-
PDF
- Split View
-
Views
-
Cite
Cite
Jun Morinaga, Tatsuyuki Kakuma, Hirotaka Fukami, Manabu Hayata, Kohei Uchimura, Teruhiko Mizumoto, Yutaka Kakizoe, Taku Miyoshi, Naoki Shiraishi, Masataka Adachi, Yuichiro Izumi, Takashige Kuwabara, Yusuke Okadome, Michio Sato, Haruki Horiguchi, Taichi Sugizaki, Tsuyoshi Kadomatsu, Keishi Miyata, Saeko Tajiri, Tetsuya Tajiri, Kimio Tomita, Kenichiro Kitamura, Yuichi Oike, Masashi Mukoyama, Circulating angiopoietin-like protein 2 levels and mortality risk in patients receiving maintenance hemodialysis: a prospective cohort study, Nephrology Dialysis Transplantation, Volume 35, Issue 5, May 2020, Pages 854–860, https://doi.org/10.1093/ndt/gfz236
- Share Icon Share
Abstract
Patients undergoing hemodialysis treatment have a poor prognosis, as many develop premature aging. Systemic inflammatory conditions often underlie premature aging phenotypes in uremic patients. We investigated whether angiopoietin-like protein 2 (ANGPTL 2), a factor that accelerates the progression of aging-related and noninfectious inflammatory diseases, was associated with increased mortality risk in hemodialysis patients.
We conducted a multicenter prospective cohort study of 412 patients receiving maintenance hemodialysis and evaluated the relationship between circulating ANGPTL2 levels and the risk for all-cause mortality. Circulating ANGPTL2 levels were log-transformed to correct for skewed distribution and analyzed as a continuous variable.
Of 412 patients, 395 were included for statistical analysis. Time-to-event data analysis showed high circulating ANGPTL2 levels were associated with an increased risk for all-cause mortality after adjustment for age, sex, hemodialysis vintage, nutritional status, metabolic parameters and circulating high-sensitivity C-reactive protein levels {hazard ratio [HR] 2.04 [95% confidence interval (CI) 1.10–3.77]}. High circulating ANGPTL2 levels were also strongly associated with an increased mortality risk, particularly in patients with a relatively benign prognostic profile [HR 3.06 (95% CI 1.86–5.03)]. Furthermore, the relationship between circulating ANGPTL2 levels and mortality risk was particularly strong in patients showing few aging-related phenotypes, such as younger patients [HR 7.99 (95% CI 3.55–18.01)], patients with a short hemodialysis vintage [HR 3.99 (95% CI 2.85–5.58)] and nondiabetic patients [HR 5.15 (95% CI 3.19–8.32)].
We conclude that circulating ANGPTL2 levels are positively associated with mortality risk in patients receiving maintenance hemodialysis and that ANGPTL2 could be a unique marker for the progression of premature aging and subsequent mortality risk in uremic patients, except those with significant aging-related phenotypes.
INTRODUCTION
The number of chronic kidney disease (CKD) patients is increasing worldwide, with estimates of >30 million and >13 million people affected in the United States and Japan, respectively [1, 2]. A large proportion of patients with end-stage CKD have no alternative but to undergo lifesaving renal replacement therapy, often in the form of hemodialysis. In 2016, >457 000 individuals underwent hemodialysis in the United States and >329 000 in Japan [1, 3]. Clinically, the prognosis is poor in this patient group [4]. Therefore, evaluating mortality risk for these patients is extremely important.
Patients receiving hemodialysis exhibit significant premature aging phenotypes compared with healthy individuals or patients undergoing renal transplant, and these phenotypes increase mortality risk in these individuals [5]. The pathological mechanisms underlying premature aging in these patients are complex, as several factors, such as uremia, fluid overload, oxidative stress, comorbidities including heart failure and exogenous factors including dialysis treatment itself, may play a role contributing to the aging phenotypes [6, 7]. However, each of these pathological mechanisms is associated with chronic inflammation [6, 7]. In advanced CKD, inflammatory triggers include activation of the innate immune system, defective regulation of inflammatory processes and increased cytokine secretion by uremic senescent cells—a collection of events known as the senescence-associated secretory phenotype [8]. Subsequently, chronic systemic inflammation in uremia promotes significant modification of metabolism in favor of increased catabolic pathways and proaging mechanisms and away from anabolic pathways and antiaging mechanisms [6, 7]. These changes accelerate the onset of phenotypes of premature aging such as muscle wasting, osteoporosis, vascular calcification and cardiovascular hypertrophy and contribute to patient mortality [5, 7]. Accordingly, we hypothesized that circulating levels of inflammation-related factors may be correlated with premature aging phenotypes and associated mortality in uremic patients.
Angiopoietin-like protein 2 (ANGPTL 2), which possesses an N-terminal coiled-coil domain used for oligomerization and a C-terminal fibrinogen-like domain, is a secreted protein structurally similar to angiopoietin, although it does not bind to the angiopoietin receptor [9]. Previously we demonstrated that ANGPTL2 participates in physiological tissue remodeling and plays a crucial role in pathological conditions associated with chronic noninfectious inflammation or aging [10–12]. ANGPTL2 also plays a pivotal role in the progression of multiple age-related conditions such as atherosclerosis, carcinogenesis, sarcopenia, frailty and CKD and is a significant inducer of chronic inflammation [12–16]. Interestingly, because circulating ANGPTL2 levels parallel the local secretion of the protein in tissues, it has been suggested that analysis of circulating ANGPTL2 levels would represent a useful biomarker of inflammation and aging-related outcomes, including de novo incidence of diabetes and cardiovascular disease in nonuremic subjects [17, 18]. However, an association between ANGPTL2 levels and clinical outcomes has not been investigated in the uremic population.
To address this question, we conducted a multicenter prospective cohort study of patients receiving maintenance hemodialysis in Kumamoto, Japan, to determine whether circulating ANGPTL2 levels predict mortality risk in patients on maintenance hemodialysis, after adjustment for confounding factors.
MATERIALS AND METHODS
Study design
This study had an observational, multicenter prospective cohort design targeting a population of patients receiving maintenance hemodialysis in five clinics in Japan. From March 2011 to March 2012, 412 of 515 patients who received hemodialysis treatment in these five clinics were enrolled after written informed consent to participate in the study was provided by all patients (Supplementary Figure S1). The participating patients were then followed up for 6 years. This study was conducted in accordance with the Helsinki Declaration and with approval by the ethics committees for clinical research at Kumamoto University.
Measurement of circulating ANGPTL2 levels
Serum specimens were stored at −80°C until subsequent use. ANGPTL2 protein levels were measured at the Department of Nephrology, Kumamoto University, using a human ANGPTL2 enzyme-linked immunosorbent assay kit designed to detect full-length ANGPTL2 using antibodies targeting the N- and C-termini of the protein (Immuno-Biological Laboratories, Gunma, Japan) [11, 17, 18]. Antibody specificity was confirmed, with no antibody cross-reaction detected with other ANGPTLs.
Statistical analysis
Prior to statistical analysis, listwise case deletion was applied to the data set and the number of subjects (412 subjects) was reduced to 395 (Supplementary Figure S1). All variables were visually examined and ANGPTL2, hemodialysis vintage, high-sensitivity C-reactive protein (hs-CRP), whole parathyroid hormone, ferritin, iron (Fe), transferrin saturation, triglycerides, creatine kinase, platelets, amylase, γ-glutamyl transferase, alkaline phosphatase, aspartate aminotransferase, alanine transaminase, total bilirubin and frequency of percutaneous transluminal angioplasties were log-transformed to correct for skewed distribution.
Prognostic staging was determined in two steps. First, the random forest method was applied to ranking according to the strength of association with the outcome, using all 52 variables collected from routine clinical data. After clinical consideration, the 10 strongest risk factors were selected to establish the prognostic stages. Then the survival tree method was applied to create nine patient groups with similar prognostic profiles [19]. To generate the final prognostic stage, hazard ratios (HRs) for the nine patient groups were compared and combined to obtain a parsimonious model with four prognostic strata.
To ensure the robustness of hazard estimates using the Cox PH model, bootstrap analysis with 10 000 replications was performed and the average HR with 95% confidence interval was determined. To examine the relationship between circulating ANGPTL2 levels and aging-related parameters, we applied a mixed model adjusting for medical facility location to account for random effects. All statistical analyses were performed using Stata 15.0 (StataCorp, College Station, TX, USA), except for random forest analysis, which was conducted using JMP Pro 13.2.1 (SAS Institute, Cary, NC, USA). All P-values were two-tailed and P < 0.05 was taken as statistically significant.
RESULTS
The characteristics of study participants at baseline are shown in Table 1. Figure 1 depicts a histogram of circulating ANGPTL2 levels and the corresponding proportion of patients (%) receiving hemodialysis [median 3.3 ng/mL (interquartile range 2.5–4.0)]. For the 395 subjects included in our statistical analysis, the median follow-up time for survival analysis was 2213 days, and 94 of the 395 patients died. To assess for a potential association between circulating ANGPTL2 levels and patient mortality risk using survival data, we applied the Cox PH model and found a significant association of high circulating ANGPTL2 levels with high mortality risk in patients undergoing hemodialysis, after adjustment for age, sex, smoking, diabetes, hypertension, dyslipidemia, body mass index, cancer, hemodialysis vintage, albumin, cardiothoracic ratio (CTR) and the product of adjusted calcium and inorganic phosphorus levels (Table 2, Model 2). This association remained significant following adjustment for hs-CRP levels (Table 2, Model 3). These data suggest that circulating ANGPTL2 levels could serve as a useful biomarker to predict mortality risk in patients receiving maintenance hemodialysis, after adjustment for confounding factors.
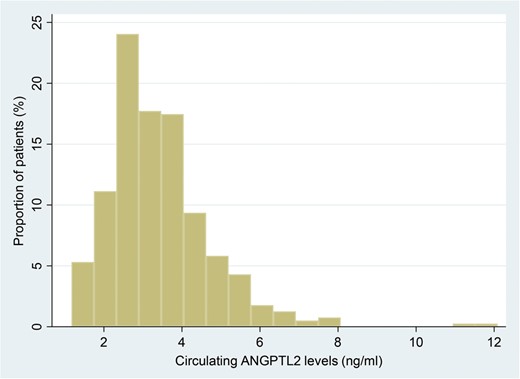
Histogram showing circulating ANGPTL2 levels and the corresponding proportion of patients (%) receiving hemodialysis. Median 3.3 ng/mL (interquartile range 2.5–4.0).
Categorical variables . | Number . | Percentage . |
---|---|---|
Male gender | 250 | 63.3 |
Current smoker | 66 | 16.7 |
Obesity | 57 | 14.4 |
Hypertension | 325 | 82.3 |
Diabetes | 162 | 41 |
Dyslipidemia | 205 | 51.9 |
Cancer | 32 | 8.1 |
Rheumatoid arthritis | 5 | 1.3 |
PAD | 85 | 21.5 |
Stroke | 65 | 16.5 |
Statin | 82 | 20.8 |
RAS inhibitor | 211 | 53.4 |
Categorical variables . | Number . | Percentage . |
---|---|---|
Male gender | 250 | 63.3 |
Current smoker | 66 | 16.7 |
Obesity | 57 | 14.4 |
Hypertension | 325 | 82.3 |
Diabetes | 162 | 41 |
Dyslipidemia | 205 | 51.9 |
Cancer | 32 | 8.1 |
Rheumatoid arthritis | 5 | 1.3 |
PAD | 85 | 21.5 |
Stroke | 65 | 16.5 |
Statin | 82 | 20.8 |
RAS inhibitor | 211 | 53.4 |
Continuous variables . | Median . | IQR . |
---|---|---|
Age (years) | 65 | 58–74 |
BMI (kg/m2) | 21.17 | 19.2–23.6 |
Systolic BP (mmHg) | 148 | 134–161 |
Diastolic BP (mmHg) | 77 | 67–87 |
Total protein (g/dL) | 6.6 | 6.3–7.0 |
Albumin (g/dL) | 3.8 | 3.6–4.0 |
BUN (mg/dL) | 60.7 | 52.3–71.3 |
Cr (mg/dL) | 11.09 | 9.2–12.5 |
UA (mg/dL) | 7.8 | 6.9–8.7 |
TC (mg/dL) | 151 | 131–172 |
HDLC (mg/dL) | 45 | 37–57 |
LDLC (mg/dL) | 78 | 63–94 |
Triglyceride (mg/dL) | 84 | 59–126 |
aCa (mg/dL) | 9.4 | 8.9–9.9 |
IP (mg/dL) | 5.3 | 4.6–6.2 |
Ca × P (mg/dL)2 | 48.5 | 42.2–57.0 |
Mg (mg/dL) | 2.7 | 2.5–2.9 |
AST (U/L) | 13 | 9–17 |
ALT (U/L) | 10.0 | 7–13 |
LDH (IU/L) | 182 | 163–212 |
GGT (IU/L) | 19 | 13–28 |
ALP (IU/L) | 216 | 171–279 |
T-bil (mg/dL) | 0.3 | 0.3–0.4 |
Amylase (IU/L) | 112 | 87–147 |
CK (U/L) | 86 | 58–132 |
WBC (/µL) | 5430 | 4420–6580 |
Hb (g/dL) | 10.7 | 9.9–11.4 |
Plt (×10 000/µL) | 15.9 | 12.9–19.5 |
Fe (µg/dL) | 60 | 46–79 |
Ferritin (ng/mL) | 42.6 | 23.1–92.9 |
TSAT (%) | 25 | 18–30 |
w-PTH (pg/mL) | 45 | 24–93 |
hs-CRP (ng/mL) | 706 | 295–1830 |
CTR (%) | 48.4 | 45.0–52.4 |
Vintage (years) | 5.8 | 2.9–12.2 |
Dialysis time (h) | 4.5 | 4.0–5.0 |
QB (mL/min) | 200 | 180–200 |
Kt/V | 1.44 | 1.25–1.62 |
Increasing body weight (kg) | 2.8 | 2.1–3.5 |
PTA treatment frequency | 0 | 0–0 |
Continuous variables . | Median . | IQR . |
---|---|---|
Age (years) | 65 | 58–74 |
BMI (kg/m2) | 21.17 | 19.2–23.6 |
Systolic BP (mmHg) | 148 | 134–161 |
Diastolic BP (mmHg) | 77 | 67–87 |
Total protein (g/dL) | 6.6 | 6.3–7.0 |
Albumin (g/dL) | 3.8 | 3.6–4.0 |
BUN (mg/dL) | 60.7 | 52.3–71.3 |
Cr (mg/dL) | 11.09 | 9.2–12.5 |
UA (mg/dL) | 7.8 | 6.9–8.7 |
TC (mg/dL) | 151 | 131–172 |
HDLC (mg/dL) | 45 | 37–57 |
LDLC (mg/dL) | 78 | 63–94 |
Triglyceride (mg/dL) | 84 | 59–126 |
aCa (mg/dL) | 9.4 | 8.9–9.9 |
IP (mg/dL) | 5.3 | 4.6–6.2 |
Ca × P (mg/dL)2 | 48.5 | 42.2–57.0 |
Mg (mg/dL) | 2.7 | 2.5–2.9 |
AST (U/L) | 13 | 9–17 |
ALT (U/L) | 10.0 | 7–13 |
LDH (IU/L) | 182 | 163–212 |
GGT (IU/L) | 19 | 13–28 |
ALP (IU/L) | 216 | 171–279 |
T-bil (mg/dL) | 0.3 | 0.3–0.4 |
Amylase (IU/L) | 112 | 87–147 |
CK (U/L) | 86 | 58–132 |
WBC (/µL) | 5430 | 4420–6580 |
Hb (g/dL) | 10.7 | 9.9–11.4 |
Plt (×10 000/µL) | 15.9 | 12.9–19.5 |
Fe (µg/dL) | 60 | 46–79 |
Ferritin (ng/mL) | 42.6 | 23.1–92.9 |
TSAT (%) | 25 | 18–30 |
w-PTH (pg/mL) | 45 | 24–93 |
hs-CRP (ng/mL) | 706 | 295–1830 |
CTR (%) | 48.4 | 45.0–52.4 |
Vintage (years) | 5.8 | 2.9–12.2 |
Dialysis time (h) | 4.5 | 4.0–5.0 |
QB (mL/min) | 200 | 180–200 |
Kt/V | 1.44 | 1.25–1.62 |
Increasing body weight (kg) | 2.8 | 2.1–3.5 |
PTA treatment frequency | 0 | 0–0 |
PAD, peripheral artery disease; RAS, renin–angiotensin system; IQR, interquartile range; BMI, body mass index; BP, blood pressure; BUN, blood urea nitrogen; TC, total cholesterol; HDLC, high-density lipoprotein cholesterol; LDLC, low-density lipoprotein cholesterol; aCa, adjusted calcium; IP, inorganic phosphorus; Mg, magnesium; AST, aspartate aminotransferase; ALT, alanine transaminase; LDH, lactate dehydrogenase; GGT, γ-glutamyltransferase; ALP, alkaline phosphatase; T-bil, total bilirubin; CK, creatine kinase; WBC, white blood cell; Hb, hemoglobin; plt, platelets; TSAT, transferrin saturation; w-PTH, whole parathyroid hormone, Ca × P, the product of aCa and IP; hs-CRP, high-sensitivity C-reactive protein; QB, quantity of blood flow; Kt/V, dialysis dose; PTA, percutaneous transluminal angioplasty.
Categorical variables . | Number . | Percentage . |
---|---|---|
Male gender | 250 | 63.3 |
Current smoker | 66 | 16.7 |
Obesity | 57 | 14.4 |
Hypertension | 325 | 82.3 |
Diabetes | 162 | 41 |
Dyslipidemia | 205 | 51.9 |
Cancer | 32 | 8.1 |
Rheumatoid arthritis | 5 | 1.3 |
PAD | 85 | 21.5 |
Stroke | 65 | 16.5 |
Statin | 82 | 20.8 |
RAS inhibitor | 211 | 53.4 |
Categorical variables . | Number . | Percentage . |
---|---|---|
Male gender | 250 | 63.3 |
Current smoker | 66 | 16.7 |
Obesity | 57 | 14.4 |
Hypertension | 325 | 82.3 |
Diabetes | 162 | 41 |
Dyslipidemia | 205 | 51.9 |
Cancer | 32 | 8.1 |
Rheumatoid arthritis | 5 | 1.3 |
PAD | 85 | 21.5 |
Stroke | 65 | 16.5 |
Statin | 82 | 20.8 |
RAS inhibitor | 211 | 53.4 |
Continuous variables . | Median . | IQR . |
---|---|---|
Age (years) | 65 | 58–74 |
BMI (kg/m2) | 21.17 | 19.2–23.6 |
Systolic BP (mmHg) | 148 | 134–161 |
Diastolic BP (mmHg) | 77 | 67–87 |
Total protein (g/dL) | 6.6 | 6.3–7.0 |
Albumin (g/dL) | 3.8 | 3.6–4.0 |
BUN (mg/dL) | 60.7 | 52.3–71.3 |
Cr (mg/dL) | 11.09 | 9.2–12.5 |
UA (mg/dL) | 7.8 | 6.9–8.7 |
TC (mg/dL) | 151 | 131–172 |
HDLC (mg/dL) | 45 | 37–57 |
LDLC (mg/dL) | 78 | 63–94 |
Triglyceride (mg/dL) | 84 | 59–126 |
aCa (mg/dL) | 9.4 | 8.9–9.9 |
IP (mg/dL) | 5.3 | 4.6–6.2 |
Ca × P (mg/dL)2 | 48.5 | 42.2–57.0 |
Mg (mg/dL) | 2.7 | 2.5–2.9 |
AST (U/L) | 13 | 9–17 |
ALT (U/L) | 10.0 | 7–13 |
LDH (IU/L) | 182 | 163–212 |
GGT (IU/L) | 19 | 13–28 |
ALP (IU/L) | 216 | 171–279 |
T-bil (mg/dL) | 0.3 | 0.3–0.4 |
Amylase (IU/L) | 112 | 87–147 |
CK (U/L) | 86 | 58–132 |
WBC (/µL) | 5430 | 4420–6580 |
Hb (g/dL) | 10.7 | 9.9–11.4 |
Plt (×10 000/µL) | 15.9 | 12.9–19.5 |
Fe (µg/dL) | 60 | 46–79 |
Ferritin (ng/mL) | 42.6 | 23.1–92.9 |
TSAT (%) | 25 | 18–30 |
w-PTH (pg/mL) | 45 | 24–93 |
hs-CRP (ng/mL) | 706 | 295–1830 |
CTR (%) | 48.4 | 45.0–52.4 |
Vintage (years) | 5.8 | 2.9–12.2 |
Dialysis time (h) | 4.5 | 4.0–5.0 |
QB (mL/min) | 200 | 180–200 |
Kt/V | 1.44 | 1.25–1.62 |
Increasing body weight (kg) | 2.8 | 2.1–3.5 |
PTA treatment frequency | 0 | 0–0 |
Continuous variables . | Median . | IQR . |
---|---|---|
Age (years) | 65 | 58–74 |
BMI (kg/m2) | 21.17 | 19.2–23.6 |
Systolic BP (mmHg) | 148 | 134–161 |
Diastolic BP (mmHg) | 77 | 67–87 |
Total protein (g/dL) | 6.6 | 6.3–7.0 |
Albumin (g/dL) | 3.8 | 3.6–4.0 |
BUN (mg/dL) | 60.7 | 52.3–71.3 |
Cr (mg/dL) | 11.09 | 9.2–12.5 |
UA (mg/dL) | 7.8 | 6.9–8.7 |
TC (mg/dL) | 151 | 131–172 |
HDLC (mg/dL) | 45 | 37–57 |
LDLC (mg/dL) | 78 | 63–94 |
Triglyceride (mg/dL) | 84 | 59–126 |
aCa (mg/dL) | 9.4 | 8.9–9.9 |
IP (mg/dL) | 5.3 | 4.6–6.2 |
Ca × P (mg/dL)2 | 48.5 | 42.2–57.0 |
Mg (mg/dL) | 2.7 | 2.5–2.9 |
AST (U/L) | 13 | 9–17 |
ALT (U/L) | 10.0 | 7–13 |
LDH (IU/L) | 182 | 163–212 |
GGT (IU/L) | 19 | 13–28 |
ALP (IU/L) | 216 | 171–279 |
T-bil (mg/dL) | 0.3 | 0.3–0.4 |
Amylase (IU/L) | 112 | 87–147 |
CK (U/L) | 86 | 58–132 |
WBC (/µL) | 5430 | 4420–6580 |
Hb (g/dL) | 10.7 | 9.9–11.4 |
Plt (×10 000/µL) | 15.9 | 12.9–19.5 |
Fe (µg/dL) | 60 | 46–79 |
Ferritin (ng/mL) | 42.6 | 23.1–92.9 |
TSAT (%) | 25 | 18–30 |
w-PTH (pg/mL) | 45 | 24–93 |
hs-CRP (ng/mL) | 706 | 295–1830 |
CTR (%) | 48.4 | 45.0–52.4 |
Vintage (years) | 5.8 | 2.9–12.2 |
Dialysis time (h) | 4.5 | 4.0–5.0 |
QB (mL/min) | 200 | 180–200 |
Kt/V | 1.44 | 1.25–1.62 |
Increasing body weight (kg) | 2.8 | 2.1–3.5 |
PTA treatment frequency | 0 | 0–0 |
PAD, peripheral artery disease; RAS, renin–angiotensin system; IQR, interquartile range; BMI, body mass index; BP, blood pressure; BUN, blood urea nitrogen; TC, total cholesterol; HDLC, high-density lipoprotein cholesterol; LDLC, low-density lipoprotein cholesterol; aCa, adjusted calcium; IP, inorganic phosphorus; Mg, magnesium; AST, aspartate aminotransferase; ALT, alanine transaminase; LDH, lactate dehydrogenase; GGT, γ-glutamyltransferase; ALP, alkaline phosphatase; T-bil, total bilirubin; CK, creatine kinase; WBC, white blood cell; Hb, hemoglobin; plt, platelets; TSAT, transferrin saturation; w-PTH, whole parathyroid hormone, Ca × P, the product of aCa and IP; hs-CRP, high-sensitivity C-reactive protein; QB, quantity of blood flow; Kt/V, dialysis dose; PTA, percutaneous transluminal angioplasty.
Circulating ANGPTL2 levels and mortality risk in hemodialysis patients (n = 395)
Model . | HR . | 95% CI . | P-value . |
---|---|---|---|
1 | 2.83 | 1.54–5.19 | 0.001 |
2 | 2.32 | 1.43–3.75 | 0.001 |
3 | 2.04 | 1.10–3.77 | 0.023 |
Model . | HR . | 95% CI . | P-value . |
---|---|---|---|
1 | 2.83 | 1.54–5.19 | 0.001 |
2 | 2.32 | 1.43–3.75 | 0.001 |
3 | 2.04 | 1.10–3.77 | 0.023 |
HR of log(ANGPTLT2) for mortality, 95% CI and P-value are indicated. Model 1: crude. Model 2: multivariate Cox PH model adjusted for age, sex, smoking status, diabetes, hypertension, dyslipidemia, BMI, cancer, hemodialysis vintage, albumin, CTR, Ca × P and medical facility location. Model 3: Model 2 plus hs-CRP. hs-CRP and ANGPTL2 were transformed to natural log values.
CI, confidence interval; BMI, body mass index; Ca × P, a product of adjusted calcium and inorganic phosphorus.
Circulating ANGPTL2 levels and mortality risk in hemodialysis patients (n = 395)
Model . | HR . | 95% CI . | P-value . |
---|---|---|---|
1 | 2.83 | 1.54–5.19 | 0.001 |
2 | 2.32 | 1.43–3.75 | 0.001 |
3 | 2.04 | 1.10–3.77 | 0.023 |
Model . | HR . | 95% CI . | P-value . |
---|---|---|---|
1 | 2.83 | 1.54–5.19 | 0.001 |
2 | 2.32 | 1.43–3.75 | 0.001 |
3 | 2.04 | 1.10–3.77 | 0.023 |
HR of log(ANGPTLT2) for mortality, 95% CI and P-value are indicated. Model 1: crude. Model 2: multivariate Cox PH model adjusted for age, sex, smoking status, diabetes, hypertension, dyslipidemia, BMI, cancer, hemodialysis vintage, albumin, CTR, Ca × P and medical facility location. Model 3: Model 2 plus hs-CRP. hs-CRP and ANGPTL2 were transformed to natural log values.
CI, confidence interval; BMI, body mass index; Ca × P, a product of adjusted calcium and inorganic phosphorus.
Establishment of prognostic profiles of hemodialysis patients
Next, to define the clinical characteristics of patients in whom there was a strong association between circulating ANGPTL2 levels and mortality risk, we performed stratified analysis using a variable that indicated a patient’s prognostic profile. We thus generated a synthetic stratum variable indicative of the patient’s mortality risk by using 52 variables collected from routine medical assessment (Table 1). In generating the stratum variable, we first performed random forest analysis to rank variables according to the strength of the association with mortality risk. After clinical consideration, we selected the 10 highest-ranking, i.e. strongest, risk factors as prognostic indicators (Supplementary Table S1). We then used the survival tree method to categorize patients into nine groups, compared the HRs among these nine groups and finally combined the HRs to obtain a parsimonious model called the ‘prognostic stage’, which consists of four prognostic strata (Supplementary Figure S2 and Table S2). Patients were divided into three groups: a young group (age ≤69 years), a ‘middle age to elderly’ group (age 70–80 years) and the ‘most elderly’ group (age ≥81 years) (Supplementary Figure S2). In the young group, patients with the most benign prognostic profile were assigned prognostic stage 1 (Supplementary Figure S2) and decreased levels of circulating creatinine (Cr; <9.7 mg/dL) or uric acid (UA; ≤7.5 mg/dL) were selected as indicators of worsening prognosis. Patients in the middle age to elderly group were assigned prognostic stage 2 or higher and indicators of worsening prognosis for these patients included cardiomegaly (CTR >52.4%) and decreased circulating levels of UA (≤6.3 mg/dL) (Supplementary Figure S2). Finally, patients in the most elderly group were assigned prognostic stages 2 and 4. In this group, higher hs-CRP (>992.3 ng/mL) or higher ferritin (>40 ng/mL) levels were significant indicators of high mortality risk (Supplementary Figure S2). Overall, prognostic stages were defined as stage 1, patients ≤69 years of age with Cr levels ≥9.7 mg/dL and UA levels >7.5 mg/dL; stage 2, patients ≤69 years of age with Cr levels ≥9.7 mg/dL and UA levels ≤7.5 mg/dL, patients ≤69 years of age with Cr levels <9.7 mg/dL, patients 70–80 years of age with CTR <52.4% and UA levels >6.3 mg/dL or patients ≥81 years of age with hs-CRP levels ≤992.3 ng/mL and ferritin levels ≤40 ng/mL; stage 3, patients 70–80 years of age with either CTR <52.4% and UA levels ≤6.3 mg/dL or with CTR >52.4%; and stage 4, patients ≥81 years of age with either hs-CRP levels ≤992.3 ng/mL and ferritin levels >40 ng/mL or with hs-CRP levels >992.3 ng/mL (Supplementary Figure S2).
Kaplan–Meier survival curves for patients at each prognostic stage are shown in Figure 2. The Cox PH model and corresponding bootstrap replication analysis also revealed that the HR for patient mortality risk increased with advanced prognostic stage (Supplementary Table S3). In addition, circulating ANGPTL2 levels also increased with advanced prognostic stage (Supplementary Figure S3). Taken together, these data suggest that the prognostic stages established here are useful as statistical adjustment to suppress confounding factors when evaluating the relationship between circulating ANGPTL2 levels and mortality risk. Thus we conducted multivariate survival analysis to evaluate this relationship, following adjustment using our prognostic staging.
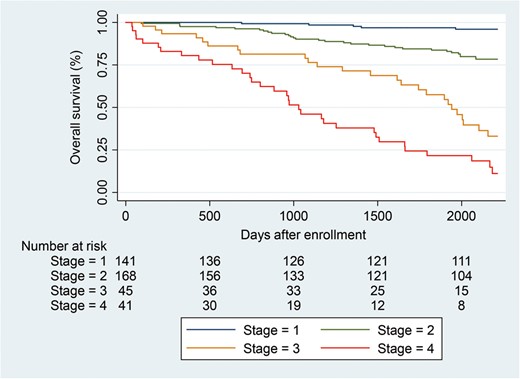
Kaplan–Meier survival curve of patients receiving hemodialysis at each prognostic stage. Table below the graph indicates the number of at-risk patients at each prognostic stage. Blue line, stage 1; green line, stage 2; yellow line, stage 3; red line, stage 4.
Clinical characteristics of patients whose circulating ANGPTL2 levels are strongly associated with mortality risk
We next analyzed a potential association between circulating ANGPTL2 levels and mortality risk at each prognostic stage using the Cox PH model and bootstrap analysis. This analysis revealed that high circulating ANGPTL2 levels were significantly associated with an increased mortality risk in hemodialysis patients with a relatively benign prognostic profile, i.e. those assigned prognostic stages 1, 2 and 3, but this association was not significant in the highest-risk group, i.e. those assigned prognostic stage 4 (Table 3). To simplify the clinical interpretation of this analysis, we next evaluated the combined HR of circulating ANGPTL2 levels for mortality risk at prognostic stages 1, 2 and 3, but not stage 4, and found the combined HR for mortality risk was significant (Table 3). In addition, residual analysis of this model revealed that ANGPTL2 levels and the HR for mortality risk were almost linearly associated (Supplementary Figure S4). In contrast, the association between circulating ANGPTL2 levels and mortality risk was weaker in prognostic stage 4 patients, who had poor prognosis and exhibited aging-related phenotypes such as older age and elevated circulating hs-CRP or ferritin levels (Table 3 and Supplementary Figure S2). Next, to determine whether the relationship between circulating ANGPTL2 levels and mortality risk was influenced by aging-related factors, we conducted stratification analyses on patients assigned prognostic stages 1, 2 and 3. These analyses revealed that there was a strong association between high circulating ANGPTL2 levels and increased mortality risk, especially in subjects with fewer aging-related phenotypes such as those younger than the median age (<63 years), those in the group without diabetes and those on shorter dialysis vintage than the median (<6.2 years) (Table 4). In addition, our analysis showed that these aging-related parameters (age, complications of diabetes and longer hemodialysis vintage) were positively correlated with circulating ANGPTL2 levels (Supplementary Table S4).
Relationship between circulating ANGPTL2 levels and HR for mortality risk in hemodialysis patients at each prognostic stage
Prognostic stage . | Number at risk . | Number of events . | HR . | 95% CI . | P-value . | HR (BS) . | 95% CI (BS) . |
---|---|---|---|---|---|---|---|
1 | 141 | 5 | 8.88 | (5.35–14.74) | <0.001 | 8.25 | (1.61–3.04) |
2 | 168 | 31 | 1.72 | (1.20–2.49) | 0.004 | 1.65 | (1.21–2.51) |
3 | 45 | 25 | 6.56 | (1.93–22.26) | 0.003 | 6.37 | (2.07–25.57) |
(Combined stages 1, 2 and 3) | 354 | 61 | 3.06 | (1.86–5.03) | <0.001 | 2.90 | (1.72–4.92) |
4 | 41 | 33 | 1.47 | (0.78–2.79) | 0.235 | 1.44 | (0.78–2.97) |
Prognostic stage . | Number at risk . | Number of events . | HR . | 95% CI . | P-value . | HR (BS) . | 95% CI (BS) . |
---|---|---|---|---|---|---|---|
1 | 141 | 5 | 8.88 | (5.35–14.74) | <0.001 | 8.25 | (1.61–3.04) |
2 | 168 | 31 | 1.72 | (1.20–2.49) | 0.004 | 1.65 | (1.21–2.51) |
3 | 45 | 25 | 6.56 | (1.93–22.26) | 0.003 | 6.37 | (2.07–25.57) |
(Combined stages 1, 2 and 3) | 354 | 61 | 3.06 | (1.86–5.03) | <0.001 | 2.90 | (1.72–4.92) |
4 | 41 | 33 | 1.47 | (0.78–2.79) | 0.235 | 1.44 | (0.78–2.97) |
The Cox PH model was used and adjusted for prognostic stage and medical facility location (n = 395). Combined analysis of prognostic stages 1, 2 and 3 was adjusted for prognostic stage and medical facility location (n = 354).
CI, confidence interval; HR (BS), average HR estimated by bootstrap replication analysis; 95% CI (BS), 95% CI estimated by bootstrap replication analysis.
Relationship between circulating ANGPTL2 levels and HR for mortality risk in hemodialysis patients at each prognostic stage
Prognostic stage . | Number at risk . | Number of events . | HR . | 95% CI . | P-value . | HR (BS) . | 95% CI (BS) . |
---|---|---|---|---|---|---|---|
1 | 141 | 5 | 8.88 | (5.35–14.74) | <0.001 | 8.25 | (1.61–3.04) |
2 | 168 | 31 | 1.72 | (1.20–2.49) | 0.004 | 1.65 | (1.21–2.51) |
3 | 45 | 25 | 6.56 | (1.93–22.26) | 0.003 | 6.37 | (2.07–25.57) |
(Combined stages 1, 2 and 3) | 354 | 61 | 3.06 | (1.86–5.03) | <0.001 | 2.90 | (1.72–4.92) |
4 | 41 | 33 | 1.47 | (0.78–2.79) | 0.235 | 1.44 | (0.78–2.97) |
Prognostic stage . | Number at risk . | Number of events . | HR . | 95% CI . | P-value . | HR (BS) . | 95% CI (BS) . |
---|---|---|---|---|---|---|---|
1 | 141 | 5 | 8.88 | (5.35–14.74) | <0.001 | 8.25 | (1.61–3.04) |
2 | 168 | 31 | 1.72 | (1.20–2.49) | 0.004 | 1.65 | (1.21–2.51) |
3 | 45 | 25 | 6.56 | (1.93–22.26) | 0.003 | 6.37 | (2.07–25.57) |
(Combined stages 1, 2 and 3) | 354 | 61 | 3.06 | (1.86–5.03) | <0.001 | 2.90 | (1.72–4.92) |
4 | 41 | 33 | 1.47 | (0.78–2.79) | 0.235 | 1.44 | (0.78–2.97) |
The Cox PH model was used and adjusted for prognostic stage and medical facility location (n = 395). Combined analysis of prognostic stages 1, 2 and 3 was adjusted for prognostic stage and medical facility location (n = 354).
CI, confidence interval; HR (BS), average HR estimated by bootstrap replication analysis; 95% CI (BS), 95% CI estimated by bootstrap replication analysis.
Relationship between circulating ANGPTL2 levels and HR for mortality risk in hemodialysis patients according to aging-related parameters
Stratum . | Number at risk . | Number of events . | HR . | 95% CI . | P-value . |
---|---|---|---|---|---|
Age (years) | |||||
<63 | 157 | 13 | 7.99 | (3.55–18.01) | <0.001 |
≥63 | 197 | 48 | 2.02 | (0.76–5.33) | 0.157 |
Diabetes | |||||
No | 209 | 32 | 5.15 | (3.19–8.32) | <0.001 |
Yes | 145 | 29 | 1.48 | (0.74–2.88) | 0.278 |
Vintage (years) | |||||
<6.2 | 177 | 30 | 3.99 | (2.85–5.58) | <0.001 |
≥6.2 | 177 | 31 | 2.17 | (1.01–4.68) | 0.047 |
Stratum . | Number at risk . | Number of events . | HR . | 95% CI . | P-value . |
---|---|---|---|---|---|
Age (years) | |||||
<63 | 157 | 13 | 7.99 | (3.55–18.01) | <0.001 |
≥63 | 197 | 48 | 2.02 | (0.76–5.33) | 0.157 |
Diabetes | |||||
No | 209 | 32 | 5.15 | (3.19–8.32) | <0.001 |
Yes | 145 | 29 | 1.48 | (0.74–2.88) | 0.278 |
Vintage (years) | |||||
<6.2 | 177 | 30 | 3.99 | (2.85–5.58) | <0.001 |
≥6.2 | 177 | 31 | 2.17 | (1.01–4.68) | 0.047 |
Patients assigned prognostic stages 1, 2 and 3 were analyzed (n = 354). The Cox PH model was used and adjusted for prognostic stage and medical facility location. ANGPTL2 levels were transformed to natural log values.
CI, confidence interval.
Relationship between circulating ANGPTL2 levels and HR for mortality risk in hemodialysis patients according to aging-related parameters
Stratum . | Number at risk . | Number of events . | HR . | 95% CI . | P-value . |
---|---|---|---|---|---|
Age (years) | |||||
<63 | 157 | 13 | 7.99 | (3.55–18.01) | <0.001 |
≥63 | 197 | 48 | 2.02 | (0.76–5.33) | 0.157 |
Diabetes | |||||
No | 209 | 32 | 5.15 | (3.19–8.32) | <0.001 |
Yes | 145 | 29 | 1.48 | (0.74–2.88) | 0.278 |
Vintage (years) | |||||
<6.2 | 177 | 30 | 3.99 | (2.85–5.58) | <0.001 |
≥6.2 | 177 | 31 | 2.17 | (1.01–4.68) | 0.047 |
Stratum . | Number at risk . | Number of events . | HR . | 95% CI . | P-value . |
---|---|---|---|---|---|
Age (years) | |||||
<63 | 157 | 13 | 7.99 | (3.55–18.01) | <0.001 |
≥63 | 197 | 48 | 2.02 | (0.76–5.33) | 0.157 |
Diabetes | |||||
No | 209 | 32 | 5.15 | (3.19–8.32) | <0.001 |
Yes | 145 | 29 | 1.48 | (0.74–2.88) | 0.278 |
Vintage (years) | |||||
<6.2 | 177 | 30 | 3.99 | (2.85–5.58) | <0.001 |
≥6.2 | 177 | 31 | 2.17 | (1.01–4.68) | 0.047 |
Patients assigned prognostic stages 1, 2 and 3 were analyzed (n = 354). The Cox PH model was used and adjusted for prognostic stage and medical facility location. ANGPTL2 levels were transformed to natural log values.
CI, confidence interval.
DISCUSSION
We conducted a prospective cohort study to assess whether circulating ANGPTL2 levels are associated with mortality risk in patients receiving hemodialysis treatment. We first demonstrated that high circulating ANGPTL2 levels are a significant risk factor for mortality. Next, exploratory stratified analysis revealed that high circulating ANGPTL2 levels are associated with high mortality risk, particularly in patients with a relative benign prognostic profile and fewer aging-related phenotypes. To our knowledge, this study is the first to show that circulating ANGPTL2 levels can predict clinically important outcomes in the uremic population.
Circulating ANGPTL2 levels and mortality risk in patients undergoing hemodialysis
In this study, multivariate survival analysis revealed a significant relationship between high circulating ANGPTL2 levels and increased mortality risk after adjustment for age, sex, hemodialysis vintage, nutritional status and metabolic parameters such as complications of diabetes, dyslipidemia and hypertension. Moreover, this relationship remained significant after further adjustment for hs-CRP-related inflammatory status. Previously our group reported that excess ANGPTL2 secretion promotes progression of aging-related diseases such as metabolic syndrome, sarcopenia, cancer, CKD and atherosclerosis in animal models, as well as in vitro [11–16, 21]. In the progression of aging-related diseases, ANGPTL2 is a potent accelerator of aging-related phenotypes through activation of reactive oxygen species or signaling through transforming growth factor β or nuclear factor κB [9, 12, 13, 16]. These reports are in agreement with our current data on uremic patients who exhibit phenotypes of accelerated premature aging compared with healthy individuals. Our analysis suggests that circulating ANGPTL2 levels reflect the progression of premature aging in uremic individuals.
Interestingly, our analysis showed a significant relationship between high circulating ANGPTL2 levels and increased mortality risk in patients with a relatively benign prognostic profile (prognostic stages 1, 2 and 3), unlike in the middle age to elderly group (prognostic stage 4). Accordingly, our exploratory analysis revealed that this association persisted in patients showing fewer aging-related phenotypes, including younger patients, individuals without diabetes and those on shorter hemodialysis vintage. Evaluating mortality risk is difficult in hemodialysis patients, particularly those who do not exhibit poor prognostic/aging-related indicators such as aging, accelerated inflammation, excess intracorporeal Fe, long hemodialysis vintage or diabetes complications [22, 23]. Although both ANGPTL2 and CRP are inflammatory markers, calculation of Spearman’s correlation coefficient between circulating ANGPTL2 levels and hs-CRP at baseline indicated a significant, but not strongly positive correlation (correlation coefficient 0.27; P < 0.001). Furthermore, our finding of a significant association between high circulating ANGPTL2 levels and increased mortality risk in patients undergoing hemodialysis after adjustment for confounding factors, including hs-CRP levels, supports the idea that circulating ANGPTL2 levels could serve as a useful, unique biomarker of mortality risk that is not detected by analysis of hs-CRP levels. However, we also noted that in the most elderly group showing significant aging-related phenotypes, including those with diabetes or on prolonged hemodialysis, circulating ANGPTL2 levels did not predict mortality risk, for reasons that are unknown and require further investigation.
Next, based on our preliminary analysis in this study, circulating ANGPTL2 levels in uremic patients were higher compared with a general Japanese population in the Hisayama study, based on multivariate analysis and adjusted for age and gender [17, 18]. Further investigations using the same assay conditions on one cohort are needed to determine whether ANGPTL2 levels in uremic populations are increased compared with healthy subjects.
Prognostic staging of patients receiving hemodialysis
Survival tree analysis (Supplementary Figure S2) revealed that decreased levels of Cr (<9.7 mg/dL at ages ≤69 years) or UA (≤7.5 mg/dL at ages ≤69 years or ≤6.3 mg/dL at ages 70–80 years) were indicators of poor prognosis. Others reported that high circulating Cr levels are associated with lowered mortality risk in hemodialysis patients [22, 24, 25]. Circulating Cr levels have also been shown to reflect changes in muscle mass as a result of imbalanced anabolic/catabolic metabolism in uremic individuals [25]. In addition, others reported that high circulating UA levels are associated with reduced mortality risk in hemodialysis patients and suggested that UA levels may reflect patient nutritional status [26–28]. Moreover, UA has been shown to counteract the adverse effects of uremic toxins such as indoxyl sulfate through antioxidant activity or by enhancing nitric oxide bioavailability in vascular endothelial cells [26, 29]. These reports are in agreement with our data, which indicate that decreased circulating Cr or UA levels are associated with higher all-cause mortality risk in hemodialysis patients. Next, results of our survival tree analysis (Supplementary Figure S2) also indicated that increased CTR (>52.4% at ages 70–80 years) is an indicator of poor prognosis. These findings are supported by a previous report indicating that cardiomegaly increased mortality risk in uremic patients [30, 31]. Finally, high hs-CRP levels (>992.3 ng/mL at ages ≥81 years) or high ferritin levels (>40 ng/mL at ages ≥81 years) were significant indicators of increased mortality risk, which is consistent with previous reports showing that circulating CRP or ferritin levels were significantly associated with an increased risk of mortality in uremic patients [23, 32, 33]. In the most elderly age group (≥81 years), patients may be more vulnerable to inflammatory stress due to either uremia or infection or due to the presence of excess intracorporeal Fe as a result of decreased Fe bioavailability or excess Fe loading.
Taken together, prognostic staging of uremic patients in this study indicates that prognostic risk factors differ across age groups, which is an important factor to consider when assessing mortality risk.
A limitation of our study is that we did not assess whether circulating ANGPTL2 levels could predict cause-specific, including cardiovascular-, cancer- and infection-related, mortality risk. Moreover, using clinical data, we determined ANGPTL2 levels at baseline and did not examine any association between time-dependent changes in ANGPTL2 levels and clinical outcomes. Further clinical cohort studies using larger sample sizes would be needed to assess uremic hemodialysis patients.
In summary, we demonstrated that circulating levels of the aging-associated factor ANGPTL2 are significantly associated with mortality risk in patients receiving hemodialysis who have a relatively benign prognostic profile. We conclude that ANGPTL2 could serve as a biomarker of progression of premature aging in uremic patients, except those with significant aging-related phenotypes.
SUPPLEMENTARY DATA
Supplementary data are available at ndt online.
ACKNOWLEDGEMENTS
The authors thank N. Nakagawa, K. Saito, N. Hirano and H. Shibuta for technical assistance. The authors also thank Rika Yamazoe, MD for supporting data collection.
FUNDING
This work was supported by JSPS KAKENHI (grant number 17K09703 to J.M. and grant number 17K09706 to M.M.).
CONFLICT OF INTEREST STATEMENT
None declared.
REFERENCES
United States Renal Data System. Annual Data Report: Epidemiology of Kidney Disease in the United States. https://www.usrds.org/. https://www.usrds.org/. (29 May 2019, date last accessed)
Comments