-
PDF
- Split View
-
Views
-
Cite
Cite
Kevin Y Yang, Manching Ku, Kathy O Lui, Single-cell transcriptomics uncover distinct innate and adaptive cell subsets during tissue homeostasis and regeneration, Journal of Leukocyte Biology, Volume 108, Issue 5, Nov 2020, Pages 1593–1602, https://doi.org/10.1002/JLB.6MR0720-131R
- Share Icon Share
Abstract
Recently, immune cell-mediated tissue repair and regeneration has been an emerging paradigm of regenerative medicine. Immune cells form an essential part of the wound as induction of inflammation is a necessary step to elicit tissue healing. Rapid progress in transcriptomic analyses by high-throughput next-generation sequencing has been developed to study gene regulatory network and establish molecular signatures of immune cells that could potentially predict their functional roles in tissue repair and regeneration. However, the identification of cellular heterogeneity especially on the rare cell subsets has been limited in transcriptomic analyses of bulk cell populations. Therefore, genome-wide, single-cell RNA sequencing (scRNA-Seq) has offered an unprecedented approach to unravel cellular diversity and to study novel immune cell populations involved in tissue repair and regeneration through unsupervised sampling of individual cells without the need to rely on prior knowledge about cell-specific markers. The analysis of gene expression patterns at a single-cell resolution also holds promises to uncover the mechanisms and therefore the development of therapeutic strategy promoting immunoregenerative medicine. In this review, we will discuss how scRNA-Seq facilitates the characterization of immune cells, including macrophages, innate lymphoid cells and T and B lymphocytes, discovery of immune cell heterogeneity, identification of novel subsets, and tracking of developmental trajectories of distinct immune cells during tissue homeostasis, repair, and regeneration.
1 Introduction
Since decades, the immune system has been well studied for its central role in maintaining tissue homeostasis through the regulation of tissue repair after injury as well as replacement of aged tissues. In addition to tissue-resident immune cells, inflammation recruits more immune cells from the circulation to the injured site whereby they interact with nonimmune cells such as stem cells of the niche to mediate repair and regeneration (for review, see Ref. 1). The immune cell-mediated tissue healing processes are complicated with both positive and negative regulators as the latter is essential for minimizing tissue damage as a result of an overreactive immune response.2 For instances, we have recently reported that overactive CD4+ Th13,4 and CD8+ cytotoxic5 T cells are detrimental for cardiovascular regeneration, whereas CD4+FOXP3+ regulatory T cells (Treg) directly promote vascular3 and heart muscle6 regeneration. Therefore, the heterogeneous immune cell populations orchestrate tightly together and their deficient or dysregulated interactions can lead to impaired healing and pathologic fibrosis. In some tissues such as the skin, scar tissue formation is part of the healing process, whereas in other tissues such as the heart, existence of noncardiac tissues such as the scar could contribute to arrhythmia and eventually heart failure. Therefore, tissue repair and regeneration require tipping the balance of immune response (for review, see Refs. 1,7). Nevertheless, the roles of various immune cells, characterization of their subsets, as well as the molecular mechanisms involved in tissue-specific repair and regeneration remain poorly understood.
In fact, accumulating evidence suggests that many transcriptional programs are activated in immune cells during tissue repair and regeneration.8 The precise temporal and spatial regulation of immune response after injury determines the fate of the tissue. For example, a temporal shift of macrophage subsets has been found in the injured mouse skin with M1 macrophages being dominated at an earlier stage (day 7) that express high levels of proinflammatory cytokines (e.g., TNFα, IL-1, and IL-6), whereas the majority at the later stage (day 35) was M2 macrophages that express high levels of anti-inflammatory cytokines (e.g., IL-10, vascular endothelial growth factor, and TGFβ).9 On the other hand, the dermis and epidermis of the skin house distinct immune properties. The dermis comprises dendritic cells, macrophages, mast cells, and neutrophils, whereas the epidermis includes dendritic cells, NK T-cell, γδ T cells, and αβ T cells. These cells contribute distinctively during skin homeostasis and regeneration. The activation of M1 macrophages in the dermis produces proinflammatory cytokines that can activate antigen presentation and phagocytosis, whereas γδ T cells and αβ T cells in the epidermis maintain the homeostasis of the skin by recognition and clearance of dead cells.10 Deciphering such transcriptomes in bulk immune cells would provide a wealth of information for better understanding the mechanisms involved with analysis on their regulatory gene network. Multiomics analysis on a single-cell level can also uncover the cellular and functional heterogeneity of these cells. However, traditional single-cell approaches including flow cytometry or immunofluorescence have suffered from limited information obtained from few RNAs or proteins; and prior knowledge about cell-specific markers is needed to assist data interpretation.11 On the other hand, analysis on bulk transcriptomic profiles derived from the sorted subpopulations is also limited by the requirement of prior knowledge to predict cell identity of the heterogeneous populations, and the difficulty in acquiring sufficient RNA materials from small numbers of cells.11
Since 2009 when the first study has been published,12 the throughput of scRNA-Seq expanded exponentially from hundreds to tens of thousands of cells, using plate-based (e.g., STRT-Seq,13 Smart-Seq,14 Smart-Seq2,15 and CEL-Seq216) or droplet-based microwell (e.g., Drop-Seq,17 InDrop,18 10x Chromium Genomics,19 Seq-well,20 and sci-RNA-Seq21) technologies. Typically, protocols for using these scRNA-Seq technologies involve the following steps (after tissue injury): (1) tissue/cell dissociation by enzymatic digestion or manual disaggregation, (2) optional enrichment of cell types by flow cytometry or bead-based immune-selection, (3) scRNA-Seq libraries preparation and sequencing, and (4) computational analysis. Each protocol has different features and performance efficiencies that contribute to both benefits and limitations.22 For example, in terms of the captured transcript coverage, these protocols can be further divided into 2 categories: (1) full-length transcript sequencing approaches (Smart-Seq and Smart-Seq2) and (2) tag-based 3′- or 5′-end transcript sequencing approaches (STRT-Seq, CEL-Seq2, Drop-Seq, InDrop, 10x, Seq-well, and sci-RNA-Seq). The former sequences the full length of the transcripts, allowing further detection of splice variants, alternative transcripts, as well as fusion transcripts.23 With the full length of transcripts, it is possible to obtain the genotypes of T and B cell receptors from the transcriptome.24 However, the overall cost and labor requirement for these full-length methods has also increased as early cellular barcoding and pooling during library preparation is not allowed. On the other hand, the latter methods sacrifice the coverage but only sequence the 5′ or 3′ of the transcripts, allowing multiplexing the cDNA amplification and increasing the throughput of scRNA-Seq. Although the overall sensitivity is reduced, these tag-based methods are now widely used in studies of cell-type discovery and comparison. Altogether, these new methods allow researchers to investigate the potential functional roles of many genes in individual cells at the same time, facilitating the characterization of the heterogeneity of immune cells, identification of novel or rare immune cell subtypes, analysis of gene regulatory network, and reconstruction of the developmental trajectory of immune cells during tissue repair and regeneration. To date, the number of publications using scRNA-Seq for immune cell studies has also been increasing yearly since 2016 (Figure 1). In the following sessions, we will summarize some of these works with a particular focus on immune cell-mediated repair and regeneration of various tissues including the heart, lung, gut, skeletal muscle, CNS, and skin.
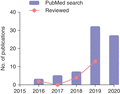
An overtime increase in the number of publications related to the use of scRNA-Seq in studying immune cell-mediated tissue homeostasis, repair, and regeneration. The blue bars show the number of publications indexed in NCBI PubMed per year after searching for key words. The pink dots show the number of publications that are summarized in this review
2 Understanding the Heterogeneity of Tissue-Resident Immune Cells in Homeostasis
Large body of evidence has demonstrated the critical role of immune cells in nonlymphoid tissues. In fact, both innate and adaptive cells have been found residing in tissues for the maintenance of homeostasis.25 Recently, studies using scRNA-Seq have uncovered a large amount of transcriptional information from small amounts of RNAs that reshapes our understanding of cellular composition and plasticity of various immune cell types in different tissues, including the heart,26–28 liver,29 lung,30,31 and skin.32 For instance, Skelly et al.26 have characterized the transcriptional profiles of individual cells of the murine ventricular nonmyocytes and identified 12 distinct cell clusters including lymphocytes, macrophages, and dendritic cell-like cells. Based on the gene expression patterns, the authors subclustered these populations and found at least 5 subtypes of macrophages, including Lyve1+Ccr2−H2-Aalo, Lyve1−Ccr2+H2-Aahi, Lyve1−Ccr2−H2-Aalo, Lyve1−Ccr2−H2-AahiTlr2hi, and Lyve1−Ccr2−H2-AahiLira5hi.26 Among them, a subset exhibits a hybrid molecular signature of both macrophages (expressing Col1a1, Pdgfra, and Tcf21) and fibroblasts (expressing Fcgr1, Cd14, and Ptprc), implying the multifaceted feature of macrophages responding to a diverse range of stimuli.26 This study offers unique insights into the structure and function of the cardiac cellulome and provides a new database for in-depth investigation of the immunity during heart development, homeostasis, and disease.
Indeed, macrophages are pivotal players in maintaining tissue homeostasis. Tissue-resident macrophages originate from the yolk sac or fetal liver during development and persist in many tissues after self-renewal.33 It plays a predominant role in the restoration of tissue homeostasis by clearing away cellular debris, remodeling the extracellular matrix, and synthesizing multiple cytokines and growth factors essential for tissue repair (for review, see Refs. 33,34). Recent studies have employed single-cell transcriptomics to identify distinct tissue-specific macrophage populations. For example, Dick et al.35 have paired Ccr2−/− mice that lack circulating Ly6Chi monocytes with Ccr2+/+ mice that carry a constitutively expressed TdTomato reporter in the Rosa26 locus (Rosa26mTmG/mTmG) for 6 months through parabiosis. The authors found that >80% CCR2+ and ∼25% CCR2−MHC-IIhi macrophages of the heart were replaced by macrophages derived from Ccr2+/+ monocytes of the circulation, whereas CCR2−MHC-IIlo macrophages were not markedly replaced.35 They further sorted CD45+CD64+CD11b+ macrophages and CD45+CD64−CD11c+MHC-IIlo-hi dendritic cells from the adult murine heart for scRNA-Seq analysis. Their results demonstrated that, at steady state, 4 populations of macrophages exist in the murine healthy adult myocardium, including a TIMD4+LYVE1+MHC-IIloCCR2− subset that is maintained independently from blood monocytes, a TIMD4−LYVE1−MHC-IIhiCCR2− subset and 2 MHC-IIhiCCR2+ subsets that are partially or fully replaced by monocytes. Moreover, the authors also identified 3 similar subsets of cardiac macrophages in humans based on expression of MHC-II and CCR2. Similarly, Chakarov et al.36 have used the scRNA-Seq and fate-mapping technologies to interrogate the heterogeneity, interrelationships, and ontogeny of murine lung resident tissue macrophages. The authors identified 2 different interstitial macrophage populations, namely LYVEloMHC-IIhiCX3CR1hi and LYVE1hiMHC-IIloCX3CR1lo, in addition to alveolar macrophages (AMs). They further used the S100a4Cre-RosaEYFP lineage tracing mice to demonstrate that both populations are slowly replaced by LY6Chi monocytes since birth till 20 weeks of age. By using a mouse model that allows temporal ablation of macrophages during induction of fibrosis, the authors found that depletion of LYVE1hiMHC-IIloCX3CR1lo cells exacerbate lung fibrosis, suggesting the critical role of these cells in tissue inflammation.36 Intriguingly, similar findings can also be observed in the gut. De Schepper et al.37 have identified a small self-maintaining population of gut macrophages that was not replenished by circulating monocytes in the lamina propria and muscularis externa during homeostasis. The difference in ontogeny, rate of replacement from the circulation, and capacity for self-renewal may imply that various macrophage subsets likely play distinct roles in tissue homeostasis.33
In addition, the adaptive immunity particularly subsets of T cells also plays a critical role in maintaining tissue homeostasis. The classical view of adaptive immunity is defined by the presence of lymphocytes, either T or B cells, which circulate continuously between the blood and lymph. In contrast to this view, efforts of the past decade have led to the characterization of lymphocyte populations permanently residing in nonlymphoid organs, including tissue-resident memory T cells, unconventional T cells (e.g., invariant NK cells, mucosal-associated invariant T cells, and γδ T cells), and a diverse family of innate lymphoid cells (ILC).38 Particularly, ILCs have been increasingly considered as important participants in homeostasis and the course of inflammation. Through scRNA-Seq, Björklund et al.39 have performed unbiased transcriptional clustering to delineate the heterogeneity of human Lin−CD127+ ILCs and CD45+Lin−CD127−NKG2A+CD56+CD16− NK cells isolated from tonsil tissue. There are 4 distinct populations that correspond to ILC1, ILC2, ILC3, and NK cells as demonstrated by their elevated expression of specific transcripts, that is, CXCR3, GATA3, RORC, and GZMA, respectively. Moreover, profiling at the single-cell resolution additionally categorizes ILC3 cells into 3 diverse subsets including the NKp44+, CD62L+, and HLA-DR+ subsets, which showed distinct functionality as revealed by simultaneous intracellular cytokine detection after stimulation.39 Furthermore, a sophisticated study by Gury-BenAri et al.40 have reported the heterogeneity of CD127+ ILCs isolated from the small intestine of RORγt-GFP reporter mice using scRNA-Seq together with chromatin immunoprecipitation-sequencing and assay for transposase-accessible chromatin-sequencing (ATAC-Seq). In addition to the known ILC subsets respectively expressing NKp46 (ILC1), RORγt (ILC2), and IFNγ (ILC3), there are 2 novel CD127+ ILC subsets that express IL-2 or CCL22.40 Altogether, these preliminary studies uncover the heterogeneity of immune cells of both the innate and adaptive arms in the maintenance of tissue homeostasis. Future experiments are needed to validate the function of these new subsets in healthy tissues as well as their potential role, if any, in pathologic conditions.
3 Understanding the Heterogeneity of Immune Cells in Tissue Repair and Regeneration
Regeneration is a process coordinated by multiple cell types including the immune cells to repair or replace the damaged tissue. Successful regeneration involves multiple steps, such as activation of endothelial cells for recruitment of immune cells, removal of cellular debris, proliferation and differentiation of stem cells, and integration of newly formed cells into existing tissues.41 Unfortunately, mammals possess a limited regenerative capability that is often seen in some tissues such as the skin and liver but not in others such as the heart.42 After injury, inflammation recruits immune cells that interact with both the damaged and healthy cells at the wound site, leading to a cascade of events that further determine the fate of the damaged tissue.43 However, persistent inflammation or other inappropriate immune response can lead to fibrosis and scaring that can interfere the repair and regenerative process.44 Therefore, the immune system acts as a double-edged sword and exhibits complementary or inhibitory functions during regeneration.45 Recently, much research attention focuses on how immune cells contribute to tissue repair and regeneration. In this section, we will summarize the studies that utilize scRNA-Seq in examining the role of immune cells in tissue-specific repair and regeneration.
3.1 Heart
In contrast to the lower vertebrates, zebrafish, or neonates of the mouse and pig, the adult mammalian heart is notorious in its inability to regenerate after injury.46–48 Recent evidence has demonstrated that the neonatal mouse heart fails to regenerate after ablation of macrophages with fibrotic scar formed after injury, resulting in reduced cardiac function and angiogenesis.49 Therefore, it is believed that macrophages provide the necessary signals to drive neonatal heart regeneration.50 As aforementioned, Dick et al.35 have dissected the cellular heterogeneity of cardiac macrophages during homeostasis by combing single-cell transcriptomics and cell fate mapping studies. They identified 7 unique clusters of macrophages in the myocardium after myocardial infarction (MI) that occupy about 64% of the sequenced CD45+CD11b+CD64lo-hi macrophages, indicating that the transcriptional signatures of the majority of macrophages are distinct from those of the noninfarcted heart tissue.35 In another study, Farbehi et al.28 have performed scRNA-Seq on the immune and stromal (noncardiac muscle) cells of the murine adult hearts at days 3 and 7 after sham or MI surgery. The authors found the major tissue-resident and infiltrating macrophages, namely the Ccr2+ and Ccr2− tissue-resident macrophages, proinflammatory M1 macrophages at day 3, nonclassical M2 macrophages at day 7, and a few minor populations.28 Their data, combined with the communication map of other cell types, provide a preliminary framework for further investigation on the intersubset relationship in homeostasis and in response to heart injury.28
In addition to macrophages, a recent study by Vafadarnejad et al.51 has also revealed the temporal heterogeneity of another innate immune cell population, the CD64−LY6G+ neutrophil, at days 1, 3, and 5 after MI. The authors demonstrated that neutrophils can be subclustered into 6 distinct subsets with respect to specific time points after injury. They further performed scRNA-Seq on the total CD45+ cells at days 0, 1, 3, 5, and 7 after MI, from which they observed an enrichment of Siglecf expressing neutrophils at days 3 and 5 after MI. They have validated the result by flow cytometry and found that the LY6G+SIGLECFhi neutrophils were absent in the bone marrow, spleen, and blood, suggesting a local transition after injury. These results constituted valuable resources for further investigation on the temporal heterogeneity with functional implications of neutrophils in the infarcted myocardium.51
Besides, adaptive immune cells especially the Treg have been recently uncovered as one of the essential regulators of organ regeneration (for review, see Refs. 1,52). Our recent study has shown that T-cell deficient NOD/SCID mice failed to regenerate their injured myocardium at as early as postnatal day (P) 3 after cryoinfarction.6 We further demonstrated that specific ablation of Treg in the Foxp3-DTR mice following administration of diphtheria toxin inhibits neonatal heart regeneration, whereas adoptive transfer of hCD2+ Treg from the Foxp3hCD2 mice to NOD/SCID mice promotes the regeneration.6 Moreover, we purified hCD2+ Treg from the regenerating and nonregenerating hearts for transcriptomic profiling of individual cells as well as in vitro coculture with neonatal cardiomyocytes, and observed that they promoted neonatal cardiomyocyte proliferation in a paracrine manner.6 More recently, we have also demonstrated that specific ablation of CD4+ cells but not CD8+ cells using depleting antibodies rescues juvenile heart regeneration.4 Our data suggest a regulatory role of CD4+ cells in neonatal/juvenile heart regeneration.
Furthermore, Martini et al.27 have analyzed the immune cell heterogeneity of CD45+ cells in the murine heart with pressure overload by scRNA-Seq and flow cytometry. Their results showed that immune activation of many cell types including neutrophils, B cells, NK cells, and mast cells occurs following heart injury; and up-regulation of oncostatin M in proinflammatory macrophages and PD-1 in Treg might serve as potential therapeutic targets for treatment of human heart failure.27 All these studies highlight the complexity and plasticity of the resident or recruited immune cells following tissue injury, repair, and regeneration. Better understanding of this heterogeneous dynamic immune process would shed light on the development of novel therapeutic strategies targeting heart repair and regeneration.
3.2 Skeletal muscle
Inflammation following muscle injuries such as trauma is a common feature of the repair and regenerative process.53 It is clear that a complex and coordinated interaction between muscle and immune cells can determine the outcome of tissue regeneration (for review, see Ref. 53). A recent study by Pawlikowski et al.54 have provided the first cellular and transcriptomic landscape of individual muscle cells at the preinjury, postinjury, and aged stages. The authors demonstrated that a heterogeneous macrophage and T cell populations change dramatically during muscle repair. They also uncovered that the transcriptional profiles of muscle stem cells and T cells of aged muscles are similar to that of injured tissues, respectively. This work represents an example suggesting the transcriptional information at a single-cell resolution facilitates the identification of novel cell subsets and the molecular signals involved could potentially implicate strategies for driving muscle regeneration.54
3.3 Lung
The adult lung has a remarkable potential of regeneration.55,56 Immune cells, particularly macrophages, are well recognized for their roles in orchestrating inflammatory responses and regulating lung tissue repair following injury.57 To identify the heterogeneity of inflammatory AMs, Mould et al.57 have performed transcriptional profiling of individual AMs marked as DAPI−LY6G−F480+CD64+ within the murine lung at days 0 (homeostasis), 3 (peak neutrophilic inflammation), and 6 (resolution of inflammation) after injury. The authors identified 5 transcriptionally distinct clusters during lung homeostasis, peak of inflammation, and resolution of inflammation. They also identified resident and recruited AMs within these subsets with respect to their expression of known marker gene sets such as Mrc1, Itgax, SiglecF, and Siglec1, and Cd14, Ly6c1, ApoE, Ccr5, Mafb, and Sell, respectively.57 They further revealed 2 previously uncharacterized subpopulations of AMs that could be specialized in the proliferation and inflammatory processes.57
Fibrosis is part of the tissue healing process that is mainly governed by anti-inflammatory responses.58 Reyfman et al.30 have investigated the cellular diversity of human lung tissues derived from 8 transplant donors, 8 recipients with pulmonary fibrosis, and 2 normal mice. The authors identified different cell types including alveolar type I/II cells, epithelial cells, fibroblasts, AMs, and some immune cells. They also found fibrosis-associated transcriptomic changes in macrophages, alveolar type II cells and fibroblasts isolated from normal and fibrotic lungs, respectively.30 More recently, the same research team performed single-cell transcriptomic profiling with lung tissues derived from the wild-type or treatment group in which mice were exposed to either asbestos or titanium dioxide (TiO2) for 14 days to induce fibrosis.59 By focusing analysis on the Cd68, Mrc1, Lyz2, Adgre1, and Axl-expressing macrophage populations, they identified AMs and tissue-resident interstitial macrophages as the predominant macrophage population. The latter population could be further subcategorized into Ccr2 expressing peribronchial and Lyve1 expressing perivascular macrophages. On the other hand, AMs contained 3 subsets that express gene sets correlated to normal homeostatic function (Ear1, Fabp1), inflammatory response (Car4, Ctsk, Chil3, S100a1, Wfdc21), and immature phenotype (Itgam, Cd36, Gpnmb). They have validated these results by in situ RNA hybridization, and found that Csf1r, encoding for M-CSFR, was up-regulated in the immature subset that was further detected in the fibrotic areas in proximity to Pdgfra+ fibroblasts. Indeed, blocking the M-CSF signaling pathway led to disappearance of this population, suggesting that monocyte-derived AMs are persistent in the fibrotic niche through autocrine regulation of the M-CSF/M-CSFR signaling pathway.59
3.4 CNS
Damage to the mammalian CNS leads to neuronal cell death, axon degeneration, and formation of a glial and fibrotic scar that can ultimately results in functional and behavioral defects. Other vertebrates, such as the fish and salamander, have retained the ability to functionally regenerate the CNS by both the innate and adaptive arms of immunity after injury (for review, see Ref. 60). To gain more precise insights into the role of immunity in the regulation of development, homeostasis, disease, and degeneration of the CNS, scRNA-Seq has been widely used in recent years. For instances, Hammond et al.61 have analyzed the RNA expression patterns of the murine Cd45loCd11bhiCx3cr1hi microglia, the resident immune cells of the brain, derived from early embryos, neonates, adults (100 days), and aged (540 days) mice after brain injury. They uncovered at least 9 transcriptionally distinct microglial states across various development stages and the microglia re-expressed developmental markers indicating that there could be a reversion of mature to immature microglia in response to brain injury.61 Moreover, Masuda et al.62 have combined scRNA-Seq analysis, single-molecule fluorescence in situ hybridization, advanced immunohistochemistry, and computational modeling to comprehensively characterize the subsets of microglia at a single-cell resolution in multiple regions of the murine brain including the forebrain, midbrain, cerebellum, spinal cord, cortex, corpus callosum, hippocampus, and facial nucleus during development and disease. Their data revealed that there are transcriptomically distinguishable subpopulations of microglia during homeostasis but they did not appear as distinct clusters, indicating their ability to swiftly adapt to a changing environmental cue. Indeed, there were disease-specific subtypes of microglia that differed between neurodegenerative conditions and toxic demyolination.
It remains controversial about the role of resident and infiltrating immune cells during neurodegeneration, which is often accompanied by neuroinflammation. Ronning et al.63 have used the Arr1−/− mouse model, which is a light-induced photoreceptor degeneration model, to study the heterogeneity of immune responders within 48 h of the onset of rod degeneration. The authors purified CD11b+CD45+ microglia and monocytes from the retinas of healthy dark-reared control and light-exposed Arr1−/− littermates for scRNA-seq analysis. They found that almost all immune cells of the control retinas were resident microglia that were mostly of the resting stage, whereas the majority of immune cells isolated from the degenerating tissues were of the peripheral origin. Interestingly, they found that MHCII+ monocytes/macrophages formed prominent clusters but validation by flow cytometry did not show localized MHCII+ cells in any particular layer of the retina, suggesting that monocyte-derived macrophages did not rapidly accumulate in the photoreceptor layers. These results also highlight the importance of protein and functional validation before conclusion can be drawn from interpretation of the scRNA-seq data.
3.5 Skin
The skin is the largest organ of the body that provides a variety of defensive strategies to protect the host from invading pathogens. Several types of resident immune cells have been reported to serve as sentinels in response to local inflammation of the skin during the event of an insult.64 Four highly orchestrated phases of wound healing are operated by immune cells, namely the hemostasis, inflammation, proliferation, and remodeling (for review, see Ref. 64). Guerrero-Juarez et al.65 have demonstrated through scRNA-Seq analyses that the cells of the skin wound were heterogeneous consisting of at least 12 different subsets, including a subset of fibroblasts expressing hematopoietic markers (e.g., Lyz2) that suggest their myeloid origin. In addition, Cheng et al.32 have studied the transcriptomic profiles of individual human epidermal cells derived from 9 normal and 3 inflamed skin samples. The authors identified the molecular fingerprints of the inflammatory states, including enrichment of CD1C+CD301A+ myeloid dendritic cells and IL1βhiCCL3hiCD14+ monocyte-derived macrophages.32 Taken together, single-cell transcriptomics facilitate researchers to understand the cellular dynamics and plasticity of immune cells operated during skin repair.
4 Reconstructing the Temporal Dynamic of Immune Cells in Tissue Repair and Regeneration
In addition to studying the cellular heterogeneity of different tissue atlases, scRNA-Seq also allows reconstruction of the temporal dynamic of a specific biologic process. For instance, a snapshot of cell states is generated during single-cell sampling of a tissue for revealing regions occupied by mature and transient cell types.66 Moreover, profiling individual cells with scRNA-Seq enables the characterization of intermediate cell states and, therefore, cellular transition dynamics or so-called “trajectories.” To do this, methods such as single-cell trajectory inference and pseudotime estimation have been emerged.66 Since its first demonstration in 2014,67,68 more than 50 trajectory inference methods have been developed for reconstructing the trajectories from single-cell transcriptomic data. These methods order single cells along the deterministic69–71 or probabilistic72–74 trajectories, and assign each cell with a numeric value (pseudotime), which demonstrates how far a cell has progressed along the process. Unlike other live cell approaches that track the change of cellular events within a single cell, reconstruction of trajectory using scRNA-seq requires the comparison of variation among multiple cell types or cell states within the measured population.66 Saelens et al.75 have comprehensively evaluated 45 trajectory inference tools on real and synthetic datasets for cellular ordering, topology, scalability, and usability and provided a guideline for users to choose the correct method for making trajectory inference that should be primarily based on the anticipated trajectory topology of a given scRNA-Seq dataset.75
Furthermore, reconstruction of the temporal dynamic of individual cells enables the investigation of many immune-related biologic processes, including the development and migration of various immune cells in response to self-antigens during tissue homeostasis, repair, and regeneration. For instances, Miragaia et al.76 have profiled individual CD4+FOXP3+ Treg and CD4+FOXP3−CD44hi memory T cell derived from both the lymphoid (e.g., lymph nodes and spleen) and nonlymphoid (skin and colon) tissues to study adaptation. Psuedotime ordering for reconstruction of trajectories demonstrated a similar regulatory sequence in the recruitment of Treg from the lymph nodes to the skin and colon; and a tissue-specific response in the priming stage in lymph nodes and the final stages of functional adaptation in localized tissues. Moreover, Dick et al.35 have also employed the Monocle and Mpath algorithms to temporally order 4 cardiac macrophage populations with monocytes in order to determine whether hypothetical developmental relationships exist between these clusters in the healthy myocardium. The trajectories revealed that the cluster enriched with many interferon-stimulated genes (Isg cluster) could arise from the Ccr2 cluster (CCR2+ macrophages) instead of circulating monocytes. Further investigation of gene expression along the pseudotime further showed that the Ccr2, Mhc-II, and Isg clusters could make up a spectrum of related lineages.
More recently, our group has also demonstrated the heterogeneity of CD8+ T cells in the skeletal muscles of healthy (Leprdb/+) and obesity-related diabetic (Leprdb/db) mice after ischemic injury.5 We showed by scRNA-Seq that there were 3 subsets of CD8+ T cells and trajectories inference by Monocle revealed 2 alternative fates of CD8+ T cells of healthy and diabetic mice in response to the ischemic injury: CD8+ T cells differentiated from an angiogenic, central memory precursor subset to a tissue-resident memory subset in healthy mice, whereas the angiogenic precursor subset is skewed to an effector and effector memory subset in diabetic mice. Although trajectory inferred from scRNA-Seq data could provide temporal information during many comprehensive immunologic processes, these transcriptome-based data should be validated by users to confirm expression at the protein level possibly with in vivo lineage tracing models to record their lineage commitment during immune cell development.77
5 Future Perspectives
Recently, much research attention has been given to explore the role of immune response after tissue injury as it regulates the balance between tissue inflammation, fibrosis, and repair. Development of proper immunomodulatory therapies might promote tissue repair and regeneration. However, several clinical trials targeting patients with heart diseases through immunosuppressive agents have failed to show any beneficial outcome,50 suggesting that an entire halt of postischemic inflammation is insufficient. To achieve this goal, it is required to identify specific immune cell subsets and understand their time course of actions after tissue injury.78
Advances in single-cell transcriptomic sequencing offer a unique opportunity for better understanding on the heterogeneity and developmental trajectory of specific and even rare immune cells during tissue repair and regeneration (Figure 2). In this review, we have discussed how scRNA-Seq facilitates the investigation of immune cell-mediated repair and regeneration of different organ tissues. However, not all subsets of immune cells have well-characterized function in tissue repair and regeneration (for reviews, see Table 1), which could be worthy of future investigations.
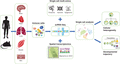
Understanding the heterogeneity and temporal dynamics of individual immune cells by scRNA-Seq. Immune cells can be isolated from any tissue organ of interest from human or mouse in homeostasis, disease, or regeneration. Individual cells are profiled by scRNA-Seq for understanding the cellular heterogeneity, identifying novel or rare subsets, and tracking developmental trajectory during the biologic process. scRNA-Seq can also be coupled with other single-cell technologies to study multiomics at the proteome, genome, or epigenome level; and/or combined with spatial transcriptomics analysis to study 3-dimensional localization of individual cells (the cartoon is adopted from Ref. 96). Altogether, scRNA-Seq offers an unprecedented opportunity to study the functional role of immune cells during tissue homeostasis, disease, and regeneration
. | . | Macrophages . | Dendritic cells . | Granulocytes . | T lymphocytes . | B lymphocytes . | Innate lymphoid cells . | NK . | Mast cells . |
---|---|---|---|---|---|---|---|---|---|
Heart | Skelly et al.26 | ✓ | ✓ | ✓ | ✓ | ✓ | ✓ | ✓ | |
Li et al.1 | ✓ | ||||||||
Dick et al.35 | ✓ | ✓ | |||||||
Farbehi et al.28 | ✓ | ✓ | ✓ | ✓ | |||||
Vafadarnejad et al.51 | ✓ | ✓ | |||||||
Martini et al.27 | ✓ | ✓ | ✓ | ✓ | ✓ | ✓ | ✓ | ||
Lung | Chakarov et al.36 | ✓ | |||||||
Mould et al.57 | ✓ | ||||||||
Reyfman et al.30 | ✓ | ✓ | ✓ | ✓ | ✓ | ✓ | |||
Joshi et al.59 | ✓ | ✓ | ✓ | ✓ | ✓ | ✓ | ✓ | ||
Gut | De Schepper et al.37 | ✓ | |||||||
Gury-BenAri et al.40 | ✓ | ||||||||
Skeletal muscle | Pawlikowski et al.54 | ✓ | ✓ | ✓ | ✓ | ||||
Tonsil | Björklund et al.39 | ✓ | ✓ | ||||||
Central nervous system | Hammond et al.61 | ✓ | |||||||
Masuda et al.62 | ✓ | ||||||||
Ronning et al.63 | ✓ | ||||||||
Skin | Cheng et al.32 | ✓ | ✓ | ✓ | |||||
Guerrero-Juarez et al.65 | ✓ | ✓ | ✓ |
. | . | Macrophages . | Dendritic cells . | Granulocytes . | T lymphocytes . | B lymphocytes . | Innate lymphoid cells . | NK . | Mast cells . |
---|---|---|---|---|---|---|---|---|---|
Heart | Skelly et al.26 | ✓ | ✓ | ✓ | ✓ | ✓ | ✓ | ✓ | |
Li et al.1 | ✓ | ||||||||
Dick et al.35 | ✓ | ✓ | |||||||
Farbehi et al.28 | ✓ | ✓ | ✓ | ✓ | |||||
Vafadarnejad et al.51 | ✓ | ✓ | |||||||
Martini et al.27 | ✓ | ✓ | ✓ | ✓ | ✓ | ✓ | ✓ | ||
Lung | Chakarov et al.36 | ✓ | |||||||
Mould et al.57 | ✓ | ||||||||
Reyfman et al.30 | ✓ | ✓ | ✓ | ✓ | ✓ | ✓ | |||
Joshi et al.59 | ✓ | ✓ | ✓ | ✓ | ✓ | ✓ | ✓ | ||
Gut | De Schepper et al.37 | ✓ | |||||||
Gury-BenAri et al.40 | ✓ | ||||||||
Skeletal muscle | Pawlikowski et al.54 | ✓ | ✓ | ✓ | ✓ | ||||
Tonsil | Björklund et al.39 | ✓ | ✓ | ||||||
Central nervous system | Hammond et al.61 | ✓ | |||||||
Masuda et al.62 | ✓ | ||||||||
Ronning et al.63 | ✓ | ||||||||
Skin | Cheng et al.32 | ✓ | ✓ | ✓ | |||||
Guerrero-Juarez et al.65 | ✓ | ✓ | ✓ |
. | . | Macrophages . | Dendritic cells . | Granulocytes . | T lymphocytes . | B lymphocytes . | Innate lymphoid cells . | NK . | Mast cells . |
---|---|---|---|---|---|---|---|---|---|
Heart | Skelly et al.26 | ✓ | ✓ | ✓ | ✓ | ✓ | ✓ | ✓ | |
Li et al.1 | ✓ | ||||||||
Dick et al.35 | ✓ | ✓ | |||||||
Farbehi et al.28 | ✓ | ✓ | ✓ | ✓ | |||||
Vafadarnejad et al.51 | ✓ | ✓ | |||||||
Martini et al.27 | ✓ | ✓ | ✓ | ✓ | ✓ | ✓ | ✓ | ||
Lung | Chakarov et al.36 | ✓ | |||||||
Mould et al.57 | ✓ | ||||||||
Reyfman et al.30 | ✓ | ✓ | ✓ | ✓ | ✓ | ✓ | |||
Joshi et al.59 | ✓ | ✓ | ✓ | ✓ | ✓ | ✓ | ✓ | ||
Gut | De Schepper et al.37 | ✓ | |||||||
Gury-BenAri et al.40 | ✓ | ||||||||
Skeletal muscle | Pawlikowski et al.54 | ✓ | ✓ | ✓ | ✓ | ||||
Tonsil | Björklund et al.39 | ✓ | ✓ | ||||||
Central nervous system | Hammond et al.61 | ✓ | |||||||
Masuda et al.62 | ✓ | ||||||||
Ronning et al.63 | ✓ | ||||||||
Skin | Cheng et al.32 | ✓ | ✓ | ✓ | |||||
Guerrero-Juarez et al.65 | ✓ | ✓ | ✓ |
. | . | Macrophages . | Dendritic cells . | Granulocytes . | T lymphocytes . | B lymphocytes . | Innate lymphoid cells . | NK . | Mast cells . |
---|---|---|---|---|---|---|---|---|---|
Heart | Skelly et al.26 | ✓ | ✓ | ✓ | ✓ | ✓ | ✓ | ✓ | |
Li et al.1 | ✓ | ||||||||
Dick et al.35 | ✓ | ✓ | |||||||
Farbehi et al.28 | ✓ | ✓ | ✓ | ✓ | |||||
Vafadarnejad et al.51 | ✓ | ✓ | |||||||
Martini et al.27 | ✓ | ✓ | ✓ | ✓ | ✓ | ✓ | ✓ | ||
Lung | Chakarov et al.36 | ✓ | |||||||
Mould et al.57 | ✓ | ||||||||
Reyfman et al.30 | ✓ | ✓ | ✓ | ✓ | ✓ | ✓ | |||
Joshi et al.59 | ✓ | ✓ | ✓ | ✓ | ✓ | ✓ | ✓ | ||
Gut | De Schepper et al.37 | ✓ | |||||||
Gury-BenAri et al.40 | ✓ | ||||||||
Skeletal muscle | Pawlikowski et al.54 | ✓ | ✓ | ✓ | ✓ | ||||
Tonsil | Björklund et al.39 | ✓ | ✓ | ||||||
Central nervous system | Hammond et al.61 | ✓ | |||||||
Masuda et al.62 | ✓ | ||||||||
Ronning et al.63 | ✓ | ||||||||
Skin | Cheng et al.32 | ✓ | ✓ | ✓ | |||||
Guerrero-Juarez et al.65 | ✓ | ✓ | ✓ |
It is important to note that there are dropout events in scRNA-Seq studies. Therefore, scRNA-Seq data could be incomplete and noisy, and the missing (zero) rate can reach over 80%.79 These zero values can be false negatives, meaning that genes even expressed at a relatively high level can be undetected due to technical limitations such as failure of amplification of the original RNA transcripts.80 These dropout events may lead to misinterpretation of data in the downstream analysis such as clustering and pseudotime reconstruction,81 and contribute errors in cell-type identification.82 To address this problem, several computational methods have been developed including DrImpute,83 RESCUE,84 and DeepImpute.85 Nevertheless, scRNA-Seq can only assist investigation at the transcriptome level, which does not delineate the protein abundance, post-translational modifications of target proteins, or spatial information of individual cells. A key parallel advance, Cytometry by Time Of Flight (CyTOF, or mass cytometry), through the heavy metal-conjugated antibodies that can be detected by atomic mass spectrometry allows us to detect dozens of proteins in millions of individual cells at once86 and to perform deep immunophenotyping of heterogeneous single-cell populations. For instance, Hawley et al.87 have utilized the combined scRNA-seq and CyTOF technologies to investigate cells involved in lacrimal gland repair. Clustering of significantly differentially expressed genes revealed that new subsets of cells expressed the muscle proteins, in addition to the myoepithelial cells, indicating their potential role in tissue regeneration. Furthermore, the sequencing-based spatial transcriptomics methodology that combines histology and transcriptome profiling88 has been developed, allowing visualization of gene activity of individual cells in a 3-dimensional localized microenvironment. This new technology facilitates researchers to examine the spatial contribution of individual immune cells during tissue repair and regeneration in the future.
In addition to the transcriptome and proteome levels, cellular heterogeneity can also be examined at the genomic and epigenetic levels. For instances, methylation and histone modification patterns of individual cells can be profiled by methods such as scBS-Seq89 or scM&Tseq,90 and CUT&Tag91 or CoBATCH,92 respectively. Genome-wide chromatin accessibility of individual cells can also be assessed by single-cell Assay for Transposase Accessible Chromatin using sequencing.93,94 Moreover, it is possible to capture the genome conformation at a single-cell resolution by using single-cell Hi-C.95 In the future, integrative analysis of these multiomics data could address many unresolved questions in the field. Supporting these advances, innumerable computational methods are also needed. In particular, the wide adoption of cutting-edge machine learning and artificial intelligence methods would enhance the efficiency and throughput of data analysis. Taken together, future integrative multiomics analysis will provide new insights into understanding the cellular diversity and individual immune cell behavior during tissue repair and regeneration, facilitating the specificity and precision of immunoregenerative medicine.
Acknowledgments
This work was supported by Research Grants Council of Hong Kong (14108420, C4024-16W, C4026-17WF); National Natural Science Foundation of China (81922077); University Grants Committee Research Matching Grant Scheme (2019, 2020); Croucher Foundation (Innovation Award and Start-up Allowance); Direct Grant, Faculty Innovation Award, Research Committee Funding, and Seed Fund from Lui Chi Woo Institute of Innovative Medicine from CUHK.
References