-
PDF
- Split View
-
Views
-
Cite
Cite
Zuoxian Hou, Gu-Mu-Yang Zhang, Yixin MA, Peng Xia, Xiaoxiao Shi, Hao Sun, Zhengguang Chen, Limeng Chen, #5276 DEVELOPMENT OF A MULTIMODAL “KIDNEY AGE” PREDICTION BASED ON AUTOMATIC SEGMENTATION CT IMAGE IN PATIENTS WITH NORMAL RENAL FUNCTION, Nephrology Dialysis Transplantation, Volume 38, Issue Supplement_1, June 2023, gfad063d_5276, https://doi.org/10.1093/ndt/gfad063d_5276
- Share Icon Share
Abstract
Renal function, for decades, has been of interest to clinicians and researchers to describe. For example, serum creatinine (Scr) and estimated glomerulus filtration rate (eGFR) is familiar but also limited in many circumstances. Meanwhile, the physiological volumes of the kidney cortex and medulla are presumed to change with age and have been proven to change with decreasing kidney function.
We recruited 182 patients with normal Scr levels and contrasted CT images between Oct. 2021 and Feb 2022 in Peking Union Medical College Hospital (PUMCH) with their demographic and clinical data. The automatic segmentation method was modified from U-NET and used for both cortex and medullary separation and their volume calculation, respectively. We combined the kidney volume and clinical data as multimodal features of the machine learning model. All the data were separated into a training dataset (PUMCH training set, 80%) and a test dataset (PUMCH test set, 20%). Besides, we included patients with the same inclusion criteria but with diabetes (PUMCH-DM test set) and diabetic nephropathy (PUMCH-DN test set) for internal comparison to verify the possible clinical value of “KIDNEY AGE” (K-AGE). Data from DongZhiMen Hospital (DZMH test set) was used as separate external validation sets to evaluate model generalizability.
The PUMCH training set included 146 participants with a mean age of 47.5±7.4 years. 58.9% were female, and the mean Scr is 63.5±12.3 μmol/L. The PUMCH test set included 36 participants with a mean age of 47.1±7.9 years, 52.7% were female, and the mean Scr was 66.9±13.0 μmol/L. For segmented kidneys, volume differences between non-contrast-enhanced CT and non-contrast-enhanced CT in the left and right kidneys were not evident. The multimodal neural network predicted: K-AGE approximately close to the patient's actual physiological age, with 92% prediction within the 95% confidential interval (Figure 1). We also compared the K-AGE prediction in PUMCH, PUMCH-DM, and PUMCH-DN test sets by applying U-NET-nonCon segmentation algorithm in non-contrast CT images. The mean absolute error increases along with the disease process (control group 5.00, diabetes group 6.99, diabetic nephropathy group 9.32) (Figure 2).
We established a machine learning model for predicting the K-AGE of normal Scr patients.
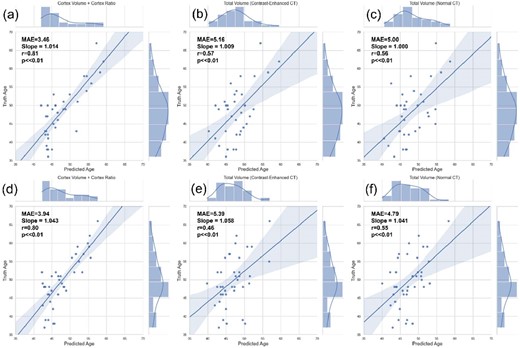
The agreement of K-Age and actual age in the test set obtained from contrast-enhanced CT and non-contrast-enhanced CT automatic segmentation in PUMCH test set and DZMH test set.
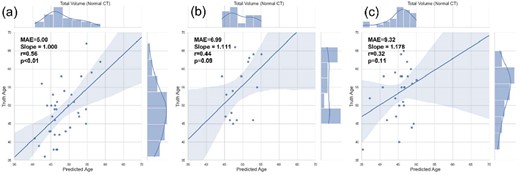
The K-Age prediction in PUMCH, PUMCH-DM, and PUMCH-DN test set applying U-NET-nonCon segmentation algorithm in non-contrast CT images.
Comments