-
PDF
- Split View
-
Views
-
Cite
Cite
Anfan Chen, Kaiping Chen, Jingwen Zhang, Jingbo Meng, Cuihua Shen, When national identity meets conspiracies: the contagion of national identity language in public engagement and discourse about COVID-19 conspiracy theories, Journal of Computer-Mediated Communication, Volume 28, Issue 1, January 2023, zmac034, https://doi.org/10.1093/jcmc/zmac034
- Share Icon Share
Abstract
There are growing concerns about the role of identity narratives in spreading misinformation on social media, which threatens informed citizenship. Drawing on the social identity model of deindividualization effects (SIDE) and social identity theory, we investigate how the use of national identity language is associated with the diffusion and discourse of COVID-19 conspiracy theories on Weibo, a popular social media platform in China. Our results reveal a pattern of identity communication contagion in public conversations about conspiracies: national identity language usage in original posts is associated with more frequent use of such language in all subsequent conversations. Users who engaged in discussions about COVID-19 conspiracies used more national identity expressions in everyday social media conversations. By extending the SIDE model and social identity theory to misinformation studies, our article offers theoretical and empirical insight into how identity–contagious communication might exacerbate public engagement with misinformation on social media in non-Western contexts.
Lay Summary
This article examined the use and consequences of national identity language in public discourse related to COVID-19 conspiracy theories on Weibo, one of the largest social media platforms in China. We investigated how social media users discussed conspiracy theories about the origins of COVID-19 to understand how national identity expressions on Weibo affected public engagement with these conspiracy theories. Our findings reveal a contagion of national identity language between the original posts and all subsequent replies. We also discovered that users who employed national identity language during discussions about COVID-19 conspiracy theories subsequently used more of this language, even in everyday posts that were unrelated to COVID-19. Our findings uncover how social media platforms are used as public spheres for identity–contagious communication that challenges misinformation correction and public understanding of other social groups.
Over the past decade, identity narratives have burgeoned on social media, on subjects ranging from politics to scientific issues, including the recent COVID-19 pandemic (Chen et al., 2020). Social media’s inherent nature of constructing identities has fomented identity-driven communication—a form of communication that highlights intergroup and intragroup distinctions (Krings et al., 2021). The use of identity language has become increasingly prevalent in geopolitical conflicts related to the COVID-19 pandemic (Wang, 2021). For example, labeling the coronavirus as “Kung flu” signals otherness and creates conspiratorial arguments about the country of origin.
Responding to this escalation of identity-driven communication and conspiracy theories, scholars have started to explore the relationship between people’s identities and conspiracy beliefs, mainly through self‐administered surveys (Krings et al., 2021). However, little is known about how identity narratives on social media contribute to the spread of misinformation and public engagement with conspiracy narratives. Although recent studies have revealed that identity-driven communication (such as the use of out-group animosity) can increase online public engagement (Rathje et al., 2021), the focus has been on the subsequent diffusion of identity-related posts on social media. However, this approach cannot explain how this type of communication generates even more identity-driven content in social media discussions through a contagion effect. In this article, we examine the use of identity narratives (especially national identity language) in the context of the Sino–U.S. relationship during the COVID-19 pandemic to understand how such narratives prompt the spread of conspiracies on social media and influence conspiracy-related discussions.
By adopting a multidimensional approach, we study public engagement with conspiracy theories about the origin of the virus by examining user engagement size (e.g., number of reposts, likes, and diffusion size), discourse (e.g., user replies), and the traits of users who debunked or propagated COVID-19 conspiracies. Grounded in the social identity model of deindividuation effects (SIDE) and social identity theory, we hypothesize how national identity language in the form of in-group favoritism and out-group hostility might influence these various outcomes of public engagement with conspiracies.
After examining a massive Sina Weibo (hereafter Weibo) corpus of over 40 million posts related to COVID-19, we identified 1,516 original conspiracy-related posts dated between December 1, 2019, and April 30, 2020. Subsequently, we reconstructed their user reply chains (i.e., a total of 51,050 reposts and replies) and performed content analyses to examine the use of in-group and out-group national identity language in public engagement with conspiracies. In this article, we first describe how identity-driven communication has become a new norm on social media responding to geopolitical tensions. We then draw on the SIDE model and social identity theory to hypothesize how the use of national identity language can affect public engagement with both COVID-19 conspiracies and debunking posts (Table 1).
Literature review
Identity-driven communication is characterized by an increasing salience about group identity, which draws attention to group relationships (such as intragroup solidarity and intergroup conflicts). Identity-driven communication takes various forms, from using group pronouns such as “we” and “them” in fake news (Li & Su, 2020) to geopolitical discourse that centers on hostility toward actors in other countries (Chen et al., 2020). Identity-driven communication has been used to generate popular and divisive online content by referencing sensitive or potentially opposing group identities, creating a media environment that rewards extreme views and penalizes moderate positions (Bail, 2021).
National identity is one major form of identity-based communication that comprises emotionally fueled in-group favoritism and out-group hostility (Jardina, 2021; Levin & Sidanius, 1999). Conspiracy theories can invoke extensive national identity narratives by portraying politically powerful competing nations as conspirators who act for their own benefit against the common good (Keeley, 1999). This framing of messages emphasizes group conflicts (van Prooijen & van Vugt, 2018). For example, a prominent COVID-19 conspiracy theory about its origin framed the virus as a bioweapon (Jamieson & Albarracin, 2020) designed to kill Chinese or American people.
Existing studies have explored how message characteristics, such as fake news versus accurate information (Vosoughi et al., 2018), emotions (Brady et al., 2019), and animosity (Rathje et al., 2021), can affect the virality of conspiracy theories on social media. However, the question of how identity language, a critical component of the SIDE model and social identity theory, influences engagement with conspiracies on social media and fosters conspiratorial mindsets has not been systematically examined. This research offers new insights into the role of group identity in the spread and discussion of misinformation and conspiracies.
In the following subsections, we first introduce the growth and importance of national identity narratives on Chinese social media. We then discuss the SIDE model and the consequences of these national narratives on public engagement with conspiracies on Chinese social media.
National identity as a key feature on Chinese social media
In the Chinese context, national identity narratives are one of the strongest manifestations of group identity on social media that often center on the theme of denouncing the humiliation of the nation while at the same time advocating for patriotism (Schneider, 2018). These national narratives criticize Western powers interfering with Chinese sovereignty and internal affairs and dominating the global order (Schneider, 2018). In terms of advocating for patriotism, recent years have witnessed the growth of pro-Xi discourses on social media. As Neagli (2021) noted, more control on social media is consolidated through the State Internet Information Office and the revised cybersecurity laws under President Xi to guide public opinions on social media. Other scholars (e.g., Bolsover, 2017) have pointed out the increasing crackdowns on online rumors and misinformation through computational propaganda technologies such as content filtering and ranking. Scholars such as Han (2021) have studied netizen discourses on a popular overseas Chinese forum and found a positive relationship between nationalism and social media users’ attitude toward the Chinese regime. As Han (2021) noted, these pro-regime discourses often involve attacking other countries (e.g., the United States or the West in general) or Taiwan as enemy forces that seek to disrupt China’s rise. These pro-regime discourses also involve the Chinese identity of users by referring to overseas Chinese’ living experiences and using words such as “overseas Chinese” and “overseas students.”
Multiple stakeholders contribute to these growing nationalist narratives. Nationalist narratives are created and posted organically by ordinary users who voluntarily post pro-regime and patriotic content to celebrate Chinese identity and criticize anti-China discourses (Han, 2015). A small but consistent force consists of the 50 Cent Party members who are paid by the Chinese government authorities to influence public opinion on digital platforms. For instance, Miller (2016) found that government “astroturfers” contributed around 15% of all comments to the news of 19 popular news outlets in China. King et al. (2017) estimated that 50 Cent Party members publish approximately 448 million posts on Weibo each year. These members invoke their Chinese identity by posting content that glorifies the revolutionary history of the Chinese Communist Party.
Since the beginning of the COVID-19 pandemic, a new trend of nationalist narratives has emerged that provides misinformation and conspiracies regarding the origin of the virus. Mu (2020) termed this new trend “nationalism conspiracy theories,” that is, conspiracy theories mixed with nationalist sentiments. For instance, Chen et al. (2020) demonstrated that narratives that attribute responsibility for the virus to other countries (e.g., the United States) constitute one of the most prevalent discourses when users discuss the virus’ origins and debate whether the virus was created in laboratories as a bioweapon. In these nationalist discourses surrounding COVID-19 misinformation and conspiracy theories, netizens use in-group identity terms such as “compatriots” (同胞), as well as out-group hostility terms such as “American imperialism” (美帝国主义).
Looking at national identity narratives through the lens of SIDE
In this study, we investigate the influence of national identity language by focusing on Weibo (one of the most popular Chinese social media platforms) to examine and theorize about how in-group favoritism language about China and out-group hostility toward the United States influence public engagement with conspiracy theories.
Social identity model of deindividuation effects and public engagement with conspiracy theories on social media
The SIDE model provides a valuable framework for investigating how national identity narratives can influence public engagement with conspiracy theories and counternarratives. When discussing public issues on social media, individuals tend to invoke social identity over individual identity. However, in doing so, “individuals do not lose all sense of self; rather, they shift from the personal to the social level of identification” (Reicher et al., 1995, p. 177). Individuals emphasize social identity through various mechanisms, including social categorization of the self, in-group prototyping, and depersonalization to embody group identity (Hogg & Hains, 1996). Social (or group) identity encompasses many aspects, such as gender, race, ethnicity, political partisanship, and national identity. Classic works in social psychology have explained the formation of in-group identification and out-group derogation as forces that can drive individuals to affirm and defend their views (Tajfel, 2010; Turner & Reynolds, 2012). These actions can influence the quantity and quality of online discussions through group norm conformity (Kim et al., 2019).
How in-group favoritism language influences public engagement with conspiracy theories
In this part, we discuss how users react to in-group favoritism language about conspiracy theories on social media. Here, the concept of social identification as defined in social identity theory provides a useful explanation. In a classic experiment that examined the behavioral impact of ethnocentrism (in-group bias), Tajfel (1970) found that participants assigned more money to others who were perceived as in-group members. Tajfel (1974) further explained the psychological mechanism under which in-group favoritism can increase a person’s emotional attachment to their social group (p. 69), driving individuals to place more emphasis on their social identity as a member of the group than on their personal identity (Tajfel & Turner, 1986).
Extending the social identity approach to computer-mediated communication (CMC) domains, Reicher et al. (1995) proposed the SIDE phenomena, that is, polarization is strongest in computer-assisted group discussions when individuals are isolated in different rooms (anonymity) and are referred to using a group code (group salience). As highlighted by the authors, deindividuation engendered by the CMC context can strengthen the cognitive salience of social identity. This cognitive aspect of the SIDE model suggests that strong identification with an in-group can drive individuals to take action to adhere to the in-group’s standards and norms. Applying the SIDE model to the new media environment, Spears and Postmes (2015) noted the mass dissemination potential of social media to produce collective actions, both online and offline. For instance, in the Arab Spring movements, the expression of in-group identity narratives (e.g., the use of “we”) mobilized social support from online users (e.g., via retweeting) and facilitated large-scale offline protests.
In the Chinese social media context, research has indicated how using in-group favoritism language has increased public engagement with these posts. For example, Repnikova and Fang (2018, p. 763) noted a pattern of the bottom-up in-group cheerleading and termed it “authoritarian participatory persuasion 2.0.” In this bottom-up cheerleading, ordinary netizens encourage others to explicitly demonstrate their Chinese identity by reposting positive content about their invoked social identity, such as sharing solidarity content during disasters or pro-regime content. Such user behavior can create a “contagion effect,” motivating other users to respond with messages containing language that invokes and affirms their Chinese identity, such as praising the achievements of the Chinese government and its people.
The explicit affirmation of social identity can be intensified during times of crisis. For example, scholars found increased national narcissism and patriotism during the COVID-19 pandemic in China, which encouraged public endorsement and dissemination of conspiracy theories denouncing other countries (Mu, 2020; Wang et al., 2021; Zhai & Yan, 2022). Therefore, grounded in the SIDE model and social identity theory, which highlight the impact of using in-group favoritism to affirm group identities and values, we hypothesize that there is a positive association between the use of in-group favoritism language in conspiracy-related posts and users’ interactions with these posts:
H1: Conspiracy-related posts that use in-group favoritism language are likely to receive more likes, comments, and a larger diffusion size than those that do not use in-group favoritism language.
Besides expanding user engagement size, in-group favoritism language can also influence how users respond to original posts. The SIDE model and social identity theory suggest a potential contagion effect of in-group favoritism language on users’ replies. As explained by Sherman and Cohen (2006, p. 189), individuals affirm their identity by engaging in activities that remind them of who they are. One typical activity that affirms in-group identity is mimicking the language used by the in-group. For instance, in an experimental setting, Rösner and Krämer (2016) found that participants wrote more aggressive comments (e.g., using offensive and crude words or disparaging remarks) when responding to peer comments that used more aggressive expressions. This language mimicking is also found on Chinese social media. For example, research on an online Chinese nationalist group demonstrated that comments such as “We are all Chinese, we are all yellow skin” became viral during the Cross-Strait memes wars (Fang & Repnikova, 2018). While the aim is to strengthen in-group solidarity and homophily, it also produces a contagion pattern of in-group favoritism language (Fang & Repnikova, 2018). Thus, we propose the following as our second hypothesis:
H2: Conspiracy-related posts that use in-group favoritism language are likely to receive replies containing more in-group favoritism language compared with posts that do not.
How out-group hostility language influences public engagement with conspiracy theories
In this part, we discuss how users react to out-group hostility language about conspiracy theories on social media. Social identity theory argues that “the mere awareness of the presence of an out-group is sufficient to provoke intergroup competitive or discriminatory responses on the part of the in-group” (Tajfel & Turner, 1986, p. 281). When people’s in-group identity becomes entangled with out-group hostility, they can take defensive action against perceived hostility. For instance, a patriotic individual will attack the credibility of an out-group member to protect their country’s identity (Sherman & Cohen, 2006). Applying these ideas to CMC, the SIDE model suggests that one’s social identity is reinforced in the intergroup context, such as when faced with out-group opposition. The strategic aspect of the SIDE model proposes that in this deindividualization environment, individuals are likely to coordinate resistance toward out-groups (i.e., through collective expressions and actions) to express and defend their social identities. For example, when users view posts of out-group hostility toward the in-group, their social identity can be fueled, which encourages them to leave replies that criticize the out-group and strengthen their in-group pride. Recent studies on Western social media platforms have found that using out-group hostility language in posts (e.g., animosity toward the other parties) is associated with more retweets (Rathje et al., 2021).
In the Chinese social media context, there is scant evidence of the relationship between the use of out-group hostility language and online public engagement. However, heightened Sino–U.S. tensions during the COVID-19 pandemic (Zhao et al., 2021) manifested as increasingly prevalent out-group hostility expressions on Chinese social media. For instance, Chen et al. (2020) revealed that Weibo users tended to use more blame-centered language, attributing responsibility for the pandemic to the United States when former President Trump tweeted “China virus” and proposed immigration bans. Chinese social media users responded with expressions of hostility toward the United States, with some using blaming language, such as “American imperialism.” Others used more derogatory language, such as “warmongers” and “Western devil.”
Out-group hostility language can threaten social identity, and the SIDE model suggests that individuals will invoke social identity over individual identity as a strategic way of resisting the out-group. Therefore, we hypothesize that there are fewer likes of posts that use out-group hostility language because these posts threaten in-group identity. However, we also hypothesize that posts using out-group hostility language will receive more comments in defense of in-group pride. Moreover, these posts will be shared more widely to rally support because in-group members need to maintain and affirm their group values, as suggested by social identity theory. Therefore, we propose the following hypotheses:
H3(a): Conspiracy-related posts that use out-group hostility language are likely to receive fewer likes than those that do not.
H3(b): Conspiracy-related posts that use out-group hostility language are likely to receive more comments and have larger diffusion compared with those that do not.
Out-group hostility language can also influence how users reply to original posts. As suggested by social identity theory, users are more likely to choose identity language in their replies as a means of “defensive bolstering” (Tetlock et al., 1989). Empirical evidence supports this identity protection mechanism, and scholars (e.g., Bail, 2021) argue that social media amplifies extremist viewpoints, which can create a contagion effect through conversations (e.g., replies and commenting). Using out-group hostility language in replies also serves as a way of affirming positive bias toward users’ social identity to repudiate original posts that threaten users’ social identity. In China, Diba Expedition (帝吧出征) is one example of cybernationalism, in which youths born during and after the 1990s use Baidu’s discussion forum Diba to post memes to attack and educate out-groups, such as those that support Taiwan being independent of China (Yang, 2018). For example, the Diba group posted out-group derogatory comments on the Facebook pages of Taiwan’s pro-independence media, referring to Taiwan’s president as the “provincial governor” of China and Taiwanese people as “frogs at the bottom of a well” (Fang & Repnikova, 2018). These replies invoked out-group hostility language to foment more out-group hostility, creating a vicious cycle. Therefore, we propose the following:
H4: Conspiracy-theory-related posts that use out-group hostility language are likely to receive replies containing more out-group hostility language than posts that do not use out-group hostility language.
From national identity in conspiracy theories to nationalists in everyday social media chat
Social media studies often focus on messages instead of individuals who engage with conspiracy theories and counternarratives. However, the existence and forms of network structures and discourse content can only occur when individuals participate. Studying individuals and their messages allows us to understand social identity at both the message and individual levels. This is because the SIDE model primarily focuses on how individuals engage in group behavior instead of on the messages they generate on social media. A deeper examination of the individuals will also provide useful information regarding the types of users that are more susceptible to conspiracy theories.
As demonstrated in the homophily and echo chamber phenomena (Barberá, 2015), people who engage with similar social media content share similar traits, such as political identities. Beyond the previously examined traits (such as demographics), linguistic traits (Park et al., 2015), including the use of identity language (e.g., national identity), can serve as an important basis for observing social media clusters of public engagement with conspiracy theories. In recent literature, researchers have started to study the personalities and psychological traits of individuals who believe in and share conspiracy theories (Krings et al., 2021). Klein et al. (2019) revealed that users who engage in conspiracy theory discussions on Reddit forums (subreddits) often use language that suggests an abuse of power and deception, compared with those who do not engage in conspiracy theory subreddits.
To expand our investigation using social identity theory from social media messages to the users who engage with messages, we studied the use of national identity language in users’ everyday social media posts beyond the COVID-19 pandemic. One way of understanding the traits of users is by examining the content of their historical posts to learn about their discourse styles. This is useful because users’ profile information is often limited and self-reported; hence, it may not be as accurate as their historical posts in revealing their traits. We compared the use of national identity language in everyday social media posts among users who did or did not use national identity language when discussing COVID-19 conspiracy theories. Informed by the echo chamber literature that suggests homophily of user traits beyond the COVID-19 period, we propose the following final hypothesis:
H5: People who use national identity language (i.e., in-group favoritism and out-group hostility words) when discussing COVID-19 conspiracy theories are also more likely to use such language in their everyday posts compared with those who do not use national identity language when discussing COVID-19 conspiracy theories.
Data and methods
This study collected a novel dataset from Weibo, which allowed us to adopt a multidimensional approach to examine public engagement with COVID-19 conspiracy theories, operationalized in the form of user engagement size, discourse, and the traits of users who debunked or propagated COVID-19 conspiracy theories. Specifically, user engagement size refers to metrics, including the number of reposts, likes, and comments, as well as the extent of diffusion of a conspiracy theory post. Discourse refers to discussions around a conspiracy theory post (in the form of comments and replies to the original post/reposts), which forms a conversation between users with similar interests in the conspiracy narratives. The traits of users, in this study, refer to the average frequency with which these users utilize in-group favoritism words and out-group hostility words in their daily posts.
Data collection and text pre-processing
Weibo is one of the most popular microblogging platforms in China, with 560 million monthly active users at the end of 2019 (Sina Weibo, 2021). Our data collection followed three steps (see Supplementary Appendix A). First, we retrieved more than 40 million COVID-19-related posts from a large-scale public coronavirus Weibo dataset (Weibo-COV) created by Hu et al. (2020). Second, we drew from mainstream fact-checking websites to identify 35 types of COVID-19 conspiracy-related narratives which were used as the basis for classifying whether a post qualified as containing a conspiracy theory. Third, we had three coders to develop keyword combinations to summarize each conspiracy narrative to retrieve posts using a Boolean query search method from the Weibo-COV database in MongoDB. These keyword combinations were designed to allow for the retrieval of the maximal number of potential posts related to a conspiracy theory to achieve exhaustion (see Supplementary Appendix B). After the above three steps, these COVID-19 conspiracy theories and their representative keyword combinations allowed us to retrieve 6,735 originally crafted conspiracy-related posts (which sparked a total of 346,783 reposts and 38,075 replies) from 6,735 unique users.
To ensure these 6,735 originally crafted posts retrieved with the keyword search method are indeed related to conspiracy theories, three coders manually categorized these 6,735 potential COVID-19 conspiracy theory-related posts into three types: conspiracy theory posts (n = 923), debunking posts (N = 593), and posts irrelevant to conspiracy theories or debunking (5,219 posts). Intercoder reliability was high (Krippendorff’s α = 0.834).
In this research, we only focused on studying the 1,516 original COVID-19-related posts, including conspiracy theory posts and debunking posts. The final dataset contained 1,516 unique and originally crafted Weibo posts (originally crafted posts are posts that start threads, not reposts or comments) about COVID-19 conspiracy theories dated from January 1, 2020, to April 30, 2020. These 1,516 original posts reached a large audience, generating 27,689 reposts, 150,636 likes, and 25,067 comments. The diffusion chains (Figure 1) of each conspiracy theory (n = 923) and debunking (n = 593) posts were then reconstructed by collecting their respective reposts in the diffusion chain using WeiboEvents (Figure 2). This open-access Weibo analytic system retrospectively tracks and collects each post’s retweeting information based on the uniform resource locator (URL) of the original post, resulting in 51,050 reposts across all diffusion cascades, of which 17,405 are reposts with replies (7,607 are conspiracy theory posts and 9,798 are debunking posts), by 16,938 unique Weibo users.
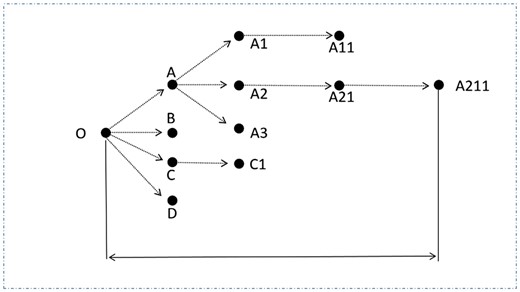
Demonstration of the diffusion chain of a post. In this example, the diffusion size is 11.

Finally, to construct the dataset of users’ daily posts, the 700 most recent posts1 of each of the 16,938 unique Weibo users who reposted with comments were retrieved from their Weibo main pages on February 5, 2021, resulting in 7,064,299 posts generated by 16,439 Weibo users. It should be noted that 499 unique Weibo user accounts in Hu et al.’s (2020) original dataset have since been censored or deleted as of October 31, 2021 (when we extracted the recent posts).
Measurements
The user engagement size of conspiracy-related posts was quantified using three indicators: number of likes (M = 10.35, SD = 9.62), number of comments a post received (M = 3.78, SD = 3.99), and diffusion size (M = 1.50, SD = 1.97). For a unique post related to COVID-19 conspiracy theories, the diffusion size was quantified by the number of users a related message reached at all stages of the diffusion chain (see Figure 1 for illustration).
National identity language usage
We operationalized national identity language usage as whether a post adopts in-group favoritism and out-group hostility language. The national identity language, including in-group favoritism and out-group hostility, was conceptualized according to the definition in social psychology and political science theories (Jardina, 2021). Specifically, in-group favoritism language refers to positive words that address in-group identification. These words promote intra-group favoritism and maintain intra-group feelings of solidarity, with examples including “descendants of civilized China,” “descendants of the Chinese nation,” “compatriot,” and “greater China,” which emphasize pride in Chinese national identity. In contrast, the use of out-group hostility language expresses negative attitudes, derogation, and perceived intergroup threats toward the out-groups. These words would include phrases such as “the Eight-Power Allied Forces,” “imperialism,” “colonialism,” “imperialist powers,” and “white pig,” which derogate the out-group. These keywords also capture national identity in various dimensions, including ethnicity, culture, and civic identity.
In this study, following the practices of dictionary-based textual analysis (Thomas et al., 2021), two dictionaries were developed (see Supplementary Appendix C). One captured in-group favoritism language and the other captured out-group hostility language used in the collected original posts, reposts in each diffusion chain, and users’ historical posts. To construct the dictionary for our target concept (national identity language), we integrated manual and computer-assisted content analysis (see Supplementary Appendix D). After several rounds of iteration and cross-validation, we retained a dictionary with 128 words, consisting of 47 words related to in-group favoritism and 81 words related to out-group hostility. Within this dictionary, in-group favoritism words capture the concepts of nation-building, ethnicity, and race in the Chinese national context. These include “descendants of civilized China” (炎黄子孙), “descendants of the dragon” (龙的传人), “civilized China” (华夏), and “motherland” (祖国). The out-group hostility words capture hatred or derogation toward groups outside the Chinese national context, such as “empire” (帝国), “American imperialism” (美帝国主义), “foreign devils” (洋鬼子), “foreign powers” (外国势力), and “Western powers” (西方势力).
Using the national identity dictionary developed in our study, we identified whether the COVID-19 conspiracy-related posts mentioned words about in-group favoritism (M = 0.52, SD = 0.34) and out-group hostility (M = 0.23, SD = 0.14). In addition, we calculated the word frequency of in-group favoritism and out-group hostility language for the collected reposts with replies (in-group favoritism: M = 0.29, SD = 0.26; out-group hostility: M = 0.23, SD = 0.18). In addition, we defined these users as follows: (a) in-group favoritism or (b) out-group hostility language adopters who used (a) in-group favoritism or (b) out-group hostility words in their discussions of conspiracy-theory-related posts/reposts. By comparison, non-adopters were those who did not use such national identity language. Finally, we analyzed the users’ 700 most recent posts (7,064,299 posts by 16,439 Weibo users). A mean identity language usage score was then aggregated for each user (in-group favoritism: M = 1.54, SD = 0.50; out-group hostility: M = 1.10, SD = 0.66).
Control variables
We included two types of control variables that have been found to be correlated with user engagement size in the existing scholarship, including content-related features (e.g., URL, hashtag, and sentiment) (Brady et al., 2019; Rathje et al., 2021; Shin & Thorson, 2017) and user-level characteristics (e.g., user gender, number of followers of an account, user type) (Chen et al., 2020; Rathje et al., 2021). In terms of how users engage with conspiracy theories, scholars have also proposed an additional content-related feature, post type, to be associated with user engagement size, such as the diffusion of a post (Vosoughi et al., 2018). The post type centers on whether a post debunks a conspiracy theory (i.e., refuting/disapproving, reporting, or correcting conspiracy narratives) or propagates conspiracy theories (i.e., fabricating, endorsing, or spreading conspiracy theories). Therefore, we also included post type as our content-level control (see Supplementary Appendix E for examples of debunking and conspiracy theory posts). A series of control variables, consisting of both content-level features of a post, including post type, text length, containing URL or not, hashtag count, and text sentiment score (see Supplementary Appendix F for the sentiment analysis method adopted in this study), and user-level features, including user verification status, user’s gender, user’s follower number, followee number, and historical posts number, were added in the following regression analysis.
Analysis methods
By adapting methods used in similar studies (Fletcher & Nielsen, 2018), we adjusted regression models to examine how language use containing in-group favoritism and out-group hostility predicted the user engagement size of conspiracy-theory-related posts and the use of identity language in users’ replies. Table 2 presents the rationale for our model selection. The dependent variables were as follows: number of likes (Model 1a), number of comments (Model 1b), and diffusion size were discrete count variables (Model 1c), while the number of in-group favoritism and out-group hostility words in replies (Models 2a and 2b) reflected the number of occurrences of behavior over a period. To test the hypotheses, Poisson regression models were adopted. In addition, to examine H5, we conducted a series of t-tests to examine the associations between national identity language frequency in users’ historical posts and their national identity language adoption when discussing COVID-19 conspiracy theories.
Hypotheses . | Independent variables . | Dependent variables . | Model selected . |
---|---|---|---|
H1, H3a, and H3b (results in Table 3) |
| User engagement size (i.e., number of likes, comments, and diffusion size) | Poisson regression |
H2 (Table 4) |
| In-group favoritism word count in replies | Poisson regression |
H4 (Table 4) |
| Out-group hostility word count in replies | Poisson regression |
H5 |
| (a) In-group favoritism or (b) out-group hostility average word count in historical posts | t-tests |
Hypotheses . | Independent variables . | Dependent variables . | Model selected . |
---|---|---|---|
H1, H3a, and H3b (results in Table 3) |
| User engagement size (i.e., number of likes, comments, and diffusion size) | Poisson regression |
H2 (Table 4) |
| In-group favoritism word count in replies | Poisson regression |
H4 (Table 4) |
| Out-group hostility word count in replies | Poisson regression |
H5 |
| (a) In-group favoritism or (b) out-group hostility average word count in historical posts | t-tests |
Notes: (1) Adopters are those who used (a) in-group favoritism or (b) out-group hostility language in their discussions about conspiracy-related posts, while non-adopters are those who did not use such national identity language. (2) The rationale for model selection is explained in Supplementary Appendix G.
Hypotheses . | Independent variables . | Dependent variables . | Model selected . |
---|---|---|---|
H1, H3a, and H3b (results in Table 3) |
| User engagement size (i.e., number of likes, comments, and diffusion size) | Poisson regression |
H2 (Table 4) |
| In-group favoritism word count in replies | Poisson regression |
H4 (Table 4) |
| Out-group hostility word count in replies | Poisson regression |
H5 |
| (a) In-group favoritism or (b) out-group hostility average word count in historical posts | t-tests |
Hypotheses . | Independent variables . | Dependent variables . | Model selected . |
---|---|---|---|
H1, H3a, and H3b (results in Table 3) |
| User engagement size (i.e., number of likes, comments, and diffusion size) | Poisson regression |
H2 (Table 4) |
| In-group favoritism word count in replies | Poisson regression |
H4 (Table 4) |
| Out-group hostility word count in replies | Poisson regression |
H5 |
| (a) In-group favoritism or (b) out-group hostility average word count in historical posts | t-tests |
Notes: (1) Adopters are those who used (a) in-group favoritism or (b) out-group hostility language in their discussions about conspiracy-related posts, while non-adopters are those who did not use such national identity language. (2) The rationale for model selection is explained in Supplementary Appendix G.
Results
The scale of national identity language use in conspiracy theory and debunking posts
Among the 1,516 original posts related to COVID-19 conspiracy theories, we compared the use of in-group favoritism and out-group hostility language in posts that propagated or debunked the conspiracy theories. An independent sample t-test indicated that conspiracy theory propagation posts used a larger number of in-group favoritism words (M = 0.59) compared with debunking posts (M = 0.40, p < .01, t = 2.632, df = 1,514). In terms of out-group hostility language usage, conspiracy theory propagation posts also adopted a greater number of out-group identity words (M = 0.32) compared with debunking posts (M = 0.11, p < .001, t = 4.241, df = 1,514).2
Association between in-group favoritism, out-group hostility language, and user engagement size of conspiracy-theory-related posts
Three Poisson regression models were conducted to examine the role of in-group favoritism and out-group hostility language on user engagement size (i.e., number of likes, comments, and diffusion size).
We found a positive association between in-group favoritism language and user engagement size of conspiracy-theory-related posts (H1 supported). As indicated in Table 3, using in-group favoritism words in posts was associated with 1.163 times (e0.151) more likes (b = 0.151, p < .001), 1.077 times (e0.074) more comments (b = 0.074, p < .001), and 1.259 times (e0.230) larger diffusion size (b = 0.230, p < .001). For out-group hostility, the results indicated that using out-group hostility words was associated with 1.123 times (e0.116) more comments (b = 0.116, p < .001) and 1.174 times larger (e0.160) diffusion size (b = 0.160, p < .05), but 0.280 times (e−0.328) less likes (b = −0.328, p < 0.001). Thus, H3(a) and (b) are supported.
Poisson regression models predicting user engagement size of conspiracy-related posts on Weibo
. | Model 1a . | Model 1b . | Model 1c . | |||
---|---|---|---|---|---|---|
. | User engagement size . | |||||
. | Number of likes . | Number of comments . | Diffusion size . | |||
. | b . | SE . | b . | SE . | b . | SE . |
Use of national identity language | ||||||
In-group favoritism (ref. without in-group favoritism words) | 0.151*** | 0.020 | 0.074*** | 0.035 | 0.230*** | 0.045 |
Out-group hostility (ref. without out-group hostility words) | −0.328*** | 0.033 | 0.116*** | 0.049 | 0.160* | 0.071 |
Control variables | ||||||
Content-level controls | ||||||
Debunking post (ref. conspiracy post) | 0.555*** | 0.018 | 0.633*** | 0.032 | 0.739*** | 0.041 |
Text length | 0.002*** | 0.001 | 0.002*** | 0.001 | 0.002*** | 0.001 |
URL (ref. no URL) | 0.135** | 0.018 | 0.210*** | 0.031 | 0.509*** | 0.038 |
Hashtag count | 0.134*** | 0.004 | 0.113*** | 0.006 | 0.145*** | 0.008 |
Text sentiment score | −0.022*** | 0.001 | −0.014*** | 0.001 | −0.017*** | 0.002 |
User-level controls | ||||||
Verified individual users (ref. = ordinary user) | −0.004 | 0.023 | −0.1.017*** | 0.035 | −0.771*** | 0.036 |
Verified organizational users (ref. = ordinary user) | −0.620*** | 0.028 | −1.748*** | 0.045 | −1.611*** | 0.050 |
Gender of user (ref. = female) | −0.201*** | 0.018 | −0.604*** | 0.031 | −0.607*** | 0.039 |
User’s follower number | 0.537*** | 0.003 | 0.591*** | 0.005 | 0.744*** | 0.007 |
User’s followee number | −0.054 | 0.008 | −0.080*** | 0.015 | −0.175*** | 0.018 |
User’s number of historical posts | −0.271*** | 0.007 | −0.179*** | 0.011 | −0.319*** | 0.014 |
Constant | −0.173** | 0.053 | −1.758*** | 0.093 | −2.706*** | 0.126 |
Pseudo-R2 | 49.7% | 54.8% | 74.0% | |||
N | 1,516 |
. | Model 1a . | Model 1b . | Model 1c . | |||
---|---|---|---|---|---|---|
. | User engagement size . | |||||
. | Number of likes . | Number of comments . | Diffusion size . | |||
. | b . | SE . | b . | SE . | b . | SE . |
Use of national identity language | ||||||
In-group favoritism (ref. without in-group favoritism words) | 0.151*** | 0.020 | 0.074*** | 0.035 | 0.230*** | 0.045 |
Out-group hostility (ref. without out-group hostility words) | −0.328*** | 0.033 | 0.116*** | 0.049 | 0.160* | 0.071 |
Control variables | ||||||
Content-level controls | ||||||
Debunking post (ref. conspiracy post) | 0.555*** | 0.018 | 0.633*** | 0.032 | 0.739*** | 0.041 |
Text length | 0.002*** | 0.001 | 0.002*** | 0.001 | 0.002*** | 0.001 |
URL (ref. no URL) | 0.135** | 0.018 | 0.210*** | 0.031 | 0.509*** | 0.038 |
Hashtag count | 0.134*** | 0.004 | 0.113*** | 0.006 | 0.145*** | 0.008 |
Text sentiment score | −0.022*** | 0.001 | −0.014*** | 0.001 | −0.017*** | 0.002 |
User-level controls | ||||||
Verified individual users (ref. = ordinary user) | −0.004 | 0.023 | −0.1.017*** | 0.035 | −0.771*** | 0.036 |
Verified organizational users (ref. = ordinary user) | −0.620*** | 0.028 | −1.748*** | 0.045 | −1.611*** | 0.050 |
Gender of user (ref. = female) | −0.201*** | 0.018 | −0.604*** | 0.031 | −0.607*** | 0.039 |
User’s follower number | 0.537*** | 0.003 | 0.591*** | 0.005 | 0.744*** | 0.007 |
User’s followee number | −0.054 | 0.008 | −0.080*** | 0.015 | −0.175*** | 0.018 |
User’s number of historical posts | −0.271*** | 0.007 | −0.179*** | 0.011 | −0.319*** | 0.014 |
Constant | −0.173** | 0.053 | −1.758*** | 0.093 | −2.706*** | 0.126 |
Pseudo-R2 | 49.7% | 54.8% | 74.0% | |||
N | 1,516 |
Notes: (1) Reference group: gender = female, verified = unverified Weibo user; (2) Activeness, # of followers, and # of followees have been log-transformed; (3) ∗p < .05, ∗∗p < .01, ∗∗∗p < .001; (4) SE: standard error; (5) The beta coefficients can be interpreted as the log of the ratio of expected counts.
Poisson regression models predicting user engagement size of conspiracy-related posts on Weibo
. | Model 1a . | Model 1b . | Model 1c . | |||
---|---|---|---|---|---|---|
. | User engagement size . | |||||
. | Number of likes . | Number of comments . | Diffusion size . | |||
. | b . | SE . | b . | SE . | b . | SE . |
Use of national identity language | ||||||
In-group favoritism (ref. without in-group favoritism words) | 0.151*** | 0.020 | 0.074*** | 0.035 | 0.230*** | 0.045 |
Out-group hostility (ref. without out-group hostility words) | −0.328*** | 0.033 | 0.116*** | 0.049 | 0.160* | 0.071 |
Control variables | ||||||
Content-level controls | ||||||
Debunking post (ref. conspiracy post) | 0.555*** | 0.018 | 0.633*** | 0.032 | 0.739*** | 0.041 |
Text length | 0.002*** | 0.001 | 0.002*** | 0.001 | 0.002*** | 0.001 |
URL (ref. no URL) | 0.135** | 0.018 | 0.210*** | 0.031 | 0.509*** | 0.038 |
Hashtag count | 0.134*** | 0.004 | 0.113*** | 0.006 | 0.145*** | 0.008 |
Text sentiment score | −0.022*** | 0.001 | −0.014*** | 0.001 | −0.017*** | 0.002 |
User-level controls | ||||||
Verified individual users (ref. = ordinary user) | −0.004 | 0.023 | −0.1.017*** | 0.035 | −0.771*** | 0.036 |
Verified organizational users (ref. = ordinary user) | −0.620*** | 0.028 | −1.748*** | 0.045 | −1.611*** | 0.050 |
Gender of user (ref. = female) | −0.201*** | 0.018 | −0.604*** | 0.031 | −0.607*** | 0.039 |
User’s follower number | 0.537*** | 0.003 | 0.591*** | 0.005 | 0.744*** | 0.007 |
User’s followee number | −0.054 | 0.008 | −0.080*** | 0.015 | −0.175*** | 0.018 |
User’s number of historical posts | −0.271*** | 0.007 | −0.179*** | 0.011 | −0.319*** | 0.014 |
Constant | −0.173** | 0.053 | −1.758*** | 0.093 | −2.706*** | 0.126 |
Pseudo-R2 | 49.7% | 54.8% | 74.0% | |||
N | 1,516 |
. | Model 1a . | Model 1b . | Model 1c . | |||
---|---|---|---|---|---|---|
. | User engagement size . | |||||
. | Number of likes . | Number of comments . | Diffusion size . | |||
. | b . | SE . | b . | SE . | b . | SE . |
Use of national identity language | ||||||
In-group favoritism (ref. without in-group favoritism words) | 0.151*** | 0.020 | 0.074*** | 0.035 | 0.230*** | 0.045 |
Out-group hostility (ref. without out-group hostility words) | −0.328*** | 0.033 | 0.116*** | 0.049 | 0.160* | 0.071 |
Control variables | ||||||
Content-level controls | ||||||
Debunking post (ref. conspiracy post) | 0.555*** | 0.018 | 0.633*** | 0.032 | 0.739*** | 0.041 |
Text length | 0.002*** | 0.001 | 0.002*** | 0.001 | 0.002*** | 0.001 |
URL (ref. no URL) | 0.135** | 0.018 | 0.210*** | 0.031 | 0.509*** | 0.038 |
Hashtag count | 0.134*** | 0.004 | 0.113*** | 0.006 | 0.145*** | 0.008 |
Text sentiment score | −0.022*** | 0.001 | −0.014*** | 0.001 | −0.017*** | 0.002 |
User-level controls | ||||||
Verified individual users (ref. = ordinary user) | −0.004 | 0.023 | −0.1.017*** | 0.035 | −0.771*** | 0.036 |
Verified organizational users (ref. = ordinary user) | −0.620*** | 0.028 | −1.748*** | 0.045 | −1.611*** | 0.050 |
Gender of user (ref. = female) | −0.201*** | 0.018 | −0.604*** | 0.031 | −0.607*** | 0.039 |
User’s follower number | 0.537*** | 0.003 | 0.591*** | 0.005 | 0.744*** | 0.007 |
User’s followee number | −0.054 | 0.008 | −0.080*** | 0.015 | −0.175*** | 0.018 |
User’s number of historical posts | −0.271*** | 0.007 | −0.179*** | 0.011 | −0.319*** | 0.014 |
Constant | −0.173** | 0.053 | −1.758*** | 0.093 | −2.706*** | 0.126 |
Pseudo-R2 | 49.7% | 54.8% | 74.0% | |||
N | 1,516 |
Notes: (1) Reference group: gender = female, verified = unverified Weibo user; (2) Activeness, # of followers, and # of followees have been log-transformed; (3) ∗p < .05, ∗∗p < .01, ∗∗∗p < .001; (4) SE: standard error; (5) The beta coefficients can be interpreted as the log of the ratio of expected counts.
The contagion of using national identity language in users’ replies
All conversations in the diffusion trees of conspiracy-theory-related posts were examined. As indicated in Table 4, H2 and H4, which pertain to the positive association between the use of national identity language (i.e., in-group favoritism and out-group hostility words) in both posts and replies, are supported. Table 4 indicates that posts using in-group favoritism language received 6.190 times (e1.823) more in-group favoritism word counts (b = 1.823, p < .001) in their replies than posts that did not use in-group favoritism words. This contagion pattern in user replies also appeared in posts that contained out-group hostility language. Posts using out-group hostility language received 5.479 times (e1.701) more out-group hostility words in their replies (b = 1.701, p < .001) compared with posts that did not use out-group hostility words.
Poisson regression models predicting the use of national identity language in replies
. | Model 2a . | Model 2b . | ||
---|---|---|---|---|
. | National identity language usage in subsequent replies . | |||
. | In-group favoritism in replies . | Out-group hostility in replies . | ||
. | b . | SE . | b . | SE . |
Use of national identity language | ||||
In-group favoritism in posts (ref. without in-group favoritism words) | 1.823*** | 0.027 | −0.391 | 0.030 |
Out-group hostility in posts (ref. without out-group hostility words) | −0.201*** | 0.027 | 1.701*** | 0.027 |
Control variables | ||||
Content-level controls | ||||
Debunking post (ref. conspiracy post) | 0.094*** | 0.048 | 0.326*** | 0.048 |
Text length | 0.024*** | 0.001 | 0.021*** | 0.001 |
URL (ref. no URL) | −0.790* | 0.197 | 0.272* | 0.138 |
Hashtag count | −0.014* | 0.006 | 0.050 | 0.006 |
Text sentiment score | −0.016*** | 0.002 | −0.012*** | 0.002 |
User-level controls | ||||
Verified individual users (ref. = ordinary user) | −0.170*** | 0.034 | 0.007 | 0.035 |
Verified organizational users (ref. = ordinary user) | 0.030 | 0.043 | −0.086* | 0.044 |
Gender of user (ref. = female) | 0.314*** | 0.034 | −0.376*** | 0.030 |
User’s follower number | 0.010* | 0.005 | 0.057*** | 0.005 |
User’s followee number | −0.021 | 0.010 | 0.006 | 0.010 |
User’s number of historical posts | 0.031*** | 0.008 | 0.039*** | 0.008 |
Constant | −2.753*** | 0.084 | −3.020*** | 0.086 |
Pseudo-R2 | 52.9% | 53.0% | ||
N | 17,405 |
. | Model 2a . | Model 2b . | ||
---|---|---|---|---|
. | National identity language usage in subsequent replies . | |||
. | In-group favoritism in replies . | Out-group hostility in replies . | ||
. | b . | SE . | b . | SE . |
Use of national identity language | ||||
In-group favoritism in posts (ref. without in-group favoritism words) | 1.823*** | 0.027 | −0.391 | 0.030 |
Out-group hostility in posts (ref. without out-group hostility words) | −0.201*** | 0.027 | 1.701*** | 0.027 |
Control variables | ||||
Content-level controls | ||||
Debunking post (ref. conspiracy post) | 0.094*** | 0.048 | 0.326*** | 0.048 |
Text length | 0.024*** | 0.001 | 0.021*** | 0.001 |
URL (ref. no URL) | −0.790* | 0.197 | 0.272* | 0.138 |
Hashtag count | −0.014* | 0.006 | 0.050 | 0.006 |
Text sentiment score | −0.016*** | 0.002 | −0.012*** | 0.002 |
User-level controls | ||||
Verified individual users (ref. = ordinary user) | −0.170*** | 0.034 | 0.007 | 0.035 |
Verified organizational users (ref. = ordinary user) | 0.030 | 0.043 | −0.086* | 0.044 |
Gender of user (ref. = female) | 0.314*** | 0.034 | −0.376*** | 0.030 |
User’s follower number | 0.010* | 0.005 | 0.057*** | 0.005 |
User’s followee number | −0.021 | 0.010 | 0.006 | 0.010 |
User’s number of historical posts | 0.031*** | 0.008 | 0.039*** | 0.008 |
Constant | −2.753*** | 0.084 | −3.020*** | 0.086 |
Pseudo-R2 | 52.9% | 53.0% | ||
N | 17,405 |
Poisson regression models predicting the use of national identity language in replies
. | Model 2a . | Model 2b . | ||
---|---|---|---|---|
. | National identity language usage in subsequent replies . | |||
. | In-group favoritism in replies . | Out-group hostility in replies . | ||
. | b . | SE . | b . | SE . |
Use of national identity language | ||||
In-group favoritism in posts (ref. without in-group favoritism words) | 1.823*** | 0.027 | −0.391 | 0.030 |
Out-group hostility in posts (ref. without out-group hostility words) | −0.201*** | 0.027 | 1.701*** | 0.027 |
Control variables | ||||
Content-level controls | ||||
Debunking post (ref. conspiracy post) | 0.094*** | 0.048 | 0.326*** | 0.048 |
Text length | 0.024*** | 0.001 | 0.021*** | 0.001 |
URL (ref. no URL) | −0.790* | 0.197 | 0.272* | 0.138 |
Hashtag count | −0.014* | 0.006 | 0.050 | 0.006 |
Text sentiment score | −0.016*** | 0.002 | −0.012*** | 0.002 |
User-level controls | ||||
Verified individual users (ref. = ordinary user) | −0.170*** | 0.034 | 0.007 | 0.035 |
Verified organizational users (ref. = ordinary user) | 0.030 | 0.043 | −0.086* | 0.044 |
Gender of user (ref. = female) | 0.314*** | 0.034 | −0.376*** | 0.030 |
User’s follower number | 0.010* | 0.005 | 0.057*** | 0.005 |
User’s followee number | −0.021 | 0.010 | 0.006 | 0.010 |
User’s number of historical posts | 0.031*** | 0.008 | 0.039*** | 0.008 |
Constant | −2.753*** | 0.084 | −3.020*** | 0.086 |
Pseudo-R2 | 52.9% | 53.0% | ||
N | 17,405 |
. | Model 2a . | Model 2b . | ||
---|---|---|---|---|
. | National identity language usage in subsequent replies . | |||
. | In-group favoritism in replies . | Out-group hostility in replies . | ||
. | b . | SE . | b . | SE . |
Use of national identity language | ||||
In-group favoritism in posts (ref. without in-group favoritism words) | 1.823*** | 0.027 | −0.391 | 0.030 |
Out-group hostility in posts (ref. without out-group hostility words) | −0.201*** | 0.027 | 1.701*** | 0.027 |
Control variables | ||||
Content-level controls | ||||
Debunking post (ref. conspiracy post) | 0.094*** | 0.048 | 0.326*** | 0.048 |
Text length | 0.024*** | 0.001 | 0.021*** | 0.001 |
URL (ref. no URL) | −0.790* | 0.197 | 0.272* | 0.138 |
Hashtag count | −0.014* | 0.006 | 0.050 | 0.006 |
Text sentiment score | −0.016*** | 0.002 | −0.012*** | 0.002 |
User-level controls | ||||
Verified individual users (ref. = ordinary user) | −0.170*** | 0.034 | 0.007 | 0.035 |
Verified organizational users (ref. = ordinary user) | 0.030 | 0.043 | −0.086* | 0.044 |
Gender of user (ref. = female) | 0.314*** | 0.034 | −0.376*** | 0.030 |
User’s follower number | 0.010* | 0.005 | 0.057*** | 0.005 |
User’s followee number | −0.021 | 0.010 | 0.006 | 0.010 |
User’s number of historical posts | 0.031*** | 0.008 | 0.039*** | 0.008 |
Constant | −2.753*** | 0.084 | −3.020*** | 0.086 |
Pseudo-R2 | 52.9% | 53.0% | ||
N | 17,405 |
Nationalist narratives in users’ everyday posts beyond the COVID-19 pandemic
We also compared the use of national identity language in historical Weibo posts between users who had employed this language when discussing COVID-19 conspiracy theories and users who had not used this language. Our findings support H5. Among the 16,439 Weibo users, those who employed in-group favoritism language when discussing COVID-19 conspiracy theories (i.e., adopters of COVID-19 conspiracy theory in-group favoritism language) used a significantly higher amount of in-group favoritism language in their historical Weibo posts (M = 1.59) compared with non-adopters (M = 1.52) (t = −6.65, p < .001). Similarly, these COVID-19 conspiracy theory out-group hostility language adopters also presented a higher amount of out-group hostility language in their everyday social media posts (M = 1.22) compared with non-adopters (M = 1.07) (t = −11.64, p < .001).
Discussion
The purpose of this study was to examine the role of national identity language in public engagement with conspiracy theories during the COVID-19 pandemic and in users’ everyday social media posts. Drawing on the SIDE model and social identity theory and situating the discussion within the Chinese social media context, our article offers a theoretical explanation for variations of in-group favoritism and out-group hostility language in public engagement with conspiracy theories and replies. We found a contagion pattern of using national identity language in original posts and subsequent related conservations. This finding accords with the few recent studies that have identified similar patterns on Western platforms (Marchal, 2022; Rathje et al., 2021). Moreover, this implies that there is a need to expand the theorization of identity on social media engagement to gain a better understanding of its impact on social media conversations. Several other findings merit discussion, which we explain in the order of our hypotheses to highlight the contributions and limitations of our study.
First, our article proposes identity-driven communication as an important framework to examine how misinformation spreads on social media, thus extending the application of the SIDE model to misinformation studies. The results across all our hypotheses indicate that, relative to other content-level features (such as sentiment) or user-level characteristics, which have been extensively studied in the literature to predict the spread of misinformation, national identity language has a much larger association with public engagement with conspiracies, not only in engagement size but also in users’ replies. These findings not only demonstrate how to apply the SIDE model in a new context but also highlight identity-driven communication as a key motivator that should be considered when attempting to understand how misinformation and conspiracy theories spread on digital platforms.
Second, our findings also suggest the importance of theorizing about and examining any variations of in-group favoritism and out-group hostility language on different outcomes of public engagement. While the presence of in-group favoritism language was always associated with more likes, comments, and a larger diffusion size (H1), the presence of out-group hostility language displayed a heterogeneous association with public engagement size. On Western platforms, out-group hostility language has been found to increase all types of engagement size (Makki & Zappavigna, 2021; Rathje et al., 2021). However, our findings provide a more complex picture, indicating that out-group hostility language is associated with more comments and a larger diffusion size (H3b), and fewer likes (H3a). As discussed in the literature review, clicking “like” reveals supportive attitudes toward a post, suggesting that Weibo users are less likely to support posts that use out-group hostility language.
This negative relationship between out-group identity narratives and the number of likes on Chinese social media has important implications for extending our understanding of how social identity might mean different things to Western users versus Chinese social media users. Clicking likes could signal different psychological concepts in Chinese and Western cultures. In Western cultures, “like” often signals solidarity, as we observed in the George Floyd and Black Lives Matter movements. The group of users associated with the victims try to remember and hold the victims as icons rather than avoiding and forgetting their voices. Clicking likes serves as a way to show their solidarity with those social groups. By comparison, in Chinese culture, being associated with out-groups often brings a sense of humiliation (as is highlighted in the literature, many nationalist narratives are about out-group humiliation). This humiliation discourages users from showing favor toward those out-group language. Moreover, while users on Western platforms might be more polarized, consisting of both out-group and in-group members that attract likes from their own members, users on Chinese social media tend to share more similar identities that support in-group language and dislike out-group language.
This difference between the liking behaviors of Western users and Chinese users suggests that more research is required to study the role of political culture in influencing how people participate online, which is still at an early stage (Chen et al., 2020). While some conclusions about identity effects, such as the impact of in-group identity language on public engagement, might indicate similar contagion patterns across users in different cultures, other types of identity language, such as out-group identity, can indicate divergent patterns depending on users’ culture of digital participation and identification with these groups. Thus, it will be fruitful for future research to identify the underlying mechanism(s) that drive these differences in identity effects on different social media and in different socio-political environments.
Although in-group favoritism language and out-group hostility language play different roles in the number of likes and reposts through which users engage with these conspiracy theories, the contagion pattern of national identity language in users’ replies was consistent in both cases. The use of in-group favoritism language or out-group hostility language in original posts is associated with more frequent use of the corresponding identity language in all subsequent conversations (H2 and H4). This finding aligns with the strategic aspect of the SIDE model, which has received comparatively less attention in the literature. The original SIDE model provides a cognitive explanation for why people are more likely to act at the level of social group identity in CMC. However, the strategic aspect of SIDE concerns “identity performance,” referring to the communicative actions of consolidating and mobilizing identity (Klein et al., 2007). Consolidating identity includes communicating conformity to group norms and expressing popular group opinions. We demonstrated that Weibo users consolidated their identities by mimicking and conforming to group norms of language use with respect to prototypical identity words. The contagion pattern of language use in social media replies revealed in our study goes beyond the current literature, which has mostly examined contagion in terms of user engagement metrics, such as the number of views and retweets (Rathje et al., 2021). Contagion appears to operate more deliberately at a deeper cognitive level (i.e., users’ replies).
A third set of findings that merits discussion involves our analyses of users’ historical Weibo posts, which provide an understanding of the differences in general linguistic patterns between users who engaged with national identity narratives of COVID-19 conspiracy theories and users who did not (H5). These findings on users’ everyday use of nationalism language can contribute to the literature on conspiracy theories in several ways. Existing studies on misinformation have rarely examined everyday social media posting behaviors, due to the challenge of collecting large-scale historical social media data. However, without situating public engagement with misinformation in daily social media conversations, insights into how users form misbeliefs would be difficult. Our article provides the first step in addressing this issue. We found a strong correlation between the use of national identity language during COVID-19 conspiracy theory discussions and users’ daily social media posts. This prevalence of national identity language beyond politics and the COVID-19 pandemic has practical implications for misinformation correction. For instance, the use of national identity language on social media could serve as a proxy for identifying those who are likely to be susceptible to misinformation and conspiracy theories, allowing targeted interventions. Future research can go beyond analyzing national identity language use in historical posts to other linguistic characteristics, which would improve the prediction of who would be more likely to spread misinformation on social media. In other words, although user profile information is often limited on digital platforms, a linguistic analysis of what users post and in what conversations they engage would open a new avenue for understanding individuals who are at the center of misinformation discussions.
We acknowledge several limitations to this research study. First, our article focuses on how national identity narratives are spread on Weibo. We have not systematically investigated who spread these narratives. In a word, as we highlighted in the literature section, it would be valuable for future research to identify institutional-hired nationalists versus individual-based nationalists to examine the impact of their posts separately because both actors shape discourses on Chinese social media. While SIDE theory focuses more on the use of identity language than on who spreads these language, unique distinctions of users on Chinese social media could help advance SIDE theory in terms of determining whether the contagion of identity discourse is advanced more by individual-based nationalists or by paid posters. Such an analysis would also allow us to understand to what extent online users are persuaded or not by nationalist narratives from different types of users (e.g., 50 Cent Party members versus voluntary 50 Cent armies). In fact, nationalist narratives are not only promoted top-down, but also from the bottom up. As Schneider (2018) stressed, nationalism is not simply a form of top-down indoctrination and instead they are actively consumed by different stakeholders including private Internet users and commercial enterprises. In recent years, scholarship has increasingly paid attention to these individual-based users who express nationalist narratives (Zhang et al., 2018). These users are termed the “voluntary 50 Cent army” (Han, 2015). They defend the regime on an unpaid basis. Han (2015) has pointed out that these voluntary 50 Cent armies are not unthinking tools of the state. Rather, these users debate the merits and faults of leaders and post across major platforms in China. Han (2015) has highlighted the importance of acknowledging cyberspace in China as “fragmented netizen constituencies championing pluralized political values, ideas and norms” (p. 1007).
Similar to other studies based on Twitter that need to address the challenge of identifying bots, it is a persistent challenge to accurately identify government-paid accounts on Chinese Weibo. Nevertheless, for our dataset, we performed some 50 Cent Party member identification, drawing from King et al. (2017) and Miller’s (2016) methods. We found that the proportion of potential 50 Cent Party members is small (see Supplementary Appendix H).
Second, our study is based on observational data. There could be alternative explanations as to why users are more likely to use national identity language when responding to posts that utilize national identities to discuss issues related to COVID-19. For instance, one explanation could be homophily, where users who are likely to use national identity language self-select to respond to posts that discuss identities or follow others who also use such language. As results indicated, users who engaged in conspiracy theory nationalist narratives also tended to share similar content and tend to use nationalist narratives in their everyday posts. Future research could design experiments to investigate how viewing identity-focused messages causes users to respond with identity language.
Conclusion
This article theorizes and demonstrates the varying impacts of in-group favoritism versus out-group hostility language on public engagement with conspiracies on social media. By revealing the contagion cascade in social media discussions on COVID-19 conspiracies, this article advances our understanding of the strategic aspect of the SIDE model through the lens of in- and out-group communication dynamics. This article also reveals the relationships between users’ everyday conversations and their engagement with conspiracy messages, highlighting the importance to study misinformation through tracing back users’ daily social media conversations.
Funding
Anfan Chen would like to acknowledge the funding support by the National Social Science Fund of China [Grant No. 21CXW018]. Kaiping Chen would like to acknowledge the funding support by the University of Wisconsin-Madison, Office of the Vice Chancellor for Research and Graduate Education.
Data availability
The data underlying this article will be shared on a reasonable request to Anfan Chen.
Supplementary material
Supplementary material is available at Journal of Computer-Mediated Communication online.
Dimensions . | Examples . |
---|---|
In-group favoritism words | 炎黄子孙 (descendants of Civilized China), 龙的传人 (descendants of the dragon), 中华 (greater China), 华夏 (civilized China), 先辈 (ancestors), 先烈 (martyrs), 祖国 (motherland), 中国梦 (Chinese dream), and 长江文明 (Yangtze River civilization) |
Out-group hostility words | 帝国 (empire), 洋人 (outlanders), 外国势力 (foreign powers), 国外势力 (foreign powers), 西方势力 (Western powers), 境外势力 (foreign forces), 白左 (white left), 八国联军 (the Eight-Power Allied Forces), 列强 (imperialist powers), 昂撒 (Angsa), and 白皮猪(white pig) |
Dimensions . | Examples . |
---|---|
In-group favoritism words | 炎黄子孙 (descendants of Civilized China), 龙的传人 (descendants of the dragon), 中华 (greater China), 华夏 (civilized China), 先辈 (ancestors), 先烈 (martyrs), 祖国 (motherland), 中国梦 (Chinese dream), and 长江文明 (Yangtze River civilization) |
Out-group hostility words | 帝国 (empire), 洋人 (outlanders), 外国势力 (foreign powers), 国外势力 (foreign powers), 西方势力 (Western powers), 境外势力 (foreign forces), 白左 (white left), 八国联军 (the Eight-Power Allied Forces), 列强 (imperialist powers), 昂撒 (Angsa), and 白皮猪(white pig) |
Dimensions . | Examples . |
---|---|
In-group favoritism words | 炎黄子孙 (descendants of Civilized China), 龙的传人 (descendants of the dragon), 中华 (greater China), 华夏 (civilized China), 先辈 (ancestors), 先烈 (martyrs), 祖国 (motherland), 中国梦 (Chinese dream), and 长江文明 (Yangtze River civilization) |
Out-group hostility words | 帝国 (empire), 洋人 (outlanders), 外国势力 (foreign powers), 国外势力 (foreign powers), 西方势力 (Western powers), 境外势力 (foreign forces), 白左 (white left), 八国联军 (the Eight-Power Allied Forces), 列强 (imperialist powers), 昂撒 (Angsa), and 白皮猪(white pig) |
Dimensions . | Examples . |
---|---|
In-group favoritism words | 炎黄子孙 (descendants of Civilized China), 龙的传人 (descendants of the dragon), 中华 (greater China), 华夏 (civilized China), 先辈 (ancestors), 先烈 (martyrs), 祖国 (motherland), 中国梦 (Chinese dream), and 长江文明 (Yangtze River civilization) |
Out-group hostility words | 帝国 (empire), 洋人 (outlanders), 外国势力 (foreign powers), 国外势力 (foreign powers), 西方势力 (Western powers), 境外势力 (foreign forces), 白左 (white left), 八国联军 (the Eight-Power Allied Forces), 列强 (imperialist powers), 昂撒 (Angsa), and 白皮猪(white pig) |
Notes
The selection of 700 recently published posts of each user was determined by an empirical study by Kawo (Alex, 2019), a Chinese social media management platform. The study indicated that over 50% of accounts published an average of once per day or less, which means 700 posts per user cover approximately a 2-year range of their historical published posts.
Chi-squared (Franke et al., 2012) (χ2) tests were performed to identify whether the adoption of in-group favoritism language in posts had any significant associations with out-group hostility language in posts. The test results indicate that in-group favoritism language adoption in posts have no significant association with out-group hostility language adoption (χ2 = 2,959.29, p > .05), indicating there was no significant association between the two types of language adopted in posts.
References
Sina Weibo (
Author notes
Anfan Chen and Kaiping Chen contributed equally to this work.