-
PDF
- Split View
-
Views
-
Cite
Cite
Keaton Karlinsey, Lili Qu, Alyssa J Matz, Beiyan Zhou, A novel strategy to dissect multifaceted macrophage function in human diseases, Journal of Leukocyte Biology, Volume 112, Issue 6, December 2022, Pages 1535–1542, https://doi.org/10.1002/JLB.6MR0522-685R
- Share Icon Share
Abstract
Macrophages are widely distributed immune cells that play central roles in a variety of physiologic and pathologic processes, including obesity and cardiovascular disease (CVD). They are highly plastic cells that execute diverse functions according to a combination of signaling and environmental cues. While macrophages have traditionally been understood to polarize to either proinflammatory M1-like or anti-inflammatory M2-like states, evidence has shown that they exist in a spectrum of states between those 2 phenotypic extremes. In obesity-related disease, M1-like macrophages exacerbate inflammation and promote insulin resistance, while M2-like macrophages reduce inflammation, promoting insulin sensitivity. However, polarization markers are expressed inconsistently in adipose tissue macrophages, and they additionally exhibit phenotypes differing from the M1/M2 paradigm. In atherosclerotic CVD, activated plaque macrophages can also exist in a range of proinflammatory or anti-inflammatory states. Some of these macrophages scavenge lipids, developing into heterogeneous foam cell populations. To better characterize the many actions of macrophages in human disease, we have designed a novel set of computational tools: MacSpectrum and AtheroSpectrum. These tools provide information on the inflammatory polarization status, differentiation, and foaming of macrophages in both human and mouse samples, allowing for better characterization of macrophage subpopulations based on their function. Using these tools, we identified disease-relevant cell states in obesity and CVD, including the novel concept that macrophage-derived foam cell formation can follow homeostatic noninflammatory or pathogenic inflammatory foaming programs.
INTRODUCTION
Macrophages are the most widely distributed immune population in the body.1,2 They reside in nearly every tissue, performing many diversified functions.1,3 Macrophages are highly plastic, adapting to their environment to effect necessary functions based on various and often conflicting cues.3–7 Beyond their canonical innate immune and biodefense functions, macrophages also play essential roles in many processes such as tissue homeostasis,8 wound healing,9 cancer,10 autoimmunity,11,12 and cardiovascular disease (CVD).13,14 The range of phenotypes that macrophages acquire in changing environments and disease contexts has made it challenging to characterize their functions well, especially before the advent of single-cell technologies.1,15 To better characterize these diverse functions for the development of effective disease therapies, it is clear that more comprehensive, function-based approaches are needed.1,15–17
Macrophages arise from both fetal and bone marrow origins, forming populations of self-renewing tissue-resident cells as well as maturing from circulating monocytes.18,19 Traditionally, macrophages are characterized as polarizing toward either proinflammatory “classical” M1 or anti-inflammatory “alternative” M2 phenotypes.9,20 This concept was based on the observation that macrophages acquired activation states similar to either Th1 or Th2 lymphocytes depending on culture conditions, and thus it was termed that macrophages could “polarize” to either phenotype.21 In transitioning between polarization states, macrophages undergo changes in protein expression, transcriptional networks, epigenetic states, and metabolism.6,21–25 These changes reflect responses to stimulus necessary to perform functions in various environments, and they have been reviewed elsewhere.19,23,26–29 While the M1/M2 model successfully described 2 phenotypic extremes in activated macrophages, a combination of conflicting experimental findings and the advent of single-cell technologies have demonstrated that macrophages exist along a whole spectrum of states in between the M1 and M2 phenotypes.1,19,22 These heterogeneous activation states are dynamic, often regulated by subtle transcriptional changes not easily identified by bulk comparisons of large groups of cells.30,31
Single-cell RNA sequencing (scRNA-seq) is a powerful technology that allows for transcriptomic analysis at the individual cell level. It has revolutionized our understanding of dynamic and diverse changes in cell state. Macrophages are an ideal target for scRNA-seq analysis as it provides the resolution necessary to capture their heterogeneous phenotypes under different conditions and the dynamic regulatory networks that control them. Here we describe disease-specific challenges in characterizing macrophage diversity, current strategies to annotate macrophage functions, and a set of computational tools we have developed to characterize macrophages’ diversified actions in vivo.
MACROPHAGE HETEROGENEITY IN OBESITY-RELATED DISEASE
Obesity is characterized by the hallmarks of chronic inflammation and insulin resistance, which can lead to type 2 diabetes. Macrophages are the most abundant immune population in visceral adipose tissue (VAT), and they accumulate in VAT under obese conditions, contributing to disease pathogenesis.32–34 Inflammation from adipose tissue macrophages (ATM) is a significant instigator of insulin resistance and diabetes development; therefore, understanding the full spectrum of ATM activation is critical to preventing and treating obesity-related metabolic disease.33,34 Their roles here have been discussed thoroughly elsewhere,35–37 so we will focus on essential components of ATM in metabolic disease and challenges in characterizing them.
Resident ATMs typically exhibit an anti-inflammatory M2-like phenotype, but in obese humans and mice, they polarize toward an M1-like state38,39 (Figure 1). M1-like ATMs under obese conditions usually secrete proinflammatory cytokines such as TNF-α, IL-1β, IL-12, and IL-23.38,39 They also express inflammatory transcriptional pathways, including JNK, ERK, p38, and NF-κB.38,39 ATM inflammatory signals in obesity alter adipocyte MAPK signaling, leading to down-regulation of GLUT4 and subsequent insulin resistance.35,40,41 Under lean conditions, murine ATMs undergo anti-inflammatory M2-like activation, producing cytokines such as IL-10 and TGF-β.42,43 M2-like macrophages were shown to protect cultured adipocytes from TNF-α-induced insulin resistance, highlighting their protective role in disease.38
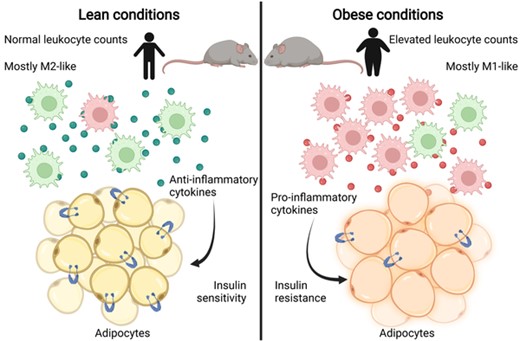
Spectrum of adipose tissue macrophage states in lean and obese conditions. Under lean conditions, ATMs are predominantly polarized toward an M2-like state, releasing mostly anti-inflammatory cytokines. Adipocytes maintain normal insulin sensitivity in lean conditions. Under obese conditions, there is an increase in the number of macrophages, primarily polarized toward an M1-like state. These ATMs secrete proinflammatory cytokines, contributing to inflammation and insulin resistance in adipocytes
Obesity-related inflammation has also exposed the limitations of viewing M1/M2 polarization as a pure dichotomy, highlighting the spectrum of states that macrophages can adopt.41 In one study, classical M1 activation markers were absent on human ATMs that showed enriched inflammatory and lipid-handling pathways.44 Complicating matters are findings that these macrophages can perform either detrimental or beneficial functions depending on the stage of obesity progression.45 Because macrophages can adapt rapidly and dynamically to short-term metabolic and inflammatory circumstances, and they can even regulate adipocyte energy storage, additional tools are necessary to understand the plasticity and diversity of ATMs in human disease.46,47
MACROPHAGE HETEROGENEITY AND PLASTICITY IN CVD
Macrophage polarization and activation also play significant roles in CVD,48–50 and the topic has been reviewed extensively elsewhere.13,14,48 During atherosclerosis development, circulating monocytes are recruited into the vascular intima at sites of low and disturbed blood flow.14 The invading monocytes scavenge oxidized lipoproteins present due to dysregulated lipid metabolism and mature into activated macrophages called foam cells.14,48 Over time, these lesions coalesce into fatty streaks, which in turn progress into atherosclerotic plaques. Accumulating plaques can become unstable over time, leading to rupture and release of potentially occlusive clots.
Macrophages are central players in every step of atherosclerosis pathogenesis. Circulating monocytes are recruited in the initial stage, prior to activation and polarization 13,51 (Figure 2). Monocytes that infiltrate atherosclerotic lesions and scavenge oxidized lipids generally acquire M1-like inflammatory phenotypes, releasing cytokines, reactive oxygen species, and matrix metalloproteases (MMPs).48,52 Inflammation recruits additional monocytes from systemic circulation, which enter the lesion and exacerbate progression. MMPs released from plaque macrophages destabilize the extracellular matrix, causing unstable plaques and predisposing them to rupture.13,48,52 M2-like macrophages, on the other hand, were thought to be protective against disease progression, and in some experiments, even drove regression of atherosclerotic lesions.53–55 More recent studies have shown more significant heterogeneity and plasticity among plaque macrophages, with both M1- and M2-polarized subsets having protective and deleterious effects.50,54,56
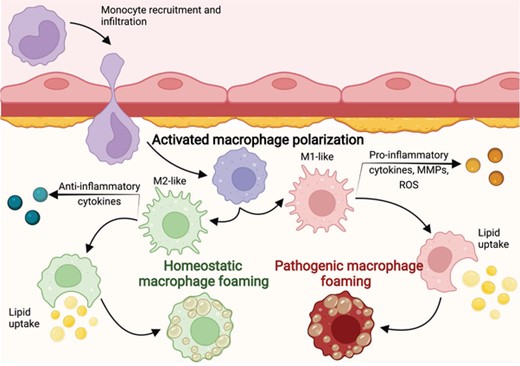
Homeostatic and pathogenic foaming programs. Circulating monocytes are recruited to atherosclerotic lesions and are activated after infiltration. The monocyte–macrophages can be activated anywhere along the M1/M2 spectrum, after which some will scavenge lipids and become foam cells. Inflammatory macrophages will undergo the pathogenic foaming program, while noninflammatory macrophages will follow the homeostatic foaming program
An emerging concept that further indicates the need for better characterization of macrophages in CVD is that foam cells may not all be pathogenic. Foam cells had classically been understood to be among the principal offenders in atherosclerosis pathology, but recent scRNA-seq studies identified foam cells that were noninflammatory and that did not contribute to disease pathogenesis.57–59 Based on these observations, we recently proposed that foaming, like other macrophage functions, exists on a continuum under both inflammatory and noninflammatory conditions.58 We labeled noninflammatory foaming as homeostatic and inflammatory foaming as pathogenic. This concept only emerged after the arrival of scRNA-seq technology, and it highlights the need for macrophage annotation tools capable of capturing their dynamic changes in cell state.
CURRENT BIOINFORMATICS STRATEGIES TO EVALUATE MACROPHAGE POLARIZATION
Current strategies to determine macrophage polarization and maturation status typically involve measurement of expressed markers at the protein or RNA levels.60,61 Flow cytometry and cell sorting are effective and efficient tools for identifying cells maximally polarized to the M1/M2 states, but the range of phenotypes in between these states is less easily captured using these techniques. Transcriptomic technologies, such as microarray and RNA-sequencing at the bulk or individual cell levels, aim to delineate the status of macrophage transcriptomes, which can be further analyzed for cell state and function using programs based on enrichment of particular gene sets or transcription factors.62,63 The advent of scRNA-seq technology has provided additional resolution to evaluate macrophage states and has led to the development of a number of bioinformatics tools and analysis strategies.
To deal with high-dimensional data, most computational programs for analyzing scRNA-seq data rely on whole-transcriptome similarity comparisons to establish a cell matrix, which is used for dimension reduction and downstream cell clustering. Unbiased algorithms such as t-distributed stochastic neighbor embedding64 or uniform manifold approximation and projection65 are employed to portray cells in visualizable low-dimensional space, after which cells are clustered based on relative distances transformed from the cell matrix. In order to study subpopulations of interest, groups of cells within the cluster for their lineage must first be identified and annotated using known biomarker genes, followed by cell feature characterization. Due to the high dropout rate of scRNA-seq data, lineage-specific biomarker genes are not reliably detected. To address this issue, various groups have used both supervised and unsupervised machine learning-based classification algorithms to assign cell identities, such as Garnett,66 CellAssign,67 and others.31,68–70 Recent advances in single-cell multiomics such as Cellular Indexing of Transcriptomes and Epitopes by Sequencing have also provided the ability to simultaneously measure mRNA and surface protein expression, further facilitating cell type identification.71,72
However, although cell functions are often associated with their identity (i.e., cell state), cells of a given type can perform multiple functions, and multiple cell types can perform similar functions. None of the above-mentioned programs intentionally incorporate cell function-relevant gene sets to characterize cell actions, meaning that they do not track relatively subtle transcriptomic features crucial for cell function, including for monocytes or macrophages.15,73 Furthermore, the rapid yet tightly orchestrated responses of macrophages to a wide variety of stimuli (cytokines, chemokines, pathogens, etc.) 22,74 and metabolites (i.e., glucose, lipids, amino acids) 25,75,76 induce vibrant transcriptomic dynamics to facilitate their multifaceted functions in different tissue and disease contexts.20 For example, monocytes and M2-polarized macrophages primarily rely on oxidative phosphorylation for cell metabolism, whereas M1-polarized cells employ enhanced glycolysis.28,29 In one study, the metabolite α-ketoglutarate impaired proinflammatory responses in M1-polarized macrophages while promoting M2-polarized activation via metabolic and epigenetic reprogramming.76 Another study found significant heterogeneity and cross-regulation in macrophage transcriptomic networks in response to combined LPS, IFN-γ, and IL-4 stimulation.69 Capturing complex stimulation-induced signaling network changes poses an important technical challenge in the bioinformatics field.62
MACSPECTRUM AND ATHEROSPECTRUM AS TOOLS TO DISSECT MACROPHAGE FUNCTION IN HUMAN DISEASE
Therefore, because no existing transcriptomic annotation program could capture the full spectrum of macrophage activation states in vivo, we sought to develop improved characterization methods facilitating identification in human disease based on functional characteristics. To allow the representation of the full-resolution continuum of cell states and subpopulations, we used a novel approach to annotate macrophages based on function-relevant gene sets to generate the cell matrix, followed by visualization in low-dimensional space15,58 (Figure 3).
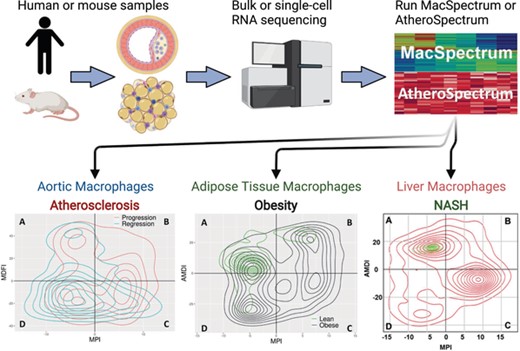
MacSpectrum and AtheroSpectrum workflow. Gene expression data from human or mouse monocyte-derived cells is input into the web tool or R package. MacSpectrum and AtheroSpectrum will calculate the MPI, AMDI, or MDFI scores to indicate the polarization, activation state, or foaming state of the cells, allowing for further characterization of cells and subpopulations
MacSpectrum, the first macrophage annotation tool we developed, depicts macrophage function on 2 indices: the Macrophage Polarization Index (MPI) and the Activation-induced Macrophage Differentiation Index (AMDI). MPI represents the inflammatory status of each cell based on polarization toward either the M1-like or M2-like extreme. AMDI represents maturation ranging between monocytes in an “M0” state and mature, maximally polarized M1-like or M2-like macrophages. Each cell analyzed in MacSpectrum is given MPI and AMDI scores, after which they are plotted along the MPI and AMDI axes. Cells are divided into quadrants distinguishing individual function-based subpopulations: M1-like or M2-like mature macrophages, transitional monocyte-macrophages, and preactivation monocyte–macrophages. The full methodology for developing MacSpectrum was described in detail in the initial publication,15 but we will give a brief description here.
To generate an index that could capture dynamic differences in macrophage polarization between subpopulations, we used scRNA-seq profiles from in vitro polarized primary bone marrow-derived macrophages stimulated under various polarization conditions: LPS + IFNγ for M1, IL-4 + IL-13 for M2, unstimulated for M0. First, we identified a gene set (termed “Polarization Signature Genes”) consisting of the top 500 genes that could separate M1- and M2-polarized cells using differentially expressed genes that were preferentially expressed in either phenotype. We then used linear regression to create a “polarization axis” based on the correlation of each of the polarization signature genes to the M1 and M2 average expression for each gene, after which the adjusted distance between each cell and the polarization axis was used to generate an MPI score. M1 and M2 samples separated well along the axis, and classic markers were expressed in a pattern consistent with their MPI values (high MPI scores are M1-like cells; low MPI scores are M2-like cells).15
The AMDI was generated in a similar manner to MPI. M0 gene expression was compared with M1 and M2 gene expression (representing mature, maximally polarized macrophages), excluding polarization signature genes. A signature gene list of 435 genes was developed, and a score was calculated based on the distance to the axis. These signature genes were enriched for cell cycle and macrophage development functions, consistent with macrophage maturation. We then combined the algorithms for calculating MPI and AMDI into the MacSpectrum program.15
To better characterize the functional heterogeneity of macrophages in atherosclerotic plaques, we extended MacSpectrum to depict foam cell development, creating a novel program called AtheroSpectrum.58 AtheroSpectrum depicts foam cell development on the Macrophage-Derived Foam cell Index (MDFI), which was developed similarly to MPI in MacSpectrum. Foaming signature genes were identified by comparing 2 sets of reference transcriptome profiles generated from arterial plaque macrophages from mice on atherogenic or normal chow diets.58,59 Any foaming signature genes overlapping with the polarization signature genes or activation-induced macrophage differentiation signature genes were excluded. To characterize macrophage inflammation states, we retained the MPI.
MacSpectrum and AtheroSpectrum generate relative information to be compared within each study, meaning that the MPI, AMDI, and MDFI scores for each sample represent activation features unique to each individual analysis. While this prevents direct comparison of these scores between studies, this design provides the ability to analyze data from multiple species, platforms, and operators without concern for batch effects, which are a critical challenge in the bioinformatics field.77,78 We anticipate the application of MacSpectrum and AtheroSpectrum between studies will be supported in the near future as robust batch correction algorithms are developed.
APPLICATION OF MACSPECTRUM AND ATHEROSPECTRUM
We applied MacSpectrum to scRNA-seq data from purified ATMs from diet-induced obese or normal chow diet lean mice. After calculating MPI and AMDI scores, we observed clear separation of the ATMs into preactivation, transitional, M1-like, and M2-like subpopulations. ATMs from lean tissue were predominantly in the M2-like region with high AMDI and low MPI, and ATMs from obese mice displayed a more diverse profile, with cells clustered in each region. Obese ATMs had a larger proportion of cells with high MPI scores, consistent with the inflammatory state seen in obesity. To further validate MacSpectrum, we used it to evaluate human datasets related to obesity: using signature gene lists identified from subpopulation analysis on MacSpectrum data, we analyzed genes from circulating monocytes from (1) obese human patients with or without diabetes and (2) obese human patients before and after bariatric surgery. With this strategy, we identified genes expected to be involved in the early stages of obesity-related inflammation.15
AtheroSpectrum successfully identified subpopulations of both inflammatory and noninflammatory foam cells and nonfoam cells in human and mouse monocytes and macrophages. Using AtheroSpectrum, we demonstrated the transition states between infiltrating monocytes, polarized macrophages, and macrophage-derived foam cells. From this analysis, we delineated the novel concept of homeostatic and pathogenic foaming programs in macrophages. We then applied these insights into macrophage cellular programs to build a predictive model for cardiovascular events.58
In MacSpectrum and AtheroSpectrum, we have created robust programs that enable mapping of the spectrum of monocyte and macrophage polarization, maturity states, and foaming in multiple disease contexts. MacSpectrum and AtheroSpectrum can be applied to both human or mouse gene expression data, including sequencing, microarray, or RT-qPCR, at either the bulk or single-cell level. We and others have successfully used these tools on gene expression data from monocytes, macrophages, and dendritic cells from a variety of tissues and diseases. They can be applied to monocyte/macrophage gene expression data from any setting, including purified cells, monocytes/macrophages identified in whole-tissue scRNA-seq, and other contexts.
CONCLUDING REMARKS
The development of high-throughput scRNA-seq technologies has empowered researchers with the resolution necessary to dissect the multifaceted functions of macrophages in human disease. Both obesity and CVD pathogenesis are heavily influenced by dynamic and heterogeneous populations of macrophages. In order to understand their role in disease, we created a novel set of computational tools to characterize macrophage function. With these tools, macrophage polarization, development, and foam cell formation can easily be interpreted from gene expression data.
MacSpectrum and AtheroSpectrum have been published both as free web tools and as R packages (https://macspectrum.uconn.edu), and they have since been used to further evaluate macrophages in a number of disease conditions, including obesity,79,80 diabetes,81 pulmonary fibrosis,82 liver disease,83,84 cancer,85,86 and interstitial cystitis 87 in addition to our own work on CVD 58 and adipose tissue thermogenesis.88 In the future, we hope that these tools are applied to characterize macrophage functions in additional pathologies. Additional indices could be developed to further describe macrophage actions; for example, components describing monocyte infiltration or immune metabolic profiles could be useful to researchers. These tools provide function-based evaluation of diverse monocyte-macrophage data, improving the capacity to identify disease-relevant subpopulations and cell states.
ACKNOWLEDGMENTS
The projects cited in this review were funded by American Heart Association grant 19TPA34910079 and National Institutes of Health/National Institute of Diabetes and Digestive and Kidney Diseases R01DK121805 to B. Z. All figures were created with BioRender.com. Figure 2 was adapted from “Blood Vessel and Interstitium” template, by BioRender.com (2022); retrieved from https://app.biorender.com/biorender-templates.
AUTHORSHIP
K. K. wrote the manuscript and prepared the figures; L. Q., A. J. M., and B. Z. assisted with editing and figure preparation. All authors have reviewed the manuscript.
DISCLOSURE
The authors declare no conflicts of interest.
REFERENCES
Abbreviations
- AMDI
Activation-induced Macrophage Differentiation Index
- ATM
adipose tissue macrophage
- BMDM
bone marrow-derived macrophages
- CVD
cardiovascular disease
- MDFI
Macrophage-Derived Foam cell Index
- MMP
matrix metalloprotease
- MPI
Macrophage Polarization Index
- NASH
nonalcoholic steatohepatitis
- scRNA-seq
single-cell RNA sequencing
- VAT
Visceral adipose tissue
Author notes
Summary sentence: Review of the current models to understand dynamic actions of macrophages in various diseases and new strategies to characterize their molecular network using single-cell transcriptomics.