-
PDF
- Split View
-
Views
-
Cite
Cite
Emilie Dedeban, Quinn M R Webber, Michel P Laforge, Alec L Robitaille, Eric Vander Wal, Uncertainty during migration: the role of predictable resources in unpredictable conditions in determining movement in Woodland Caribou (Rangifer tarandus), Journal of Mammalogy, Volume 104, Issue 6, December 2023, Pages 1290–1301, https://doi.org/10.1093/jmammal/gyad081
- Share Icon Share
Abstract
Environmental and climatic variation drive animal migration. Animals must adjust their behavioral strategies, for example, habitat selection, to match best variation in resources whose value likely varies with conditions such as weather. For example, climate change makes processes such as snowmelt and the emergence of vegetation less predictable at the scale of months or weeks. Furthermore, climate change makes meteorological conditions unpredictable—or dynamic—at the scale of days and hours. The profitability of selecting any particular resource may vary according to local meteorological condition. We studied the impact of dynamic weather conditions on fine-scale movement strategies and resource selection during spring migration of adult female Woodland Caribou (Rangifer tarandus). We assessed the role played by static resources such as open, lichen, and closed forest habitats and their role in a context-dependent of dynamic weather conditions, including temperature, precipitation, and changing snow water equivalent. We tested the role of static resources and dynamic conditions in three contexts: (1) whether caribou were encamped or moving using hidden Markov models; state-specific habitat selection for, and the interaction between, resources and conditions while (2) encamped and (3) moving. Weather conditions influenced the probability of moving or staying encamped, and the probability of selecting for a resource, which likely reflects the profitability of a given resource in a particular condition. The probability of staying encamped in the forest increases as temperature increases, and the probability of selecting an open area is higher when precipitation is lower. We highlight how meteorological conditions modify the selection of a static resource and likely the profitability of a given resource. The resource utility to a consumer is increasingly susceptible to climate change-induced effects.
Climate change may exacerbate variation in spatially and temporally heterogeneous environments (Sharma et al. 2009; Pearson et al. 2013). For example, varying temperatures, precipitation, snow dynamics, sea ice cover, fire regimes, and vegetative growth lead to unpredictable variations to which animals must acclimate (Pearson et al. 2013; Descamps et al. 2017). One way that animals can acclimate to variable environments is by adjusting their movement. Movement allows animals to match their patterns of space use to dynamic resources, and cope with unpredictable local conditions, such as weather (Kays et al. 2015). Among movements, migration may be particularly susceptible to disruption from climate change as migration is primarily synchronized to predictable warm–cold seasonal variations of the annual cycle (Dingle and Drake 2007). Some species face strong seasonal environmental variation forcing them to modify movement, for example, through unexpected or extended stopovers during migration (Klaassen et al. 2012). Stopover sites are defined as areas where migrants can accumulate energy reserves necessary to complete the migration or movement to the next stopover site, whereas the function of movement corridors is to facilitate movement between stopover sites (Hedenström 2003).
Seasonal variation in temperature or precipitation can further lead to earlier snowmelt and modification in plant phenology (Ward et al. 2016; Gurarie et al. 2019). Altered plant phenology can subsequently influence the migratory timing (Aikens et al. 2020) and the fine-scale movement of animals during migration. Climate affects the accessibility of some resources within habitats, and their value to animals may be further altered by weather conditions. The quality of stopover sites may vary according to unpredictable factors such as climatic variation. By adjusting fine-scale movement during migration, for example, extending or shortening stopover events, animals can buffer the potential deleterious effects of local variability in weather conditions (Brown et al. 2021).
In unpredictable environments, animals respond rapidly when conditions change in order to increase energy gain and minimize energy loss. For migratory movements, selecting high-quality stopover sites is one way in which animals can balance energy trade-offs (Sawyer and Kauffman 2011). The choice that animals make to either stop or continue moving depends on local resource availability, which can vary in space and time (Mueller and Fagan 2008). Climate is changing weather and has a persistent directional change. For example, in unpredictable conditions including heavy rain, snow, or ice storm events, migrants may experience impediments to physical accessibility that modifies the value of resources (Tyler 2010). For terrestrial mammals, snow may increase costs of locomotion because energy expenditure increases with increasing snow depth (Fancy and White 1987). Variation in climate can also produce the opposite effect of earlier warm periods in spring. Early warming periods can result in earlier snowmelt, and thus increase the emergence of vegetation in spring. Migration is a relatively flexible behavior and migrants can cope with climatic unpredictability by shifting their departure date, increasing movement rate during migration, or reducing the duration of stopovers to time arrival at the summer grounds with the peak of primary productivity (Weber et al. 1998; Ward et al. 2016; Gurarie et al. 2019).
Resource dynamics also drive animal movements causing animals to move according to temporal and spatial gradients of predictable or unpredictable factors that depend on conditions (Singh et al. 2012). For example, herbivores rely on vegetation, the profitability of which differs if animals need to dig through snow to access plants underneath. Thus, condition-dependent factors, such as snow depth, interact with food resources and modify their value. Resource availability for migrating animals can therefore be unpredictable due to changing weather state, which can be considered as a proximal factor. For example, heavy rainfall or high temperatures may change the perception of the quality of a given resource. Condition-dependent modifications amplify for migratory species that often follow phenological events at the intersection between seasons (Leclerc et al. 2019; Rivrud et al. 2019). The role of fine-scale movement within a migratory period may reveal that weather can modify the use of resources and thus also influence the duration of migration. Habitat selection of migrating animals, including during stopovers and during movement between stopover sites, relate to resources and weather conditions. Indeed, although environments are often spatially heterogeneous, they tend to be predictable spatially and temporally and it should be advantageous for an individual to remember the locations of the most profitable resource patches along a migration route (Merkle et al. 2019; Robira et al. 2021).
Animal movement in general, and migratory behavior in particular, pose major conservation challenges (Sawyer et al. 2009; Kauffman et al. 2021a). Migratory ungulates exhibit plasticity in migratory behavior between and within species (Merkle et al. 2016) and the migratory patterns for many temperate ungulates are vulnerable to change as a result of climate change and the associated changes in local and regional weather state (Le Corre et al. 2016; Gurarie et al. 2019; Leclerc et al. 2021). Here, we examined the role of variation in weather conditions on movement and resource selection during the spring migration of Woodland Caribou (Rangifer tarandus) in Newfoundland, Canada. Caribou in Newfoundland are partially migratory, where some herds display limited migratory behavior and others migrate between distinct winter and calving areas (Laforge et al. 2021; Mahoney and Schaefer 2002). Compared to other Woodland Caribou in Canada (Le Corre et al. 2020) and Barren Ground Caribou in the arctic (Joly et al. 2021), caribou in Newfoundland migrate relatively short distances (mean = 60.8; SD = 12.9; range = 36–88 km; Table 1) over relatively long periods of time (mean = 61.5; SD = 17.6; range = 6–94 days; Table 1). As a result, we expect that movement and habitat selection for caribou in Newfoundland during migration are sensitive to fine-scale variation in weather conditions.
Migration timing, duration (days), total distance (meters), and step length (meters) of Woodland Caribou in Newfoundland during 4 years of monitoring. Mean values ± SD, range in brackets.
. | 2010 (n = 11) . | 2011 (n = 21) . | 2012 (n = 6) . | 2013 (n = 9) . |
---|---|---|---|---|
Departure date (days) | 6 March ± 12.8 (18 February to 4 April) | 1 March ± 15.5 (12 February to 22 April) | 27 February ± 11.6 (16 February to 18 March) | 12 March ± 8.3 (27 February to 8 April |
Arrival date (days) | 2 May ± 14.5 (4 April to 17 May) | 4 May ± 11.4 (13 April to 20 May) | 1 May ± 12.1 (21 March to 16 May) | 26 April ± 6.5 (9 April to 1 May) |
Duration (days) | 56.4 ± 18 (27–88) | 64.5 ± 17.3 (16–94) | 62.5 ± 16.2 (6–73) | 44.6 ± 9.7 (13–63) |
Total migration distance (km) | 56.9 ± 11.4 (39–78) | 57.1 ± 12.5 (36–82) | 73.9 ± 7.7 (56–88) | 65.2 ± 5.8 (48–73) |
Step length: encamped (m) | 223.57 ± 186.6 (2–1,188) | 120.6 ± 156.8 (0–1,562) | 107.2 ± 142.7 (0–1,494) | 135.9 ± 171.6 (0–1,028) |
Step length: movement (m) | 798.5 ± 898.3 (3–6,868) | 735.7 ± 764.1 (1–6,269) | 734.7 ± 737 (2–6,661) | 758 ± 890.3 (1–6,957) |
. | 2010 (n = 11) . | 2011 (n = 21) . | 2012 (n = 6) . | 2013 (n = 9) . |
---|---|---|---|---|
Departure date (days) | 6 March ± 12.8 (18 February to 4 April) | 1 March ± 15.5 (12 February to 22 April) | 27 February ± 11.6 (16 February to 18 March) | 12 March ± 8.3 (27 February to 8 April |
Arrival date (days) | 2 May ± 14.5 (4 April to 17 May) | 4 May ± 11.4 (13 April to 20 May) | 1 May ± 12.1 (21 March to 16 May) | 26 April ± 6.5 (9 April to 1 May) |
Duration (days) | 56.4 ± 18 (27–88) | 64.5 ± 17.3 (16–94) | 62.5 ± 16.2 (6–73) | 44.6 ± 9.7 (13–63) |
Total migration distance (km) | 56.9 ± 11.4 (39–78) | 57.1 ± 12.5 (36–82) | 73.9 ± 7.7 (56–88) | 65.2 ± 5.8 (48–73) |
Step length: encamped (m) | 223.57 ± 186.6 (2–1,188) | 120.6 ± 156.8 (0–1,562) | 107.2 ± 142.7 (0–1,494) | 135.9 ± 171.6 (0–1,028) |
Step length: movement (m) | 798.5 ± 898.3 (3–6,868) | 735.7 ± 764.1 (1–6,269) | 734.7 ± 737 (2–6,661) | 758 ± 890.3 (1–6,957) |
Migration timing, duration (days), total distance (meters), and step length (meters) of Woodland Caribou in Newfoundland during 4 years of monitoring. Mean values ± SD, range in brackets.
. | 2010 (n = 11) . | 2011 (n = 21) . | 2012 (n = 6) . | 2013 (n = 9) . |
---|---|---|---|---|
Departure date (days) | 6 March ± 12.8 (18 February to 4 April) | 1 March ± 15.5 (12 February to 22 April) | 27 February ± 11.6 (16 February to 18 March) | 12 March ± 8.3 (27 February to 8 April |
Arrival date (days) | 2 May ± 14.5 (4 April to 17 May) | 4 May ± 11.4 (13 April to 20 May) | 1 May ± 12.1 (21 March to 16 May) | 26 April ± 6.5 (9 April to 1 May) |
Duration (days) | 56.4 ± 18 (27–88) | 64.5 ± 17.3 (16–94) | 62.5 ± 16.2 (6–73) | 44.6 ± 9.7 (13–63) |
Total migration distance (km) | 56.9 ± 11.4 (39–78) | 57.1 ± 12.5 (36–82) | 73.9 ± 7.7 (56–88) | 65.2 ± 5.8 (48–73) |
Step length: encamped (m) | 223.57 ± 186.6 (2–1,188) | 120.6 ± 156.8 (0–1,562) | 107.2 ± 142.7 (0–1,494) | 135.9 ± 171.6 (0–1,028) |
Step length: movement (m) | 798.5 ± 898.3 (3–6,868) | 735.7 ± 764.1 (1–6,269) | 734.7 ± 737 (2–6,661) | 758 ± 890.3 (1–6,957) |
. | 2010 (n = 11) . | 2011 (n = 21) . | 2012 (n = 6) . | 2013 (n = 9) . |
---|---|---|---|---|
Departure date (days) | 6 March ± 12.8 (18 February to 4 April) | 1 March ± 15.5 (12 February to 22 April) | 27 February ± 11.6 (16 February to 18 March) | 12 March ± 8.3 (27 February to 8 April |
Arrival date (days) | 2 May ± 14.5 (4 April to 17 May) | 4 May ± 11.4 (13 April to 20 May) | 1 May ± 12.1 (21 March to 16 May) | 26 April ± 6.5 (9 April to 1 May) |
Duration (days) | 56.4 ± 18 (27–88) | 64.5 ± 17.3 (16–94) | 62.5 ± 16.2 (6–73) | 44.6 ± 9.7 (13–63) |
Total migration distance (km) | 56.9 ± 11.4 (39–78) | 57.1 ± 12.5 (36–82) | 73.9 ± 7.7 (56–88) | 65.2 ± 5.8 (48–73) |
Step length: encamped (m) | 223.57 ± 186.6 (2–1,188) | 120.6 ± 156.8 (0–1,562) | 107.2 ± 142.7 (0–1,494) | 135.9 ± 171.6 (0–1,028) |
Step length: movement (m) | 798.5 ± 898.3 (3–6,868) | 735.7 ± 764.1 (1–6,269) | 734.7 ± 737 (2–6,661) | 758 ± 890.3 (1–6,957) |
Integrating broad-scale analysis of movement strategies across gradients of dynamic temperature, precipitation, and snow conditions, with fine-scale analyses of resource selection during the migratory period may reconcile previous incongruities in our understanding of animal migration. Using hidden Markov models, we determined when caribou were in one of two behavioral states during the migratory period—encamped (i.e., stopover) or moving. We then used habitat selection analyses to model selection for static resources and dynamic weather conditions during either stopover or movement within the migratory period. Finally, we tested the hypothesis that the interactive effects of selection for static resources and dynamic weather conditions affect variation in stopover behavior and movement between stopover sites (summarized in Table 2). We tested three predictions: (1) given that the function of stopover sites is to help animals refuel during migration, we predicted that the probability of stopover for caribou would be higher in lichen barrens associated with foraging (P1a) and when weather conditions are warmer (increasing temperature), drier (decreasing rainfall), and less snowfall (decreasing snow water equivalent; P2a); (2) during stopover, we predicted that caribou would select open habitats associated with lichen barrens to refuel for subsequent movements between stopover sites (P1b), and that caribou would select open habitats when temperature increases and when precipitation and snowfall decrease because these weather conditions increase the accessibility of resources previously buried under the snow (P3b); and (3) during movements between stopover sites, we predicted that caribou would select open habitats associated with wetlands or rocky barrens as these habitats are likely to facilitate movement (P1c). For additional predictions about the interactions between movement and weather conditions, see Table 2.
Summary of behavioral state and resource selection hypotheses and related predictions.
Hypothesis . | Movement or encamped . | Resource selection during stopover . | Resource selection during movement . | References . |
---|---|---|---|---|
H1: Habitat resources | P1a: Stop: Lichen areas Movement: Mostly in open areas/lichen areas and at lower rate in forest areas | P1b: Selection: Open areas/Lichen areas Avoidance: Forest areas | P1c: Selection: Open areas/Lichen areas Avoidance: Forest areas | (Schaefer et al. 2016; Webber et al. 2022) |
H2: Weather conditions | P2a: Probability to stop increases when temperature increases and precipitation and snow decrease | P2b: Positive selection for areas where weather conditions decrease | P2c: Selection for areas where weather conditions are warmer, wetter, and snowier | (Le Corre et al. 2016; Gurarie et al. 2019; Leclerc et al. 2019, 2021; Bright Ross et al. 2021) |
H3: Static resources × dynamic weather conditions | P3a: Probability to stop in open and lichen areas increases when temperature increases and precipitation and snow decrease. Probability of movement would increase in closed areas when weather conditions are hotter, wetter, and snowier. | P3b: Selection for open and lichen habitat is higher when temperature increases and precipitation and snow decrease. Selection for closed areas increases when precipitation and snow increase. | P3c: Selection for open habitat decreases when weather conditions are hotter, wetter, and snowier. Selection for closed habitat increases when weather conditions are hotter, wetter. | (Shepard et al. 2013; Alston et al. 2020) |
Hypothesis . | Movement or encamped . | Resource selection during stopover . | Resource selection during movement . | References . |
---|---|---|---|---|
H1: Habitat resources | P1a: Stop: Lichen areas Movement: Mostly in open areas/lichen areas and at lower rate in forest areas | P1b: Selection: Open areas/Lichen areas Avoidance: Forest areas | P1c: Selection: Open areas/Lichen areas Avoidance: Forest areas | (Schaefer et al. 2016; Webber et al. 2022) |
H2: Weather conditions | P2a: Probability to stop increases when temperature increases and precipitation and snow decrease | P2b: Positive selection for areas where weather conditions decrease | P2c: Selection for areas where weather conditions are warmer, wetter, and snowier | (Le Corre et al. 2016; Gurarie et al. 2019; Leclerc et al. 2019, 2021; Bright Ross et al. 2021) |
H3: Static resources × dynamic weather conditions | P3a: Probability to stop in open and lichen areas increases when temperature increases and precipitation and snow decrease. Probability of movement would increase in closed areas when weather conditions are hotter, wetter, and snowier. | P3b: Selection for open and lichen habitat is higher when temperature increases and precipitation and snow decrease. Selection for closed areas increases when precipitation and snow increase. | P3c: Selection for open habitat decreases when weather conditions are hotter, wetter, and snowier. Selection for closed habitat increases when weather conditions are hotter, wetter. | (Shepard et al. 2013; Alston et al. 2020) |
Summary of behavioral state and resource selection hypotheses and related predictions.
Hypothesis . | Movement or encamped . | Resource selection during stopover . | Resource selection during movement . | References . |
---|---|---|---|---|
H1: Habitat resources | P1a: Stop: Lichen areas Movement: Mostly in open areas/lichen areas and at lower rate in forest areas | P1b: Selection: Open areas/Lichen areas Avoidance: Forest areas | P1c: Selection: Open areas/Lichen areas Avoidance: Forest areas | (Schaefer et al. 2016; Webber et al. 2022) |
H2: Weather conditions | P2a: Probability to stop increases when temperature increases and precipitation and snow decrease | P2b: Positive selection for areas where weather conditions decrease | P2c: Selection for areas where weather conditions are warmer, wetter, and snowier | (Le Corre et al. 2016; Gurarie et al. 2019; Leclerc et al. 2019, 2021; Bright Ross et al. 2021) |
H3: Static resources × dynamic weather conditions | P3a: Probability to stop in open and lichen areas increases when temperature increases and precipitation and snow decrease. Probability of movement would increase in closed areas when weather conditions are hotter, wetter, and snowier. | P3b: Selection for open and lichen habitat is higher when temperature increases and precipitation and snow decrease. Selection for closed areas increases when precipitation and snow increase. | P3c: Selection for open habitat decreases when weather conditions are hotter, wetter, and snowier. Selection for closed habitat increases when weather conditions are hotter, wetter. | (Shepard et al. 2013; Alston et al. 2020) |
Hypothesis . | Movement or encamped . | Resource selection during stopover . | Resource selection during movement . | References . |
---|---|---|---|---|
H1: Habitat resources | P1a: Stop: Lichen areas Movement: Mostly in open areas/lichen areas and at lower rate in forest areas | P1b: Selection: Open areas/Lichen areas Avoidance: Forest areas | P1c: Selection: Open areas/Lichen areas Avoidance: Forest areas | (Schaefer et al. 2016; Webber et al. 2022) |
H2: Weather conditions | P2a: Probability to stop increases when temperature increases and precipitation and snow decrease | P2b: Positive selection for areas where weather conditions decrease | P2c: Selection for areas where weather conditions are warmer, wetter, and snowier | (Le Corre et al. 2016; Gurarie et al. 2019; Leclerc et al. 2019, 2021; Bright Ross et al. 2021) |
H3: Static resources × dynamic weather conditions | P3a: Probability to stop in open and lichen areas increases when temperature increases and precipitation and snow decrease. Probability of movement would increase in closed areas when weather conditions are hotter, wetter, and snowier. | P3b: Selection for open and lichen habitat is higher when temperature increases and precipitation and snow decrease. Selection for closed areas increases when precipitation and snow increase. | P3c: Selection for open habitat decreases when weather conditions are hotter, wetter, and snowier. Selection for closed habitat increases when weather conditions are hotter, wetter. | (Shepard et al. 2013; Alston et al. 2020) |
Materials and Methods
Data collection and study species.
We studied adult female caribou between 2010 and 2013 from the Middle Ridge herd located on the island of Newfoundland, Canada. Adult female caribou (n = 41 individuals) were captured via helicopter net-gunning and darting while they were on their winter range and fit with GPS telemetry collars (Lotek Wireless Inc., Newmarket, Ontario, Canada, GPS4400M collars, 1,250 g), which recorded locations at 1-, 2-, 4-, 8-, or 12-h intervals, depending on the individuals and season. Collars were deployed on individuals for 1–3 years. To calculate movement behavior, we rarefied all location data to a consistent fix rate of 2 h.
Caribou in the Middle Ridge herd live in a large (~13,000 km2) area in central Newfoundland and experience a humid continental climate (see Supplementary Data SD4). Caribou throughout Newfoundland—including the Middle Ridge herd—forage primarily on lichen, grasses, sedges, and other deciduous browse with access to these resources changing between the seasons (Bergerud 1974). In general, caribou in Middle Ridge have distinct winter and summer home range sizes (Peignier et al. 2019) and most individuals migrate from winter grounds to summer calving grounds (Laforge et al. 2021). During calving, approximately 80% of the herd give birth aggregated on calving grounds, while the other 20% of animals give birth solitarily off the calving grounds (Fifield et al. 2012; Bonar et al. 2020). Migration and calving are inherently linked in caribou because most caribou give birth synchronously near the end of May or early June (Bonar et al. 2020) and migrants arrive to the calving grounds an average of two weeks prior to calving. While migration duration varies among individuals, most caribou time their arrival on the calving grounds with the emergence of vegetation (Laforge et al. 2021). However, seasonal environmental conditions in Newfoundland are variable across years, which might have promoted the persistence of partially migratory behavior, with migrants moving relatively short distances Laforge et al. (2021).
Delineating migratory movements using net squared displacement.
We determined migration timing using net squared displacement (NSD) calculated using the R programming language (R Core Team 2021) and Migration Mapper V 2.0 (Merkle et al. 2022). NSD is the square of the straight-line distance between the first location of an animal in a series of relocations and all its subsequent points. We identified the beginning of the migratory period as the time where an animal moves away from winter range. For this study, NSD was calculated for each individual using 10 February as a starting point (i.e., when caribou are in their winter range) until 2 weeks before the end of migration. After determining the start and end of migration for each individual, we visually inspected migratory tracks to determine which individuals had nonoverlapping seasonal ranges and we considered these individuals to be migratory (Middleton et al. 2013). Only individuals with a mean of four fixes per day within the migratory period for a given year were retained in our analysis. To ensure a greater variation of data within stopover and movement behaviors and to ensure that nonmigratory individuals were excluded, we retained only individuals that migrated a minimum of 30 km. In total, 11 individuals were removed from our analysis, resulting in a final sample size of 33,805 GPS locations for 30 individuals (n = 33,805 locations; caribou-years = 47) between 2010 and 2013 (Table 2).
Meteorological covariates.
To assess the effect of meteorological variables on migratory movements, we sampled daily weather parameters for each individual using Daymet with Google Earth Engine (Gorelick et al. 2017). Daymet provides a gridded estimate of daily weather parameters with a spatial resolution of 1 × 1 km (Thornton et al. 2017). Meteorological parameters were extracted for location points within the study period (19 February to 30 April between 2010 and 2013). Of the available parameters in Daymet, we extracted precipitation (mm/day), maximum air temperature (°C), and snow water equivalent (kg/m²) (see Supplementary Data SD1 for details).
Land cover variables.
To quantify patterns of habitat selection by Woodland Caribou during spring migration, we used 30 m resolution land cover data derived from Landsat 7 satellite imagery. We aggregated available land cover classes to forest (conifer, broadleaf, scrub, and mixed-wood forests), lichen barrens, and other open land cover types (wetland, rocky barrens, and water) (see Supplementary Data SD3 for details).
Behavioral states.
To distinguish caribou stopover sites from areas used as movement corridors, we used hidden Markov models (Langrock et al. 2012), which differentiate between behavioral states in animal movements using step length and turn angle distributions. The model was fit using the moveHMM package version 1.7 (Michelot et al. 2016). We modeled step lengths using a gamma distribution and turning angles using a von Mises distribution and extracted two distinct behavioral states. Stopover, or encamped, states were defined as periods with low step length and relatively high turn angles (more tortuous movement). Movement was defined by relocations with longer step lengths and low turn angles which the model interpreted as active movement phases (see Supplementary Data SD2 for details).
State-dependent habitat selection in a dynamic environment.
We used resource selection functions (RSFs; Manly et al. 2007) to assess patterns of habitat selection by Woodland Caribou during spring migration and to estimate the influence of environmental and meteorological variables on habitat selection. RSFs compare resource covariates at observed locations to those at randomly selected available locations. Given that our RSF models contained variables that changed during the analysis period, we used a conditional RSF model. The conditional logistic regression approach allowed us to account for temporal availability of dynamic variables by specifying temporal strata within the data, thus pairing spatial locations and with temporal weather conditions. Models were fit using a conditional model using package mclogit (Elff et al. 2022). We focused our analysis at the herd level by generating 95% fixed kernel (h = 400) for the entire herd using all locations for all individuals and generating 10 available points for each used location. Meteorological parameters and percentage of each habitat within a 200-m buffer were extracted for each observed and random point. RSF parameters were estimated using a generalized linear mixed model (GLMM), with a binomial distribution that had the following form:
where x is the geographical point in space (used or available), β0 is the intercept, and βi is the coefficient of selection for each habitat variable h. Positive and negative βi values, respectively, indicate selection or avoidance for that variable. The range of βi represents the strength of selection/avoidance for habitat relative to an available alternative. In this study, individual identity and year were included as random effects. Our RSF models included a suite of meteorological (i.e., precipitation, temperature, and snow water equivalent) and land cover (i.e., forest, lichen, and wetland habitats) explanatory variables.
Statistical analysis.
All statistical analyses were conducted in R software version 3.6.1 (R Core Team 2021). Variables were normally distributed and there was no correlation (Pearson’s) greater than r = 0.7 detected among them. We generated two sets of models. The first quantified the probability of animals being in either of the two states defined by our hidden Markov model (hereafter, behavioral state models) to assess the effects of dynamic (weather) and static (land cover) variables on the probability of stopover. The second set of analyses were RSFs (hereafter, resource selection models) to assess the effects of dynamic variables on patterns of habitat selection in stopover and in movement (Table 3). We include caribou-year as random effect in our GLMMs to take into account the potential for variability between years and individuals and we interpret the results from this model at the population level.
Description of candidate models to assess the effects of dynamic weather on fine-scale movement on Woodland Caribou (random effect: caribou-years = 47). Each model set (i.e., behavioral choice, stopover, and movement) involved a separate model. Selection and results for each model set are not comparable.
Model set . | Covariates included . | . | . | |
---|---|---|---|---|
. | . | K . | LL . | ΔAIC . |
Behavioral choice | (1) Habitat model: Habitat: Open + Lichen + Forest | 5 | −11,358.7 | 1916.05 |
(2) Weather model: Temperature + Snow water equivalent + Precipitation | 5 | −16,614.5 | 427.62 | |
(3) Interaction model: Habitat × Weather | 17 | −16,388.6 | 0 | |
Stopover | (1) Habitat model | 3 | −63,485.7 | 4022.9 |
(2) Weather model | 3 | −65,255.4 | 7562.3 | |
(3) Interaction model | 15 | −61,462.3 | 0 | |
Movement | (1) Habitat model | 3 | −22,640.4 | 667.6 |
(2) Weather model | 3 | −22,785.9 | 958.6 | |
(3) Interaction model | 15 | −22,294.6 | 0 |
Model set . | Covariates included . | . | . | |
---|---|---|---|---|
. | . | K . | LL . | ΔAIC . |
Behavioral choice | (1) Habitat model: Habitat: Open + Lichen + Forest | 5 | −11,358.7 | 1916.05 |
(2) Weather model: Temperature + Snow water equivalent + Precipitation | 5 | −16,614.5 | 427.62 | |
(3) Interaction model: Habitat × Weather | 17 | −16,388.6 | 0 | |
Stopover | (1) Habitat model | 3 | −63,485.7 | 4022.9 |
(2) Weather model | 3 | −65,255.4 | 7562.3 | |
(3) Interaction model | 15 | −61,462.3 | 0 | |
Movement | (1) Habitat model | 3 | −22,640.4 | 667.6 |
(2) Weather model | 3 | −22,785.9 | 958.6 | |
(3) Interaction model | 15 | −22,294.6 | 0 |
Candidate models are listed with numbers of parameters (K), log-likelihood (LL), difference in Akaike Information Criterion value versus the best-supported model (ΔAIC). Habitat is described as % of presence within 200-m buffer. Weather variables are described as the mean value per day.
Description of candidate models to assess the effects of dynamic weather on fine-scale movement on Woodland Caribou (random effect: caribou-years = 47). Each model set (i.e., behavioral choice, stopover, and movement) involved a separate model. Selection and results for each model set are not comparable.
Model set . | Covariates included . | . | . | |
---|---|---|---|---|
. | . | K . | LL . | ΔAIC . |
Behavioral choice | (1) Habitat model: Habitat: Open + Lichen + Forest | 5 | −11,358.7 | 1916.05 |
(2) Weather model: Temperature + Snow water equivalent + Precipitation | 5 | −16,614.5 | 427.62 | |
(3) Interaction model: Habitat × Weather | 17 | −16,388.6 | 0 | |
Stopover | (1) Habitat model | 3 | −63,485.7 | 4022.9 |
(2) Weather model | 3 | −65,255.4 | 7562.3 | |
(3) Interaction model | 15 | −61,462.3 | 0 | |
Movement | (1) Habitat model | 3 | −22,640.4 | 667.6 |
(2) Weather model | 3 | −22,785.9 | 958.6 | |
(3) Interaction model | 15 | −22,294.6 | 0 |
Model set . | Covariates included . | . | . | |
---|---|---|---|---|
. | . | K . | LL . | ΔAIC . |
Behavioral choice | (1) Habitat model: Habitat: Open + Lichen + Forest | 5 | −11,358.7 | 1916.05 |
(2) Weather model: Temperature + Snow water equivalent + Precipitation | 5 | −16,614.5 | 427.62 | |
(3) Interaction model: Habitat × Weather | 17 | −16,388.6 | 0 | |
Stopover | (1) Habitat model | 3 | −63,485.7 | 4022.9 |
(2) Weather model | 3 | −65,255.4 | 7562.3 | |
(3) Interaction model | 15 | −61,462.3 | 0 | |
Movement | (1) Habitat model | 3 | −22,640.4 | 667.6 |
(2) Weather model | 3 | −22,785.9 | 958.6 | |
(3) Interaction model | 15 | −22,294.6 | 0 |
Candidate models are listed with numbers of parameters (K), log-likelihood (LL), difference in Akaike Information Criterion value versus the best-supported model (ΔAIC). Habitat is described as % of presence within 200-m buffer. Weather variables are described as the mean value per day.
For the behavioral states models, we used GLMMs with animal identity included as a random effect fit with a binomial distribution using the R package lme4 version 1.1-26 (Bates et al. 2015). We considered behavioral state (1 = encamped and 0 = movement) as the response variable in the behavioral state models. The first model included only habitat variables (forest, lichen, and wetland) as fixed effects; the second model included only weather variables (i.e., precipitation, temperature, and snow water equivalent) as fixed effects; the third model included all habitat and weather variables as fixed effects, including two-way interactions between each weather variable and each land cover variable.
For the resource selection models, we generated two separate RSF models for encamped and moving behavioral states. We used conditional logistic regression with R package mclogit. For the first model we tested the probability that caribou select or avoid a given habitat (forest, lichen, and wetland); for the second model we tested the probability that caribou select or avoid interactive effects of habitat and weather conditions. Using an information theoretic approach, we selected the most parsimonious model for each of our three analyses identified as the models with the lowest AIC (Akaike Information Criterion). Finally, for all RSF models, we used k-fold cross-validation to evaluate the predictive performance of the top models (Boyce et al. 2002; Roberts et al. 2017).
Results
Using the predefined filtering criteria, we extracted data for 30 individuals (47 caribou-years). The migration period started on average on 2 March and ended on 2 May. On average, there were 1,263 locations per individual during the study period. Our hidden Markov models classified 25,089 locations as encamped and 8,716 as movement during spring migration.
Behavioral state models.
Our top model had the most complex structure (Table 3). Specifically, caribou were more likely to stop in lichen and open habitat and less likely to stop in forest habitat (Figs. 1 and 2). Caribou were more likely to be encamped when temperature and snow water equivalent were higher; however, precipitation did not affect behavioral state (Table 4, Fig. 1). The interaction between weather conditions and land cover influenced the probability of being encamped (Fig. 2). When snow water equivalent and precipitation were higher, caribou were more likely to be encamped in wetland areas. In contrast, warmer temperatures reduced the probability that caribou would be encamped in lichen habitat and increased the probability to be encamped in forest habitat. When precipitation increased, caribou were more likely to be encamped in forest habitat while when snow water equivalent increased, caribou were more likely to be in movement.
Estimates and 95% confidence interval (CI) of the best-supported model to assess fine-scale movement and habitat selection under weather conditions during spring migration of Woodland Caribou in Newfoundland, Canada, between 2010 and 2013. Numbers in bold represent effects with P < 0.05. Interactions between variables are represented by the symbol ×.
Models . | Behavioral choicea . | Encampedb . | Movementb . | ||||||
---|---|---|---|---|---|---|---|---|---|
. | Estimates . | CI (95%) . | Estimates . | CI (95%) . | Estimates . | CI (95%) . | |||
. | . | Low . | High . | . | Low . | High . | . | Low . | High . |
Open | 0.21 | 0.13 | 0.28 | 0.27 | 0.23 | 0.31 | 0.12 | 0.07 | 0.17 |
Lichen | 0.34 | 0.27 | 0.41 | 0.42 | 0.38 | 0.45 | 0.18 | 0.14 | 0.22 |
Forest | 0.03 | −0.03 | 0.09 | −0.25 | −0.29 | −0.21 | −0.16 | −0.21 | −0.11 |
Temperature | 0.08 | 0.05 | 0.11 | 0.90 | 0.75 | 1.05 | 1.32 | 1.10 | 1.55 |
Snow water equivalent | 0.64 | 0.61 | 0.68 | −0.04 | −0.07 | −0.01 | 0.18 | 0.06 | 0.29 |
Precipitation | 0.01 | −0.02 | 0.04 | −0.47 | −0.53 | −0.41 | 0.10 | 0.01 | 0.20 |
Open × Temperature | 0.35 | 0.25 | 0.44 | 0.28 | 0.24 | 0.32 | 0.15 | 0.10 | 0.20 |
Open × Snow water equivalent | −0.01 | −0.07 | 0.04 | 0.06 | 0.03 | 0.08 | 0.07 | 0.03 | 0.11 |
Open × Precipitation | 0.10 | 0.03 | 0.17 | 0.02 | −0.02 | 0.06 | −0.03 | −0.08 | 0.01 |
Lichen × Temperature | 0.16 | 0.07 | 0.25 | −0.12 | −0.15 | −0.08 | −0.12 | −0.16 | −0.08 |
Lichen × Snow water equivalent | −0.08 | −0.13 | −0.02 | 0.07 | 0.04 | 0.09 | −0.01 | −0.05 | 0.02 |
Lichen × Precipitation | 0.09 | 0.03 | 0.16 | 0.01 | −0.02 | 0.04 | −0.03 | −0.06 | 0.01 |
Forest × Temperature | 0.34 | 0.27 | 0.42 | 0.44 | 0.40 | 0.48 | 0.23 | 0.18 | 0.28 |
Forest × Snow water equivalent | −0.08 | −0.12 | −0.03 | −0.03 | −0.05 | 0 | 0.01 | −0.03 | 0.05 |
Forest × Precipitation | 0.06 | 0.00 | 0.12 | −0.02 | −0.06 | 0.02 | −0.05 | −0.10 | −0.01 |
Models . | Behavioral choicea . | Encampedb . | Movementb . | ||||||
---|---|---|---|---|---|---|---|---|---|
. | Estimates . | CI (95%) . | Estimates . | CI (95%) . | Estimates . | CI (95%) . | |||
. | . | Low . | High . | . | Low . | High . | . | Low . | High . |
Open | 0.21 | 0.13 | 0.28 | 0.27 | 0.23 | 0.31 | 0.12 | 0.07 | 0.17 |
Lichen | 0.34 | 0.27 | 0.41 | 0.42 | 0.38 | 0.45 | 0.18 | 0.14 | 0.22 |
Forest | 0.03 | −0.03 | 0.09 | −0.25 | −0.29 | −0.21 | −0.16 | −0.21 | −0.11 |
Temperature | 0.08 | 0.05 | 0.11 | 0.90 | 0.75 | 1.05 | 1.32 | 1.10 | 1.55 |
Snow water equivalent | 0.64 | 0.61 | 0.68 | −0.04 | −0.07 | −0.01 | 0.18 | 0.06 | 0.29 |
Precipitation | 0.01 | −0.02 | 0.04 | −0.47 | −0.53 | −0.41 | 0.10 | 0.01 | 0.20 |
Open × Temperature | 0.35 | 0.25 | 0.44 | 0.28 | 0.24 | 0.32 | 0.15 | 0.10 | 0.20 |
Open × Snow water equivalent | −0.01 | −0.07 | 0.04 | 0.06 | 0.03 | 0.08 | 0.07 | 0.03 | 0.11 |
Open × Precipitation | 0.10 | 0.03 | 0.17 | 0.02 | −0.02 | 0.06 | −0.03 | −0.08 | 0.01 |
Lichen × Temperature | 0.16 | 0.07 | 0.25 | −0.12 | −0.15 | −0.08 | −0.12 | −0.16 | −0.08 |
Lichen × Snow water equivalent | −0.08 | −0.13 | −0.02 | 0.07 | 0.04 | 0.09 | −0.01 | −0.05 | 0.02 |
Lichen × Precipitation | 0.09 | 0.03 | 0.16 | 0.01 | −0.02 | 0.04 | −0.03 | −0.06 | 0.01 |
Forest × Temperature | 0.34 | 0.27 | 0.42 | 0.44 | 0.40 | 0.48 | 0.23 | 0.18 | 0.28 |
Forest × Snow water equivalent | −0.08 | −0.12 | −0.03 | −0.03 | −0.05 | 0 | 0.01 | −0.03 | 0.05 |
Forest × Precipitation | 0.06 | 0.00 | 0.12 | −0.02 | −0.06 | 0.02 | −0.05 | −0.10 | −0.01 |
aGeneralized linear mixed model to assess the probability to be in stopover or in movement under static (habitat) and dynamic (weather) conditions.
bMixed conditional logistic regression model to assess the resource selection under static (habitat) and dynamic (weather) conditions.
Models are evaluated for each behavioral state.
Estimates and 95% confidence interval (CI) of the best-supported model to assess fine-scale movement and habitat selection under weather conditions during spring migration of Woodland Caribou in Newfoundland, Canada, between 2010 and 2013. Numbers in bold represent effects with P < 0.05. Interactions between variables are represented by the symbol ×.
Models . | Behavioral choicea . | Encampedb . | Movementb . | ||||||
---|---|---|---|---|---|---|---|---|---|
. | Estimates . | CI (95%) . | Estimates . | CI (95%) . | Estimates . | CI (95%) . | |||
. | . | Low . | High . | . | Low . | High . | . | Low . | High . |
Open | 0.21 | 0.13 | 0.28 | 0.27 | 0.23 | 0.31 | 0.12 | 0.07 | 0.17 |
Lichen | 0.34 | 0.27 | 0.41 | 0.42 | 0.38 | 0.45 | 0.18 | 0.14 | 0.22 |
Forest | 0.03 | −0.03 | 0.09 | −0.25 | −0.29 | −0.21 | −0.16 | −0.21 | −0.11 |
Temperature | 0.08 | 0.05 | 0.11 | 0.90 | 0.75 | 1.05 | 1.32 | 1.10 | 1.55 |
Snow water equivalent | 0.64 | 0.61 | 0.68 | −0.04 | −0.07 | −0.01 | 0.18 | 0.06 | 0.29 |
Precipitation | 0.01 | −0.02 | 0.04 | −0.47 | −0.53 | −0.41 | 0.10 | 0.01 | 0.20 |
Open × Temperature | 0.35 | 0.25 | 0.44 | 0.28 | 0.24 | 0.32 | 0.15 | 0.10 | 0.20 |
Open × Snow water equivalent | −0.01 | −0.07 | 0.04 | 0.06 | 0.03 | 0.08 | 0.07 | 0.03 | 0.11 |
Open × Precipitation | 0.10 | 0.03 | 0.17 | 0.02 | −0.02 | 0.06 | −0.03 | −0.08 | 0.01 |
Lichen × Temperature | 0.16 | 0.07 | 0.25 | −0.12 | −0.15 | −0.08 | −0.12 | −0.16 | −0.08 |
Lichen × Snow water equivalent | −0.08 | −0.13 | −0.02 | 0.07 | 0.04 | 0.09 | −0.01 | −0.05 | 0.02 |
Lichen × Precipitation | 0.09 | 0.03 | 0.16 | 0.01 | −0.02 | 0.04 | −0.03 | −0.06 | 0.01 |
Forest × Temperature | 0.34 | 0.27 | 0.42 | 0.44 | 0.40 | 0.48 | 0.23 | 0.18 | 0.28 |
Forest × Snow water equivalent | −0.08 | −0.12 | −0.03 | −0.03 | −0.05 | 0 | 0.01 | −0.03 | 0.05 |
Forest × Precipitation | 0.06 | 0.00 | 0.12 | −0.02 | −0.06 | 0.02 | −0.05 | −0.10 | −0.01 |
Models . | Behavioral choicea . | Encampedb . | Movementb . | ||||||
---|---|---|---|---|---|---|---|---|---|
. | Estimates . | CI (95%) . | Estimates . | CI (95%) . | Estimates . | CI (95%) . | |||
. | . | Low . | High . | . | Low . | High . | . | Low . | High . |
Open | 0.21 | 0.13 | 0.28 | 0.27 | 0.23 | 0.31 | 0.12 | 0.07 | 0.17 |
Lichen | 0.34 | 0.27 | 0.41 | 0.42 | 0.38 | 0.45 | 0.18 | 0.14 | 0.22 |
Forest | 0.03 | −0.03 | 0.09 | −0.25 | −0.29 | −0.21 | −0.16 | −0.21 | −0.11 |
Temperature | 0.08 | 0.05 | 0.11 | 0.90 | 0.75 | 1.05 | 1.32 | 1.10 | 1.55 |
Snow water equivalent | 0.64 | 0.61 | 0.68 | −0.04 | −0.07 | −0.01 | 0.18 | 0.06 | 0.29 |
Precipitation | 0.01 | −0.02 | 0.04 | −0.47 | −0.53 | −0.41 | 0.10 | 0.01 | 0.20 |
Open × Temperature | 0.35 | 0.25 | 0.44 | 0.28 | 0.24 | 0.32 | 0.15 | 0.10 | 0.20 |
Open × Snow water equivalent | −0.01 | −0.07 | 0.04 | 0.06 | 0.03 | 0.08 | 0.07 | 0.03 | 0.11 |
Open × Precipitation | 0.10 | 0.03 | 0.17 | 0.02 | −0.02 | 0.06 | −0.03 | −0.08 | 0.01 |
Lichen × Temperature | 0.16 | 0.07 | 0.25 | −0.12 | −0.15 | −0.08 | −0.12 | −0.16 | −0.08 |
Lichen × Snow water equivalent | −0.08 | −0.13 | −0.02 | 0.07 | 0.04 | 0.09 | −0.01 | −0.05 | 0.02 |
Lichen × Precipitation | 0.09 | 0.03 | 0.16 | 0.01 | −0.02 | 0.04 | −0.03 | −0.06 | 0.01 |
Forest × Temperature | 0.34 | 0.27 | 0.42 | 0.44 | 0.40 | 0.48 | 0.23 | 0.18 | 0.28 |
Forest × Snow water equivalent | −0.08 | −0.12 | −0.03 | −0.03 | −0.05 | 0 | 0.01 | −0.03 | 0.05 |
Forest × Precipitation | 0.06 | 0.00 | 0.12 | −0.02 | −0.06 | 0.02 | −0.05 | −0.10 | −0.01 |
aGeneralized linear mixed model to assess the probability to be in stopover or in movement under static (habitat) and dynamic (weather) conditions.
bMixed conditional logistic regression model to assess the resource selection under static (habitat) and dynamic (weather) conditions.
Models are evaluated for each behavioral state.
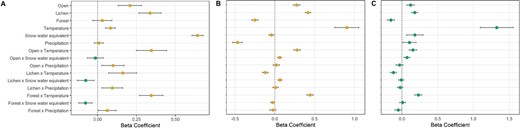
Beta coefficients and 95% confidence interval indicating the population level behavioral response of Woodland Caribou as a function of land cover and weather. Panel A represents the first model (behavioral choice) with probability to be more likely in stopover (>0) and in movement (<0). Panel B (stopover) and C (movement) represent the resource selection model with probability to select or avoid habitat resources in interactions with weather conditions.
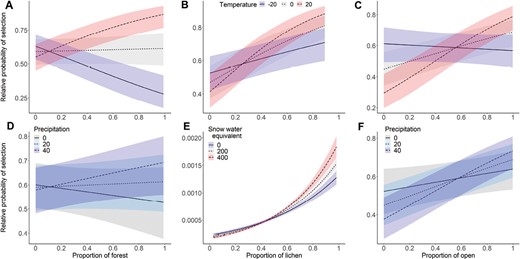
Relative probability (± 95% confidence interval) of Woodland Caribou resource selection in relation to weather conditions from the top behavioral state model within three weather intervals during spring migration from 2013 to 2015 in the Middle Ridge area in Newfoundland. Panel A), B), and C) represent significant interaction with temperature. Panel D) represents interaction between forest and precipitation, E) lichen and snow water equivalent, and F) open and precipitation. Colors represent different level of weather conditions.
Habitat selection models: stopover.
Our top model included land cover, weather, and two-way interactions between land cover and weather covariates (Table 3). During the encamped state, caribou selected open habitat types (i.e., lichen and wetland; Fig. 1). Dynamic weather conditions affected selection of encamped sites where they were more likely to avoid areas where snow water equivalent and precipitation were higher. However, caribou were always more likely to select areas where temperature was higher (Table 4, Fig. 1). The negative interaction between lichen and temperature means that those two effects are not additive—they do not select as highly for these variables as they both increase. Caribou selected for lichen habitat when snow water equivalent was higher (Fig. 3). However, the interaction between forest and precipitation was not significant (Fig. 3). When temperature and snow water equivalent were higher, caribou selected for open habitat, which means that weather conditions did not affect the selection for open habitat. The stopover model had a strong k-fold cross-validation score (mean ρ = 0.79, SD = 0.15).

Relative probability (± 95% confidence interval) of Woodland Caribou resource selection in relation to weather conditions from the top conditional logistic model (in stopover) within three weather intervals during spring migration from 2013 to 2015 in the Middle Ridge area in Newfoundland. Panel A), B), and C) represent significant interaction with temperature. Panel D) represents interaction between forest and precipitation, E) lichen and snow water equivalent, and F) open and precipitation. Colors represent different level of weather conditions.
Habitat selection models: movement.
Our top model included habitat, weather, and two-way interactions between covariates (Table 3). During the movement state, caribou selected for lichen and open habitats, but avoided forest habitats (Fig. 1). In general, caribou were more likely to move through areas where temperature and snow water equivalent were higher. Weather conditions had less of an effect on habitat selection during movement than during encampment. Specifically, precipitation did not interact significantly with open and lichen habitat, and there was no significance of snow water equivalent in interaction with lichen and forest (Table 4, Fig. 4). Only snow water equivalent interacted to positively affect probability of selection for open habitat. Caribou were more likely to avoid lichen habitats when temperature increased, while they were more likely to select forest habitats when temperature increased. The movement model had a low to moderate k-fold cross-validation score (mean ρ = 0.34, SD = 0.19).
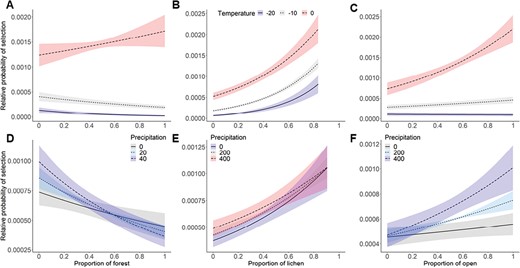
Relative probability (± 95% confidence interval) of Woodland Caribou resource selection in relation to weather conditions from the top conditional logistic model (in movement) within three weather intervals during spring migration from 2013 to 2015 in the Middle Ridge area in Newfoundland. Panel A), B), and C) represent significant interaction with temperature. Panel D) represents interaction between forest and precipitation, E) lichen and precipitation, and F) open and precipitation. Colors represent different level of weather conditions.
Discussion
Animal migration is influenced by both predictable features of the landscape and unpredictable weather conditions. During migration, animals must decide between staying in an area to exploit its resources to gain energy or moving to a potentially better area, where there may be a long-term gain but short-term energy loss. Here, we investigated the effect of weather conditions on resource selection during spring migration of Woodland Caribou. In accordance with our predictions, we found that during migration, both static—that is, predictable—resources, and dynamic—that is, unpredictable—weather conditions affect the choice caribou make to move or to stay. We also found that caribou modified migratory movements and resource selection as a function of weather conditions. Specifically, even if caribou select a specific resource during migration, weather conditions can alter the selection, even when the environment provides high-quality resources. Taken together, our results suggest that an important interaction between static and dynamic environmental variables influences patterns of habitat selection during migration for Woodland Caribou.
Weather can impact the decisions an animal makes during migration. For example, caribou in northern Québec migrated more slowly when snow was deeper and there was higher precipitation (Leclerc et al. 2021). Similarly, we found the probability of stopping during migration—that is, remaining encamped—increases with increasing temperatures and increasing snow cover. These seemingly similar findings are supported by caribou natural history and biology. At the end of winter, when caribou begin their migration, snow is present, and since movement is more constrained in the snow, caribou are likely to move less, an expectation that is linked to physical effort or a longer search for food (Fancy and White 1987). Snow-covered environments during spring migration prevent caribou from easily accessing vegetation and increasing temperature melts snow more quickly, which can provide easier access to resources. It is possible that caribou increase movement rates at the end of migration by selecting habitats where the snow has already melted combined with selection for areas with higher temperature (Laforge et al. 2021). This strategy would allow individuals to time their arrival on the calving grounds with the emergence of vegetation (Laforge et al. 2021). In addition to weather conditions, other factors may also influence daily movements during migration, for example, individuals demonstrate familiarity toward certain conspecifics, a social process that affects selection of foraging habitats.
Caribou migratory patterns were diverse and ranged from relatively short to relatively long periods of time. Short-distance migrants—similar to caribou in Newfoundland—are known to demonstrate greater migratory flexibility, for example, they are more likely to delay migration if environmental conditions are poor (Laforge et al. 2021). Regardless of migratory strategy, individuals are predicted to maximize the profitability of stopovers during migration. Dynamic weather conditions that caribou face during stopovers and while moving between stopover sites affects resource selection during migration. Many plants emerge in spring during periods of calf growth and maturation (Laforge et al. 2021). During migration, caribou are more likely to stay encamped in open areas where they are less vulnerable to predators and can feed in safety (Bergerud 1972). The probability of caribou staying encamped in open areas that contain lichen was high. Our resource selection model indicates that although caribou select lichen, variability in temperature-related conditions affects its selection such that the probability of lichen selection decreased as temperature increased. A similar pattern was observed for forested habitat. Caribou are more likely to keep moving in forested areas, potentially to facilitate travel and balancing predator avoidance (Leblond et al. 2016; Viejou et al. 2018). During movement, the probability of selecting forest increased as temperature increased. For example, selection for areas with high temperatures may result in a gain in energy from resource availability due to increased snowmelt (Fancy and White 1987), but also may incur a cost in the form of increased thermoregulation and energy expended (Alston et al. 2021; Holmes et al. 2021). Here, precipitation did not affect habitat selection. However, caribou may use precipitation as a cue to predict snow abundance during migration and adjust migration to limit costs of moving through deep snow (Le Corre et al. 2016). Higher precipitation and snow depth are unfavorable conditions for resource access and we expect the energetic trade-off is unbalanced if caribou select open habitat under higher precipitation and snow depth conditions.
Regarding predators, the Coyote (Canis latrans) and Black Bear (Ursus americanus) are the primary predators of caribou in Newfoundland, although adults face relatively limited risk of predation (Rayl et al. 2014). We did not quantify the effects of predation on migration in this study. Nevertheless, the presence of predators remains a threat to caribou and represents a nonconsumptive effect (Bastille-Rousseau et al. 2016) that can have physiological costs as a result of reduced foraging opportunities or heightened stress (Gaynor et al. 2019).
Climate change will increase the intensity and frequency of extreme weather events (IPCC 2014), which are known to limit food access and reduce fitness of caribou (Stien et al. 2012). Our findings that meteorological variables influence movement and habitat selection of caribou during migration suggest the possibility that shifts in weather could have deleterious effects on the ability for caribou to successfully reach calving grounds. In view of global climate change, caribou will have to increasingly adapt to greater spatial–temporal unpredictability in weather conditions, likely changing the profitability of the landscape during migrations (Kubelka et al. 2022). To survive and maintain access to food resources, and to reach calving grounds and give birth, it will be necessary for migratory strategies to be flexible to cope with unpredictable conditions. The ability of migrants to synchronize movement with environmental variables such as vegetation greenness will be more affected by unpredictability in weather and could have a negative effect on calving post-migration (Gurarie et al. 2019). The increase in temperature could have an effect on the speed of snowmelt, which would result in a rapid increase in a food resource, forcing caribou to synchronize quickly with these resources. An alternative strategy, already present in other ungulates populations, such as Pronghorn (Antilocapra americana; Kauffman et al. 2021b), would be to reduce the migration distance or even become resident. While altering migratory strategies to such an extreme may be suboptimal in many cases, it is possible that in some cases, resident individuals may have higher survival and reproductive success compared to migrants, thus resulting in a shift toward residency over migration (e.g., Hebblewhite and Merrill 2011).
Migration is a behavior that is at risk of being altered, or potentially lost altogether, by climate change (Bright Ross et al. 2021; Kauffman et al. 2021a). Particularly for species at risk, the effects of more frequent and intense weather events on migratory behavior may have unknown consequences (Kauffman et al. 2021b). We show that caribou can modify migratory space use in response to unpredictable weather conditions. At a broader scale, our results suggest that caribou might be cuing their movement behaviors into local phenology during migration, which may buffer changing climate. However, if resource availability is influenced by weather conditions, and thus climate change, then changing weather could affect the capacity of caribou to select appropriate resources during migration. Overall, our study highlights proximate behavioral modifications of female caribou during migration. Ultimately, the ability for caribou to adjust their behavior during migration could have an impact on the likelihood of rearing a calf to adulthood. We propose that future work consider behavioral plasticity (e.g., Vander Wal et al. 2022) of the fine-scale movements during migration in response to changes in static resources and dynamic weather conditions. By decomposing migration as a series of stopovers and movements between stopovers, and assessing the habitat selection choice sets as a function of weather conditions, we showed the importance of environmental unpredictability on caribou movements.
Supplementary Data
Supplementary data are available at Journal of Mammalogy online.
Supplementary Data SD1.—Mean ± SD, minimum and maximum value of meteorological and environmental variables between February 2010 and April 2013 in the Middle Ridge area (Newfoundland, Canada).
Supplementary Data SD2.—Distribution of step lengths and turning angles between hourly relocations, respectively, for encamped (1) and movement behavior (2). X-axis for step length was truncated at 5,000 m limit to facilitate visualization (maximum observed step length was 6,957 m).
Supplementary Data SD3.—Land cover and weather covariates showing median, 25% and 75% quartiles, and 95% confidence intervals between locations classified as encamped (orange) and moving (green).
Supplementary Data SD4.—Newfoundland map, including study area (Middle Ridge herd).
Acknowledgments
We respectfully acknowledge the territory in which data were collected and analyzed as the ancestral homelands of the Beothuk and the Island of Newfoundland as the ancestral homelands of the Mi’kmaq and Beothuk. The authors acknowledge members of the Newfoundland and Labrador Wildlife Division including S. Moores, B. Adams, C. Doucet, W. Barney, F. Norman, R. Otto, J. Neville, P. Saunders, T. Porter, P. Tremblett, S. Gullage, T. Hodder, D. Jennings, and J. McGinn for managing and curating data on Newfoundland caribou. We thank T. Bergerud and S. Mahoney for their vision in initiating much of the work on caribou in Newfoundland. The authors acknowledge all members of the Wildlife Evolutionary Ecology Lab for helpful comments on previous versions of this manuscript.
Conflict of Interest
The authors declare that they have no conflicts of interest.
Funding
Funding was provided by Natural Sciences and Engineering Research Council of Canada grants to QMRW (Vanier CGS), MPL (PGS-D), and EVW (Discovery).
Author Contributions
ED, MPL, QMRW, and EVW conceived the idea and designed the study. ED analyzed the data. ALR helped with the code. ED led the writing of the manuscript, supported by QMRW and EVW. All coauthors contributed critically to manuscript drafts and gave final approval for publication.
Data Availability
Code for performing all analyses and generating the figures is available at https://github.com/wildlifeevoeco/UncertaintyDuringMigration (Dedeban et al. 2023) and released under the GNU General Public License version 3.0. We depend on Earth Engine (Gorelick et al. 2017) and R (R Core Team 2021), including numerous R packages notably for the analysis: moveHMM (Michelot et al. 2016), mclogit, lme4 (Bates et al. 2015) and for the figures: ggplot2 (Wickham 2016).