-
PDF
- Split View
-
Views
-
Cite
Cite
Dana Yelin, Neta Shirin, Itai Harris, Yovel Peretz, Dafna Yahav, Eli Schwartz, Eyal Leshem, Ili Margalit, Performance of ChatGPT-4o in the diagnostic workup of fever among returning travellers requiring hospitalization: a validation study, Journal of Travel Medicine, 2025;, taaf005, https://doi.org/10.1093/jtm/taaf005
- Share Icon Share
Abstract
Febrile illness in returned travellers presents a diagnostic challenge in non-endemic settings. Chat generative pretrained transformer (ChatGPT) has the potential to assist in medical tasks, yet its diagnostic performance in clinical settings has rarely been evaluated. We conducted a validation assessment of ChatGPT-4o’s performance in the workup of fever in returning travellers.
We retrieved the medical records of returning travellers hospitalized with fever during 2009–2024. Their clinical scenarios at time of presentation to the emergency department were prompted to ChatGPT-4o, using a detailed uniform format. The model was further prompted with four consistent questions concerning the differential diagnosis and recommended workup. To avoid training, we kept the model blinded to the final diagnosis. Our primary outcome was ChatGPT-4o’s success rates in predicting the final diagnosis when requested to specify the top three differential diagnoses. Secondary outcomes were success rates when prompted to specify the single most likely diagnosis, and all necessary diagnostics. We also assessed ChatGPT-4o as a predicting tool for malaria and qualitatively evaluated its failures.
ChatGPT-4o predicted the final diagnosis in 68% [95% confidence interval (CI) 59–77%], 78% (95% CI 69–85%) and 83% (95% CI 74–89%) of the 114 cases, when prompted to specify the most likely diagnosis, top three diagnoses and all possible diagnoses, respectively. ChatGPT-4o showed a sensitivity of 100% (95% CI 93–100%) and a specificity of 94% (95% CI 85–98%) for predicting malaria. The model failed to provide the final diagnosis in 18% (20/114) of cases, primarily by failing to predict globally endemic infections (16/21, 76%).
ChatGPT-4o demonstrated high diagnostic accuracy when prompted with real-life scenarios of febrile returning travellers presenting to the emergency department, especially for malaria. Model training is expected to yield an improved performance and facilitate diagnostic decision-making in the field.
Introduction
Chat generative pretrained transformer (ChatGPT) is an artificial intelligence (AI)-derived chatbot developed by OpenAI.1 As a large language model, GPT was trained by its operators for general-purpose cognitive capabilities in both closed- and open-domain contexts.2 ChatGPT-4o, the web-based chatbot platform implementing of the GPT-4o model, is accessible for public use since May 2024 at https://chat.openai.com/.3
Although not specifically trained for medical purposes, GPT shows varying degrees of competence in the field. The frequently published literature on its medical applications demonstrates that GPT possesses the capability to retain medical knowledge, including in infectious diseases,4 solve medical board and licencing examinations,5,6 assist in medical note taking,7 provide some degree of medical consultation,8,9 and aid managing medical data for research10 and clinical purposes.11
In travel medicine, ChatGPT-generated pre-travel advice was comparable to travel health guidance recommendations, although responses were generic and failed to consider the individual travel itinerary and medical history.12 Accordingly, encouraging patients to consult generative AI-derived chatbots prior to their travel medicine clinic visit may efficiently facilitate a more personalized travel health consultation,13,14 and assist in managing health issues whilst travelling abroad.15 However, currently there are no generative AI tools for diagnostic management of febrile returning travellers. Considering tropical illnesses among the differential diagnosis in non-endemic regions might be challenging for nonexpert physicians.16 Misdiagnosis of travel-related conditions can have severe and even fatal consequences, particularly with Plasmodium falciparum malaria, one of the most frequent infections among travellers to tropical Africa.17 Several computerized tools were developed to facilitate the diagnostic management of returning travellers.18 Nonetheless, AI-models have the potential to personalize the diagnostic approach.
In this study we aimed to assess the utility of ChatGPT-4o in the diagnostic workup of febrile returning travellers, based on the data gathered in their initial assessment at our institutional emergency department (ED).
Methods
Study design and population
We conducted a preliminary validation study of ChatGPT-4o’s performance using the medical records of febrile travellers who returned to Israel and were admitted to Sheba Medical Center between 2009 and 2024. Eligible cases included adults (≥18 years) with fever (>38°C; either reported or measured upon admission), and a final laboratory-confirmed infectious diagnosis. We screened medical charts consecutively to identify cases that met these criteria. Only travellers who had crossed international borders and returned from outside the World Health Organization (WHO) Eastern Mediterranean region were included. We excluded cases for whom necessary information (see ‘study variables of interest’ below) was missing and non-residents (tourists visiting Israel, asylum seekers or immigrants).
Study variables of interest
We extracted information on the following variables, as recorded in the electronic medical records: demographics, comorbidities and chronic pharmacotherapy; pre-travel medical encounter: recommendations and their actual implementation (vaccination, malaria prophylaxis); type of travel (recreation, business, etc.); travel destinations, dates, sites, activities and exposures; disease presentation: symptoms, date of first symptom, date of arrival to the ED, vital signs, physical examination findings and laboratory parameters upon arrival to the ED; diagnostic workup throughout hospitalization and final laboratory-confirmed diagnosis. Further in-depth details are provided as Supplementary material.
Trial implementation
The trial was conducted during 7–11 September 2024, using ChatGPT-4o. Each patient in the cohort was treated as a single case study and was randomly assigned to one of three independent researchers, who prompted it to ChatGPT-4o using a uniform text format. The format (Supplementary material) contained a structured frame allowing variation in individual patient data only [patients’ medical background, travel purpose and itinerary, adherence to pre-travel recommendations given in a travel clinic visit, travel activities and exposures, illness presentation (symptoms) and findings in the ER (vital signs, physical examination and laboratory results)]. To refrain from possible training of the system, we deliberately chose not to provide ChatGPT-4o with feedback concerning its responses. Moreover, to diminish unblinding resulting from cross-check of cases published as peer-reviewed case-reports or in popular journalism, we systematically altered patients’ age by an addition of 1 year. Following each case presentation, we prompted the model with four uniform questions (Table 1). For each case, we collected the model responses (see examples in the Supplementary material) and compared them to the final diagnosis, considered as the gold standard.
Question #1 | What is the most likely diagnosis? (please specify one diagnosis) |
Question #2 | What are the three most likely diagnoses? |
Question #3 | What are the three most important diagnostic tests recommended to reach a final diagnosis in this case? |
Question #4 | Disregarding the previous questions, if you were not limited by the number of tests, what diagnostic tests would you recommend, considering only those tests that are necessary to confirm or rule out relevant diagnoses in this scenario? |
Question #1 | What is the most likely diagnosis? (please specify one diagnosis) |
Question #2 | What are the three most likely diagnoses? |
Question #3 | What are the three most important diagnostic tests recommended to reach a final diagnosis in this case? |
Question #4 | Disregarding the previous questions, if you were not limited by the number of tests, what diagnostic tests would you recommend, considering only those tests that are necessary to confirm or rule out relevant diagnoses in this scenario? |
aUniformly prompted to ChatGPT-4o immediately after prompting each case presentation.
Question #1 | What is the most likely diagnosis? (please specify one diagnosis) |
Question #2 | What are the three most likely diagnoses? |
Question #3 | What are the three most important diagnostic tests recommended to reach a final diagnosis in this case? |
Question #4 | Disregarding the previous questions, if you were not limited by the number of tests, what diagnostic tests would you recommend, considering only those tests that are necessary to confirm or rule out relevant diagnoses in this scenario? |
Question #1 | What is the most likely diagnosis? (please specify one diagnosis) |
Question #2 | What are the three most likely diagnoses? |
Question #3 | What are the three most important diagnostic tests recommended to reach a final diagnosis in this case? |
Question #4 | Disregarding the previous questions, if you were not limited by the number of tests, what diagnostic tests would you recommend, considering only those tests that are necessary to confirm or rule out relevant diagnoses in this scenario? |
aUniformly prompted to ChatGPT-4o immediately after prompting each case presentation.
Outcomes and assessment
Our primary outcome was the success rate (proportion of correct responses) of ChatGPT-4o to predict the final diagnosis when requested to specify the top three differential diagnoses (question #2). We defined success as an inclusion of the final diagnosis within the top three differential diagnoses suggested by the model. The secondary outcomes included the model’s success rates in predicting the final diagnosis when requested to specify the single most likely diagnosis (question #1) and in recommending a diagnostic test that could confirm the final diagnosis when prompted for unrestricted test recommendations (question #4). Additionally, we assessed the model’s diagnostic accuracy measures as a prediction tool for malaria and qualitatively evaluated its failures in successfully predicting the final diagnosis. Assessment of question #3 results is beyond the scope of the current analysis. For each outcome, we defined ChatGPT-4o’s failure rate as the proportion of incorrect responses.
Sample size calculation
We estimated that in similar scenarios, an expert in tropical medicine would successfully predict the final diagnosis at an assumed rate of 75% of cases if requested to provide the 3 top differential diagnoses. ChatGPT’s diagnostic performance was tested in other fields, demonstrating variable results, with a sensitivity reaching 100%.19 Accordingly, based on an assumed success rate of 85% for the primary outcome, with a required statistical power of 80% and a significance level of 0.05, we determined a sample size of 133 individuals. As we expected non-inclusion of ~ 40% of cases, we strived to sample 221 cases.
Statistical analysis
We calculated the ChatGPT-4o success rates as the proportion of cases for which the outcome was achieved out of all cases and used binomial exact calculation to generate the 95% confidence intervals (CIs) for each proportion. For the assessment of ChatGPT-4o as a diagnostic tool for malaria we drew 2 × 2 tables and calculated the sensitivity, specificity, Kappa index and area under the receiver-operator curve (AUROC). Comparison of the number of diagnostic tests recommended by the model to that ordered by physicians was facilitated using the Wilcoxon signed-rank test.
Ethical considerations
The study was approved by the Institutional Review Board at Sheba Medical Center. An exemption from informed consent was granted because of the de-identified, retrospective design of the study.
Results
Study population
During 2009–2024, a total of 236 febrile returning travellers were hospitalized. We reviewed their medical charts and omitted 122 (52%) individuals who met the exclusion criteria (Figure 1). The trial’s cohort consisted of the remaining 114 individuals. The median age was 38 (IQR 27, 52) years and 27 (24%) were women. Most of them (66%) were generally healthy. Among those with comorbidities, essential hypertension and dyslipidemia were the most frequent (Table S1, Supplementary material).
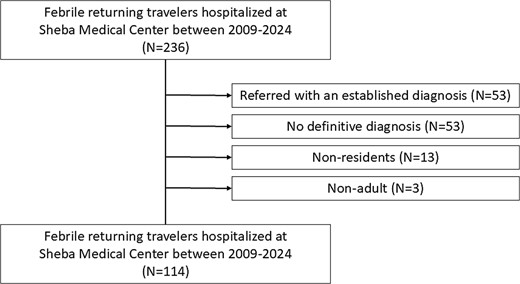
Of the 114 individuals included in the cohort, 111 (97%) travelled to a single WHO region. The most frequent travel destination was the African region (N = 63, 57%), followed by Southeast Asian region (N = 21, 19%), Western Pacific region (N = 16, 14%), region of the Americas (N = 10, 9%) and the European region (N = 1, 1%). The remaining three individuals travelled to more than a single region: the Southeast Asian and the Western Pacific regions (N = 2) and the European and African regions (N = 1).
A total of 54 (47%) individuals travelled for recreation purposes, 24 (44%) of whom as backpackers; 45 (39%) individuals travelled for business purposes, and 11 (10%) were expatriates living in Sub-Saharan Africa; 3 (3%) individuals visited friends and relatives; and one (1%) individual travelled for education and volunteering purposes.
The most frequent diagnosis of the cohort population was malaria (N = 48, 42%; caused by P. falciparum [N = 42; 88%], Plasmodium ovale [N = 3; 6%], Plasmodium vivax [N = 2; 4%] and Plasmodium malariae [N = 1; 2%]), followed by dengue (N = 19, 17%). Additional frequent diagnoses included influenza (N = 8, 7%), leptospirosis (N = 7, 6%), typhoid fever (N = 6, 5%), acute schistosomiasis (N = 5, 4%; one of these patients presented with neuro-schistosomiasis), Rickettsial infection (N = 4, 4%), Cytomegalovirus infection (N = 4, 4%) and infectious mononucleosis (IMN) (N = 3, 3%). An additional eight diagnoses, each appearing only once in the cohort were disseminated gonococcal infection (DGI), Respiratory Syncytial Virus (RSV), histoplasmosis, acute human immunodeficiency virus (HIV) infection, acute hepatitis A virus (HAV) infection, acute Q fever, bacterial pneumonia and cellulitis caused by Streptococcus pyogenes.
Assessment of ChatGPT-4o’s performance
The performance of ChatGPT-4o is presented in Table 2. For the primary outcome (question #2), predicting the final diagnosis when prompted to provide the top 3 main differential diagnoses, ChatGPT-4o had a success rate of 78% (95% CI 69–85%). For the secondary outcomes, ChatGPT-4o achieved somewhat lower success rates. In predicting the final diagnosis when requested to provide the most likely diagnosis (question #1), ChatGPT-4o showed a success rate of 68% (95% CI 59–77%). When prompted to recommend unrestricted diagnostic tests, (question #4), ChatGPT-4o would have diagnosed an additional 5 cases, leading to an overall success rate of 83% (95% CI 74–89%) at time of presentation to the healthcare system (Table 2).
ChatGPT-4o performance in predicting the final diagnosis of febrile returning travellers presented to the emergency department at Sheba Medical Centre during 2009–2024 (N = 114)
. | ChatGPT-4o correct hits . | |
---|---|---|
. | N . | % (95% CI) . |
Primary outcome: | ||
Success rate in predicting the final diagnosis when requested to specify the top three differentials | ||
All cases (N = 114) | 89 | 78.1 (69.4, 85.3) |
Only malaria cases (N = 48) | 47 | 97.9 (88.9, 100) |
Only dengue cases (N = 19) | 18 | 94.7 (74.0, 99.9) |
Secondary outcomes: | ||
Success rate in predicting the final diagnosis when requested to specify the single most likely diagnosis | ||
All cases (N = 114) | 78 | 68.4 (59.1, 76.8) |
Only malaria cases (N = 48) | 43 | 89.6 (77.3, 96.5) |
Only dengue cases (N = 19) | 16 | 84.2 (60.4, 96.6) |
Success rate in recommending a diagnostic test that could confirm the final diagnosis | ||
All cases (N = 114) | 94 | 82.5 (74.2, 88.9) |
Only malaria cases (N = 48) | 48 | 100 (93–100) |
Only dengue cases (N = 19) | 18 | 94.7 (74.0, 99.9) |
. | ChatGPT-4o correct hits . | |
---|---|---|
. | N . | % (95% CI) . |
Primary outcome: | ||
Success rate in predicting the final diagnosis when requested to specify the top three differentials | ||
All cases (N = 114) | 89 | 78.1 (69.4, 85.3) |
Only malaria cases (N = 48) | 47 | 97.9 (88.9, 100) |
Only dengue cases (N = 19) | 18 | 94.7 (74.0, 99.9) |
Secondary outcomes: | ||
Success rate in predicting the final diagnosis when requested to specify the single most likely diagnosis | ||
All cases (N = 114) | 78 | 68.4 (59.1, 76.8) |
Only malaria cases (N = 48) | 43 | 89.6 (77.3, 96.5) |
Only dengue cases (N = 19) | 16 | 84.2 (60.4, 96.6) |
Success rate in recommending a diagnostic test that could confirm the final diagnosis | ||
All cases (N = 114) | 94 | 82.5 (74.2, 88.9) |
Only malaria cases (N = 48) | 48 | 100 (93–100) |
Only dengue cases (N = 19) | 18 | 94.7 (74.0, 99.9) |
ChatGPT-4o performance in predicting the final diagnosis of febrile returning travellers presented to the emergency department at Sheba Medical Centre during 2009–2024 (N = 114)
. | ChatGPT-4o correct hits . | |
---|---|---|
. | N . | % (95% CI) . |
Primary outcome: | ||
Success rate in predicting the final diagnosis when requested to specify the top three differentials | ||
All cases (N = 114) | 89 | 78.1 (69.4, 85.3) |
Only malaria cases (N = 48) | 47 | 97.9 (88.9, 100) |
Only dengue cases (N = 19) | 18 | 94.7 (74.0, 99.9) |
Secondary outcomes: | ||
Success rate in predicting the final diagnosis when requested to specify the single most likely diagnosis | ||
All cases (N = 114) | 78 | 68.4 (59.1, 76.8) |
Only malaria cases (N = 48) | 43 | 89.6 (77.3, 96.5) |
Only dengue cases (N = 19) | 16 | 84.2 (60.4, 96.6) |
Success rate in recommending a diagnostic test that could confirm the final diagnosis | ||
All cases (N = 114) | 94 | 82.5 (74.2, 88.9) |
Only malaria cases (N = 48) | 48 | 100 (93–100) |
Only dengue cases (N = 19) | 18 | 94.7 (74.0, 99.9) |
. | ChatGPT-4o correct hits . | |
---|---|---|
. | N . | % (95% CI) . |
Primary outcome: | ||
Success rate in predicting the final diagnosis when requested to specify the top three differentials | ||
All cases (N = 114) | 89 | 78.1 (69.4, 85.3) |
Only malaria cases (N = 48) | 47 | 97.9 (88.9, 100) |
Only dengue cases (N = 19) | 18 | 94.7 (74.0, 99.9) |
Secondary outcomes: | ||
Success rate in predicting the final diagnosis when requested to specify the single most likely diagnosis | ||
All cases (N = 114) | 78 | 68.4 (59.1, 76.8) |
Only malaria cases (N = 48) | 43 | 89.6 (77.3, 96.5) |
Only dengue cases (N = 19) | 16 | 84.2 (60.4, 96.6) |
Success rate in recommending a diagnostic test that could confirm the final diagnosis | ||
All cases (N = 114) | 94 | 82.5 (74.2, 88.9) |
Only malaria cases (N = 48) | 48 | 100 (93–100) |
Only dengue cases (N = 19) | 18 | 94.7 (74.0, 99.9) |
ChatGPT-4o’s failure rate was 18% (20 cases). Among these, there were three cases requiring immediate antibiotic therapy (two cases of typhoid fever and one case of DGI). Sixteen (80%) of these cases were globally endemic infections, i.e. cosmopolitan infections (influenza, Cytomegalovirus infection, IMN, RSV infection, DGI and acute Q fever). Among these, all seven cases of Cytomegalovirus infection and IMN, and 75% (6/8) cases of influenza were missed (Table S2, Supplementary material). Determining whether these infections were acquired prior, during, or after the trip was usually impossible (Table S3, Supplementary material). In all, the model succeeded in predicting the final diagnosis only in 24% (5/21) of cosmopolitan infections. ChatGPT-4o also failed in diagnosing two cases of arboviruses (one case of dengue and one case of Chikungunya virus infection) (Figure 2).
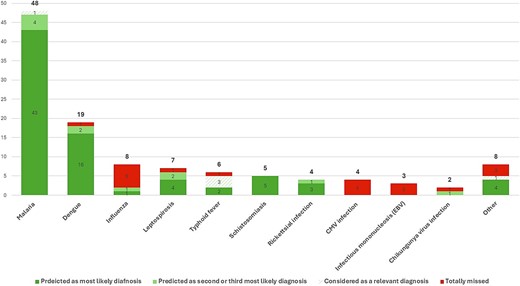
Distribution of diagnoses within the cohort (N = 114). The category of other diagnoses includes diagnoses appearing in the cohort only once: histoplasmosis, acute Human Immunodeficiency Virus infection, acute Hepatitis A Virus infection, and cellulitis caused by Streptococcus pyogenes were suggested by the model as the most likely diagnosis; bacterial pneumonia would have been diagnosed through an unrestricted workup; ChatGPT-4o failed in diagnosing disseminated gonococcal infection, Respiratory Syncytial Virus infection, and acute Q fever were
Assessment of ChatGPT-4o’s performance for malaria and dengue
The model achieved 98% (95% CI 89–100%) and 95% (95% CI 74–100%) success rates for malaria and dengue, respectively, in the top three differential diagnoses (Table 2).
For malaria, we further assessed ChatGPT-4o‘s diagnostic accuracy in predicting malaria as the most likely diagnosis at time of presentation to the ED (secondary outcome, question #1). This has resulted in a sensitivity of 90% (95% CI 78–96%) and a specificity of 94% (95% CI 85–98%), with an AUROC of 92% (95% CI 87–97%) and a Kappa index of 0.84. Out of the five failures of diagnosing malaria as the most likely diagnosis (Table 3), four cases were suggested by the model as the second most likely diagnosis. For the remaining case, ChatGPT-4o still recommended malaria testing when it was requested to specify all necessary diagnostic tests, even though malaria was not considered among the top three diagnoses. Accordingly, the sensitivity for malaria diagnosis was 100% (95% CI 93–100%), with a specificity of 94% (95% CI 85–98%), an AUROC of 97% (95% CI 94–100%), and a Kappa index of 0.93. Our cohort included 6 non-falciparum malaria cases (Supplementary Table S4).
Qualitative assessment of ChatGPT-4o failures
ChatGPT-4o tended to fall for red herrings, particularly those concerning typical exposures. For instance, histoplasmosis was suggested as the most likely diagnosis in a case where the patient returning from Guatemala reported strolling in a cave full of bats, whilst the final diagnosis of leptospirosis was suggested as the second most likely diagnosis. Similarly, whenever a patient reported unprotected sexual exposures, or non-adherence to antimalarial prophylaxis, the system tended to suggest sexually transmitted diseases or malaria, respectively, as the most likely diagnoses, even though the clinical presentation was atypical. Re-prompting these cases a few weeks later without mentioning the unique exposures, has led to an omission of these misled diagnoses from the differential.
Furthermore, ChatGPT-4o occasionally recommended inappropriate diagnostic tests. These included viral serologies for respiratory viruses, and interferon-Gamma release assay for suspected disseminated tuberculosis. Infrequently, it also produced general recommendations carrying no practical value, e.g. ‘serologic tests for other tropical infections.’
ChatGPT-4o’s diagnostic accuracy for malaria among febrile returning travellers presented to the emergency department at Sheba Medical Center during 2009–2024 (N = 114): 2 × 2 tables
. | ChatGPT-4o . | ||
---|---|---|---|
Yes . | No . | ||
Success rate in predicting malaria as the most likely diagnosis | |||
Gold Standard | Yes | 43 | 5a |
No | 4b | 62 | |
Success rate in predicting malaria through listing the differential diagnosis relevant for the case | ChatGPT-4o | ||
Yes | No | ||
Gold Standard | Yes | 48 | 0 |
No | 4 | 62 |
. | ChatGPT-4o . | ||
---|---|---|---|
Yes . | No . | ||
Success rate in predicting malaria as the most likely diagnosis | |||
Gold Standard | Yes | 43 | 5a |
No | 4b | 62 | |
Success rate in predicting malaria through listing the differential diagnosis relevant for the case | ChatGPT-4o | ||
Yes | No | ||
Gold Standard | Yes | 48 | 0 |
No | 4 | 62 |
aCaused by P. falciparum (N = 4) and P. vivax (N = 1); 4 cases, caused by P. falciparum (N = 3) and P. vivax (N = 1), were suggested by the model as the second most likely diagnosis. For the remaining case, caused by P. falciparum, ChatGPT-4o still recommended malaria testing when it was requested to specify all necessary diagnostic tests, even though malaria was not considered among the top three diagnoses
bAll were febrile returning travellers arriving from malaria endemic regions [Tanzania (N = 2); Ethiopia (N = 1), India (N = 1)].
ChatGPT-4o’s diagnostic accuracy for malaria among febrile returning travellers presented to the emergency department at Sheba Medical Center during 2009–2024 (N = 114): 2 × 2 tables
. | ChatGPT-4o . | ||
---|---|---|---|
Yes . | No . | ||
Success rate in predicting malaria as the most likely diagnosis | |||
Gold Standard | Yes | 43 | 5a |
No | 4b | 62 | |
Success rate in predicting malaria through listing the differential diagnosis relevant for the case | ChatGPT-4o | ||
Yes | No | ||
Gold Standard | Yes | 48 | 0 |
No | 4 | 62 |
. | ChatGPT-4o . | ||
---|---|---|---|
Yes . | No . | ||
Success rate in predicting malaria as the most likely diagnosis | |||
Gold Standard | Yes | 43 | 5a |
No | 4b | 62 | |
Success rate in predicting malaria through listing the differential diagnosis relevant for the case | ChatGPT-4o | ||
Yes | No | ||
Gold Standard | Yes | 48 | 0 |
No | 4 | 62 |
aCaused by P. falciparum (N = 4) and P. vivax (N = 1); 4 cases, caused by P. falciparum (N = 3) and P. vivax (N = 1), were suggested by the model as the second most likely diagnosis. For the remaining case, caused by P. falciparum, ChatGPT-4o still recommended malaria testing when it was requested to specify all necessary diagnostic tests, even though malaria was not considered among the top three diagnoses
bAll were febrile returning travellers arriving from malaria endemic regions [Tanzania (N = 2); Ethiopia (N = 1), India (N = 1)].
Discussion
In diagnosing the febrile retuning traveller at the time of the initial medical presentation, ChatGPT-4o exhibited a substantial diagnostic ability in our cohort, achieving success rates of 78% and 68% for predicting the final diagnosis (top three and most likely, respectively). Importantly, regarding malaria, arguably the most life-threatening, treatable condition in returning travellers, ChatGPT-4o would not have missed a single case. This suggests that using ChatGPT-4o as a decision support tool for ordering malaria diagnostics in the ED might improve primary visit detection rate and decrease overall time from presentation to appropriate treatment, thereby improving outcomes.18 Leire Balerdi-Sarasola et al. have recently reported their MALrisk tool, a machine-learning-based model with 100% sensitivity and 72% specificity for predicting malaria among febrile returning travellers.20 Our findings pointed out a similar sensitivity (100%) and a greater specificity (94%) for GPT-4o, even though it was not specifically trained for predicting malaria. This emphasizes GPT-4o’s tremendous potential if appropriately trained. However, its performance in other regards is currently far from acceptable for incorporating it for clinical decision support.
Our study demonstrated that GPT-4o failed to consider the final diagnosis among the differentials in a substantial proportion of cases. Prominently, it disregarded globally endemic (cosmopolitan) infections such as those caused by Cytomegalovirus, EBV, IMN and influenza, narrowing the differential diagnoses in the context of travel medicine. Moreover, GPT-4o occasionally lacked clinical reasoning and was deceived by focusing on a single typical exposure. Whilst AI has the potential to surmount human cognitive biases and flaws, once incorporated into routine clinical workflow, healthcare professionals tend to over-depend on automated systems.21,22 Accordingly, overreliance on AI may lead to misdiagnosis, misuse of diagnostics and deterioration of the clinical competence of healthcare professionals in the long-term. Nonetheless, appropriate context training combined with reinforcement through human feedback23 supervised by experts in the field, is expected to improve GPT-4o’s diagnostic capabilities, potentially overtaking human skills. Large patient-level databases are required to facilitate optimal training.24 Moreover, we did not compare ChatGPT-4o’s performance with that of physicians, leaving the question of who performs better yet to be investigated.
Among returning travellers, fever is a leading cause for pursuing medical assistance, frequently leading to hospitalization. Among 784 Americans travelling abroad for up to 3 months, febrile episodes were documented in 3%.25 Out of 24 920 returning travellers seeking medical care, 28% described fever as their chief reason.26 Among 211 returning travellers requiring hospitalization in Israel, febrile illnesses accounted for 77% of admissions.27 Considering the increase in international tourism in the post-COVID-19 pandemic,28 numbers of febrile returning travellers are expected to rise. In many cases, the physicians initially treating these returning travellers in their home country lack experience with tropical illnesses and are unfamiliar with the necessary diagnostic workup involved in such differential diagnoses. The urgency to rule out malaria, a life-threatening though treatable condition, should be facilitated through a risk-based approach taking together the patients’ exposures (geographical regions, activities and use of prophylactic measures) and presentation.18 This approach might be challenging for a non-tropical medicine expert who is inexperienced with these illnesses. Considering the relative paucity of tropical medicine experts in non-tropical countries, well-trained AI-derived chatbots have the potential to contribute to the management of these patients. Accordingly, further studies on the performance of models specifically trained in travel health and tropical medicine are warranted to determine whether this potential translates into practical, real-world effectiveness.
Our study had several limitations. Primarily, as we did not train the model, our study did not evaluate GPT-4o’s full potential. Further training is expected to improve the overall performance of the model. Appropriate training requires big data input accompanied by reinforcement learning with human feedback.23 This cannot be carried out by an end user. In addition, given that the clinical scenarios were collected retrospectively from patients’ charts, differential reporting bias is likely, particularly regarding exposures that were highly suggestive of the final diagnosis. Yet, this bias was largely mitigated by deliberate extraction of information gathered at the time of the initial presentation to the ED. Additionally, our sample size calculation was based on an assumed success rate of 85%, requiring a cohort of 133 travellers. However, the final sample consisted of 114 cases, and the observed success rate was 78%, implying the study was underpowered. Finally, the external validity of our study was somewhat diminished in a few aspects. First, our usage of a single prompt format was not compared to alternative formats. Moreover, consistent prompts do not reflect real-life consultations with the model, as style and focus changes between physicians; Second, to keep the population of this validation study homogenous, we included only Israeli residents. Accordingly, migrant workers, individuals visiting friends and relatives, and asylum seekers were underrepresented; Third, our cohort comprised only individuals requiring hospitalization; Lastly, the short interval cutoff (≤30 days) used for inclusion, limited the capture of diseases with long incubation periods.
In conclusion, ChatGPT-4o has the potential to improve the diagnostic workup of fever among returning travellers seeking medical attention, particularly in deciding whether malaria diagnostics should be ordered. Nonetheless, further training of the model, particularly regarding cosmopolitan illnesses, alongside reinforcement learning and feedback will likely enhance its performance to meet the standards required for clinical use.
Funding
This report was prepared with no specific funding.
Author contributions
Dana Yelin (Conceptualization, Methodology, Investigation, Writing—Original draft), Neta Shirin (Investigation), Itai Harris (Investigation), Yovel Peretz (Data curation), Dafna Yahav (Writing—Review & editing), Eli Schwartz (Writing—Review & editing), Eyal Leshem (Methodology, Writing—Review & editing) and Ili Margalit (Conceptualization, Methodology, Investigation, Formal analysis, Writing—original draft).
Conflict of interest
Eyal Leshem reports honoraria from Novartis, Sanofi Pasteur, and GSK, consulting fees from Sanofi Pasteur, and Moderna, and consulting World Health Organization and CDC foundation outside the submitted work. All other authors declare no conflict of interest.
Usage of generative artificial intelligence
The authors declare that they have used generative artificial intelligence (ChatGPT-4o) for performing the trial. However, the authors declare that they have not been using any generative artificial intelligence for data analysis, table and figure creation, or manuscript writing.
Financial support
None.
This study was presented in a workshop conducted at the 14th Asia Pacific Travel Health Conference (APTHC 2024), Kathmandu, Nepal, 20 September 2024.