-
PDF
- Split View
-
Views
-
Cite
Cite
Salvatore Capozziello, Rocco D'Agostino, Orlando Luongo, Cosmographic analysis with Chebyshev polynomials, Monthly Notices of the Royal Astronomical Society, Volume 476, Issue 3, May 2018, Pages 3924–3938, https://doi.org/10.1093/mnras/sty422
- Share Icon Share
Abstract
The limits of standard cosmography are here revised addressing the problem of error propagation during statistical analyses. To do so, we propose the use of Chebyshev polynomials to parametrize cosmic distances. In particular, we demonstrate that building up rational Chebyshev polynomials significantly reduces error propagations with respect to standard Taylor series. This technique provides unbiased estimations of the cosmographic parameters and performs significatively better than previous numerical approximations. To figure this out, we compare rational Chebyshev polynomials with Padé series. In addition, we theoretically evaluate the convergence radius of (1,1) Chebyshev rational polynomial and we compare it with the convergence radii of Taylor and Padé approximations. We thus focus on regions in which convergence of Chebyshev rational functions is better than standard approaches. With this recipe, as high-redshift data are employed, rational Chebyshev polynomials remain highly stable and enable one to derive highly accurate analytical approximations of Hubble's rate in terms of the cosmographic series. Finally, we check our theoretical predictions by setting bounds on cosmographic parameters through Monte Carlo integration techniques, based on the Metropolis-Hastings algorithm. We apply our technique to high-redshift cosmic data, using the Joint Light-curve Analysis supernovae sample and the most recent versions of Hubble parameter and baryon acoustic oscillation measurements. We find that cosmography with Taylor series fails to be predictive with the aforementioned data sets, while turns out to be much more stable using the Chebyshev approach.
1 INTRODUCTION
The cosmic acceleration is today confirmed by a large number of observations (Riess et al. 1998; Perlmutter et al. 1999) and represents a consolidate challenge of modern cosmology. To disclose the physics behind it, model-independent techniques have been widely investigated during last years. Strategies towards model-independent treatments have as main target the determination of universe's expansion history without the need of postulating a priori dark energy contributions. A particular attention is currently given to cosmography (Saini et al. 2000; Visser 2004; Cattoën & Visser 2007; Arabsalmani & Sahni 2011; Cai & Tuo 2011; Capozziello, Lazkoz & Salzano 2011; Carvalho & Alcaniz 2011; Guimaraes & Lima 2011; Luongo 2011). Standard cosmography lies on Taylor expansions of cosmic distances. The method provides a powerful tool to study the dark energy evolution without assuming its functional form in the Hubble rate. Moreover, fixing limits over free cosmographic parameters alleviates degeneracy amongst models and enables to understand which paradigms are effectively favoured directly with respect to data surveys. Although cosmography candidates as a robust tool to understand whether dark energy evolves or not, cosmic data unfortunately span on intervals z ≥ 1. This limit poses severe restrictions on cosmography and makes inapplicable Taylor expansions built up around z ≃ 0.
One stratagem to overcome this problem is to parametrize Taylor expansions in terms of auxiliary variables. Unfortunately, even this case turns out to be jeopardized by severe error propagations over the final outcomes. More recently, a further effort has been the use of Padé approximation built-up to converge at higher redshift domains (Gruber & Luongo 2014; Wei, Yan & Zhou 2014). In this case, however, the expansion orders are not fixed a priori and this causes difficulties on evaluating the rate of convergence as z → ∞. Thus, the limits of standard Taylor approach lying on z ≥ 1 are essentially alleviated but not fully fixed.
Motivated by the need of reducing relative uncertainties in cosmography, we here propose a new cosmographic technique based on Chebyshev polynomials. Chebyshev polynomials represent sequences of orthogonal polynomials, recursively defined through trigonometric functions. In our approach, we develop a new Chebyshev cosmography adopting the strategy of building up rational approximations made by these polynomial functions. We demonstrate that, under the hypothesis of rational Chebyshev polynomials, distinguishing Chebyshev functions of first and second kinds is not relevant since the final output gives analogous results in both the cases. For simplicity, we limit our analysis on first kind Chebyshev polynomials only and we write the sequence of Chebyshev rational functions that better approximate Taylor series up to a certain order. We thus show that our Chebyshev ratios provide nodes in polynomial interpolation, minimizing cosmographic uncertainties leading to the most likely well-motivated approximation to cosmic distances. We even present theoretical motivations behind our choice by computing the convergence radii for different choices of polynomial approximations.
To check how well our model works, we also study Padé expansions and we compare the Chebyshev technique with them. We finally show the advantages of our procedure with respect to the old approaches using data surveys, confronting the cosmological quantities built from our method with the observables of the latest cosmological data sets. We adopt a Monte Carlo analysis employing the Metropolis–Hastings algorithm, choosing Joint Light-curve Analysis (JLA) supernova, baryon acoustic oscillation, and differential age measurements. We show the goodness of our procedures comparing the outcomes coming from standard cosmography and our method, showing that error uncertainties are effectively reduced.
The structure of the paper is as follows. In Section 2, we review the general aspects of cosmography. In Section 3, we describe the mathematical features of the Chebyshev polynomials and present the method of the rational Chebyshev approximations. In Section 4, we derive a new model-independent formula for the luminosity distance, and compare the new method with the standard cosmographic procedures to verify the goodness of our approach. In Section 5, we place observational limits on the cosmographic parameters through a confront with the most recent experimental data. Finally, in Section 6, we summarize our findings and conclude.
2 THE COSMOGRAPHIC APPROACH
The study of the cosmic evolution can be done independently of energy densities by means of cosmography. This model-independent technique only relies on the observationally justifiable assumptions of homogeneity and isotropy (Weinberg 1972; Visser 1997; Harrison 1976). The great advantage of this method is that it allows one to reconstruct the dynamical evolution of the dark energy term without assuming any particular cosmological model. Cosmography involves Taylor expansions of observable quantities that may, in principle, go up to any order. These expansions can be compared directly with data. The outcomes of this procedure ensure the independence from any postulated equation of state governing the evolution of the universe and, thus, help to break the degeneracy amongst cosmological models.
which describes the expansion history of the late-time universe up to the snap parameter.
2.1 The convergence problem
In the next section, we present the method of rational Chebyshev polynomials that we will use to obtain a new cosmographic expression for the luminosity distance.
3 RATIONAL CHEBYSHEV POLYNOMIALS
The method we propose here aims to optimize the technique of rational polynomials and consists of approximating the luminosity distance with a ratio of Chebyshev polynomials (Shafieloo 2012). In fact, the Padé approximants are built up from the Taylor approximation of dL(z) whose error bars, by construction, rapidly increase as the redshift departs from zero. Motivated by this issue, we exploit the Chebyshev polynomials. Such a choice aims at reducing the uncertainties on the estimate of the cosmographic parameters.
Since the Chebyshev approximation starts from the definition of dL, one could expect to use it even to discriminate cosmographic coefficients in the framework of extensions of general relativity, for example, in f(R) and f(T) models (Capozziello et al. 2014; Capozziello, Luongo & Saridakis 2015). In this work, for brevity, we focus on general relativity only, but an update approach can be performed revising cosmography of extended theories by following the recipe of Bamba et al. (2012) and checking whether significant departures occur as one extends the Einstein's theory.
In the next section, we apply the mathematical procedure we have presented above to find a very accurate model-independent expression for the luminosity distance. We also compare our method with the cosmographic approaches developed so far in the literature.
4 THE CHEBYSHEV COSMOGRAPHY
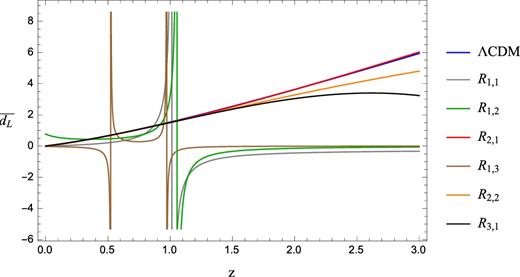
Dimensionless luminosity distance as function of the redshift for rational Chebyshev approximations of the second (R1, 1), third (R1, 2, R2, 1), and fourth (R1, 3, R2, 2, R3, 1) degrees, compared to the ΛCDM model. Here, divergences are due to the natural construction of rational approximations. Choosing arbitrarily the set of coefficients limits the possibility to plot in the whole domain the approximated cosmic distances. Choosing a viable set of priors over coefficients is the key to enable the correct orders of expansion in Chebyshev analyses.
4.1 Calibrating Chebyshev polynomials with the concordance model
In principle, to approximate the ΛCDM model some of the rational Chebyshev polynomials may present singularities turning out to give unsuitable outcomes. To overcome this issue, the preferred rational approximations are those with n − m ≥ 0, in analogy to what happens for Padé approximations (Aviles et al. 2014). In particular, as practically checked the approximant R2, 1(z) seems to give the most accurate approximation to the luminosity distance of the ΛCDM model. Assuming that the calibration with the concordance paradigm would be viable for any possible dark energy term, we assume that the most suitable approximation with Chebyshev polynomials comes from R2, 1(z).
To portray a qualitative representation of numerical improvements that one gains using our method, we compare R2, 1(z) with the standard fourth-order Taylor expansion of dL(z) given in equation (4), and with the (2,2) Padé approximation of dL(z). We choose the (2,2) Padé approximation, since it has been argued that it is robustly characterized by good convergence properties (Aviles et al. 2014) as used in computational analyses. We note that, while in the Taylor and Padé approximations the snap parameter shows up at the fourth order, in the rational Chebyshev polynomials it is present from the lowest degrees, since all the coefficients ck of equation (23) have been calculated from the Taylor series expansion of dL(z) up to the snap order (as confirmed in equation 4). For comparison, we report the expression of the (2,2) Padé approximation of dL(z) in Appendix B. In Fig. 2, we show the behaviour of |$\overline{d_L}(z)$| for the various techniques. As can be seen, the Taylor approach fails when z > 1. Our Chebyshev cosmography stands out for the excellent approximation to the ΛCDM luminosity distance, resulting mostly more effective than Padé approximations.
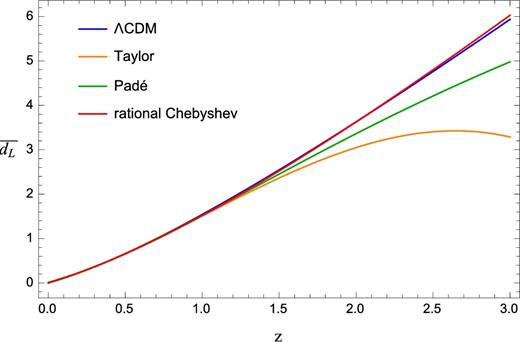
Dimensionless luminosity distance as function of the redshift for the ΛCDM model and its fourth-order Taylor, (2,2) Padé and (2,1) rational Chebyshev approximations. The maximum z for that the ΛCDM model is a good approximation is placed around z ∼ 3. This choice goes further than the highest redshift of BAO measurements and it turns out to be higher than supernova data.
4.2 The convergence radius
We here argue how to broadcast the above considerations to well-motivated theoretical scenarios. Thus, we wonder whether Chebyshev cosmography is expected to effectively improve the approximations to cosmic distances than standard cosmography. To do so, it behooves us to check how much the aforementioned approximations are stable to higher redshifts. Hence, one can test the ability of the various cosmographic techniques, able to describe high-redshift domains, by a direct comparison amongst the corresponding convergence radii, here defined by ρ.
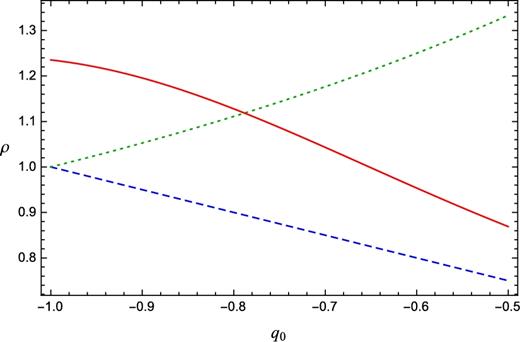
Convergence radii for the second-order Taylor (dashed curve), (1,1) Padé (dotted curve), and (1,1) rational Chebyshev (solid curve) approximations of the luminosity distance as a function of q0. For the rational Chebyshev approximation, we used the indicative values of j0 = 2, s0 = −1.
5 OBSERVATIONAL CONSTRAINTS
In this section, we present the data we use to set bounds on the cosmographic parameters.
5.1 Supernovae Ia
5.2 Observational Hubble data
5.3 Baryon acoustic oscillations
5.4 Results of the Monte Carlo analysis
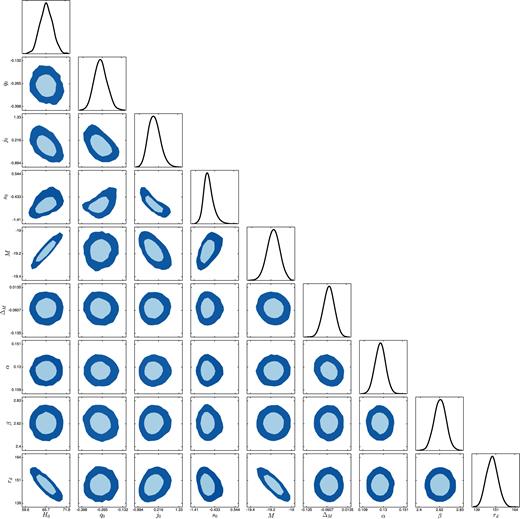
68 per cent and 95 per cent confidence level contours and posterior distributions from the MCMC analysis of SN+OHD+BAO data for the fourth-order Taylor approximation of the luminosity distance. H0 is expressed in km s−1 Mpc−1, and rd in Mpc.
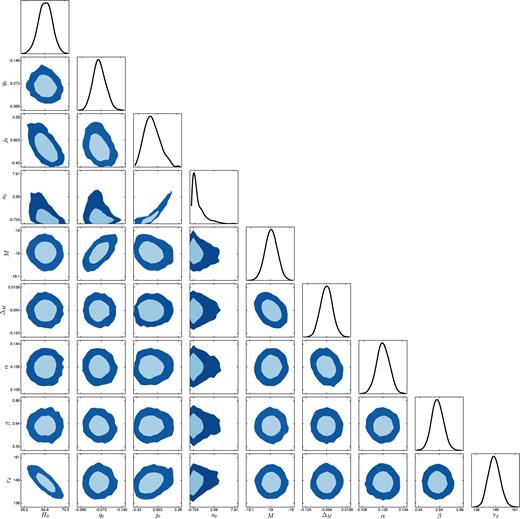
68 per cent and 95 per cent confidence level contours and posterior distributions from the MCMC analysis of SN+OHD+BAO data for the (2,2) Padé approximation of the luminosity distance. H0 is expressed in km s−1 Mpc−1, and rd in Mpc.
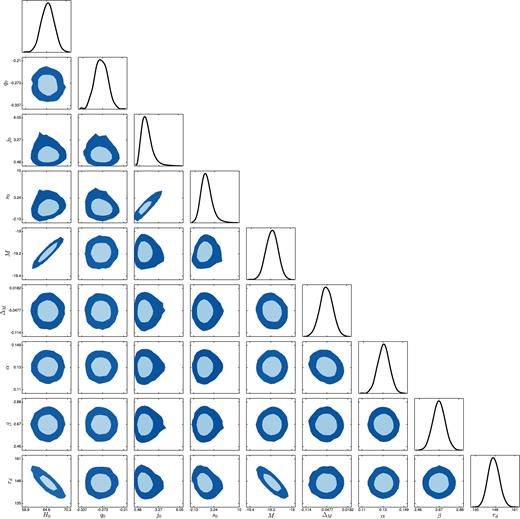
68 per cent and 95 per cent confidence level contours and posterior distributions from the MCMC analysis of SN+OHD+BAO data for the (2,1) rational Chebyshev approximation of the luminosity distance. H0 is expressed in km s−1 Mpc−1, and rd in Mpc.
Priors for parameters estimate in the MCMC numerical analysis. H0 values are given in units of km s−1 Mpc−1, while rd values in units of Mpc.
Parameters . | Priors . |
---|---|
H0 | (50, 90) |
q0 | (−10, 10) |
j0 | (−10, 10) |
s0 | (−10, 10) |
M | (−20, −18) |
ΔM | (−1, 1) |
α | (0, 1) |
β | (0, 5) |
rd | (140, 160) |
Parameters . | Priors . |
---|---|
H0 | (50, 90) |
q0 | (−10, 10) |
j0 | (−10, 10) |
s0 | (−10, 10) |
M | (−20, −18) |
ΔM | (−1, 1) |
α | (0, 1) |
β | (0, 5) |
rd | (140, 160) |
Priors for parameters estimate in the MCMC numerical analysis. H0 values are given in units of km s−1 Mpc−1, while rd values in units of Mpc.
Parameters . | Priors . |
---|---|
H0 | (50, 90) |
q0 | (−10, 10) |
j0 | (−10, 10) |
s0 | (−10, 10) |
M | (−20, −18) |
ΔM | (−1, 1) |
α | (0, 1) |
β | (0, 5) |
rd | (140, 160) |
Parameters . | Priors . |
---|---|
H0 | (50, 90) |
q0 | (−10, 10) |
j0 | (−10, 10) |
s0 | (−10, 10) |
M | (−20, −18) |
ΔM | (−1, 1) |
α | (0, 1) |
β | (0, 5) |
rd | (140, 160) |
68 per cent and 95 per cent confidence level parameter constraints from the MCMC analysis of SN+OHD+BAO data for the fourth-order Taylor, (2,2) Padé and (2,1) rational Chebyshev polynomial approximations of the luminosity distance. H0 values are given in units of km s−1 Mpc−1, while rd values in units of Mpc.
Parameter . | Taylor . | Padé . | Rational Chebyshev . | ||||||
---|---|---|---|---|---|---|---|---|---|
. | Mean . | 1σ . | 2σ . | Mean . | 1σ . | 2σ . | Mean . | 1σ . | 2σ . |
H0 | 65.80 | |$^{+2.09}_{-2.11}$| | |$^{+4.22}_{-4.00}$| | 64.94 | |$^{+2.11}_{-2.02}$| | |$^{+4.12}_{-4.13}$| | 64.95 | |$^{+1.89}_{-1.94}$| | |$^{+3.77}_{-3.77}$| |
q0 | −0.276 | |$^{+0.043}_{-0.049}$| | |$^{+0.093}_{-0.091}$| | −0.285 | |$^{+0.040}_{-0.046}$| | |$^{+0.087}_{-0.084}$| | −0.278 | |$^{+0.021}_{-0.021}$| | |$^{+0.041}_{-0.042}$| |
j0 | −0.023 | |$^{+0.317}_{-0.397}$| | |$^{+0.748}_{-0.685}$| | 0.545 | |$^{+0.463}_{-0.652}$| | |$^{+1.135}_{-1.025}$| | 1.585 | |$^{+0.497}_{-0.914}$| | |$^{+1.594}_{-1.453}$| |
s0 | −0.745 | |$^{+0.196}_{-0.284}$| | |$^{+0.564}_{-0.487}$| | 0.118 | |$^{+0.451}_{-1.600}$| | |$^{+3.422}_{-1.921}$| | 1.041 | |$^{+1.183}_{-1.784}$| | |$^{+3.388}_{-3.087}$| |
M | −19.16 | |$^{+0.07}_{-0.07}$| | |$^{+0.14}_{-0.14}$| | −19.03 | |$^{+0.02}_{-0.02}$| | |$^{+0.05}_{-0.05}$| | −19.17 | |$^{+0.07}_{-0.07}$| | |$^{+0.13}_{-0.13}$| |
ΔM | −0.054 | |$^{+0.023}_{-0.022}$| | |$^{+0.044}_{-0.045}$| | −0.054 | |$^{+0.022}_{-0.023}$| | |$^{+0.045}_{-0.045}$| | −0.050 | |$^{+0.022}_{-0.022}$| | |$^{+0.044}_{-0.045}$| |
α | 0.127 | |$^{+0.006}_{-0.006}$| | |$^{+0.012}_{-0.012}$| | 0.127 | |$^{+0.006}_{-0.006}$| | |$^{+0.012}_{-0.012}$| | 0.130 | |$^{+0.006}_{-0.006}$| | |$^{+0.012}_{-0.012}$| |
β | 2.624 | |$^{+0.071}_{-0.068}$| | |$^{+0.136}_{-0.140}$| | 2.625 | |$^{+0.065}_{-0.069}$| | |$^{+0.137}_{-0.135}$| | 2.667 | |$^{+0.068}_{-0.069}$| | |$^{+0.137}_{-0.135}$| |
rd | 149.2 | |$^{+3.7}_{-4.1}$| | |$^{+7.7}_{-7.5}$| | 148.6 | |$^{+3.5}_{-3.8}$| | |$^{+7.5}_{-7.1}$| | 147.2 | |$^{+3.7}_{-4.0}$| | |$^{+7.8}_{-7.5}$| |
Parameter . | Taylor . | Padé . | Rational Chebyshev . | ||||||
---|---|---|---|---|---|---|---|---|---|
. | Mean . | 1σ . | 2σ . | Mean . | 1σ . | 2σ . | Mean . | 1σ . | 2σ . |
H0 | 65.80 | |$^{+2.09}_{-2.11}$| | |$^{+4.22}_{-4.00}$| | 64.94 | |$^{+2.11}_{-2.02}$| | |$^{+4.12}_{-4.13}$| | 64.95 | |$^{+1.89}_{-1.94}$| | |$^{+3.77}_{-3.77}$| |
q0 | −0.276 | |$^{+0.043}_{-0.049}$| | |$^{+0.093}_{-0.091}$| | −0.285 | |$^{+0.040}_{-0.046}$| | |$^{+0.087}_{-0.084}$| | −0.278 | |$^{+0.021}_{-0.021}$| | |$^{+0.041}_{-0.042}$| |
j0 | −0.023 | |$^{+0.317}_{-0.397}$| | |$^{+0.748}_{-0.685}$| | 0.545 | |$^{+0.463}_{-0.652}$| | |$^{+1.135}_{-1.025}$| | 1.585 | |$^{+0.497}_{-0.914}$| | |$^{+1.594}_{-1.453}$| |
s0 | −0.745 | |$^{+0.196}_{-0.284}$| | |$^{+0.564}_{-0.487}$| | 0.118 | |$^{+0.451}_{-1.600}$| | |$^{+3.422}_{-1.921}$| | 1.041 | |$^{+1.183}_{-1.784}$| | |$^{+3.388}_{-3.087}$| |
M | −19.16 | |$^{+0.07}_{-0.07}$| | |$^{+0.14}_{-0.14}$| | −19.03 | |$^{+0.02}_{-0.02}$| | |$^{+0.05}_{-0.05}$| | −19.17 | |$^{+0.07}_{-0.07}$| | |$^{+0.13}_{-0.13}$| |
ΔM | −0.054 | |$^{+0.023}_{-0.022}$| | |$^{+0.044}_{-0.045}$| | −0.054 | |$^{+0.022}_{-0.023}$| | |$^{+0.045}_{-0.045}$| | −0.050 | |$^{+0.022}_{-0.022}$| | |$^{+0.044}_{-0.045}$| |
α | 0.127 | |$^{+0.006}_{-0.006}$| | |$^{+0.012}_{-0.012}$| | 0.127 | |$^{+0.006}_{-0.006}$| | |$^{+0.012}_{-0.012}$| | 0.130 | |$^{+0.006}_{-0.006}$| | |$^{+0.012}_{-0.012}$| |
β | 2.624 | |$^{+0.071}_{-0.068}$| | |$^{+0.136}_{-0.140}$| | 2.625 | |$^{+0.065}_{-0.069}$| | |$^{+0.137}_{-0.135}$| | 2.667 | |$^{+0.068}_{-0.069}$| | |$^{+0.137}_{-0.135}$| |
rd | 149.2 | |$^{+3.7}_{-4.1}$| | |$^{+7.7}_{-7.5}$| | 148.6 | |$^{+3.5}_{-3.8}$| | |$^{+7.5}_{-7.1}$| | 147.2 | |$^{+3.7}_{-4.0}$| | |$^{+7.8}_{-7.5}$| |
68 per cent and 95 per cent confidence level parameter constraints from the MCMC analysis of SN+OHD+BAO data for the fourth-order Taylor, (2,2) Padé and (2,1) rational Chebyshev polynomial approximations of the luminosity distance. H0 values are given in units of km s−1 Mpc−1, while rd values in units of Mpc.
Parameter . | Taylor . | Padé . | Rational Chebyshev . | ||||||
---|---|---|---|---|---|---|---|---|---|
. | Mean . | 1σ . | 2σ . | Mean . | 1σ . | 2σ . | Mean . | 1σ . | 2σ . |
H0 | 65.80 | |$^{+2.09}_{-2.11}$| | |$^{+4.22}_{-4.00}$| | 64.94 | |$^{+2.11}_{-2.02}$| | |$^{+4.12}_{-4.13}$| | 64.95 | |$^{+1.89}_{-1.94}$| | |$^{+3.77}_{-3.77}$| |
q0 | −0.276 | |$^{+0.043}_{-0.049}$| | |$^{+0.093}_{-0.091}$| | −0.285 | |$^{+0.040}_{-0.046}$| | |$^{+0.087}_{-0.084}$| | −0.278 | |$^{+0.021}_{-0.021}$| | |$^{+0.041}_{-0.042}$| |
j0 | −0.023 | |$^{+0.317}_{-0.397}$| | |$^{+0.748}_{-0.685}$| | 0.545 | |$^{+0.463}_{-0.652}$| | |$^{+1.135}_{-1.025}$| | 1.585 | |$^{+0.497}_{-0.914}$| | |$^{+1.594}_{-1.453}$| |
s0 | −0.745 | |$^{+0.196}_{-0.284}$| | |$^{+0.564}_{-0.487}$| | 0.118 | |$^{+0.451}_{-1.600}$| | |$^{+3.422}_{-1.921}$| | 1.041 | |$^{+1.183}_{-1.784}$| | |$^{+3.388}_{-3.087}$| |
M | −19.16 | |$^{+0.07}_{-0.07}$| | |$^{+0.14}_{-0.14}$| | −19.03 | |$^{+0.02}_{-0.02}$| | |$^{+0.05}_{-0.05}$| | −19.17 | |$^{+0.07}_{-0.07}$| | |$^{+0.13}_{-0.13}$| |
ΔM | −0.054 | |$^{+0.023}_{-0.022}$| | |$^{+0.044}_{-0.045}$| | −0.054 | |$^{+0.022}_{-0.023}$| | |$^{+0.045}_{-0.045}$| | −0.050 | |$^{+0.022}_{-0.022}$| | |$^{+0.044}_{-0.045}$| |
α | 0.127 | |$^{+0.006}_{-0.006}$| | |$^{+0.012}_{-0.012}$| | 0.127 | |$^{+0.006}_{-0.006}$| | |$^{+0.012}_{-0.012}$| | 0.130 | |$^{+0.006}_{-0.006}$| | |$^{+0.012}_{-0.012}$| |
β | 2.624 | |$^{+0.071}_{-0.068}$| | |$^{+0.136}_{-0.140}$| | 2.625 | |$^{+0.065}_{-0.069}$| | |$^{+0.137}_{-0.135}$| | 2.667 | |$^{+0.068}_{-0.069}$| | |$^{+0.137}_{-0.135}$| |
rd | 149.2 | |$^{+3.7}_{-4.1}$| | |$^{+7.7}_{-7.5}$| | 148.6 | |$^{+3.5}_{-3.8}$| | |$^{+7.5}_{-7.1}$| | 147.2 | |$^{+3.7}_{-4.0}$| | |$^{+7.8}_{-7.5}$| |
Parameter . | Taylor . | Padé . | Rational Chebyshev . | ||||||
---|---|---|---|---|---|---|---|---|---|
. | Mean . | 1σ . | 2σ . | Mean . | 1σ . | 2σ . | Mean . | 1σ . | 2σ . |
H0 | 65.80 | |$^{+2.09}_{-2.11}$| | |$^{+4.22}_{-4.00}$| | 64.94 | |$^{+2.11}_{-2.02}$| | |$^{+4.12}_{-4.13}$| | 64.95 | |$^{+1.89}_{-1.94}$| | |$^{+3.77}_{-3.77}$| |
q0 | −0.276 | |$^{+0.043}_{-0.049}$| | |$^{+0.093}_{-0.091}$| | −0.285 | |$^{+0.040}_{-0.046}$| | |$^{+0.087}_{-0.084}$| | −0.278 | |$^{+0.021}_{-0.021}$| | |$^{+0.041}_{-0.042}$| |
j0 | −0.023 | |$^{+0.317}_{-0.397}$| | |$^{+0.748}_{-0.685}$| | 0.545 | |$^{+0.463}_{-0.652}$| | |$^{+1.135}_{-1.025}$| | 1.585 | |$^{+0.497}_{-0.914}$| | |$^{+1.594}_{-1.453}$| |
s0 | −0.745 | |$^{+0.196}_{-0.284}$| | |$^{+0.564}_{-0.487}$| | 0.118 | |$^{+0.451}_{-1.600}$| | |$^{+3.422}_{-1.921}$| | 1.041 | |$^{+1.183}_{-1.784}$| | |$^{+3.388}_{-3.087}$| |
M | −19.16 | |$^{+0.07}_{-0.07}$| | |$^{+0.14}_{-0.14}$| | −19.03 | |$^{+0.02}_{-0.02}$| | |$^{+0.05}_{-0.05}$| | −19.17 | |$^{+0.07}_{-0.07}$| | |$^{+0.13}_{-0.13}$| |
ΔM | −0.054 | |$^{+0.023}_{-0.022}$| | |$^{+0.044}_{-0.045}$| | −0.054 | |$^{+0.022}_{-0.023}$| | |$^{+0.045}_{-0.045}$| | −0.050 | |$^{+0.022}_{-0.022}$| | |$^{+0.044}_{-0.045}$| |
α | 0.127 | |$^{+0.006}_{-0.006}$| | |$^{+0.012}_{-0.012}$| | 0.127 | |$^{+0.006}_{-0.006}$| | |$^{+0.012}_{-0.012}$| | 0.130 | |$^{+0.006}_{-0.006}$| | |$^{+0.012}_{-0.012}$| |
β | 2.624 | |$^{+0.071}_{-0.068}$| | |$^{+0.136}_{-0.140}$| | 2.625 | |$^{+0.065}_{-0.069}$| | |$^{+0.137}_{-0.135}$| | 2.667 | |$^{+0.068}_{-0.069}$| | |$^{+0.137}_{-0.135}$| |
rd | 149.2 | |$^{+3.7}_{-4.1}$| | |$^{+7.7}_{-7.5}$| | 148.6 | |$^{+3.5}_{-3.8}$| | |$^{+7.5}_{-7.1}$| | 147.2 | |$^{+3.7}_{-4.0}$| | |$^{+7.8}_{-7.5}$| |
68 per cent and 95 per cent relative uncertainties on the estimate of the cosmographic parameters from the MCMC analysis of SN+OHD+BAO data for the fourth-order Taylor, (2,2) Padé and (2,1) rational Chebyshev polynomial approximations of the luminosity distance.
Parameter . | Taylor . | Padé . | Rational Chebyshev . | |||
---|---|---|---|---|---|---|
. | 1σ( per cent) . | 2σ( per cent) . | |$1\sigma\,\,(\rm{per\,\,cent})$| . | |$2\sigma\,\,(\rm{per\,\,cent})$| . | |$1\sigma\,\,(\rm{per\,\,cent})$| . | |$2\sigma\,\,(\rm{per\,\,cent})$| . |
H0 | 3.19 | 6.25 | 3.17 | 6.35 | 2.95 | 4.11 |
q0 | 16.8 | 33.5 | 15.1 | 30.1 | 7.66 | 14.8 |
j0 | 1534 | 3079 | 102 | 198 | 44.5 | 96.1 |
s0 | 32.2 | 70.5 | 866 | 2258 | 142 | 311 |
Parameter . | Taylor . | Padé . | Rational Chebyshev . | |||
---|---|---|---|---|---|---|
. | 1σ( per cent) . | 2σ( per cent) . | |$1\sigma\,\,(\rm{per\,\,cent})$| . | |$2\sigma\,\,(\rm{per\,\,cent})$| . | |$1\sigma\,\,(\rm{per\,\,cent})$| . | |$2\sigma\,\,(\rm{per\,\,cent})$| . |
H0 | 3.19 | 6.25 | 3.17 | 6.35 | 2.95 | 4.11 |
q0 | 16.8 | 33.5 | 15.1 | 30.1 | 7.66 | 14.8 |
j0 | 1534 | 3079 | 102 | 198 | 44.5 | 96.1 |
s0 | 32.2 | 70.5 | 866 | 2258 | 142 | 311 |
68 per cent and 95 per cent relative uncertainties on the estimate of the cosmographic parameters from the MCMC analysis of SN+OHD+BAO data for the fourth-order Taylor, (2,2) Padé and (2,1) rational Chebyshev polynomial approximations of the luminosity distance.
Parameter . | Taylor . | Padé . | Rational Chebyshev . | |||
---|---|---|---|---|---|---|
. | 1σ( per cent) . | 2σ( per cent) . | |$1\sigma\,\,(\rm{per\,\,cent})$| . | |$2\sigma\,\,(\rm{per\,\,cent})$| . | |$1\sigma\,\,(\rm{per\,\,cent})$| . | |$2\sigma\,\,(\rm{per\,\,cent})$| . |
H0 | 3.19 | 6.25 | 3.17 | 6.35 | 2.95 | 4.11 |
q0 | 16.8 | 33.5 | 15.1 | 30.1 | 7.66 | 14.8 |
j0 | 1534 | 3079 | 102 | 198 | 44.5 | 96.1 |
s0 | 32.2 | 70.5 | 866 | 2258 | 142 | 311 |
Parameter . | Taylor . | Padé . | Rational Chebyshev . | |||
---|---|---|---|---|---|---|
. | 1σ( per cent) . | 2σ( per cent) . | |$1\sigma\,\,(\rm{per\,\,cent})$| . | |$2\sigma\,\,(\rm{per\,\,cent})$| . | |$1\sigma\,\,(\rm{per\,\,cent})$| . | |$2\sigma\,\,(\rm{per\,\,cent})$| . |
H0 | 3.19 | 6.25 | 3.17 | 6.35 | 2.95 | 4.11 |
q0 | 16.8 | 33.5 | 15.1 | 30.1 | 7.66 | 14.8 |
j0 | 1534 | 3079 | 102 | 198 | 44.5 | 96.1 |
s0 | 32.2 | 70.5 | 866 | 2258 | 142 | 311 |
An alternative approach is to start from the cosmographic expansion series of the Hubble rate and, then, evaluate the luminosity distance by numerical integrations, as pointed out in Aviles, Klapp & Luongo (2017). However, it turns out that the analysis based on rational Chebyshev approximations of H(z) does not lead to further reduction of the error propagation with respect to our original approach. An interesting fact is that, by construction, one uses Chebyshev polynomials with lower orders than Taylor series and Padé approximants. This mostly reduces the computational difficulties in implementing cosmic data, although does not accurately fixes the highest-order parameter in the approximation. This is the case of s0 whose error bars are not significatively improved adopting Chebyshev polynomials. To overcome this issue, it would be enough to increase the Chebyshev order to better fix s0 than Taylor and Padé treatments.
6 SUMMARY AND CONCLUSIONS
In this work, we revised the convergence problem in cosmography, adopting a new method to reduce error uncertainties and bias propagations as high-redshift data are used. We set bounds on the cosmographic series, considering rational approximations of the luminosity distance and we demonstrated that such approximations are also valid for any cosmic distances. In particular, our novel procedure is based on approximating the luminosity distance dL(z) with ratios of Chebyshev polynomials. Since, by definition, Chebyshev approximants are the most suitable polynomials in approximating functions, we expected to get fairly good outcomes in computing cosmographic series. Indeed, we found that our approach overcomes the convergence issues typical of standard cosmographic techniques based on Taylor approximations. This has been confirmed by computing convergence radii for different sets of cosmographic coefficients. We also compared our new approach with the consolidate procedure of Padé expansions. We showed that both numerical bounds and convergence radii are improved under precise conditions. This naturally showed that Chebyshev rational polynomials are more suitable to describe the cosmic dynamics at z > 1 than Padé series. Bearing this in mind, through the predictions of the concordance ΛCDM model, we calibrated the orders of Chebyshev rational polynomials, providing the recipe of a new Chebyshev cosmography, which turned out to be more predictive than Taylor series at all redshift domains. We evaluated the (2,1) Chebyshev series, corresponding to a fourth-order Tayor series and to a (2,2) Padé approximation. This showed that lower order Chebyshev series work better than higher ones constructed by Taylor and Padé recipes. We finally checked the goodness of our method, statistically combining the JLA supernova compilation, H(z) differential age data, and baryon acoustic oscillation measurements by performing Monte Carlo integrations based on the Metropolis–Hastings algorithm. To do so, we employed the free available Monte python code and we computed the corresponding contours up to the |$95\,\,\rm{per\,\,cent}$| confidence level. The numerical improvements have been reported in terms of percentages on 1σ and 2σ confidence levels. The error percentages are severely lowered, whereas the mean values are centred around intervals compatible with previous results on cosmography.
Our final outcomes forecast that the technique of Chebyshev cosmography substantially decreases the relative uncertainties on the estimates of cosmographic parameters respect to other previous approaches. This procedure candidates as a new way towards computing the cosmographic series and to better fix constraints on cosmography. Chebyshev cosmography is thus able to heal previous inconsistencies on convergence that plagued cosmography itself. Further, it enables to use high-redshift data surveys in cosmographic analyses with no large spreads overfitted coefficients.
Future efforts will be devoted to match Padé and Chebyshev techniques in different redshift domains. We will work on characterizing the cosmographic data over binning intervals in which one will be able to highly maximize both the convergence radii and mean values and to minimize both bias and uncertainties on free cosmographic coefficients. Hence, it will be possible to go further with our approximation, even investigating Chebyshev approximations using future data got for example from the cosmic microwave domain.
ACKNOWLEDGEMENTS
This paper is based upon work from COST action CA15117 (CANTATA), supported by COST (European Cooperation in Science and Technology). The authors warmly thank the anonymous referee for comments and suggestions that permitted to improve the quality of the paper. S.C. acknowledges the support of INFN (iniziativa specifica QGSKY). R.D. thanks Federico Tosone for useful discussions on the Monte python code.
Footnotes
The assumption of flatness overcomes problems of degeneracy amongst the cosmographic parameters entering the expression of the luminosity distance (Dunsby & Luongo 2016).
In principle, one may go further in the expansion and consider higher order coefficients. We limit our study up to the snap, since the next cosmographic parameters are poorly constrained by observations (Aviles et al. 2012).
Throughout the text, we refer to the Chebyshev polynomials of the first kind simply as Chebyshev polynomials.
We here truncate our analysis to the fifth order, since additional contributions go beyond our treatment. In so doing, we arrive to analyse up to snap parameter s0.
REFERENCES
APPENDIX A: RATIONAL CHEBYSHEV APPROXIMATIONS OF THE LUMINOSITY DISTANCE
APPENDIX B: (2,2) PADÉ APPROXIMANT OF THE LUMINOSITY DISTANCE
APPENDIX C: EXPERIMENTAL DATA
In Tables C1 and C2, we list the compilations of OHD data and BAO data used to perform the Monte Carlo analysis.
Differential age H(z) data used in this work. The Hubble rate is given in units of km s−1 Mpc−1.
z . | H ± σH . | Reference . |
---|---|---|
0.0708 | 69.00 ± 19.68 | Zhang et al. (2014) |
0.09 | 69.0 ± 12.0 | Jimenez & Loeb (2002) |
0.12 | 68.6 ± 26.2 | Zhang et al. (2014) |
0.17 | 83.0 ± 8.0 | Simon, Verde & Jimenez (2005) |
0.179 | 75.0 ± 4.0 | Moresco et al. (2012) |
0.199 | 75.0 ± 5.0 | Moresco et al. (2012) |
0.20 | 72.9 ± 29.6 | Zhang et al. (2014) |
0.27 | 77.0 ± 14.0 | Simon et al. (2005) |
0.28 | 88.8 ± 36.6 | Zhang et al. (2014) |
0.35 | 82.1 ± 4.85 | Chuang & Wang (2012) |
0.352 | 83.0 ± 14.0 | Moresco et al. (2016) |
0.3802 | 83.0 ± 13.5 | Moresco et al. (2016) |
0.4 | 95.0 ± 17.0 | Simon et al. (2005) |
0.4004 | 77.0 ± 10.2 | Moresco et al. (2016) |
0.4247 | 87.1 ± 11.2 | Moresco et al. (2016) |
0.4497 | 92.8 ± 12.9 | Moresco et al. (2016) |
0.4783 | 80.9 ± 9.0 | Moresco et al. (2016) |
0.48 | 97.0 ± 62.0 | Stern et al. (2010) |
0.593 | 104.0 ± 13.0 | Moresco et al. (2012) |
0.68 | 92.0 ± 8.0 | Moresco et al. (2012) |
0.781 | 105.0 ± 12.0 | Moresco et al. (2012) |
0.875 | 125.0 ± 17.0 | Moresco et al. (2012) |
0.88 | 90.0 ± 40.0 | Stern et al. (2010) |
0.9 | 117.0 ± 23.0 | Simon et al. (2005) |
1.037 | 154.0 ± 20.0 | Moresco et al. (2012) |
1.3 | 168.0 ± 17.0 | Simon et al. (2005) |
1.363 | 160.0 ± 33.6 | Moresco (2015) |
1.43 | 177.0 ± 18.0 | Simon et al. (2005) |
1.53 | 140.0 ± 14.0 | Simon et al. (2005) |
1.75 | 202.0 ± 40.0 | Simon et al. (2005) |
1.965 | 186.5 ± 50.4 | Moresco (2015) |
z . | H ± σH . | Reference . |
---|---|---|
0.0708 | 69.00 ± 19.68 | Zhang et al. (2014) |
0.09 | 69.0 ± 12.0 | Jimenez & Loeb (2002) |
0.12 | 68.6 ± 26.2 | Zhang et al. (2014) |
0.17 | 83.0 ± 8.0 | Simon, Verde & Jimenez (2005) |
0.179 | 75.0 ± 4.0 | Moresco et al. (2012) |
0.199 | 75.0 ± 5.0 | Moresco et al. (2012) |
0.20 | 72.9 ± 29.6 | Zhang et al. (2014) |
0.27 | 77.0 ± 14.0 | Simon et al. (2005) |
0.28 | 88.8 ± 36.6 | Zhang et al. (2014) |
0.35 | 82.1 ± 4.85 | Chuang & Wang (2012) |
0.352 | 83.0 ± 14.0 | Moresco et al. (2016) |
0.3802 | 83.0 ± 13.5 | Moresco et al. (2016) |
0.4 | 95.0 ± 17.0 | Simon et al. (2005) |
0.4004 | 77.0 ± 10.2 | Moresco et al. (2016) |
0.4247 | 87.1 ± 11.2 | Moresco et al. (2016) |
0.4497 | 92.8 ± 12.9 | Moresco et al. (2016) |
0.4783 | 80.9 ± 9.0 | Moresco et al. (2016) |
0.48 | 97.0 ± 62.0 | Stern et al. (2010) |
0.593 | 104.0 ± 13.0 | Moresco et al. (2012) |
0.68 | 92.0 ± 8.0 | Moresco et al. (2012) |
0.781 | 105.0 ± 12.0 | Moresco et al. (2012) |
0.875 | 125.0 ± 17.0 | Moresco et al. (2012) |
0.88 | 90.0 ± 40.0 | Stern et al. (2010) |
0.9 | 117.0 ± 23.0 | Simon et al. (2005) |
1.037 | 154.0 ± 20.0 | Moresco et al. (2012) |
1.3 | 168.0 ± 17.0 | Simon et al. (2005) |
1.363 | 160.0 ± 33.6 | Moresco (2015) |
1.43 | 177.0 ± 18.0 | Simon et al. (2005) |
1.53 | 140.0 ± 14.0 | Simon et al. (2005) |
1.75 | 202.0 ± 40.0 | Simon et al. (2005) |
1.965 | 186.5 ± 50.4 | Moresco (2015) |
Differential age H(z) data used in this work. The Hubble rate is given in units of km s−1 Mpc−1.
z . | H ± σH . | Reference . |
---|---|---|
0.0708 | 69.00 ± 19.68 | Zhang et al. (2014) |
0.09 | 69.0 ± 12.0 | Jimenez & Loeb (2002) |
0.12 | 68.6 ± 26.2 | Zhang et al. (2014) |
0.17 | 83.0 ± 8.0 | Simon, Verde & Jimenez (2005) |
0.179 | 75.0 ± 4.0 | Moresco et al. (2012) |
0.199 | 75.0 ± 5.0 | Moresco et al. (2012) |
0.20 | 72.9 ± 29.6 | Zhang et al. (2014) |
0.27 | 77.0 ± 14.0 | Simon et al. (2005) |
0.28 | 88.8 ± 36.6 | Zhang et al. (2014) |
0.35 | 82.1 ± 4.85 | Chuang & Wang (2012) |
0.352 | 83.0 ± 14.0 | Moresco et al. (2016) |
0.3802 | 83.0 ± 13.5 | Moresco et al. (2016) |
0.4 | 95.0 ± 17.0 | Simon et al. (2005) |
0.4004 | 77.0 ± 10.2 | Moresco et al. (2016) |
0.4247 | 87.1 ± 11.2 | Moresco et al. (2016) |
0.4497 | 92.8 ± 12.9 | Moresco et al. (2016) |
0.4783 | 80.9 ± 9.0 | Moresco et al. (2016) |
0.48 | 97.0 ± 62.0 | Stern et al. (2010) |
0.593 | 104.0 ± 13.0 | Moresco et al. (2012) |
0.68 | 92.0 ± 8.0 | Moresco et al. (2012) |
0.781 | 105.0 ± 12.0 | Moresco et al. (2012) |
0.875 | 125.0 ± 17.0 | Moresco et al. (2012) |
0.88 | 90.0 ± 40.0 | Stern et al. (2010) |
0.9 | 117.0 ± 23.0 | Simon et al. (2005) |
1.037 | 154.0 ± 20.0 | Moresco et al. (2012) |
1.3 | 168.0 ± 17.0 | Simon et al. (2005) |
1.363 | 160.0 ± 33.6 | Moresco (2015) |
1.43 | 177.0 ± 18.0 | Simon et al. (2005) |
1.53 | 140.0 ± 14.0 | Simon et al. (2005) |
1.75 | 202.0 ± 40.0 | Simon et al. (2005) |
1.965 | 186.5 ± 50.4 | Moresco (2015) |
z . | H ± σH . | Reference . |
---|---|---|
0.0708 | 69.00 ± 19.68 | Zhang et al. (2014) |
0.09 | 69.0 ± 12.0 | Jimenez & Loeb (2002) |
0.12 | 68.6 ± 26.2 | Zhang et al. (2014) |
0.17 | 83.0 ± 8.0 | Simon, Verde & Jimenez (2005) |
0.179 | 75.0 ± 4.0 | Moresco et al. (2012) |
0.199 | 75.0 ± 5.0 | Moresco et al. (2012) |
0.20 | 72.9 ± 29.6 | Zhang et al. (2014) |
0.27 | 77.0 ± 14.0 | Simon et al. (2005) |
0.28 | 88.8 ± 36.6 | Zhang et al. (2014) |
0.35 | 82.1 ± 4.85 | Chuang & Wang (2012) |
0.352 | 83.0 ± 14.0 | Moresco et al. (2016) |
0.3802 | 83.0 ± 13.5 | Moresco et al. (2016) |
0.4 | 95.0 ± 17.0 | Simon et al. (2005) |
0.4004 | 77.0 ± 10.2 | Moresco et al. (2016) |
0.4247 | 87.1 ± 11.2 | Moresco et al. (2016) |
0.4497 | 92.8 ± 12.9 | Moresco et al. (2016) |
0.4783 | 80.9 ± 9.0 | Moresco et al. (2016) |
0.48 | 97.0 ± 62.0 | Stern et al. (2010) |
0.593 | 104.0 ± 13.0 | Moresco et al. (2012) |
0.68 | 92.0 ± 8.0 | Moresco et al. (2012) |
0.781 | 105.0 ± 12.0 | Moresco et al. (2012) |
0.875 | 125.0 ± 17.0 | Moresco et al. (2012) |
0.88 | 90.0 ± 40.0 | Stern et al. (2010) |
0.9 | 117.0 ± 23.0 | Simon et al. (2005) |
1.037 | 154.0 ± 20.0 | Moresco et al. (2012) |
1.3 | 168.0 ± 17.0 | Simon et al. (2005) |
1.363 | 160.0 ± 33.6 | Moresco (2015) |
1.43 | 177.0 ± 18.0 | Simon et al. (2005) |
1.53 | 140.0 ± 14.0 | Simon et al. (2005) |
1.75 | 202.0 ± 40.0 | Simon et al. (2005) |
1.965 | 186.5 ± 50.4 | Moresco (2015) |
z . | |$d_V \pm \sigma _{d_V}$| . | Survey . | Reference . |
---|---|---|---|
0.106 | 0.336 ± 0.015 | 6dFGS | Beutler et al. (2011) |
0.15 | 0.2239 ± 0.0084 | SDSS DR7 | Ross et al. (2015) |
0.32 | 0.1181 ± 0.0023 | BOSS DR11 | Anderson et al. (2014) |
0.57 | 0.0726 ± 0.0007 | BOSS DR11 | Anderson et al. (2014) |
2.34 | 0.0320 ± 0.0016 | BOSS DR11 | Delubac et al. (2015) |
2.36 | 0.0329 ± 0.0012 | BOSS DR11 | Font-Ribera et al. (2014) |
z . | |$d_V \pm \sigma _{d_V}$| . | Survey . | Reference . |
---|---|---|---|
0.106 | 0.336 ± 0.015 | 6dFGS | Beutler et al. (2011) |
0.15 | 0.2239 ± 0.0084 | SDSS DR7 | Ross et al. (2015) |
0.32 | 0.1181 ± 0.0023 | BOSS DR11 | Anderson et al. (2014) |
0.57 | 0.0726 ± 0.0007 | BOSS DR11 | Anderson et al. (2014) |
2.34 | 0.0320 ± 0.0016 | BOSS DR11 | Delubac et al. (2015) |
2.36 | 0.0329 ± 0.0012 | BOSS DR11 | Font-Ribera et al. (2014) |
z . | |$d_V \pm \sigma _{d_V}$| . | Survey . | Reference . |
---|---|---|---|
0.106 | 0.336 ± 0.015 | 6dFGS | Beutler et al. (2011) |
0.15 | 0.2239 ± 0.0084 | SDSS DR7 | Ross et al. (2015) |
0.32 | 0.1181 ± 0.0023 | BOSS DR11 | Anderson et al. (2014) |
0.57 | 0.0726 ± 0.0007 | BOSS DR11 | Anderson et al. (2014) |
2.34 | 0.0320 ± 0.0016 | BOSS DR11 | Delubac et al. (2015) |
2.36 | 0.0329 ± 0.0012 | BOSS DR11 | Font-Ribera et al. (2014) |
z . | |$d_V \pm \sigma _{d_V}$| . | Survey . | Reference . |
---|---|---|---|
0.106 | 0.336 ± 0.015 | 6dFGS | Beutler et al. (2011) |
0.15 | 0.2239 ± 0.0084 | SDSS DR7 | Ross et al. (2015) |
0.32 | 0.1181 ± 0.0023 | BOSS DR11 | Anderson et al. (2014) |
0.57 | 0.0726 ± 0.0007 | BOSS DR11 | Anderson et al. (2014) |
2.34 | 0.0320 ± 0.0016 | BOSS DR11 | Delubac et al. (2015) |
2.36 | 0.0329 ± 0.0012 | BOSS DR11 | Font-Ribera et al. (2014) |