-
PDF
- Split View
-
Views
-
Cite
Cite
Ying Yi, Yue Zhao, Chunhua Li, Lin Zhang, Huiying Huang, Yana Li, Lanlan Liu, Ping Hou, Tianyu Cui, Puwen Tan, Yongfei Hu, Ting Zhang, Yan Huang, Xiaobo Li, Jia Yu, Dong Wang, RAID v2.0: an updated resource of RNA-associated interactions across organisms, Nucleic Acids Research, Volume 45, Issue D1, January 2017, Pages D115–D118, https://doi.org/10.1093/nar/gkw1052
- Share Icon Share
Abstract
With the development of biotechnologies and computational prediction algorithms, the number of experimental and computational prediction RNA-associated interactions has grown rapidly in recent years. However, diverse RNA-associated interactions are scattered over a wide variety of resources and organisms, whereas a fully comprehensive view of diverse RNA-associated interactions is still not available for any species. Hence, we have updated the RAID database to version 2.0 (RAID v2.0, www.rna-society.org/raid/) by integrating experimental and computational prediction interactions from manually reading literature and other database resources under one common framework. The new developments in RAID v2.0 include (i) over 850-fold RNA-associated interactions, an enhancement compared to the previous version; (ii) numerous resources integrated with experimental or computational prediction evidence for each RNA-associated interaction; (iii) a reliability assessment for each RNA-associated interaction based on an integrative confidence score; and (iv) an increase of species coverage to 60. Consequently, RAID v2.0 recruits more than 5.27 million RNA-associated interactions, including more than 4 million RNA–RNA interactions and more than 1.2 million RNA–protein interactions, referring to nearly 130 000 RNA/protein symbols across 60 species.
INTRODUCTION
Recent developments have indicated that diverse RNA-associated (RNA–RNA/RNA–Protein) interactions are also fundamental to cellular processes like protein–protein interactions. They are also essential for a system-level understanding of cellular behavior (1–4). Hence, in recent years, a wide variety of experimental and computational prediction techniques have expanded a number of diverse RNA-associated interaction data sets. Most of these interactions are available in a variety of databases (5–9), including several databases that primarily manually collect and curate diverse RNA-associated interactions with experimental evidence from literature. Other databases focus on a more generalized perspective for diverse RNAs and their partners in specific cellular processes. Another resource predicts diverse RNA-associated interactions using computational prediction algorithms. However, a fully comprehensive view of diverse RNA-associated interactions is still not available for any particular species.
Because the comprehensive regulation of crosstalk between diverse RNA and proteins still remains ambiguous, we updated the RAID database (5) to version 2.0 (RAID v2.0, http://www.rna-society.org/raid/) by integrating experimental and computational prediction interactions through the manual curation of the literature and another 18 resources under one common framework (Figure 1). Accordingly, RAID v2.0 will offer several distinctive advantages: (i) integration from numerous resources, including experimental and computational prediction databases as well as manual curation of the literature (recruiting more than 5.27 million RNA-associated interactions and exceeding an 850-fold increase over the previous version); (ii) provision of an integrative confidence score for each RNA-associated interaction, considering that an integrated scoring strategy will offer higher confidence when independent types of evidence agree; and (iii) mapping RNA-associated interactions into numerous species to facilitate studies of homology (increased coverage across 60 species).
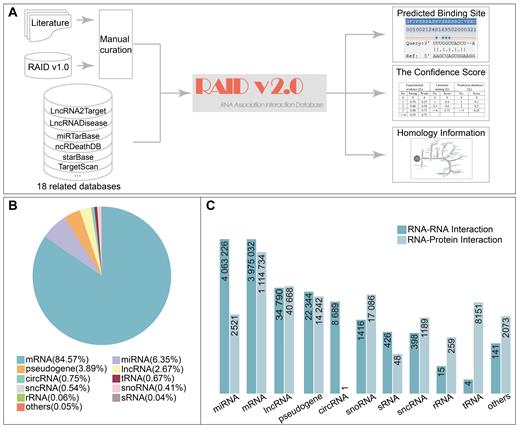
Flowchart of database construction and the statistics of RNA categories and interactions. (A) The overview of the RAID v2.0 database; (B) The percentage of diverse RNA categories in RAID v2.0 database; (C) The number of RNA–RNA/RNA–protein interactions for diverse RNA categories in RAID v2.0 database, the height of histogram transformed by log10.
DATA COLLECTION
To update this version of the RAID database, we first screened all of the literature in the PubMed database (mainly from 2000–2016) with the following keywords combinations: (i) RNA–RNA interactions: (RNA symbols or RNA category names) and (RNA symbols or RNA category names) and (e.g. interaction or binding); (ii) RNA–protein interactions: (RNA symbols or RNA category names) and (protein symbols) and (e.g. interaction or binding). The relevant hits were downloaded and prepared systematically for further manual data curation. Second, RAID v2.0 integrated diverse RNA-associated interactions from other 18 databases, including ChIPBase (10), LncRNA2Target (11), LncRNAdisease (7), miR2Disease (12), miRTarBase (13), MNDR (14), ncRDeathDB (8), NPInter (15), OncomiRDB (16), sRNATarBase (17), StarBase (6), TransmiR (18) and ViRBase (19) as well as five computational prediction databases (DroID (20), EIMMo (21), miRanda (22), miRDB (23) and TargetScan (9)).
For the RNA/protein names collected from different resources, RAID v2.0 mapped these symbols to either an official gene Symbol or a miRBase ID and presented them to NCBI Alias, HGNC ID, Ensembl ID, OMIM ID, HPRD ID and UniProtKB protein accession, among others. Furthermore, to facilitate researcher access to information from external resources, we also linked Entrez ID, miRBase accession and UniprotKB protein accession to the NCBI Gene, miRBase database and UniProt (24) database, which can efficiently retrieve a substantial amount of genomic-associated data from external resources.
INTEGRATIVE CONFIDENCE SCORES
In RAID v2.0, the RNA-associated interactions are collected from different types of resources under one common framework, including experimental, literature mining and computational prediction evidence. Furthermore, similar to miRTarBase database, the experimental evidence in RAID v2.0 was divided into strong experimental evidence (e.g. RNA immunoprecipitation and luciferase reporter assay) and weak experimental evidence (e.g. ChIP-seq and CLIP-seq) by a manual assignment, depending on the nature and qualitative annotation of the experiment method. Because multiple types of evidence contribute to the identification of a specific RNA-associated interaction, the RNA-associated interactions stored in RAID v2.0 are not equally reliable. Because it is difficult for a user to assess the quality of each interaction, we developed an integrative confidence score system to facilitate the evaluation of the reliability of each RNA-associated interaction (25). An integrative confidence score that combines scores from all of these evidence resources can give an overall estimation of the reliability of each RNA-associated interaction.
DATABASE CONTENT AND CONSTRUCTION
In total, RAID v2.0 recruits 5,272,396 RNA-associated entries (an over 850-fold increase from the previous version), including over 4 million RNA–RNA interactions and over 1.2 million RNA–protein interactions, referring to 129 857 RNA/protein symbols. RAID v2.0 involves at least 13 RNAs (including circRNA, lncRNA, miRNA, mRNA, miscRNA, pseudogenes, rRNA, scRNA, sncRNA, snoRNA, snRNA, sRNA and tRNA) and contains up to 60 species covering seven categories (bacteria, fungi, insects, nematodes, plants, vertebrates and viruses). More importantly, each RNA-associated interaction in RAID v2.0 is provided with an integrative confidence score. The user can select RNA-associated interactions by a user-specific threshold.
A ‘Homology’ option has been added to the ‘Detail Information’ page to help users investigate the conservation of RNA-associated interactions between RNA orthology/paralogy obtained from miRBase and NCBI HomoloGene (Supplementary Figure S2). In the current version, there are more than 80 000 RNAs/proteins with homology information.
In RAID v2.0, we have also modified the display of the predicted binding sites for RNA-associated interactions because several tools used in the previous version were not available. For RNA–RNA interactions, the binding sites and scores are predicted according to miRanda (22) and RISearch (26). For RNA–protein interactions, PRIdictor is used to predict RNA-binding residues in proteins. Additionally, RAID v2.0 have represented the experimental verified RNA-binding sites in proteins documented in RBPDB (27), RsiteDB (28) and PDB (29) databases (Supplementary Figure S2).
On the updated ‘Browse’ page, users can access RAID v2.0 via three different paths: ‘Interaction Type’, ‘Species’ and ‘Detection Methods’. For user convenience, we have designed the treeview and users can obtain browse results by clicking the node.
CONCLUSION AND FUTURE DIRECTIONS
In past decades, numerous protein–protein interactions databases have been established, including the most widely used STRING database. This has led to a more comprehensive understanding of protein functions and cellular processes. However, recent developments have indicated that protein–protein interactions represent perhaps only half of the story in cells. The RNA-associated interactome is likely to be much larger and more complex than we can imagine. Currently, diverse RNA-associated interactions are scattered over a wide variety of resources and organisms. A fully comprehensive view of all diverse RNA-associated interactions is still not available for any species. Consequently, we have updated the RAID database to version 2.0 by integrating manually reading literature and 18 other database resources under one common framework and providing an integrative confidence score for each RNA-associated interaction. RAID v2.0 aims to provide a comprehensive and reliably assessed collection of RNA-associated interactions across organisms. Furthermore, because each RNA-associated interaction has an integrative confidence score, users can filter the diverse RNA-associated interaction network at any threshold.
In the future, we will expand the database with more information, including RNA binding domain annotation, 2D and 3D RNA structures and improvement of the current computational prediction algorithm to obtain our own predicted data. With the emergence of more RNA-related information, we may improve the integrative confidence scoring strategy. We will keep a watchful eye on new research progress and will continuously curate and update the reference data. Hence, complemented by the successful PPI databases, RAID will provide a valuable skeleton for better understanding the functional organization of the cell.
SUPPLEMENTARY DATA
Supplementary Data are available at NAR Online.
FUNDING
Natural Science Foundation of Heilongjiang Province of China [C2015027]; Scientific Research Fund of Heilongjiang Provincial Education Department [12541426]; WeihanYu Youth Science Fund Project of Harbin Medical University. Funding for open access charge: Natural Science Foundation of Heilongjiang Province of China [C2015027]; Scientific Research Fund of Heilongjiang Provincial Education Department [12541426]; WeihanYu Youth Science Fund Project of Harbin Medical University.
Conflict of interest statement. None declared.
Comments