-
PDF
- Split View
-
Views
-
Cite
Cite
Matthew C. LaFave, Gaurav K. Varshney, Derek E. Gildea, Tyra G. Wolfsberg, Andreas D. Baxevanis, Shawn M. Burgess, MLV integration site selection is driven by strong enhancers and active promoters, Nucleic Acids Research, Volume 42, Issue 7, 1 April 2014, Pages 4257–4269, https://doi.org/10.1093/nar/gkt1399
- Share Icon Share
Abstract
Retroviruses integrate into the host genome in patterns specific to each virus. Understanding the causes of these patterns can provide insight into viral integration mechanisms, pathology and genome evolution, and is critical to the development of safe gene therapy vectors. We generated murine leukemia virus integrations in human HepG2 and K562 cells and subjected them to second-generation sequencing, using a DNA barcoding technique that allowed us to quantify independent integration events. We characterized >3 700 000 unique integration events in two ENCODE-characterized cell lines. We find that integrations were most highly enriched in a subset of strong enhancers and active promoters. In both cell types, approximately half the integrations were found in <2% of the genome, demonstrating genomic influences even narrower than previously believed. The integration pattern of murine leukemia virus appears to be largely driven by regions that have high enrichment for multiple marks of active chromatin; the combination of histone marks present was sufficient to explain why some strong enhancers were more prone to integration than others. The approach we used is applicable to analyzing the integration pattern of any exogenous element and could be a valuable preclinical screen to evaluate the safety of gene therapy vectors.
INTRODUCTION
Retroviruses have played important roles in pathology (1) and genome evolution (2–4). In practice, the introduction of exogenous DNA into host cell chromosomes has both experimental and medical utility: it can be used to disrupt endogenous genes, as in insertional mutagenesis screens (5), as well as to introduce functional alleles in the context of gene therapy (6). In both cases, an understanding of the integration pattern of the vector of choice is important for proper experimental design. Our understanding of the integration preferences for different insertional elements is rapidly changing. Initially, because of a lack of data to prove otherwise, it was assumed that retroviral integration occurred randomly (7,8). With the completion of the human genome and more efficient sequencing technologies, it became possible to map many hundreds of integration events, and data quickly emerged that demonstrated that viral integrations were non-random and specific to the viral subtypes (9–12). These original findings were still severely limited because functional genome annotation beyond transcribed genes was essentially non-existent. Since that time, more chromatin features have been identified, along with tens of thousands of integration sites (13). This has led to the inference that murine leukemia virus (MLV) integrates near regulatory elements like promoters and enhancers (14). Consistent with this, previous studies have detected associations between MLV integration and individual chromatin marks (15,16). In addition, MLV integration data have been correlated with areas of active chromatin represented by DNAse hypersensitive sites (DHS) (17,18).
Annotations from the ENCODE pilot regions were used in conjunction with pyrosequencing to analyze human immunodeficiency virus integration site selection (19). Now, the ENCODE consortium has carried out detailed studies that deeply characterize and annotate not just the pilot regions, but the entire genome of a select number of cell lines (20,21). These data potentially give researchers a comprehensive set of annotations that allows for high-resolution examination of the specific genomic features that drive viral integration site selection, or the site selection of any integrating element. Key to using this data set to its fullest is the need to map large numbers of independent integration events.
We set out to define the underlying drivers of MLV integration site selection by generating an ultra high-density map of integrations in two ENCODE-characterized cell lines. We isolated and mapped >3.7 million MLV integrations, data that are ∼100 times more dense than the largest published MLV data set (14). We found that MLV exhibited a marked preference not simply for active chromatin, but for a specific subset of enhancers and promoters that have high enrichment for a specific combination of histone marks. This preference for active promoters or enhancers was >2-fold higher than for generic markers of open chromatin such as DHS, or for a separate class of strong enhancers defined by the Broad ChromHMM predictions (21).
MATERIALS AND METHODS
Viral infection
The K562 cells were a gift from D. Bodine, and HepG2 cells from S. Rane. The Moloney MLV was prepared as described by Jao et al. (22). Two BD Falcon T175 flasks of HepG2 cells, containing 3 × 106 and 5 × 106 cells, were resuspended and infected with the virus-containing media for 24 h. In the second experiment, we infected four T75 flasks of K562 cells. We infected 1 × 106 and 2 × 106 K562 cells with supernatant from MLV producer clone GT186 for 4 h, and then transferred the virus-containing media to two new flasks of 1 × 106 and 2 × 106 K562 cells and incubated the flasks overnight. The time from infection to harvesting of each of the six flasks was 24 h; as such, the cells were expected to divide no more than once. We recovered genomic DNA by using the DNeasy Blood & Tissue Kit (Qiagen; Valencia, CA, USA).
Linker-mediated polymerase chain reaction
We amplified fragments containing the 3′-end of the MLV provirus using the method of Varshney et al. (5). Integrations from HepG2 and K562 cells were amplified separately. We split the DNA from each flask into one-third, each of which was digested overnight with a combination of MseI/PstI, BfaI/BanII (New England Biolabs; Ipswich, MA, USA) or Csp6I/Eco24I (Fermentas; Hanover, MD, USA). Each cocktail consisted of a 6-bp cutter that prevents internal amplification by cutting downstream of the MLV 5′ long terminal repeat (LTR), and a 4-bp cutter that produces a 5′ TA overhang. We pooled digested DNA and annealed 6-bp barcoded linkers to the TA overhangs with T4 DNA ligase (Invitrogen; Grand Island, NY, USA). Generic oligo sequences used to make the linkers are as follows: linker oligo A: 5′-TAGNNNNNNTATGCGCAGTTTTTTTGCAAAAA-3′ and linker oligo B: 5′-GTAATACGACTCACTATAGGGCACGCGTGGTCGACTGCGCATNNNNNNC-3′; here, ‘N’ denotes bases in the 6-bp barcode that varied among the 960 unique barcodes. We pooled 480 barcoded linkers for each flask (two) of HepG2 cells and 216 barcodes for each flask (four) of K562 cells. We then performed linker-mediated polymerase chain reaction (LM-PCR) using the pooled linkers. In the first amplification, we used a primer specific to the 3′ LTR (5′-GACTTGTGGTCTCGCTGTTCCTTGG-3′) and a primer specific to the linker (5′-GTAATACGACTCACTATAGGGC-3′) of MLV in a 25-cycle reaction, which was designed to amplify only DNA fragments that contained the 3′ LTR.
Cycle conditions:
95°C, 2 min
7 cycles of 95°C, 15 s; 72°C, 1 min
18 cycles of 95°C, 15 s; 67°C, 1 min
67°C, 4 min
4°C, ∞
The product was diluted 1:50 in dH20 and used as template for a second round of PCR. We used nested primers (nested LTR primer 5′-GAGTGATTGACTACCCGTCAGCGGGGGTCTTTCA-3′ and nested linker primer 5′-ACTATAGGGCACGCGTGGTCGACTGCGCAT-3′) to further amplify the product in a 20-cycle reaction.
Cycle conditions:
95°C, 2 min
5 cycles of 95°C, 15 s; 72°C, 1 min
15 cycles of 95°C, 15 s; 67°C, 1 min
67°C, 4 min
4°C, ∞
After the second round of PCR, we purified the sample with a MinElute PCR purification kit (Qiagen).
High-throughput sequencing
We constructed sequencing libraries for use on Illumina technologies, using adapters from the Paired-End DNA Sample Prep Kit (Illumina; San Diego, CA, USA). We ligated Illumina paired-end adapters to the products of the LM-PCR, using T4 DNA ligase for 20 min at room temperature. We purified the reaction with the MinElute PCR purification kit (Qiagen) and eluted with elution buffer (EB) buffer. We modified the adapters and further amplified the product via PCR using Phusion High-Fidelity polymerase in HF buffer, using the PE primer 1.0 and PE primer 2.0 primers from Illumina.
Cycle conditions:
98°C, 30 s
15 cycles of 98°C, 10 s; 65°C, 30 s; 72°C, 30 s
72°C, 5 min
4°C, ∞
The libraries were purified with the MinElute PCR purification kit and eluted in 20 μl of EB each. The libraries were sequenced on both Illumina MiSeq and HiSeq 2000 machines by the NIH Intramural Sequencing Center.
Integration site mapping
Our method is called GeIST (Genomic Integration Site Tracker), the outline of which is presented in Figure 1. We converted the sequencing output from both the MiSeq and the HiSeq 2000 machines from BAM format to FASTQ using BamTools version 1.0.2 (23), and concatenated the two files into a single FASTQ file. We trimmed off LTR and linker sequences from the reads using Cutadapt version 0.9.3, which was also used to trim off low-quality base calls (24); we then trimmed and recorded the barcodes using a Perl script. We discarded reads that had <11 bp of genomic DNA after removal of LTR, linker and barcode, as short reads were unlikely to map to a unique location. We created a human genome (hg19) reference file by concatenating FASTA files downloaded from ftp://hgdownload.cse.ucsc.edu/goldenPath/hg19; only the files for chr1–22, chrX and chrM were included for the K562 sample, and chrY was added for the HepG2 sample. We used Bowtie version 0.12.7 to align the trimmed reads to this reference sequence (25). We used the settings ‘-a -m 1 –best –strata’ for the alignment, indicating that the only alignments kept would be those with a uniquely low number of mismatches in the first 28 bp. No more than two mismatches were allowed within the first 28 bp of each read. The above steps were repeated for the paired reads of all aligned reads, with the only difference being that low-quality bases were not automatically removed by Cutadapt. We then compared each initially mapped read with the corresponding paired read; we retained only mapped reads that were within 1 kb of, and in the correct orientation relative to, the paired read. This allowed us to recover reads with accurate sequence, but low reported base-call quality. We removed reads from fragments in which the barcodes recorded for each read were different from each other. Next, we identified the integration site. This site is the 4 bp directly adjacent to the 3′ LTR; for simplicity, the remaining steps dealt directly with the leftmost base of the integration site. We temporarily reduced each fragment to a single entry, to avoid giving preference to short fragments in which the LTR was present in both reads. We then used the barcodes detected in previous steps to split the reads into four groups (for K562 cells) or two groups (for HepG2 cells), according to the flask that had originally produced the fragment. We gave preference to integration sites with higher fragment counts: when two integration sites from the same group were within 5 bp upstream or downstream of each other, we assumed that the site with fewer reads was composed of mismapped reads from the site with more reads because of mismatches or trimming. Therefore, we removed such integration sites, unless there was another group in which the site to be removed had a higher fragment count than other sites within 5 bp. We pooled the remaining sites, returned the read counts to their actual values and determined the total integration count by counting the barcodes at a given site, taking into account the incubation and doubling time of the original cells. As the cells only had time for one doubling after infection (24 h), a given integration event could be represented by two barcodes, at most. Therefore, we calculated the number of integrations in a given orientation at a given site to be the number of different barcodes detected, divided by two and rounded up. For example, four unique barcodes from a single grouping were taken as evidence of two integrations, while five unique barcodes indicated three integrations. Finally, we combined the counts from the barcode groups to produce the total integration count.
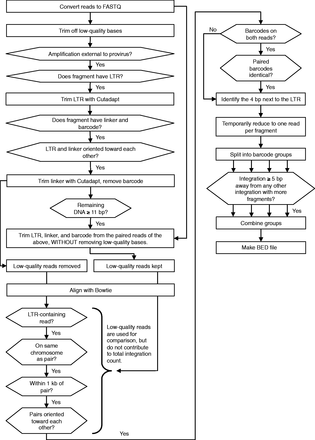
GeIST integration site mapping workflow. The GeIST workflow is available to download from http://research.nhgri.nih.gov/software/GeIST/. The details of each step are covered in the ‘Integration Site Mapping’ portion of the ‘Materials and Methods’ section. When the effect of a ‘no’ response is not explicitly stated, it is implied that reads failing to meet the criteria are removed from the analysis.
GeIST is available to download from http://research.nhgri.nih.gov/software/GeIST/ . This software is designed specifically for one of the experiments described in this article (i.e. MLV detection in K562 cells using barcoded linkers) and can be used to reproduce our mapping results with the appropriate SRA files [accession number SRS392021]. Slight modifications were made to run GeIST with the HepG2 data, such that the script treated the integrations as being from two groups instead of four. The general workflow of GeIST is applicable to mapping various types of integrated elements; as such, modifications to the code, such as the sequence trimmed by Cutadapt, can be made to apply the software to other elements. GeIST and the scripts for generating in silico matched random controls, as well as BED files of the integrations, are available at http://research.nhgri.nih.gov/software/GeIST/.
Generation of matched in silico random controls
Our approach is adapted from the approach described by Hematti et al. (12). The scripts are available to download from http://research.nhgri.nih.gov/software/GeIST/, along with files to generate additional matched controls to the K562 integrations. We used Bowtie to identify the location of all MseI, BfaI and Csp6I restriction enzyme sites. We then calculated the distance from each integration to the nearest of the three restriction sites that could have produced an alignable fragment, defined here as a sequence that could be aligned by Bowtie using the same settings with which the sequencing reads were initially aligned. If the distance was too long to fit within a single simulated read, we used a Perl script to split it into two paired-end reads with appropriate orientation. We required that both reads aligned.
We used these distances to generate 10 000 files each for both cell types; each file contained one matched random in silico integration of the same distance and same restriction site as each experimental integration. For each experimental integration, we randomly selected an instance of the given restriction site using the Perl ‘rand’ function. The in silico integration was defined as being the same distance away from the random restriction site as the actual integration was from the actual restriction site. The orientation of the random in silico site was also selected with the ‘rand’ function; it is unrelated to the orientation of the actual site on which the random site location is based. We converted all the random reads from BED to FASTA format using BEDTools version 2.16.2 (26). Random reads too long to fit on a single read length were split into paired ends, as above. We aligned the reads with Bowtie using the same settings as in the experimental workflow, with the addition of the ‘-f’ option to indicate that the random sequences were in FASTA format instead of FASTQ. Random sites that aligned were added to an output file, and the experimental sites that did not yet have an associated alignable random site were subjected to another loop through the above steps until all sites were accounted for. We repeated this process 10 000 times. In this way, the random sites account for two potential sources of bias: distance from restriction sites and alignability of the read.
Enrichment analysis
We compared the experimental and random in silico integrations with the chromatin state segmentation track, using ‘BEDTools intersect’ to detect overlap of at least one base of the 4-bp integration site with a given state (21,26). We calculated the enrichment by dividing the number of actual integrations in a state by the number of random in silico control integrations in that state. We repeated this calculation for each of the 10 000 random control data sets; the mean value of this measure is reported as the enrichment beyond random. The files used for enrichment analysis are displayed in Table 1. The state segmentation files used for the analysis were downloaded from http://genome.ucsc.edu/cgi-bin/hgTrackUi?g=wgEncodeBroadHmm (27). A similar analysis was used to calculate the enrichment within 5 kb of transcription start sites (TSS). Sites were calculated using RefSeq transcripts (release 56) downloaded from the University of California, Santa Cruz (UCSC) table browser (28). The peak files used for DNase sensitivity analysis were downloaded from the UCSC track ‘Open Chromatin by DNaseI HS from ENCODE/OpenChrom’. The file used for DNA–DNA interacting regions in K562 cells was created by using ‘BEDTools merge’ to identify regions shared by the files wgEncodeGisChiaPetK562Pol2InteractionsRep1.bed and wgEncodeGisChiaPetK562Pol2InteractionsRep2.bed, both from the UCSC track ‘Chromatin Interaction Analysis Paired-End Tags (ChIA-PET)’.
File/data set . | GEO ID . | Analysis . |
---|---|---|
Analysis files | ||
wgEncodeBroadHmmK562HMM.bed | GSM936088 | State segmentation |
wgEncodeBroadHmmHepg2HMM.bed | GSM936090 | State segmentation |
wgEncodeOpenChromDnaseHepg2Pk.narrowPeak | GSM816662 | DNAse sensitivity (HepG2) |
wgEncodeOpenChromDnaseK562PkV2.narrowPeak | GSM816655 | DNAse sensitivity (K562) |
wgEncodeGisChiaPetK562Pol2InteractionsRep1.bed | GSM970213 | DNA–DNA interaction |
wgEncodeGisChiaPetK562Pol2InteractionsRep2.bed | GSM970213 | DNA–DNA interaction |
wgEncodeBroadHistoneHepg2CtcfStdPk.broadPeak | GSM733645 | Chromatin mark enrichment (HepG2) |
wgEncodeBroadHistoneHepg2H3k04me1StdPk.broadPeak | GSM798321 | Chromatin mark enrichment (HepG2) |
wgEncodeBroadHistoneHepg2H3k4me2StdPk.broadPeak | GSM733693 | Chromatin mark enrichment (HepG2) |
wgEncodeBroadHistoneHepg2H3k4me3StdPk.broadPeak | GSM733737 | Chromatin mark enrichment (HepG2) |
wgEncodeBroadHistoneHepg2H3k9acStdPk.broadPeak | GSM733638 | Chromatin mark enrichment (HepG2) |
wgEncodeBroadHistoneHepg2H3k27acStdPk.broadPeak | GSM733743 | Chromatin mark enrichment (HepG2) |
wgEncodeBroadHistoneHepg2H3k27me3StdPk.broadPeak | GSM733754 | Chromatin mark enrichment (HepG2) |
wgEncodeBroadHistoneHepg2H3k36me3StdPk.broadPeak | GSM733685 | Chromatin mark enrichment (HepG2) |
wgEncodeBroadHistoneHepg2H4k20me1StdPk.broadPeak | GSM733694 | Chromatin mark enrichment (HepG2) |
wgEncodeBroadHistoneK562CtcfStdPk.broadPeak | GSM733719 | Chromatin mark enrichment (K562) |
wgEncodeBroadHistoneK562H3k4me1StdPk.broadPeak | GSM733692 | Chromatin mark enrichment (K562) |
wgEncodeBroadHistoneK562H3k4me2StdPk.broadPeak | GSM733651 | Chromatin mark enrichment (K562) |
wgEncodeBroadHistoneK562H3k4me3StdPk.broadPeak | GSM733680 | Chromatin mark enrichment (K562) |
wgEncodeBroadHistoneK562H3k9acStdPk.broadPeak | GSM733778 | Chromatin mark enrichment (K562) |
wgEncodeBroadHistoneK562H3k27acStdPk.broadPeak | GSM733656 | Chromatin mark enrichment (K562) |
wgEncodeBroadHistoneK562H3k27me3StdPk.broadPeak | GSM733658 | Chromatin mark enrichment (K562) |
wgEncodeBroadHistoneK562H3k36me3StdPk.broadPeak | GSM733714 | Chromatin mark enrichment (K562) |
wgEncodeBroadHistoneK562H4k20me1StdPk.broadPeak | GSM733675 | Chromatin mark enrichment (K562) |
File/data set . | GEO ID . | Analysis . |
---|---|---|
Analysis files | ||
wgEncodeBroadHmmK562HMM.bed | GSM936088 | State segmentation |
wgEncodeBroadHmmHepg2HMM.bed | GSM936090 | State segmentation |
wgEncodeOpenChromDnaseHepg2Pk.narrowPeak | GSM816662 | DNAse sensitivity (HepG2) |
wgEncodeOpenChromDnaseK562PkV2.narrowPeak | GSM816655 | DNAse sensitivity (K562) |
wgEncodeGisChiaPetK562Pol2InteractionsRep1.bed | GSM970213 | DNA–DNA interaction |
wgEncodeGisChiaPetK562Pol2InteractionsRep2.bed | GSM970213 | DNA–DNA interaction |
wgEncodeBroadHistoneHepg2CtcfStdPk.broadPeak | GSM733645 | Chromatin mark enrichment (HepG2) |
wgEncodeBroadHistoneHepg2H3k04me1StdPk.broadPeak | GSM798321 | Chromatin mark enrichment (HepG2) |
wgEncodeBroadHistoneHepg2H3k4me2StdPk.broadPeak | GSM733693 | Chromatin mark enrichment (HepG2) |
wgEncodeBroadHistoneHepg2H3k4me3StdPk.broadPeak | GSM733737 | Chromatin mark enrichment (HepG2) |
wgEncodeBroadHistoneHepg2H3k9acStdPk.broadPeak | GSM733638 | Chromatin mark enrichment (HepG2) |
wgEncodeBroadHistoneHepg2H3k27acStdPk.broadPeak | GSM733743 | Chromatin mark enrichment (HepG2) |
wgEncodeBroadHistoneHepg2H3k27me3StdPk.broadPeak | GSM733754 | Chromatin mark enrichment (HepG2) |
wgEncodeBroadHistoneHepg2H3k36me3StdPk.broadPeak | GSM733685 | Chromatin mark enrichment (HepG2) |
wgEncodeBroadHistoneHepg2H4k20me1StdPk.broadPeak | GSM733694 | Chromatin mark enrichment (HepG2) |
wgEncodeBroadHistoneK562CtcfStdPk.broadPeak | GSM733719 | Chromatin mark enrichment (K562) |
wgEncodeBroadHistoneK562H3k4me1StdPk.broadPeak | GSM733692 | Chromatin mark enrichment (K562) |
wgEncodeBroadHistoneK562H3k4me2StdPk.broadPeak | GSM733651 | Chromatin mark enrichment (K562) |
wgEncodeBroadHistoneK562H3k4me3StdPk.broadPeak | GSM733680 | Chromatin mark enrichment (K562) |
wgEncodeBroadHistoneK562H3k9acStdPk.broadPeak | GSM733778 | Chromatin mark enrichment (K562) |
wgEncodeBroadHistoneK562H3k27acStdPk.broadPeak | GSM733656 | Chromatin mark enrichment (K562) |
wgEncodeBroadHistoneK562H3k27me3StdPk.broadPeak | GSM733658 | Chromatin mark enrichment (K562) |
wgEncodeBroadHistoneK562H3k36me3StdPk.broadPeak | GSM733714 | Chromatin mark enrichment (K562) |
wgEncodeBroadHistoneK562H4k20me1StdPk.broadPeak | GSM733675 | Chromatin mark enrichment (K562) |
File/data set . | GEO ID . | Analysis . |
---|---|---|
Analysis files | ||
wgEncodeBroadHmmK562HMM.bed | GSM936088 | State segmentation |
wgEncodeBroadHmmHepg2HMM.bed | GSM936090 | State segmentation |
wgEncodeOpenChromDnaseHepg2Pk.narrowPeak | GSM816662 | DNAse sensitivity (HepG2) |
wgEncodeOpenChromDnaseK562PkV2.narrowPeak | GSM816655 | DNAse sensitivity (K562) |
wgEncodeGisChiaPetK562Pol2InteractionsRep1.bed | GSM970213 | DNA–DNA interaction |
wgEncodeGisChiaPetK562Pol2InteractionsRep2.bed | GSM970213 | DNA–DNA interaction |
wgEncodeBroadHistoneHepg2CtcfStdPk.broadPeak | GSM733645 | Chromatin mark enrichment (HepG2) |
wgEncodeBroadHistoneHepg2H3k04me1StdPk.broadPeak | GSM798321 | Chromatin mark enrichment (HepG2) |
wgEncodeBroadHistoneHepg2H3k4me2StdPk.broadPeak | GSM733693 | Chromatin mark enrichment (HepG2) |
wgEncodeBroadHistoneHepg2H3k4me3StdPk.broadPeak | GSM733737 | Chromatin mark enrichment (HepG2) |
wgEncodeBroadHistoneHepg2H3k9acStdPk.broadPeak | GSM733638 | Chromatin mark enrichment (HepG2) |
wgEncodeBroadHistoneHepg2H3k27acStdPk.broadPeak | GSM733743 | Chromatin mark enrichment (HepG2) |
wgEncodeBroadHistoneHepg2H3k27me3StdPk.broadPeak | GSM733754 | Chromatin mark enrichment (HepG2) |
wgEncodeBroadHistoneHepg2H3k36me3StdPk.broadPeak | GSM733685 | Chromatin mark enrichment (HepG2) |
wgEncodeBroadHistoneHepg2H4k20me1StdPk.broadPeak | GSM733694 | Chromatin mark enrichment (HepG2) |
wgEncodeBroadHistoneK562CtcfStdPk.broadPeak | GSM733719 | Chromatin mark enrichment (K562) |
wgEncodeBroadHistoneK562H3k4me1StdPk.broadPeak | GSM733692 | Chromatin mark enrichment (K562) |
wgEncodeBroadHistoneK562H3k4me2StdPk.broadPeak | GSM733651 | Chromatin mark enrichment (K562) |
wgEncodeBroadHistoneK562H3k4me3StdPk.broadPeak | GSM733680 | Chromatin mark enrichment (K562) |
wgEncodeBroadHistoneK562H3k9acStdPk.broadPeak | GSM733778 | Chromatin mark enrichment (K562) |
wgEncodeBroadHistoneK562H3k27acStdPk.broadPeak | GSM733656 | Chromatin mark enrichment (K562) |
wgEncodeBroadHistoneK562H3k27me3StdPk.broadPeak | GSM733658 | Chromatin mark enrichment (K562) |
wgEncodeBroadHistoneK562H3k36me3StdPk.broadPeak | GSM733714 | Chromatin mark enrichment (K562) |
wgEncodeBroadHistoneK562H4k20me1StdPk.broadPeak | GSM733675 | Chromatin mark enrichment (K562) |
File/data set . | GEO ID . | Analysis . |
---|---|---|
Analysis files | ||
wgEncodeBroadHmmK562HMM.bed | GSM936088 | State segmentation |
wgEncodeBroadHmmHepg2HMM.bed | GSM936090 | State segmentation |
wgEncodeOpenChromDnaseHepg2Pk.narrowPeak | GSM816662 | DNAse sensitivity (HepG2) |
wgEncodeOpenChromDnaseK562PkV2.narrowPeak | GSM816655 | DNAse sensitivity (K562) |
wgEncodeGisChiaPetK562Pol2InteractionsRep1.bed | GSM970213 | DNA–DNA interaction |
wgEncodeGisChiaPetK562Pol2InteractionsRep2.bed | GSM970213 | DNA–DNA interaction |
wgEncodeBroadHistoneHepg2CtcfStdPk.broadPeak | GSM733645 | Chromatin mark enrichment (HepG2) |
wgEncodeBroadHistoneHepg2H3k04me1StdPk.broadPeak | GSM798321 | Chromatin mark enrichment (HepG2) |
wgEncodeBroadHistoneHepg2H3k4me2StdPk.broadPeak | GSM733693 | Chromatin mark enrichment (HepG2) |
wgEncodeBroadHistoneHepg2H3k4me3StdPk.broadPeak | GSM733737 | Chromatin mark enrichment (HepG2) |
wgEncodeBroadHistoneHepg2H3k9acStdPk.broadPeak | GSM733638 | Chromatin mark enrichment (HepG2) |
wgEncodeBroadHistoneHepg2H3k27acStdPk.broadPeak | GSM733743 | Chromatin mark enrichment (HepG2) |
wgEncodeBroadHistoneHepg2H3k27me3StdPk.broadPeak | GSM733754 | Chromatin mark enrichment (HepG2) |
wgEncodeBroadHistoneHepg2H3k36me3StdPk.broadPeak | GSM733685 | Chromatin mark enrichment (HepG2) |
wgEncodeBroadHistoneHepg2H4k20me1StdPk.broadPeak | GSM733694 | Chromatin mark enrichment (HepG2) |
wgEncodeBroadHistoneK562CtcfStdPk.broadPeak | GSM733719 | Chromatin mark enrichment (K562) |
wgEncodeBroadHistoneK562H3k4me1StdPk.broadPeak | GSM733692 | Chromatin mark enrichment (K562) |
wgEncodeBroadHistoneK562H3k4me2StdPk.broadPeak | GSM733651 | Chromatin mark enrichment (K562) |
wgEncodeBroadHistoneK562H3k4me3StdPk.broadPeak | GSM733680 | Chromatin mark enrichment (K562) |
wgEncodeBroadHistoneK562H3k9acStdPk.broadPeak | GSM733778 | Chromatin mark enrichment (K562) |
wgEncodeBroadHistoneK562H3k27acStdPk.broadPeak | GSM733656 | Chromatin mark enrichment (K562) |
wgEncodeBroadHistoneK562H3k27me3StdPk.broadPeak | GSM733658 | Chromatin mark enrichment (K562) |
wgEncodeBroadHistoneK562H3k36me3StdPk.broadPeak | GSM733714 | Chromatin mark enrichment (K562) |
wgEncodeBroadHistoneK562H4k20me1StdPk.broadPeak | GSM733675 | Chromatin mark enrichment (K562) |
We also used BEDTools to perform comparisons of the integrations with the component factors of the state segmentation track and used peak files from the ‘Histone Modifications by ChIP-seq from ENCODE/Broad Institute’ track on the UCSC browser (29). All histone modification files were downloaded from the UCSC genome browser at http://genome.ucsc.edu/cgi-bin/hgTrackUi?g=wgEncodeBroadHistone (27). We used ‘BEDTools intersect’ to test enrichment for regions in which more than one type of chromatin mark peak was present.
Sequence motif analysis
We analyzed the sequence of integration sites by using BEDTools to generate the FASTA sequence of both the experimental integrations and the random in silico controls. We examined both the 4- and 5-bp integration sites as well as the sequences flanking the site in either direction, taking into account the orientation of the integration. Two of the 10 000 random data sets for K562 encountered problems with reads assigned to chrM because taking the 5-bp upstream of the site resulted in negative position values; we resolved this by manually correcting the sequence to take the circular nature of chrM into account. Some of the random data sets for HepG2 encountered a similar problem on linear chromosomes; these data sets were removed from the analysis, and the Bonferroni correction was adjusted accordingly. We determined the base composition by counting the presence of each base at each position using regular expressions and arrays in the UNIX program AWK and dividing those counts by the total number of integrations. We used bootstrapping to determine the significance of the differences between experimental and random control base compositions. Although most bases had significantly different frequencies than random, most differences were not large. As such, we based our description of the motif on bases with >10% change relative to random.
Statistical analysis
We carried out most analyses by bootstrapping. We looked for the values of a given random control data set that were more extreme (with respect to the mean of the random sets) than the corresponding value in the experimental set and repeated this process n = 10 000 times. For example, if a chromatin state was found to have more integrations in the experimental set than the mean value of the random sets, we would count the number of random sets in which there were more integrations in that state than had been for the experimental set. The sum, divided by 10 000 (the number of random tests), is the P-value; Bonferroni correction was used in situations that required multiple tests. To ensure an unbiased analysis, we calculated P-values for both enrichment and depletion in every category. For example, when analyzing the 15 chromatin states, this resulted in 30 tests, so we divided the significance threshold by 30. For simplicity, we only report the relevant P-values here: the P-value for enrichment if the sample was enriched relative to the mean of the random sets, and likewise for depletion. The significance threshold is P = 0.05, unless modified by Bonferroni correction.
The differences in the enrichment values were determined by analysis of variance and Tukey’s test. These analyses were carried out in R version 2.14.2, using the aov() and TukeyHSD() methods (30).
Figures
Figure 2 is a composite of graphs made in R and modified versions of images downloaded from the UCSC genome browser (31). Figures 3A, 4 and 5, Supplementary Figures S1A, Supplementary Data and Supplementary Data were generated using the ggplot2 package in R (32).
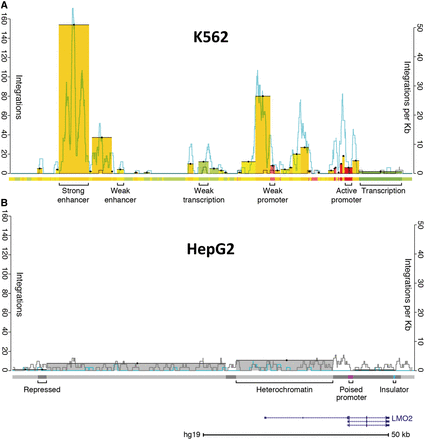
Integration pattern near LMO2 in K562 and HepG2 cells. (A) Integrations in K562 cells. Bars indicate the sum of unique integration events color-coded to match the genomic state in which they integrated (left axis); there are 471 events represented in this ∼110-kb span. Bar tops are highlighted with a black line and a dot for visibility. The right axis indicates the rate of integration in a 1-kb sliding window for both experimental integrations (blue) and a representative in silico random control (gray). The location of LMO2 is represented in the lower right of the figure. Approximately 0.15% of the 315 810 integrations scored landed in this LMO2 interval, which comprises 0.0036% of the genome. The colored track below the integrations is the chromatin state segmentation track (21); colors correspond to different states, as indicated. (B) Integrations in HepG2 cells. The scales are the same as in (A). There are 19 integrations in this region. Experimental integrations are significantly depleted relative to random control integrations in this region (bootstrapping; P < 0.0001). Note that the number of random integrations per kilobase tends to be higher for HepG2 than K562 cells. This is because there are >10-fold as many integrations in HepG2 relative to K562, resulting in a corresponding increase in the number of random control integrations. This figure clearly shows the cell-type-specific differences in the raw number of experimental integrations in the LMO2 region between K562 and HepG2 cells. These differences can likely be attributed to the presence of active LMO2 enhancers and promoters in K562 cells and their absence in HepG2 cells.
![Enrichment of integrations in chromatin segmentation states in HepG2 cells. (A) The mean value of 10 000 ratios of experimental integration versus in silico random integration; error bars represent the standard deviation of these ratios. The dotted line separates entries with more integrations than expected by chance from those with fewer. The experimental integration counts in all states are significantly different from random, as determined by bootstrapping (significance threshold = 0.0017; all differences from random each have P < 0.0001). The enrichment values in each state are all significantly different from each other, as determined by analysis of variance (P < 2 × 10−16) and Tukey’s multiple comparisons of means (all pairs differ with adjusted P < 10−7). (B) Percentage observed frequency of chromatin marks for each of the 15 states across the genome in HepG2 cells [modified from Ernst et al. (21)]. Darker blue cells indicate a higher observed frequency than lighter cells. The states are sorted by mean enrichment versus random; the horizontal dashed line separates states with more integration sites than expected by chance from states with as many or fewer, and the chromatin marks to the right of the vertical dashed line are most associated with strong enhancers. Numerical values for this table are in Ernst et al. (21). Txn, transcription; lo, low signal; CNV, copy number variation.](https://oup.silverchair-cdn.com/oup/backfile/Content_public/Journal/nar/42/7/10.1093_nar_gkt1399/4/m_nar_42_7_4257_f3.gif?Expires=1750271070&Signature=GOdT9XFfnKFTpKQMKkwvzfSrtmvqAUoSnu6P6FhDox-h-y3DSY4Txb9ds47YSlBJkLJt5OJfjt9UrVm6sfaP4vnfcVc4lmvr79S9v6WCfC~RDSRrJLcWhly97X8zRzdWHvKmenicLTxT0M6ZD7kZ~u77DfoAlUbEX4oS5d9sYQ~bChUM9dnjtIw8mCIkiPDaDLW6U5uCNMdBBHOckjP4SJd43NEFX2AVDDKrpUxtOk7PmqytGFob7SP8z5cfQ2-fAKnu002~3p8GiW5a~MQGvoI9p4hFXTZnn308aSlRT5WYwdikh9vvHura~ywjjKbd7uI-dVY1F-fOWC5s4EDlHw__&Key-Pair-Id=APKAIE5G5CRDK6RD3PGA)
Enrichment of integrations in chromatin segmentation states in HepG2 cells. (A) The mean value of 10 000 ratios of experimental integration versus in silico random integration; error bars represent the standard deviation of these ratios. The dotted line separates entries with more integrations than expected by chance from those with fewer. The experimental integration counts in all states are significantly different from random, as determined by bootstrapping (significance threshold = 0.0017; all differences from random each have P < 0.0001). The enrichment values in each state are all significantly different from each other, as determined by analysis of variance (P < 2 × 10−16) and Tukey’s multiple comparisons of means (all pairs differ with adjusted P < 10−7). (B) Percentage observed frequency of chromatin marks for each of the 15 states across the genome in HepG2 cells [modified from Ernst et al. (21)]. Darker blue cells indicate a higher observed frequency than lighter cells. The states are sorted by mean enrichment versus random; the horizontal dashed line separates states with more integration sites than expected by chance from states with as many or fewer, and the chromatin marks to the right of the vertical dashed line are most associated with strong enhancers. Numerical values for this table are in Ernst et al. (21). Txn, transcription; lo, low signal; CNV, copy number variation.
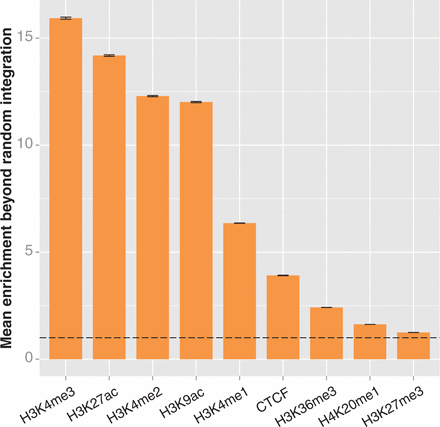
Enrichment of integrations in ChIP-seq peaks of chromatin marks in HepG2 cells. We compared experimental integrations and integrations in the 10 000 matched random control data sets to ChIP-seq peaks from the ENCODE project (29). We calculated enrichment as described in the caption of Figure 3. The error bars represent the standard deviation of the enrichment ratio. The dotted line indicates the level of enrichment expected by chance. The experimental integration counts for all marks are significantly different from random (determined as above, by bootstrapping; significance threshold = 0.0028; all differences from random have P < 0.0001). The enrichment values for each mark are all significantly different from each other, as determined by analysis of variance (P < 2 × 10−16) and Tukey’s multiple comparisons of means (all pairs differ with adjusted P < 10−7).
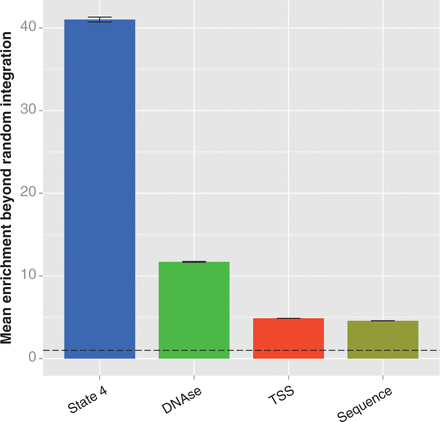
Comparison of integration enrichment across various genomic features in HepG2 cells. We compared the enrichment at features that are associated with MLV integration to measure their ability to explain non-random integration. We calculated enrichment as described in the caption of Figure 3. The error bars represent the standard deviation of the enrichment ratio, and the dotted line indicates the level of enrichment expected by chance. The enrichment values for each feature are all significantly different from random (determined as above, by bootstrapping; significance threshold = 0.00625; all differences from random have P < 0.0001), and different from each other, as determined by analysis of variance (P < 2 × 10−16) and Tukey’s multiple comparisons of means (all pairs differ with adjusted P < 10−7). Labels: state 4, the state 4 strong enhancers; DNAse, DNAse-sensitive regions; TSS, regions within 5 kb of a transcription start site; sequence, sites matching the TNVNNBNA motif.
RESULTS
We took advantage of high-throughput sequencing and DNA barcoding to generate >100 times as many MLV integrations as any previous study, and developed an analysis pipeline to map and quantify the integrations. We first infected two flasks of human HepG2 cells and four flasks of human K562 cells with MLV and recovered the proviral integration sites in a high-throughput manner (5) (see details in ‘Materials and Methods’ section). We harvested DNA from cells, subjected it to restriction digests and used ligation-mediated PCR to amplify fragments that contained the 3′-end of the integrated provirus. We used linkers containing 6-bp barcode sequences, allowing us to pool the DNA into a single sequencing sample per cell type and detect independent integration events even if they occurred at identical genomic coordinates. We sequenced both pooled integration libraries on Illumina MiSeq and HiSeq 2000 sequencers, and then used a new software package we created (the Genomic Integration Site Tracker, or GeIST) to analyze the sequencing data (Figure 1). In HepG2 cells, we obtained 16 954 554 mappable sequences representing 3 382 718 independent integration events in 2 620 203 unique integration sites; K562 cells yielded 6 397 337 sequences representing 315 810 integrations in 230 960 unique sites. The 6-bp barcode sequences enabled us to ascertain that 19.4% of HepG2 integration sites (defined as a single genomic location) were represented by multiple independent integrations, and 32% of K562 sites met this criterion. The most extreme example was observed in HepG2 cells at position chr2:191540751-191540755 (hg19), where we recorded 248 independent integration events. Genome integration hotspots (within a 20-kb window) are shown in Table 2 and Supplementary Table S1. To determine whether the MLV integration pattern differs from what would be expected by chance, we generated two sets of 10 000 control integration data sets in silico, each containing a number of matched random control integrations equal to those in the corresponding cell type. In silico controls were designed to control for bias introduced by both restriction enzyme fragmentation and the ability to align the sequenced fragment. We also used the in silico controls to compare the GC content of HepG2 experimental reads with the percentage expected by chance. We found that the GC content of the pre-adapter LM-PCR fragments was higher than that of the control fragments (51.9 versus 48.2%; bootstrapping, P < 0.0001). The difference is significant, but slight, and we consider it unlikely that PCR-based skewing had a substantial effect on our ability to recover integration sites.
A 20-kb window was used to identify the regions with the most integrations; the top 10 regions are shown
Chromosome . | Start (base pairs) . | End (base pairs) . | Integrations . | Mean random integrations ± SD . | Nearby genes . |
---|---|---|---|---|---|
HepG2 integration hotspots | |||||
chr14 | 31720463 | 31740463 | 2438 | 19.60 ± 4.41 | Downstream of HEATR5A |
chr14 | 31492094 | 31512094 | 2123 | 26.75 ± 5.23 | STRN3 and SP4S1 |
chr20 | 48290780 | 48310780 | 1841 | 26.18 ± 5.05 | B4GALT5 |
chr20 | 46079011 | 46099011 | 1834 | 16.65 ± 4.07 | Upstream of NCOA3 |
chr19 | 47597773 | 47617773 | 1825 | 19.44 ± 4.43 | ZC3H4 |
chr20 | 371219 | 391219 | 1731 | 17.46 ± 4.21 | Downstream of TIRB3, upstream of RBCK1 |
chr20 | 32916489 | 32936489 | 1634 | 15.94 ± 3.99 | Upstream of AHCY and ITCH |
chr12 | 50915923 | 50935923 | 1623 | 21.62 ± 4.69 | DIP2B |
chr16 | 15721516 | 15741516 | 1616 | 26.97 ± 5.13 | KIAA0430 and NDE1 |
chr12 | 69178983 | 69198983 | 1598 | 22.15 ± 4.72 | Upstream of LOC100130075 and MDM2 |
Chromosome . | Start (base pairs) . | End (base pairs) . | Integrations . | Mean random integrations ± SD . | Nearby genes . |
---|---|---|---|---|---|
HepG2 integration hotspots | |||||
chr14 | 31720463 | 31740463 | 2438 | 19.60 ± 4.41 | Downstream of HEATR5A |
chr14 | 31492094 | 31512094 | 2123 | 26.75 ± 5.23 | STRN3 and SP4S1 |
chr20 | 48290780 | 48310780 | 1841 | 26.18 ± 5.05 | B4GALT5 |
chr20 | 46079011 | 46099011 | 1834 | 16.65 ± 4.07 | Upstream of NCOA3 |
chr19 | 47597773 | 47617773 | 1825 | 19.44 ± 4.43 | ZC3H4 |
chr20 | 371219 | 391219 | 1731 | 17.46 ± 4.21 | Downstream of TIRB3, upstream of RBCK1 |
chr20 | 32916489 | 32936489 | 1634 | 15.94 ± 3.99 | Upstream of AHCY and ITCH |
chr12 | 50915923 | 50935923 | 1623 | 21.62 ± 4.69 | DIP2B |
chr16 | 15721516 | 15741516 | 1616 | 26.97 ± 5.13 | KIAA0430 and NDE1 |
chr12 | 69178983 | 69198983 | 1598 | 22.15 ± 4.72 | Upstream of LOC100130075 and MDM2 |
The start and end points are presented as they would be in a BED file (0-based start). Mean random integrations ± SD indicates the mean value and standard deviation of the integration count over 10 000 matched random control data sets, and represents the number of integrations expected by chance. Genes with at least part of one RefSeq transcript within the 20-kb window are displayed in the final column; nearby downstream genes that are outside the window are also indicated.
A 20-kb window was used to identify the regions with the most integrations; the top 10 regions are shown
Chromosome . | Start (base pairs) . | End (base pairs) . | Integrations . | Mean random integrations ± SD . | Nearby genes . |
---|---|---|---|---|---|
HepG2 integration hotspots | |||||
chr14 | 31720463 | 31740463 | 2438 | 19.60 ± 4.41 | Downstream of HEATR5A |
chr14 | 31492094 | 31512094 | 2123 | 26.75 ± 5.23 | STRN3 and SP4S1 |
chr20 | 48290780 | 48310780 | 1841 | 26.18 ± 5.05 | B4GALT5 |
chr20 | 46079011 | 46099011 | 1834 | 16.65 ± 4.07 | Upstream of NCOA3 |
chr19 | 47597773 | 47617773 | 1825 | 19.44 ± 4.43 | ZC3H4 |
chr20 | 371219 | 391219 | 1731 | 17.46 ± 4.21 | Downstream of TIRB3, upstream of RBCK1 |
chr20 | 32916489 | 32936489 | 1634 | 15.94 ± 3.99 | Upstream of AHCY and ITCH |
chr12 | 50915923 | 50935923 | 1623 | 21.62 ± 4.69 | DIP2B |
chr16 | 15721516 | 15741516 | 1616 | 26.97 ± 5.13 | KIAA0430 and NDE1 |
chr12 | 69178983 | 69198983 | 1598 | 22.15 ± 4.72 | Upstream of LOC100130075 and MDM2 |
Chromosome . | Start (base pairs) . | End (base pairs) . | Integrations . | Mean random integrations ± SD . | Nearby genes . |
---|---|---|---|---|---|
HepG2 integration hotspots | |||||
chr14 | 31720463 | 31740463 | 2438 | 19.60 ± 4.41 | Downstream of HEATR5A |
chr14 | 31492094 | 31512094 | 2123 | 26.75 ± 5.23 | STRN3 and SP4S1 |
chr20 | 48290780 | 48310780 | 1841 | 26.18 ± 5.05 | B4GALT5 |
chr20 | 46079011 | 46099011 | 1834 | 16.65 ± 4.07 | Upstream of NCOA3 |
chr19 | 47597773 | 47617773 | 1825 | 19.44 ± 4.43 | ZC3H4 |
chr20 | 371219 | 391219 | 1731 | 17.46 ± 4.21 | Downstream of TIRB3, upstream of RBCK1 |
chr20 | 32916489 | 32936489 | 1634 | 15.94 ± 3.99 | Upstream of AHCY and ITCH |
chr12 | 50915923 | 50935923 | 1623 | 21.62 ± 4.69 | DIP2B |
chr16 | 15721516 | 15741516 | 1616 | 26.97 ± 5.13 | KIAA0430 and NDE1 |
chr12 | 69178983 | 69198983 | 1598 | 22.15 ± 4.72 | Upstream of LOC100130075 and MDM2 |
The start and end points are presented as they would be in a BED file (0-based start). Mean random integrations ± SD indicates the mean value and standard deviation of the integration count over 10 000 matched random control data sets, and represents the number of integrations expected by chance. Genes with at least part of one RefSeq transcript within the 20-kb window are displayed in the final column; nearby downstream genes that are outside the window are also indicated.
We found strong clustering of integrations in a small total percentage of the genome demonstrating clear influences on integration site selection. Figure 2 shows the MLV integration pattern around the LMO2 gene; integrations in this region caused leukemia in some recipients of gene therapy (33,34). The K562 integration profile in this region serves as a representative example of the overall integration pattern we observed genome-wide and is consistent with previous observations (10): in both cell lines, integrations were typically enriched near TSS and showed non-random intergenic patterns. However, this region near LMO2 reveals a cell-type-specific difference in integration that cannot be explained by proximity to TSS or underlying primary sequence. To more precisely define the driver of integration site selection, we compared integration sites with the ENCODE chromatin state segmentation annotations produced for these cell lines (21,27). Ernst et al. used a hidden Markov model (HMM) to divide the human genome into different ‘states’, based on the observed chromatin immunoprecipitation sequencing (ChIP-seq) frequency of nine chromatin factors. Comparing our integrations with this model allowed us to detect associations with groups of chromatin marks (rather than individual marks) in a biologically meaningful context.
We detected a striking association, finding that MLV specifically integrated into strong enhancers and promoters in both cell lines (Figure 3 and Supplementary Figure S1). To quantify this association, we used bootstrapping of integration counts in experimental versus in silico random data sets. The depth of the integration data allowed us to detect that enrichment of integrations was significantly different from random for all states (P < 0.0001 each, significance threshold = 0.0017; Figure 3A and Supplementary Figure S1A). Notably, all seven enhancer and promoter states showed a significant increase of integrations relative to in silico control integrations in both cell lines (Figure 3A and Supplementary Figure S1A). These associations are sufficient to explain the cell-type differences near LMO2. The states in and near LMO2 with the most integrations were defined as promoters and enhancers by ENCODE in the blood-derived K562 cells (Figure 2). In contrast, the region near LMO2 in liver-derived HepG2 cells was annotated as repressive and heterochromatin. Despite containing identical sequence, this region had few integrations in HepG2 cells (Figure 2B). This observation is consistent with the inference that LMO2 was a preferred site of integration in the bone marrow-derived hematopoetic stem cells used in the gene therapy trials because of highly active elements in the region (hematopoetic stem cell gene expression is more likely to be related to K562 gene expression than HepG2).
Although active regulatory regions were generally enriched for integrations, some regions were substantially better targets than others. The most extreme enrichment of integrations was in one of two states annotated as strong enhancer (chromatin state 4). These regions were >41 times more likely to contain integrations than would be expected by chance in HepG2 cells, respectively, and similar levels of enrichment were detected in K562 cells (Figure 3A and Supplementary Figure S1A). Both cell types also exhibited substantial enrichment in state 1, annotated as active promoters. These two states are characterized by high enrichment of four to five chromatin marks, all of which are associated with enhancers and/or promoters (21) (Figure 3B and Supplementary Figure S1B). In addition to having the greatest enrichment, state 4 contains the largest number of integrations among the 15 states. State 4 regions contained 22.4% of HepG2 integrations and 32.9% of K562 integrations, despite accounting for only 0.64 and 0.90%, respectively, of the 2.83 Gb covered by the state segmentation track.
However, not all enhancers and promoters are created equal; it appears that high enrichment of specific chromatin marks is a better indicator of integration preference than annotation as an enhancer or promoter per se. For example, although states 4 and 5 are both annotated as strong enhancers (21), state 5 regions are less than half as likely to be selected for integration as state 4 (HepG2: 21.8× versus 41.0×; K562: 16.7× versus 39.4×; analysis of variance with Tukey’s test, P < 10−7 for both comparisons; Figure 3 and Supplementary Figure S1). This is a general trend: the states that have a greater number and frequency of the five factors that define state 4 had a greater enrichment of MLV integrations (Figure 3B and Supplementary Figure S1B). Turning the analysis around, the MLV preference for state 4 strong enhancers over state 5 strong enhancers proves that the ChromHMM predictions are identifying real biologically meaningful differences between the two categories of strong enhancers.
We repeated the integration enrichment analysis with each of the nine chromatin marks individually (Figure 4 and Supplementary Figure S2). We found that the highest enrichment observed was in spans of H3K4me3 in HepG2 cells and H3K4me2 in K562 cells, respectively, with 15.9× and 16.2× enrichment beyond the value that would have been expected if integrations were randomly distributed. Although this is a substantial enrichment, it is well below the ∼40× enrichment detected in state 4 enhancers; overall, the HMM in both cell types produced three chromatin states with higher enrichment than the highest of the individual marks. Thus, the integrated information from multiple marks in the HMM states are better indicators of integration site selection than their individual components. This suggests that chromatin conformation is influencing MLV site selection, although it does not exclude integrase interaction with a protein ‘tether’ that preferentially binds to areas containing the highest content of the five chromatin marks.
The integrated data from the ChromHMM track are a superior predictor to simply combining preferences from individual chromatin marks. Santoni et al. previously reported an association between MLV integration and multiple chromatin marks similar to our findings (35). They found that regions that contained H3K4me1, H3K4me3 and H3K9ac were good predictors of MLV integration in HeLa cells, and that adding in a requirement for H3K4me2 formed a good predictor in CD4+ T cells. We tested the enrichment of MLV in regions that contained the three HeLa marks on the ENCODE ChIP-seq tracks. We found MLV to be significantly enriched in both HepG2 and K562 cells (20.9× and 20.0×, respectively; P < 0.0001 each, significance threshold = 0.00625). Enrichment was further bolstered by adding in requirements for both H3K4me2 and H3K27ac, the other two marks strongly associated with state 4 in the HMM. Doing so increased enrichment to 25.7× in HepG2 cells and 27.5× in K562 cells (significantly enriched versus random with P < 0.0001). The fact that this enrichment is considerably greater than expected by chance, but remains substantially lower than enrichment in state 4 alone, indicates that the HMM is better able to identify the most relevant subset of integration targets than simply taking the intersect of ChIP-seq peaks. This suggests that the observed frequency of the marks, not just their presence, is important for determining MLV integration targets.
Taken together, it appears that the most frequent targets for MLV integration are largely determined by the presence and observed frequency of five different marks of enhancers or promoters—H3K4me1, H3K4me2, H3K4me3, H3K27ac and H3K9ac. These criteria are a substantially better predictor of MLV integration than previously proposed characteristics. The enrichment over random integration within 5 kb of TSS, previously proposed to be a major driver of MLV integration patterns, is only 4.9× in HepG2 and 6.6× in K562 cells (Figure 5 and Supplementary Figure S3). While the enrichment near TSS is significantly greater than random (bootstrapping; P < 0.0001 for both comparisons), it is more than eight times lower than the enrichment in strong enhancers in HepG2 cells; it is likely that this enrichment near TSS is caused by the presence of the highly preferred strong proximal regulatory regions. DNAse-sensitive regions have also been proposed to be the chief feature behind MLV integration (17,18); although our results confirm such regions have significantly higher enrichment than random, the magnitude of enrichment is only 11.7× in HepG2 and 14.5× in K562, three and a half times less than we see for strong enhancers. This strongly suggests that traditional concepts such as MLV preferring ‘open chromatin’ are inaccurate, and there are much more specific influences on integration site selection. Other genomic characteristics, such as regions that interact through a Pol2 intermediary, have similar results (5.2× enrichment in K562 cells; bootstrapping, different than random with P < 0.0001; Supplementary Figure S3) (36,37). While it appears that MLV integration can be somewhat promiscuous in terms of chromatin features, one-third to half of all integration sites can be predicted purely by using two of the ChromHMM-predicted chromatin states: state 4 strong enhancers and state 1 active promoters. Together, these two states comprise <2% of the genome.
We considered the role of primary sequence in influencing integration and found that it had a statistically significant, but relatively minor, role. Previous studies have reported that primary sequence plays a role in determining MLV integration sites (13,38). Wu et al. reported that MLV integrated in a weak palindromic motif, abbreviated TNVTABNA. We performed a comparable analysis on our much deeper integration data and found similar motifs in both cell types: TNVNNBNA in HepG2 and TNVTNBNA in K562 (Figure 6 and Supplementary Figure S4). The motif occurred in 18.6 and 6.64% of integrations in the experimental data sets, respectively, significantly more often than in random controls (bootstrapping; P < 0.0001 for both comparisons). However, the increase was of relatively small effect, with a mean of 4.57× and 5.27× enrichment, respectively (Figure 5 and Supplementary Figure S3). We interpret this to indicate that DNA sequence influences the final location of MLV integration to a small degree, but most likely after the major region of integration has already been determined. Our data suggest a model in which the large-scale integration preference of MLV is associated with marks of strong enhancers and active promoters; within those regions, primary sequence may influence the fine-scale integration location.
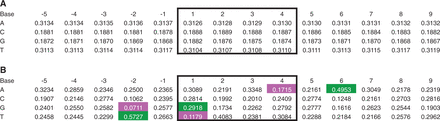
Bias of primary sequence at MLV integration sites in HepG2 cells. We ascertained the sequence of experimental and random in silico inserts with ‘BEDTools getfasta’, taking strand orientation into account (26). (A) Base composition of random control integrations. Integration occurs in positions 1–4 (black box); positions are relative to the 5′ base of the integration site. The values are the mean proportion of the ratio of the base in question at that position >9 920 random control data sets (80 random controls contained a site in which the 5 bp flanking sequence extended off the end of the chromosome; these controls were removed). (B) Base composition of experimental integrations. All proportions are significantly different from random (bootstrapping; significance threshold = 0.0004; P < 0.0001 for each). The values that differ from the mean random value by ≥10% are highlighted (green, 10% more than random; magenta, 10% less; gray, not significantly different from random).
DISCUSSION
Our collection of almost 3.7 million independent MLV integrations, coupled with the ENCODE chromatin HMM, has provided new insight into the drivers of MLV integration. The data presented show that MLV has a strong integration bias toward strong enhancers of a specific type and active promoters. Essentially 50% of all integrations occur in ≈1.6–2.0% of the genome, and both cell types examined showed ∼40× enrichment to a subset of regions annotated as strong promoters. The effect we see when examining marks of active chromatin in aggregate is substantially stronger than examining them individually, and produces a higher enrichment than previously reported determinants of MLV integration, such as DNAse sensitivity, TSSs or primary sequence. Although both cell lines are karyotypically abnormal (39,40), the abnormalities are on a scale that is not relevant to the analysis and comparisons performed here. The ChromHMM tracks from ENCODE are based on 200-bp bins and identify location-specific features. Copy number variations might increase the total number of integrations in a particular location, but they would not change the preferences for enhancers or promoters we describe.
Our findings have important implications for both genome evolution and gene therapy. The marked preference for strong enhancers and active promoters suggests these regions are beneficial for MLV survival and propagation. This pattern may give the provirus a higher probability of access to transcriptional machinery than would a random integration pattern. We have previously shown that the LTR10 and MER61 classes of endogenous retroviral retroelements caused the emergence of new tp53 binding sites (4). Although retroviruses like MLV tend to be selected against if they are near genes (41), those with a propensity for integrating into active enhancers and promoters could alter gene expression by disrupting or adding transcription factor binding sites. By having a propensity for integrating into areas specifically dedicated to gene regulation, there would be a substantial enrichment in the odds of adding new DNA binding sites with new functionality. Transmitted through the germline, these changes become drivers of evolutionary change. In addition, because of the particular preference of MLV integrations for strong enhancers and promoters, high-density mapping of MLV integration sites might prove to be an efficient technique for rapidly identifying regulatory elements throughout the genome in cells or organisms where ENCODE data are not available. As multiple types of active chromatin marks are preferred by MLV, it may be possible to determine the relative activities of each element based on the frequency of integrations per kilobase.
At the same time, this integration pattern can be detrimental to the host, although our mapping approach provides a way to reduce the risk to gene therapy patients. In the mouse, MLV integrations cause tumors at a high frequency, probably because the viruses are most likely to integrate in an active enhancer or promoter, and the LTRs contain enhancers with strong activity in mice (42). This results in occasional misregulation of genes that are oncogenic. In the context of human gene therapy, several integrations near the LMO2 gene led to leukemia (33,34). The approach outlined here provides a way to predict the safety of future gene therapy vectors before they are introduced into patients. Infection of the relevant (or at least closely related) cell type and massively parallel sequencing facilitates the identification of integration preferences, and the use of barcodes introduces a direct means of quantifying or counting specific integrations without PCR-induced skewing. Our method is suitable for determining the distribution of any genomic feature that can be amplified by ligation-mediated PCR, and can be used to track changes in clonal populations over time. We anticipate that such screening will allow researchers to design safer vectors and monitor potentially problematic integration events, thus reducing the risk to patients.
Recent findings have suggested that bromodomain and extraterminal domain (BET) proteins may serve as tethers that guide the integration of MLV (43–45). These reports indicated a role for BET proteins in causing MLV to integrate near TSS. Our findings reveal that regions with high enrichment of multiple marks of active chromatin, such as state 4 strong enhancer regions, are better predictors of MLV integration than TSSs (Figure 5 and Supplementary Figure S3). If BET proteins do serve as MLV integration tethers, perhaps their interaction with the genome is guided by multiple marks of active chromatin. It would be interesting to see the extent to which BET proteins are associated with such regions. For example, one might use ChIP-seq to compare the occupancy of a BET protein, such as Brd4, in state 4 versus its occupancy in other states.
In conclusion, we demonstrated that MLV integration site selection is substantially more specific than the perception that the retrovirus preferentially integrates in or near DHS, or near enhancers in general (14,17,18). The extremely high volume of integrations we analyzed—almost 3.7 million—greatly enhances the power of our tests and accuracy of these results. Similarly, the comprehensive genome annotation produced by the ENCODE consortium is substantially better than what was available to previous studies, revealing connections between integration and chromatin that were previously undetectable. The virus has a strong preference for a specific subset of strong enhancers representing <1% of the genome, and it is likely that other additional factors remain to be discovered. In addition, we have outlined a method amenable to inexpensively detecting hundreds of thousands of unique integrations in a high-throughput manner. We feel the use of this approach as a preclinical screen may help confirm the safety of future gene therapy vectors, and potentially as a mechanism to quickly identify active promoters or enhancers.
ACCESSION NUMBERS
The sequencing data are available at the NCBI Sequence Read Archive [Accession number SRS392021].
FUNDING
This research was supported by the Intramural Research Program of the National Human Genome Research Institute, National Institutes of Health. Funding open access charge: Intramural Research Program of the National Human Genome Research Institute, National Institutes of Health.
Conflict of interest statement. None declared.
ACKNOWLEDGEMENTS
The authors thank L. Brody for comments on the manuscript, N.S. Trivedi for statistical analysis suggestions, A.-D. Nguyen for information on randomization, D. Bodine for providing K562 cells, S. Rane for providing HepG2 cells, J. Fekecs and D. Leja for their work on Figure 2 and R.W. Blakesley, A.Young and the staff of the NIH Intramural Sequencing Center for sequencing. The content of this publication does not necessarily reflect the views or policies of the Department of Health and Human Services, nor does the mention of names, commercial products or organization imply endorsement by the U.S. government. M.C.L. and S.M.B. conceived of and designed the experiment; G.K.V. generated the integrations; G.K.V. and M.C.L. produced the sequencing library; M.C.L. wrote the analysis software and analyzed the data; D.E.G., T.G.W. and A.D.B. consulted on data analysis; M.C.L., G.K.V. and S.M.B. wrote the article. All authors declare that they have reviewed the manuscript.
REFERENCES
Author notes
†These authors contributed equally to the paper as first authors.
Comments