2020 to 2021 with the same inclusion/exclusion criteria and sample processing protocol and followed up to July 2022. Two samples were removed due to clinical information inadequateness, leaving 33 patients. Informed consent was obtained from all patients for the use of their samples, in accordance with the guidelines of the respective Ethical Committees on Human Research approved at our institution.
All patients received an HD- MTX-based chemotherapy regimen. Each HD-MTX treatment was administered as a 3-h infusion. Pre-hydration and alkalinization were initiated at least 72 h before MTX administration. Standard leucovorin rescue was initiated 24-h after the start of HD-MTX infusion. Every 3 weeks, 8 cycles were repeated until tumor progression or toxicity occurred. The clinical features of all patients were collected from the medical records, including age, gender, height, weight, performance status, time of diagnosis, surgical resection, biopsy type, lesion site, number of lesions, HIV status, serum lactate dehydrogenase (LDH) level, etc. Magnetic resonance imaging (MRI) was used to assess the location and quantity of lesions in all patients. The continuity of treatment was evaluated by contrast-enhanced MRI scans every cycle and by PET-CT after 3 cycles of treatment and after all therapeutic procedures. PFS was calculated from the date of diagnosis to the date of disease progression, the first relapse, death from any cause, or the last follow-up.
Sample Collection
All patients underwent biopsy before CSF sampling and were diagnosed pathologically. Each PCNSL patient underwent a lumbar puncture to examine cerebrospinal fluid (CSF) at baseline before chemotherapy. An additional 2 mL of CSF was obtained, then centrifuged for 10 min to remove cells and frozen at −80°C until further use.
Metabolite Extraction
The metabolomic approach was adopted from a published method.21 Centrifuge at 14 000 × g for 10 min at 4°C. Methanol: water (v:v, 80:20) was prechilled at −80°C overnight, and 4.5 mL was added to 50 microliters of cerebrospinal fluid. Mix and incubate at −80°C for 30 min. Centrifuge at 4°C with 4000 × g for 10 min, and the supernatant was then collected in another 15 mL centrifuge tube. The 80% methanol extracted metabolites were then dried using a SpeedVac (LABCONCO Refrigerated CentriVap Concentrator) and stored at −80°C before MS analysis.
Targeted Metabolomics Analysis
The metabolomic approach was adopted from a published method.22 In general, samples were resuspended in 50 μL of water: acetonitrile (v:v, 50:50), and 5 μL was injected into a 6500 QTRAP triple-quadrupole MS (SCIEX) coupled to an HPLC system (Shimadzu). Metabolites were eluted via hydrophilic interaction chromatography (HILIC) by using a 4.6-mm i.d. × 10 cm Amide XBridge column (Waters) with a flow rate of 400 μL/min using buffer A (20 mM ammonium hydroxide/20 mM ammonium acetate (pH 9.2) at a 95:5 ratio with water: acetonitrile) and buffer B (acetonitrile). Gradients were run from 85% buffer B to 42% buffer B at 0–5 min; from 42% buffer B to 0% buffer B at 5–16 min; 0% buffer B was held from 16–24 min; from 0% buffer B to 85% buffer B at 24–25 min; and 85% buffer B was held for 7 min. All ions were acquired by 306 selected reaction monitoring transitions in a positive and negative mode-switching fashion. Electrospray ionization (ESI) voltage was +4,900 and −4,500 V in positive or negative mode, respectively. Data were analyzed by MetaboAnalyst software (https://www.metaboanalyst.ca/).
Data Processing and Model
All the CSF metabolomics data were first processed with Log10 transformed and normalized using median value using MetaboAnalyst software.23 The normalized data were then corrected batch effect by an R package; sva::ComBat.24,25 For the feature selection, we compared the mostly different metabolites in patient CSF before the HD-MTX-based chemotherapy treatment and ranked the detected metabolites based on their fold change (FC) between good prognosis (GP) and poor prognosis (PP) groups. Six features were selected due to their Log2FC > 0.5 or Log2FC < −0.5. For the prediction model, we applied a logistics regression model to the discovery cohort and test such a model on the validation cohort. Comparing the P-values of different models using survival analysis,26 we selected the best-performing logistics regression model as our prediction model.
Statistical Analyses
The patient’s baseline characteristics were summarized using descriptive statistics, and descriptive analyses were conducted for all variables. The Chi-square, Fisher’s exact test and Mann–Whitney tests were used for statistical analyses. Survival curves were plotted by the Kaplan–Meier method and analyzed by the log-rank test. All tests were 2-sided, and P < .05 was taken as statistically significant. All statistical analyses were performed using Statistical Package for Social Science, version 26.0 (IBM SPSS Statistics, Armonk, NY: IBM Corp.) and Graphpad Prism version 9.0.0 (Graphpad Software).
Results
Clinical Characteristics of PCNSL Patients
The basic characteristics of 2 sets of PCNSL patient cohorts are shown in Table 1, which is similar to that of the validation set. Among 48 PCNSL patients in the discovery set, 24 patients (50%) were males and 24 (50%) were females, and the validation set was 19 males vs 14 females. White blood cell (WBC) number and protein level in CSF were comparable between the 2 cohort sets.
The Clinical Characteristics of Discovery and Validation Primary Central Nervous System Lymphoma (PCNSL) Patient Cohort
. | Discovery cohort . | Validation cohort . | P . |
---|---|---|---|
. | n = 48 . | n = 33 . | . |
Gender, n(%) | .502 | ||
Male | 24(50%) | 19(58%) | |
Female | 24(50%) | 14(42%) | |
Age (years) | 56[50–66] | 62[50.5–67] | .600 |
Weight (Kg) | 68.521 | 62.606 | .124 |
BMI (kg/m2) | 22.929 | 18.868 | .551 |
Elevated CSF WBC count | 19/46 | 9 | .200 |
Elevated CSF protein level | 33 | 26 | .187 |
. | Discovery cohort . | Validation cohort . | P . |
---|---|---|---|
. | n = 48 . | n = 33 . | . |
Gender, n(%) | .502 | ||
Male | 24(50%) | 19(58%) | |
Female | 24(50%) | 14(42%) | |
Age (years) | 56[50–66] | 62[50.5–67] | .600 |
Weight (Kg) | 68.521 | 62.606 | .124 |
BMI (kg/m2) | 22.929 | 18.868 | .551 |
Elevated CSF WBC count | 19/46 | 9 | .200 |
Elevated CSF protein level | 33 | 26 | .187 |
The Clinical Characteristics of Discovery and Validation Primary Central Nervous System Lymphoma (PCNSL) Patient Cohort
. | Discovery cohort . | Validation cohort . | P . |
---|---|---|---|
. | n = 48 . | n = 33 . | . |
Gender, n(%) | .502 | ||
Male | 24(50%) | 19(58%) | |
Female | 24(50%) | 14(42%) | |
Age (years) | 56[50–66] | 62[50.5–67] | .600 |
Weight (Kg) | 68.521 | 62.606 | .124 |
BMI (kg/m2) | 22.929 | 18.868 | .551 |
Elevated CSF WBC count | 19/46 | 9 | .200 |
Elevated CSF protein level | 33 | 26 | .187 |
. | Discovery cohort . | Validation cohort . | P . |
---|---|---|---|
. | n = 48 . | n = 33 . | . |
Gender, n(%) | .502 | ||
Male | 24(50%) | 19(58%) | |
Female | 24(50%) | 14(42%) | |
Age (years) | 56[50–66] | 62[50.5–67] | .600 |
Weight (Kg) | 68.521 | 62.606 | .124 |
BMI (kg/m2) | 22.929 | 18.868 | .551 |
Elevated CSF WBC count | 19/46 | 9 | .200 |
Elevated CSF protein level | 33 | 26 | .187 |
The status of survival was evaluated before the end of routine follow-up. Patients in the discovery cohort were classified into the GP group or PP group based on their duration of progression-free survival (PFS) longer than 12 months or shorter than 6 months, respectively. There were also similarities in gender, age, weight, and BMI between GP and PP groups (Table 2). Higher ECOG scores and number of lesions were in the GP group. There were no differences in WBC and protein in CSF between the 2 groups. In general, the patients that were classified into GP and PP groups were only dependent on their prognosis outcome after HD-MTX-based chemotherapy treatments.
Characteristics . | All . | Good prognosis . | Poor prognosis . | P . |
---|---|---|---|---|
. | (n = 48) . | (n = 25) . | (n = 23) . | . |
Gender, n (%) | .773 | |||
Male | 24 | 12 | 12 | |
Female | 24 | 13 | 11 | |
Age, median [IQR] | 56[50–66] | 54[49.5–63] | 61[51–68] | .189 |
Weight, Mean (kg) | 68.5 | 71.1 | 65.6 | .542 |
BMI, Mean (kg/m2) | 22.929 | 25.889 | 19.712 | .380 |
ECOG score | .046* | |||
0-1 | 15 | 11 | 4 | |
≥2 | 33 | 14 | 19 | |
Multiple lesions | 18 | 6 | 12 | .044* |
Involvement of deep structure | 35 | 19 | 16 | .616 |
Elevated CSF WBC count | 19/46 | 10/23 | 9/23 | .765 |
Elevated CSF protein level | 33 | 15 | 18 | .173 |
HD-MTX | .709 | |||
8 g/m2 | 30 | 15 | 15 | |
<8 g/m2 | 18 | 10 | 8 | |
Idarubicin | .613 | |||
No | 33 | 18 | 15 | |
Yes | 15 | 7 | 8 | |
Rituximab | .945 | |||
No | 45 | 24 | 21 | |
Yes | 3 | 1 | 2 | |
Dexamethasone | ||||
No | 0 | 0 | 0 | |
Yes | 48 | 25 | 23 |
Characteristics . | All . | Good prognosis . | Poor prognosis . | P . |
---|---|---|---|---|
. | (n = 48) . | (n = 25) . | (n = 23) . | . |
Gender, n (%) | .773 | |||
Male | 24 | 12 | 12 | |
Female | 24 | 13 | 11 | |
Age, median [IQR] | 56[50–66] | 54[49.5–63] | 61[51–68] | .189 |
Weight, Mean (kg) | 68.5 | 71.1 | 65.6 | .542 |
BMI, Mean (kg/m2) | 22.929 | 25.889 | 19.712 | .380 |
ECOG score | .046* | |||
0-1 | 15 | 11 | 4 | |
≥2 | 33 | 14 | 19 | |
Multiple lesions | 18 | 6 | 12 | .044* |
Involvement of deep structure | 35 | 19 | 16 | .616 |
Elevated CSF WBC count | 19/46 | 10/23 | 9/23 | .765 |
Elevated CSF protein level | 33 | 15 | 18 | .173 |
HD-MTX | .709 | |||
8 g/m2 | 30 | 15 | 15 | |
<8 g/m2 | 18 | 10 | 8 | |
Idarubicin | .613 | |||
No | 33 | 18 | 15 | |
Yes | 15 | 7 | 8 | |
Rituximab | .945 | |||
No | 45 | 24 | 21 | |
Yes | 3 | 1 | 2 | |
Dexamethasone | ||||
No | 0 | 0 | 0 | |
Yes | 48 | 25 | 23 |
Characteristics . | All . | Good prognosis . | Poor prognosis . | P . |
---|---|---|---|---|
. | (n = 48) . | (n = 25) . | (n = 23) . | . |
Gender, n (%) | .773 | |||
Male | 24 | 12 | 12 | |
Female | 24 | 13 | 11 | |
Age, median [IQR] | 56[50–66] | 54[49.5–63] | 61[51–68] | .189 |
Weight, Mean (kg) | 68.5 | 71.1 | 65.6 | .542 |
BMI, Mean (kg/m2) | 22.929 | 25.889 | 19.712 | .380 |
ECOG score | .046* | |||
0-1 | 15 | 11 | 4 | |
≥2 | 33 | 14 | 19 | |
Multiple lesions | 18 | 6 | 12 | .044* |
Involvement of deep structure | 35 | 19 | 16 | .616 |
Elevated CSF WBC count | 19/46 | 10/23 | 9/23 | .765 |
Elevated CSF protein level | 33 | 15 | 18 | .173 |
HD-MTX | .709 | |||
8 g/m2 | 30 | 15 | 15 | |
<8 g/m2 | 18 | 10 | 8 | |
Idarubicin | .613 | |||
No | 33 | 18 | 15 | |
Yes | 15 | 7 | 8 | |
Rituximab | .945 | |||
No | 45 | 24 | 21 | |
Yes | 3 | 1 | 2 | |
Dexamethasone | ||||
No | 0 | 0 | 0 | |
Yes | 48 | 25 | 23 |
Characteristics . | All . | Good prognosis . | Poor prognosis . | P . |
---|---|---|---|---|
. | (n = 48) . | (n = 25) . | (n = 23) . | . |
Gender, n (%) | .773 | |||
Male | 24 | 12 | 12 | |
Female | 24 | 13 | 11 | |
Age, median [IQR] | 56[50–66] | 54[49.5–63] | 61[51–68] | .189 |
Weight, Mean (kg) | 68.5 | 71.1 | 65.6 | .542 |
BMI, Mean (kg/m2) | 22.929 | 25.889 | 19.712 | .380 |
ECOG score | .046* | |||
0-1 | 15 | 11 | 4 | |
≥2 | 33 | 14 | 19 | |
Multiple lesions | 18 | 6 | 12 | .044* |
Involvement of deep structure | 35 | 19 | 16 | .616 |
Elevated CSF WBC count | 19/46 | 10/23 | 9/23 | .765 |
Elevated CSF protein level | 33 | 15 | 18 | .173 |
HD-MTX | .709 | |||
8 g/m2 | 30 | 15 | 15 | |
<8 g/m2 | 18 | 10 | 8 | |
Idarubicin | .613 | |||
No | 33 | 18 | 15 | |
Yes | 15 | 7 | 8 | |
Rituximab | .945 | |||
No | 45 | 24 | 21 | |
Yes | 3 | 1 | 2 | |
Dexamethasone | ||||
No | 0 | 0 | 0 | |
Yes | 48 | 25 | 23 |
Metabolomic Analysis of Retrospective PCNSL Patient Cohort
CSF was collected and processed by a targeted metabolomic analysis using a LC-MS-based technique (Figure 1A). As all the retrospective PCNSL cohort patients were classified into either the GP group or PP group based on their PFS lengths, we then preformed a survival analysis on these 2 groups. The Kaplan–Meier curves were shown in Figure 1B which indicated that PFS can distinguish GP group from the PP group significantly. The targeted metabolomic technique was used with profiles of 155 metabolites in the CSF sample from PCNSL patients, which includes amino acids, carbohydrates, organic acids, nucleic acids, vitamins, some lipids, and alkaloids (Figure 1C). As we expected, the Partial Least Squares Discrimination Analysis (PLS-DA) of GP and PP group metabolome can cluster 2 distinct ellipses, which indicates metabolites were altered differently in CSF samples in these 2 groups (Figure 1D).
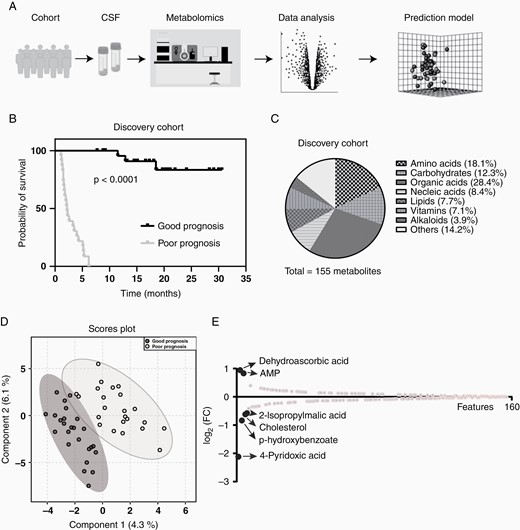
Metabolomic analysis of primary central nervous system lymphoma (PCNSL) patient CSF. (A) The workflow of metabolomic analysis; (B) Patients in discovery cohort were separated by their progression-free survival (PFS) length; (C) Metabolites detected in patient CSF covered most metabolite classes; (D) Metabolites detected in good prognosis (GP) patients’ CSF were different to the ones in poor prognosis (PP) patients; (E) Six metabolic features were selected with a higher different between good and PP patients’ CSF.
To find out the most different metabolites in patient CSF before the HD-MTX-based chemotherapy treatment, we ranked the detected metabolites based on their FC between GP and PP groups. We then selected 6 features that were highly dysregulated in GP group based on their FC (Figure 1E). In these 6 features, dehydroascorbic acid and AMP were upregulated (Log2FC > 0.5) and 2-isopropylmalic acid, cholesterol, p-hydroxybenzoate, and 4-pyridoxic acid were downregulated (Log2FC < −0.5) (Supplementary Figure S1).
Logical Regression Model Development for the Prediction of PCNSL Prognosis
To build up the prediction model that can provide a potential outcome for PCNSL patients after the HD-MTX based chemotherapy, we applied a logical regression model. The workflow of the model development is illustrated in Figure 2, we first processed data with Log10 transformed and normalized using median value and then corrected the batch effect between the discovery and validation cohorts using an empirical Bayes method.24,25 The 6 dysregulated features mentioned above were applied to the modeling buildup. Using a logistics regression-based model, we constitute our model as:
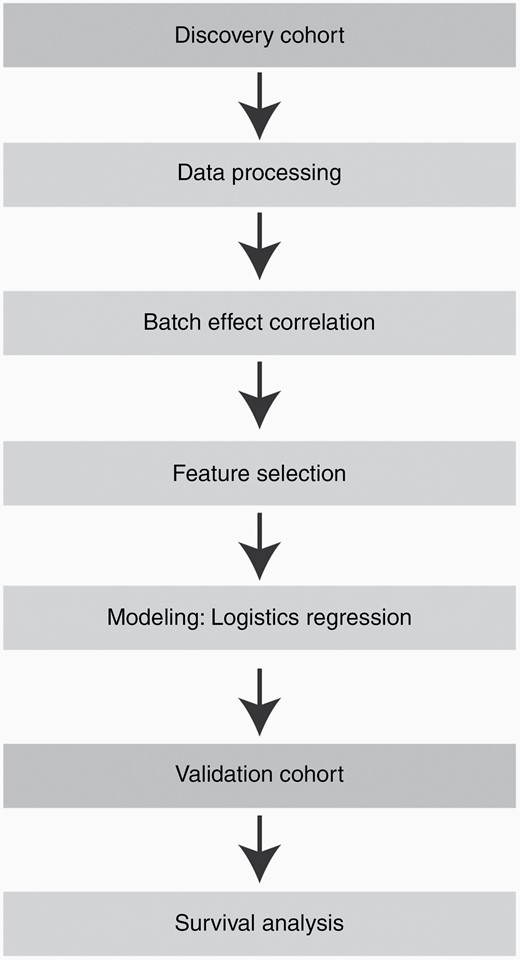
The pipeline of logical regression model development. All the features were selected from the discovery cohort, and then different indexes of the model were tested to locate the best one. The final model was validated using a prospectively collected cohort in which survival analysis was performed.
The 4PA, PHBA, CHOL, 2IA, and DHAA represented 4-pyridoxic acid, p-hydroxybenzoate, cholesterol, 2-isopropylmalic acid, and dehydroascorbic acid, respectively. Next, the logistics regression model was then validated in the discovery cohort, which gave a good performance (Figure 3A and B, AUC = 0.789). The Z scores ranged from −3.38 to 2.52, with a high score associated with a poorer outcome and a lower score indicating a better outcome. The optimal cutoff was a Z score of 0.06. As we expected, the prediction model performed well in terms of the survival time length; the GP group (Z ≤ 0.06) had a median survival time of 18.1 months (discovery cohort), whereas the PP group (Z > 0.06) had a median survival time of 2.2 months (discovery cohort). We also performed survival analyses on these 6 selected features individually, but none of their Kaplan–Meier curves showed the ability to differentiate between GP and PP groups (Figure 3C–H).
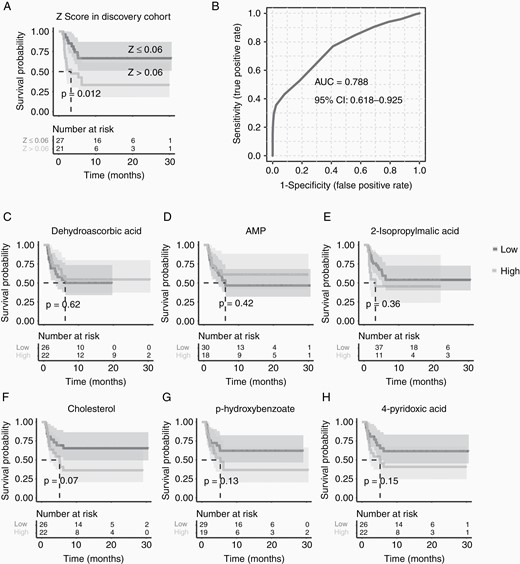
Survival analyses of the model and individual metabolic features. (A) The model of Z score could distinguish good (Z ≤ 0.06) and poor (Z > 0.06) prognosis primary central nervous system lymphoma (PCNSL) patients depending on their progression-free survival (PFS) length; (B) and receiver operating characteristic curve indicated a good classification power of Z score; while none of (C-H) the metabolic features could separate the good prognosis (GP) and poor prognosis (PP) group individually.
Validation of Prediction Model on the Validation PCNSL Cohort
Although the logical regression we built could nicely separate the patients with longer PFS from the discovery cohort, it was still not convinced that such a model can apply to a new PCNSL patient set. For such reason, we next recruited a new PCNSL patient cohort before their HD-MTX-based treatments. As we mentioned before, this validation cohort was similar to the discovery cohort on gender, age, and as well as BMI. We first collected the CSF from the validation cohort patients and profiled their CSF metabolome. Once we received the clinical outcomes of these patients 2 years later, we validated our prediction model by survival analysis. The Kaplan–Meier curve of the validation set can also be separated nicely (Figure 4A and B, P = .032, AUC = 0.745), which the patients with Z ≤ 0.06 had longer survival time (median 18.6 months) and Z > 0.06 had shorter survival time (median 2.4 months) as we expected. This result indicated that the logical regression model we built based on CSF metabolic features could nicely apply to the prediction of PCNSL patient prognosis outcomes with HD-MTX based treatments.
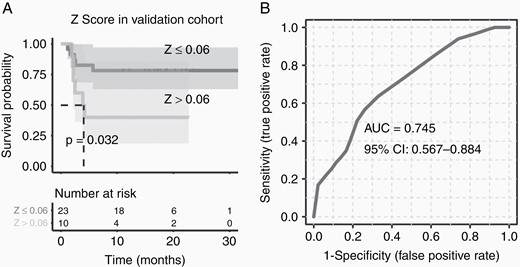
Applying the prediction model on a prospectively recruited primary central nervous system lymphoma (PCNSL) cohort. (A) The Z score could also nicely distinguish the good (Z ≤ 0.06) prognosis PCNSL patients from the validation PCNSL cohort, (B) and the receiver operating characteristic curve indicated a good classification power of Z score.
Discussion
As an aggressive lymphoma occurs in the central nervous system, the prognosis of PCNSL was generally inferior. Although HD-MTX based chemotherapies are the first-line therapeutic regimens for PCNSL patients, most patients still suffered relatively short survival.27,28 Considering the several side effects including nephrotoxicity, hepatotoxicity, neurotoxicity, mucositis, and myelosuppression of such high dosage chemotherapy,29 a prediction of HD-MTX based chemotherapy prognoses before the treatment would benefit the PCNSL patients. Ryuya Yamanaka et al. applied different factors including gene expression and cancer morphology to create multiple promising prediction models on retrospective cohort studies in the last decade.11,30 Although the prediction models revealed a good ability to identify those patients who are unlikely to benefit from the standard therapies, the models were not validated by a clinical prospective cohort. Retrospective study can provide lots of information in a relatively short period, but it has high bias risk and comparison limitations.31 In our study, it is the first time we recruited 2 sets of PCNSL patients for the prognosis predictive model development, of which a 48-patient discovery group was collected from 2018 to 2020 and 35 patients prospectively recruited from 2020 to 2021 as validation group. At the time we started this clinical study, we only obtained the prognosis results of the discovery group patients. Thus, the prediction model buildup was all based on the 48-patient discovery group retrospectively. Once we received the clinical outcomes of prospectively recruited PCNSL patients, we found our prediction model can indeed distinguish the patients with poor HD-MTX treatment outcomes (Figure 4A and B), which would be helpful for PCNSL patients to make a decision if a HD-MTX-based chemotherapy is preferred.
Although most of the prediction models of PCNSL prognosis of HD-MTX based chemotherapies were based on gene expression levels, it was interesting to notice that the gene set used for the prediction model is not strongly associated. Some protein markers such as interleukin 6 (IL-6) and myeloid differentiation primary response (88) (MYD88) were identified as PCNSL biomarkers to distinguish from other brain tumors, which are significantly higher in PCNSL CSF and serum samples.32–40 Thus, we also measured the levels of IL-6 and MyD88 in PCNSL patient samples. However, the levels of both IL-6 and MyD88 were not significantly different between good and PP patients in our cohorts (Supplementary Figure S2A–B). Considering that the patients we recruited in this study were all diagnosed as PCNSL, the IL-6 or MyD88 might not be a good feature to distinguish their HD-MTX-based treatment outcomes. In addition, the MyD88 L265D mutation was believed as a prognostic biomarker in PCNSL,37,41–43 the sensitive genome measurement would be a good tool to differentiate their HD-MTX-based treatment outcomes in the future.44–46 In addition, considering the anti-cancer target of MTX is a metabolic enzyme, DHFR, that can perturb one-carbon metabolism,19,20 it is reasonable to hypothesize that outcome of HD-MTX-based chemotherapies might be associated with patient metabolic status. Recently, Feng-Xiang et al. characterized PCNSL from 4 different types of brain tumors using 27 CSF metabolites47 and Jae et al. could also diagnose PCNSL using CSF metabolites.48 These 2 studies implied that the metabolic status in PCNSL patients’ CSF could be various. In addition, Yasuo et al. also observed an excessive glycolysis metabolism in methotrexate-resistant PCNSL-derived cells,49 which shed light on the clinical prognosis prediction studies using metabolomics approach. In our study, we applied a targeted metabolomic analysis approach that includes most compounds involved in central carbon metabolism (Figure 1C and Supplementary Table 1) to profile the metabolic alteration of the PCNSL patient CSF sample. Since the metabolomic profiling was performed before the HD-MTX based chemotherapy treatments, the dysregulated metabolites in CSF were more likely associated with patient outcomes for such treatment. To our surprise, the 6 dysregulated metabolites (dehydroascorbic acid, AMP, 2-isopropylmalic acid, cholesterol, p-hydroxybenzoate, and 4-pyridoxic acid) in CSF are not highly associated with glycolysis metabolism discovered in MTX-resistant cell.49 Such differences could be due to the observation variances between clinical and in vitro systems. Of these changed CSF metabolites, 4-pyridoxic acid caught our attention due to its involvement in one-carbon metabolism. DHFR is necessary for the change of DHF to THF, while THF can readily be turned into N5,10 methylene THF with the help of SHMT and PLP (Supplementary Figure S3A). The PLP can turn to other forms of vitamin B6 and eventually be metabolized to 4-pyridoxic acid.50 When the methotrexate is not working properly in the central nervous system, the one-carbon metabolism might be dysregulated in PCNSL tumors. The correlation between one-carbon metabolism with lymphoma was studied. Multiple research groups observed a predominated correlation between reduced risk of non-Hodgkin lymphoma (NHL) with higher dietary vitamin B6 and methionine intakes.51–53 Unhee Lim et al. took a further look at the genetic alterations and found such one carbon metabolism contributes to lymphomagenesis not only on nutrient dietary intake but also associates with genetic variation.54 In addition, such vitamin B6 metabolic dysregulation might associate with other types of cancer, that Lorenzo et al. found that vitamin B6 sensitizes nonsmall cell lung cancer (NSCLC) and low pyridoxal kinase (PDXK) expression would associate with poor disease outcome.55 In our study, the accumulation of 4-pyridoxic acid in CSF (Supplementary Figure S3B) might due to an efflux of vitamin B6. The vitamin B6 cannot perform its proper function in the one-carbon metabolism pathway but was flushed as an acidic form via CSF. For such reason, we hypothesized that the accumulation of the 4-pyridoxic acid in PP patient CSF might also result from the dysregulation of such one-carbon metabolism.
Although we successfully developed a model that can predict PCNSL patient prognosis with HD-MTX based chemotherapy treatments, there are still several limitations in this study. Because the PCNSL patient recruitment has only happened in Huashan hospital and only the Chinese population was considered in this prospective study, the results of our clinical study may have bias which needs multicentral study for a further validation in the future. Moreover, due to the relatively low occurrence rate of PCNSL, the low number of samples limited our further mechanistic studies. A long-period clinical study would be helpful in the future. It is unfortunate that the metabolites in serum could not distinguish the outcome differences of PCNSL patients (Supplementary Figure S4A and B), so this prediction model was based on the metabolome changes in CSF, not serum making the clinical test application difficult. A more convenient CSF sample collection would improve the clinical practice to predict PCNSL patient prognosis with HD-MTX-based chemotherapy treatments.
Conclusions
In summary, we applied the metabolomic technique to study the prognosis of PCNSL patients with HD-MTX based chemotherapy in the prospective cohort study. The metabolic markers can formulate a prediction model to distinguish the PP patients using a scoring standard (Z score > 0.06) before receiving the HD-MTX based chemotherapy. Such a model shows a high potential for clinical application in the future, and the metabolic markers discovered in this study would provide a direction for PCNSL mechanistic research.
Acknowledgments
We thank Ke Qiao, Lanlan Zhang, Rongrong Cheng, and Hongfang Zhao for metabolomic and lipidomic data generation from Single Cell Quantitative Metabolomics and Lipidomics Core Facility of IMIB at Fudan University.
Funding
This work was supported by National Key Research and Development Program of China (2020YFA0803800 and 2019YFA0801900), Natural Science Foundation of China (92057115) and Shanghai Sailing Program (20YF1402600) from H.H., and Shanghai Shenkang clinical science and technology innovation project (SHDC12020112) from C. B.
Conflict of Interest
None.
Institutional Review Board Statement
This study was approved by the Institutional Review Board of Huashan Hospital (protocol No.2021-777 and No.2022-767).
Author Contribution
Conceptualization, LZ, QL, JX, BC, HH; Investigation, LZ, QL, JX, HH; Analysis, LZ, QL, JX, HH; Writing, LZ, QL, JX, HH; Funding Acquisition, HH; Supervision, BC, HH. Dr. He Huang and Dr. Bobin Chen have accessed and verified the data. All authors confirm that they had full access to all the data in the study and accept responsibility to submit it for publication. All the authors promise the accuracy and completeness of the data. All authors participated in the revision and approved the final version of the manuscript.
Data Availability
The source code for the prediction model is available on Github (https://github.com/JavaScripy/2022/tree/main/PCNSL). The original data of this study are available on request from the corresponding author Dr. He Huang ([email protected]).
References
Author notes
These authors contribute equally.