-
PDF
- Split View
-
Views
-
Cite
Cite
Sunil Bhandari, Samir Mehta, Natalie Ives, Paul Cockwell, Utility of 2-year Kidney Failure Risk Equation for advanced chronic kidney disease: analysis from the STOP-ACEi trial, Nephrology Dialysis Transplantation, Volume 39, Issue 5, May 2024, Pages 884–887, https://doi.org/10.1093/ndt/gfad263
- Share Icon Share
To the Editor,
The rate of progression of advanced chronic kidney disease (CKD) to end-stage kidney disease (ESKD) varies [1] and understanding the risk of progression enables patients and clinicians to make timely shared decisions around kidney replacement therapy (KRT; dialysis and transplantation) [2, 3] to optimize outcomes and adherence to treatment [4, 5].
International guidelines recommend referral of patients with a risk of kidney failure in 1 year of 10%–20% for planning for KRT [6]. UK guidance recommends that patients who are predicted to reach ESKD within 18 months should be assessed for treatment options in advanced kidney care services [7]. A Canadian provincial service utilizes a risk of ≥40% in 2 years for kidney failure for formation of an arteriovenous fistula [8].
The 2-year Kidney Failure Risk Equation (KFRE) based on demographic and laboratory data [9] has excellent discrimination for defining the risk of progression to ESKD [10]. However, there are limited data on its utility in people with advanced CKD for transition of care such as preparation for ESKD, listing for kidney transplantation and formation of vascular access. Understanding the relationship between KFRE and risk of progression in advanced CKD will help the design of advanced kidney care services.
We examined the accuracy of the KFRE equation to predict ESRD/KRT in patients recruited to the multicentre randomized STOP-ACEi trial [11, 12], including stratification by risk thresholds for referral and intervention.
This study assessed patients from the STOP-ACEi trial recruited from 37 UK centres. Recruitment criteria comprised: age ≥18 years (male or female), CKD stage 4 or 5 [estimated glomerular filtration rate (eGFR) <30 mL/min/1.73 m2 using the Modification of Diet in Renal Disease equation] and a progressive deterioration in kidney function (fall in eGFR of >2 mL/min/year over previous 24 months) as measured by linear regression analysis based on a minimum of three eGFR measurements.
The KFRE equation was used to calculate the 2-year risk of treated kidney failure (as defined by starting dialysis or receiving a kidney transplant).
Calculation of 2-year ESKD risk was done using KFRE calibrated to a non-North American population from baseline data from both arms of the trial. The baseline visit data for each patient used in the calculation comprised: age, sex, eGFR (mL/min/1.73 m2), urine albumin-to-creatinine ratio (ACR) (mg/g) for the 4-variable KFRE, and these data together with albumin (g/dL), phosphate (mg/dL), bicarbonate (mmol/L) and calcium (mg/dL) for the 8-variable KFRE were used to estimate the 2-year risk. Patients were then categorized into risk group split at <20%, 20%–39% and ≥40% based on the KDIGO threshold for planning for KRT and the Manitoba Renal Programme [6].
We examined correlations between KFRE estimations and observed events using a competing risk (Fine and Gray) model, which accounted for the competing risk of death. The observed events were patients that had started dialysis or received a kidney transplant within 2 years of being randomized in the STOP-ACEi trial. The primary aim was to assess the model performance at 2 years so data was truncated at this time-point. Harrell's C-statistic and calibration curve were calculated to assess the model discrimination and calibration. Risk category group <20% was the reference category for all analysis.
We enrolled 411 patients, median age was 63 years, 68% were men and 85% of white ethnicity. Detailed demographic breakdown has previously been published [11, 12]. The median baseline eGFR was 18 mL/min/1.73 m2 and median baseline ACR was 680 mg/g. Median calcium, phosphate, bicarbonate and albumin were 9 mg/dL, 4 mg/dL, 22 mmol/L and 4 g/dL, respectively (Supplementary data, Table S1). Median (interquartile range) calculated 2-year risk scores based on the 4- and 8-variable equations were 24% (13%–44%) and 21% (11%–44%), respectively. Overall, 200 (49%) of participants reached kidney failure within 2 years, and 17 (4%) died before reaching kidney failure within 2 years.
The KFRE 4-variable equation calculation had a good model discrimination with a Harrell's C-statistic value of 0.71 [95% confidence interval (CI) 0.68–0.74] and showed good separation of risk based on the proposed risk categorizations (Table 1, Fig. 1a) with the strongest correlation for ≥40% cut-off [hazard ratio (HR) of 6.94 (95% CI 4.87–9.90)]. The KFRE 8-variable equation had a Harrell's C-statistic value of 0.71 (95% CI 0.67–0.74) and again showed good separation of risk based on the proposed risk categorizations (Table 1, Fig. 1b) with the strongest correlation for ≥40% cut-off [HR of 5.63 (95% CI 4.02 to 7.90)]. The KFRE 8-variable had no additional utility over the KFRE 4-variable equation. The test of interaction between KFRE estimation and intervention was insignificant for both 4- and 8-variable equations (P = .8 and P = .4, respectively), which suggested that discontinuation of angiotensin-converting enzyme inhibitor/angiotensin-receptor blocker did not impact the prediction on progression at 2 years.
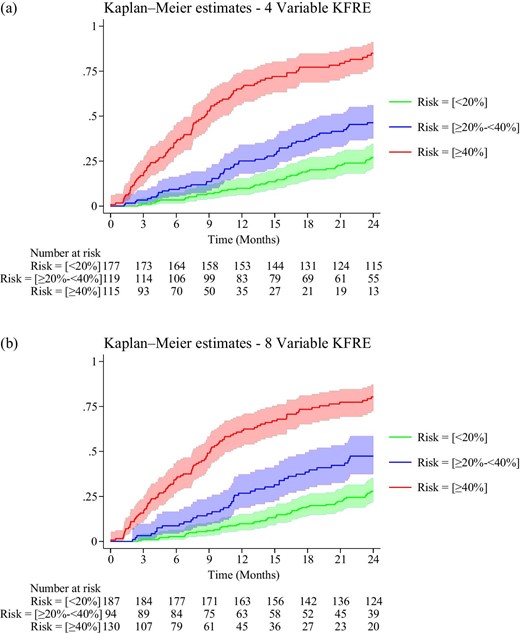
Kaplan–Meier curves with 95% CIs for 4-variable (a) and 8-variable (b) KFRE for risk based on <20%, ≥20%–39% and ≥40%.
. | Event (ESRD/KRT) within 2 years, n (%) . | . | . | . | |
---|---|---|---|---|---|
Risk category . | No (N = 194) . | Yes (N = 200) . | Competing risk of death, n (%) (N = 17) . | HR (95% CI) . | Harrell's C-statistic (95% CI) . |
4-variable KFRE model | |||||
<20% | 121 (62.4) | 48 (24) | 8 (47.1) | Reference category | 0.71 (0.68–0.74) |
≥20%–39% | 55 (28.4) | 58 (29) | 6 (35.3) | 2.13 (1.46–3.10) | |
≥40% | 18 (9.3) | 94 (47) | 3 (17.6) | 6.94 (4.87–9.90) | |
8-variable KFRE model | |||||
<20% | 126 (64.9) | 54 (27.0) | 7 (41.2) | Reference category | 0.71 (0.67–0.74) |
≥20%–39% | 41 (21.1) | 47 (23.5) | 6 (35.3) | 2.14 (1.47–3.13) | |
≥40% | 27 (13.9) | 99 (49.5) | 4 (23.5) | 5.63 (4.02–7.90) |
. | Event (ESRD/KRT) within 2 years, n (%) . | . | . | . | |
---|---|---|---|---|---|
Risk category . | No (N = 194) . | Yes (N = 200) . | Competing risk of death, n (%) (N = 17) . | HR (95% CI) . | Harrell's C-statistic (95% CI) . |
4-variable KFRE model | |||||
<20% | 121 (62.4) | 48 (24) | 8 (47.1) | Reference category | 0.71 (0.68–0.74) |
≥20%–39% | 55 (28.4) | 58 (29) | 6 (35.3) | 2.13 (1.46–3.10) | |
≥40% | 18 (9.3) | 94 (47) | 3 (17.6) | 6.94 (4.87–9.90) | |
8-variable KFRE model | |||||
<20% | 126 (64.9) | 54 (27.0) | 7 (41.2) | Reference category | 0.71 (0.67–0.74) |
≥20%–39% | 41 (21.1) | 47 (23.5) | 6 (35.3) | 2.14 (1.47–3.13) | |
≥40% | 27 (13.9) | 99 (49.5) | 4 (23.5) | 5.63 (4.02–7.90) |
. | Event (ESRD/KRT) within 2 years, n (%) . | . | . | . | |
---|---|---|---|---|---|
Risk category . | No (N = 194) . | Yes (N = 200) . | Competing risk of death, n (%) (N = 17) . | HR (95% CI) . | Harrell's C-statistic (95% CI) . |
4-variable KFRE model | |||||
<20% | 121 (62.4) | 48 (24) | 8 (47.1) | Reference category | 0.71 (0.68–0.74) |
≥20%–39% | 55 (28.4) | 58 (29) | 6 (35.3) | 2.13 (1.46–3.10) | |
≥40% | 18 (9.3) | 94 (47) | 3 (17.6) | 6.94 (4.87–9.90) | |
8-variable KFRE model | |||||
<20% | 126 (64.9) | 54 (27.0) | 7 (41.2) | Reference category | 0.71 (0.67–0.74) |
≥20%–39% | 41 (21.1) | 47 (23.5) | 6 (35.3) | 2.14 (1.47–3.13) | |
≥40% | 27 (13.9) | 99 (49.5) | 4 (23.5) | 5.63 (4.02–7.90) |
. | Event (ESRD/KRT) within 2 years, n (%) . | . | . | . | |
---|---|---|---|---|---|
Risk category . | No (N = 194) . | Yes (N = 200) . | Competing risk of death, n (%) (N = 17) . | HR (95% CI) . | Harrell's C-statistic (95% CI) . |
4-variable KFRE model | |||||
<20% | 121 (62.4) | 48 (24) | 8 (47.1) | Reference category | 0.71 (0.68–0.74) |
≥20%–39% | 55 (28.4) | 58 (29) | 6 (35.3) | 2.13 (1.46–3.10) | |
≥40% | 18 (9.3) | 94 (47) | 3 (17.6) | 6.94 (4.87–9.90) | |
8-variable KFRE model | |||||
<20% | 126 (64.9) | 54 (27.0) | 7 (41.2) | Reference category | 0.71 (0.67–0.74) |
≥20%–39% | 41 (21.1) | 47 (23.5) | 6 (35.3) | 2.14 (1.47–3.13) | |
≥40% | 27 (13.9) | 99 (49.5) | 4 (23.5) | 5.63 (4.02–7.90) |
Model calibration was examined by plotting the observed event probability against predicted probability at 2 years. The calibration plots showed very good calibration for the 4- and 8-variable KFREs at 2 years (Supplementary data, Figs S1 and S2).
We found significant concordance between the KFRE 4- and 8-variable risk estimation at the index baseline visit and actual outcomes. We showed that the KFRE 4- and 8-variable risk estimation is accurate and precise in this population for 2-year risk of progression to kidney failure. As this population comprises patients with an eGFR of <30 mL/min/1.73 m2 and a confirmed decline in kidney function prior to enrolment in the study it is a high-risk group for kidney failure progression. However, CKD stage–based classification or using eGFR thresholds such as 15 mL/min/1.73 m2 are of limited utility as they do not incorporate other variables for progression to ESKD such as age, gender and ACR—variables in the KFRE 4-variable risk equation in addition to eGFR.
This study has several strengths. The relatively large patient population and long follow-up ensured a high proportion of participants progressed to ESKD. These events were confirmed via active trial review. We enrolled patients from 37 different clinical sites, capturing variability in clinical approach and patient population. In addition, use of the model has been verified by Al-Wahsh et al. [13] in a large cohort of over 14 600 participants, where predictions about the risk of kidney failure were virtually unaffected by consideration of competing risks during the first 2 years after developing stage 4 CKD.
Death prior to starting dialysis would not prevent potential preparation for KRT. Therefore, the model remains valid but we recognize the requirement for separate assessment and decision of the appropriateness of dialysis in frail patients, and this remains a huge challenge for patients and clinicians. There is no ideal measure but clinical frailty score assessment may be of value [14].
Consistent with the main study results, there was no impact of the trial intervention on progression to ESKD. The major weakness of the study is that one of the criteria for participation is progression of CKD in the 2 years prior to recruitment. It is not known whether this is an additional risk for future progression. Also with the population of advanced kidney disease studied the results may not be generalizable to other populations.
KFRE has been identified as the best available 2-year risk prediction equation for advanced CKD and is being used to support appropriate timing of information for decision making for treatment of kidney failure and subsequent listing for kidney transplantation or vascular access formation [8]. Previously, the Kidney Disease: Improving Global Outcomes 2012 clinical practice guideline had recommended referral for planning for treatment for kidney failure from a 1-year risk of 10% [6].
In this study we used thresholds of a 2-year KFRE of <20%, 20%–39% and ≥40% to categorize patients for future interventions. Based on the KFRE 4-variable equation Kaplan–Meier estimation, at 3 months, censored for death, we found that for patients with risks of <20%, 20%–39% or ≥40%, 1.1% (2/177), 3.4% (4/119) and 16.5% (19/115) progressed to ESKD, respectively. In addition, the Kaplan–Meier plots with 95% CI show maintained separation between the curves. Hence use of these thresholds are reasonable for care transition into advanced kidney care service (using 20% 2-year risk) and for enactment of treatment options (using 40% 2-year risk) required for ESKD preparation.
ACKNOWLEDGEMENTS
We are grateful to the research nurses and research assistants and all the principal investigators who facilitated completion of this study. We are particularly grateful to all the participants who kindly consented to be part of this study.
FUNDING
This study is funded by a grant from The National Institute for Health Research and the Medical Research Council (grant number: NIHR-HTA 11/30/07. STOP ACEi EudraCT number, 2013-003798-82; ISRCTN number, 62869767).
AUTHORS’ CONTRIBUTIONS
S.B., P.C. and N.I. conceived and designed the study; S.M. and N.I. analysed the data; S.B. and P.C. interpreted and contextualized the data; S.B. wrote the first draft of the manuscript; S.B., P.C., N.I. and S.M. revised the draft manuscript; all authors approved the final manuscript.
DATA AVAILABILITY STATEMENT
The original study datasets supporting the findings of this study and the primary paper are stored within the University of Birmingham servers as per the standard operating procedures (SOPs) of the Birmingham Clinical Trials Unit (BCTU) under the auspices of the Data Sharing Committee. The data can be made available (if requested) via the data sharing processes within BCTU and upon agreement with the chief investigator of the study
CONFLICT OF INTEREST STATEMENT
The authors have no conflict of interest related to this trial and all other potential conflicts of interest are detailed in each individual ICMJE form submitted.
Comments