-
PDF
- Split View
-
Views
-
Cite
Cite
S Mo Jones-Jang, Yong Jin Park, How do people react to AI failure? Automation bias, algorithmic aversion, and perceived controllability, Journal of Computer-Mediated Communication, Volume 28, Issue 1, January 2023, zmac029, https://doi.org/10.1093/jcmc/zmac029
- Share Icon Share
Abstract
AI can make mistakes and cause unfavorable consequences. It is important to know how people react to such AI-driven negative consequences and subsequently evaluate the fairness of AI’s decisions. This study theorizes and empirically tests two psychological mechanisms that explain the process: (a) heuristic expectations of AI’s consistent performance (automation bias) and subsequent frustration of unfulfilled expectations (algorithmic aversion) and (b) heuristic perceptions of AI’s controllability over negative results. Our findings from two experimental studies reveal that these two mechanisms work in an opposite direction. First, participants tend to display more sensitive responses to AI’s inconsistent performance and thus make more punitive assessments of AI’s decision fairness, when compared to responses to human experts. Second, as participants perceive AI has less control over unfavorable outcomes than human experts, they are more tolerant in their assessments of AI.
Lay Summary
As artificial intelligence (AI) is replacing important decisions that used to be made by human experts, it is important to study how people react to undesirable outcomes caused by AI-made decisions. This study aims to identify two critical psychological processes that explain how people evaluate AI-driven failures. The first mechanism is that people have high expectations of AI’s consistent performance (called automation bias) and then are frustrated by unsatisfactory outcomes (called algorithmic aversion). The second mechanism is that people perceive that AI has less control over negative outcomes, compared to humans, which in turn, reduces negative evaluations of AI. To demonstrate these two ideas, we used two online experiments. Participants were exposed to several scenarios where they experienced undesirable outcomes from either AI or human experts.
Negative events carry a much larger weight than positive events (Tversky & Kahneman, 1985). Despite all of the benefits that people appreciate from Artificial Intelligence (AI), one single unpleasant encounter can turn their appreciation into an aversion to AI. Such a negative experience has a significant influence, especially when high-stake decisions are involved, such as medical, financial, hiring, and college admission decisions. Thus, this article examines how people perceive and evaluate AI after experiencing negative effects generated by AI.
Unlike previous research that has examined whether people favor AI over human agents (Araujo et al., 2020; Logg et al., 2019; Pew Research Center, 2018; Sundar & Kim, 2019; Wojcieszak et al., 2021), this study aims to identify and theorize the psychological paths that people take after experiencing negative events caused by AI’s decisions. Specifically, we examine how two types of AI heuristics will influence the evaluation mechanism. First, drawing on the literature on machine heuristics and automation bias (Burton et al., 2020; Sundar, 2020), we investigate how perceived violations of the perfection scheme about AI’s programmed, consistent performance negatively influence users’ fairness assessments of AI-made decisions. We expect to find that people’s initial positive assumptions of AI’s consistent performance (i.e., automation bias) will be replaced by acute disappointment over disappointing results (i.e., algorithmic aversion). Another theoretical account depends on the attribution framework to explain how people perceive an actor’s controllability over an outcome, which leads to a subsequent judgment of the actor (Burton et al., 2020; Weiner, 2006). As a result, we investigate how people’s perceptions of AI’s controllability over a negative consequence affect the fairness evaluation of AI-made decisions. It will be interesting to see if people regard AI as more controllable because of its autonomous character or as less controllable since AI is programmed and adopted by humans or organizations (Shank & DeSanti, 2018). We conducted two online experiments to demonstrate how two theorized mechanisms underpin people’s fairness assessment of AI.
Literature review
AI: a machine thinking like a human
AI is defined as a machine agent that is intelligent enough to think and act (Garnham, 1987; Russell & Norvig, 2009). Unlike other technological advances, AI is supposed to function like a human being with a certain degree of autonomous capability, which allows AI to make informed decisions based on a scanned environment. Interactions with AI are also designed to be felt like human-to-human interactions since AI agents are developed to be more intimate, proximate, embodied, and human-like (Guzman, 2020; Sundar, 2020; Westerman et al., 2020).
As AI agents display both human-like aspects and machine-like identities, it has been interesting to see how people perceive AI agents. Earlier research since the 1990s mostly highlighted that people interact with AI following socio-psychological principles that are applied to human-to-human interactions (Reeves & Nass, 1996). This suggests that people perceive and respond to computers as if they are social actors. Empirical evidence showed that people displayed gender, racial, and group biases and expressed typical emotions toward AI agents (Klein et al., 2002; Lee & Nass, 2002).
While this line of work shows that AI agents are perceived like human agents in numerous aspects, the current study focuses on distinct perceptions and reactions toward AI agents, compared to human agents. This study is interested in the defining characteristics of AI that make people perceive it as machines distinguished from human agents and how such AI-specific beliefs (i.e., machine heuristics) lead to different evaluations of AI-made (compared to human-made) decisions. The following section will review several key considerations that theorize the user’s mental model of AI agents and systems. Among them, this study focuses on automation bias and algorithmic aversion as well as perceived controllability.
Initial perceptions of AI: machine heuristics
Although AI has both human-like and machine-like features, people often focus on AI’s machine-like characteristics, ignoring its autonomous capability. Thus, once the source of interaction is identified as AI, people tend to evaluate the interaction based on machine characteristics that spring to their mind (Sundar, 2020). This psychological mechanism has many parallels with the explanation of social cognition, which describes people as cognitive misers (Fiske & Taylor, 2013). Instead of going through effortful assessment, people tend to rely on mental heuristics that are triggered by contextual cues.
There are several reasons why users tend to rely on mental shortcuts rather than effortful assessments about AI’s expected performance. First, from users’ view, AI is a black box (Shin & Park, 2019). Although the explainable AI movement (XAI) urges the need for transparency in the creation and application of AI, it is almost impossible for users to know how AI is programmed behind the interface. Second, despite recent surges of AI applications, many users still have little direct interactions with AI agents. Without users’ prior experiences and cognitive processes, their understanding of AI is bound to be highly subjective (Just et al., 2018). Such lack of transparency and prior direct experiences lead to the idea that people’s initial perceptions of AI are often shaped through media presentations of AI (Banks, 2020). People’s initial perceptions of machines or AI commonly involve the belief that AI functions in a preprogrammed, objective, and consistent fashion (Sundar & Kim, 2019).
The literature on machine heuristics suggests that the judgment process follows the if-then-therefore logic (Bellur & Sundar, 2014). For example, if source cue (AI) is recognized, then machine heuristics (programmed and consistent performance) are triggered, therefore, influencing the user’s judgment of AI experience (Cloudy et al., in press). It is worth noting that machine heuristics do not always automatically or uniformly emerge. Researchers claim that proper cues should be visually accessible to trigger machine heuristics. Moreover, the same cue may prompt different heuristics depending on contexts and users (Bellur & Sundar, 2014). Therefore, according to this literature, a recommended practice in machine heuristic research is that cues, machine heuristics, and judgments are directly measured and treated as different variables (Cloudy et al., in press).
Heuristic #1: automation bias and algorithmic aversion
Research has shown that two opposite perceptions of AI emerge as a function of heuristics-based evaluation mechanisms: automation bias and algorithmic aversion (Logg et al., 2019). Automation bias occurs when users overestimate AI’s consistent performance and accuracy, forming the perfection scheme about AI’s performance (Cummings, 2017; Lee, 2018). Experimental evidence indicates that people are more likely to take advice from AI than from humans in terms of forecasting and music recommendations (Logg et al., 2019). Additionally, research found that AI-authored stories and fact-checking messages led users to reduce partisan biases and subsequently rate AI-authored messages more credible than human-authored stories (Cloudy et al., in press; Moon et al., in press; cf., Wojcieszak et al., 2021). In practice, AI-authored or AI-feeding information is not free from biases due to the nature of the data that drive AI decisions (Hsu, 2020; Noble, 2018). However, most users are not familiar with such AI-driven implicit biases and perceive AI to be neutral.
Unlike an automation bias that reflects users’ positive expectations of AI’s programmed performance, algorithmic aversion occurs when cueing the machine heuristic leads to aversive reactions to AI (Dietvorst et al., 2015). Research has reported the evidence of algorithmic aversion by revealing the user’s tendency to favor human judgments over AI even when AI performs better (Alvarado-Valencia & Barrero, 2014; Bucher, 2017; Dietvorst et al., 2015). Often cited reasons for algorithmic aversion stems from the violation of the AI’s perfection scheme (Dietvorst et al., 2015; Highhouse, 2008), the presumed incapability of AI to integrate contextual factors (Grove & Meehl, 1996), and concerns about ethical issues of relying on machines to make important decisions (Dawes, 1979).
The key difference in perceptions of AI and human agents is that users have falsely high expectations of AI’s pre-programmed and consistent performance, resulting in greater disappointment regarding the AI’s unsatisfactory outcome (Alvarado-Valencia & Barrero, 2014). Therefore, we theorize that positive and negative assessments of AI, represented by automation bias and algorithmic aversion, are not necessarily contradicting notions, but sequential processes in the course of AI use. Empirical evidence supports this idea that falsely high expectations of AI’s consistent performance (automation bias) are a key driver of subsequent negative evaluations of AI. For example, framing the robot as possessing low technical capabilities (setting low expectations) yield less disappointment and more favorable appraisals of the robot’s competence and social interactions, compared to when setting high expectations of the robot’s capabilities (Groom et al., 2011; Paepcke & Takayama, 2010). It seems that users have a perfection scheme about AI such that AI should function perfectly every time. Then, the violation of such high expectations dramatically decreases the users’ subsequent preference of AI. Experiencing AI failures, users are likely to shun their use of AI. In contrast, as users have more realistic expectations of human agents and acknowledge that humans occasionally exhibit errors of judgment, they tend to show increasing tolerance over human-made mistakes compared to AI-made mistakes.
Fairness assessment
The significance of fairness assessments of AI has been highlighted in recent studies (e.g., Helberger et al., 2018; Sandvig et al., 2016). Fairness in AI means a perceived expectation that AI should not render unjust, poor, or discriminatory decisions (see Yang & Stoyanovich, 2017). Studies (Shin & Park, 2019; Thurman et al., 2019) found that in influencing the use of AI and the reliance on its decisions, fairness has emerged as the key normative expectation—especially because users face the “black-box” uncertainties of machine-based decision systems. That is to say, unlike human-based decisions, the expectation for fairness can be even more critical (thus, normatively punitive) when users have to rely on machines for important decisions which they cannot influence, but still attempt to understand.
After all, user experiences with the AI agents may be fundamentally based on their subjective assessments and expectations, and fairness can be a salient attribute in this process, particularly for the machine decisions which disfavor them (Hoffmann, 2019; Yang & Stoyanovich, 2017). Note that this assessment of fairness is different from the evaluation of AI agents for their accuracy. Rather, the concern is in the case of AI’s “bad” decisions which are likely to give rise to calls for better explanations. This demand for fairness can be a functioning barometer in users’ quest for how to perceive unfavorable decisions that are not human-made (Dörr & Hollnbuchner, 2017).
Therefore, our test is to examine how people assess fairness for the AI agent, differently from the human agent, but the key is to identify how the notion of automation bias and algorithmic aversion jointly explains the evaluation mechanism. Specifically, we anticipate that as people perceive AI to be more likely than humans to perform consistently (automation bias), the user is more likely to provide a negative fairness assessment of AI’s wrong decisions (algorithmic aversion).
H1: People will show more negative fairness assessments of the AI agent, compared to the human agent (algorithmic aversion), through the perception that their initial expectations of AI’s consistent performance (automation bias) have been violated.
Heuristic #2: perceived controllability
Another common belief of AI that may influence the judgment of AI performance is related to perceived controllability. Previous literature on machine heuristics has not paid much attention to the concept of perceived controllability in the evaluation of AI, but this article proposes that the perceived degree to which AI has control over the outcome is one of the crucial machine heuristics that users initially have and will influence subsequent judgment of AI, including the fairness assessment.
Attribution framework (Weiner, 2006) theorizes how individuals’ positive or negative appraisals of certain actors hinge on whether target actors are perceived to have control over the consequence. If a negative outcome is perceived to be beyond an actor’s control, the actor gets less blame. In contrast, if the same negative outcome is perceived to be under the actor’s control, the actor gets more blame. For example, attributing a poor outcome to controllable factors such as insufficient efforts or mistakes of the actor generates more punitive evaluations of the actor but to uncontrollable factors such as luck, external factors generate more sympathetic, positive evaluations (Weiner, 2006).
Applying this logic to the AI context, users’ evaluations of AI will depend on how they perceive controllability of AI over the outcome. The literature indicates that AI and robots are not perceived to have a level of control on par with humans (Stowers, 2017). Users often believe that the locus of control lies outside the AI, and targeted blame is diffused to other proximate agents such as organizations who adopt AI, software developers who program it, or the user who decides to interact with it (Shank & DeSanti, 2018). As a result, AI that is perceived to have less controllability may get less blame for negative consequences, compared to humans who are considered to have more control. Additional research into this reasoning showed that users tend to ascribe negative results when AI is perceived more autonomous than when it is perceived as less autonomous (van der Woerdt & Haselager, 2016). These findings suggest that perceived controllability serves as a key psychological factor that generates a generous or punitive assessment for AI failure.
Interestingly, there is an alternative view that AI is perceived to have a certain degree of control over the outcome because AI is supposed to have an independent and autonomous nature relative to other machines (van der Woerdt & Haselager, 2016). However, this comparison is between AI agents and other machines, not between AI and human agents. Thus, despite the perception that AI possesses some levels of intelligence and autonomy, many people still perceive AI to lack complete independence and have doubts that AI can replace human intelligence and take responsibility (Sundar, 2020). Thus, we anticipate that if people have heuristics that AI has less control over the outcome, they will assign less blame for negative consequences, compared to human actors.
H2: People will perceive that the AI agent, compared to the human agent, should have less control over the outcome, which in turn leads to generous (or less negative) fairness assessment of AI for an unsatisfactory outcome.
Study 1
Method
Participants
A national sample of adult U.S. citizens was recruited by a survey firm, Dynata (formerly Survey Sampling International). The quota for key demographic variables, including age, gender, race, and location participants was used. The study comprised 602 respondents, but screened out those who failed to pass the attention and manipulation check, resulting in a final sample of 541 participants. Their mean age was 46.50 (SD = 16.24), and 50% were female. About 48.3% of the sample had 4-year or higher-level college education, and the median category of household income was between 50,000 USD and 74,999 USD.
Procedure and manipulation
Participants were randomly assigned to either a human or AI condition. Those in the human condition read a vignette about the failure of a human actor and those in the AI condition read a scenario about the failure of an AI actor. We created two topical contexts where doctors and juries were manipulated as human and AI actors.
The following shows the vignette for the medical scenario:
The patient was a 46-year-old man who suffered from headaches and dizziness. Dr. Smith, a veteran brain surgeon who graduated from Harvard medical school in 2004 (or an AI Robot Doctor in the AI condition), recommended a brain surgery. The patient agreed, and Dr. Smith (or an AI Robot Doctor) performed brain surgery. Unfortunately, the operation did not go well. The patient died two weeks after the surgery.
The following shows the vignette for the legal scenario:
You initiated a civil lawsuit against the defendant who stole your lifetime savings of half a million dollars. In court, the jury consisting of 12 people (or the AI Robot jury) made decisions after jury deliberation. Unfortunately, the verdict was not in favor of you. You lost the case entirely.
After reading the vignette, participants were asked to fill out survey questions. As two topical contexts yield the same results, we combined them in our analysis. For example, the key variables in our study did not differ across scenarios: fairness, M = 2.51 vs. 2.48, F(1, 540) = 0.067, p = .80; perceived consistent performance, M = 2.65 vs. 2.77, F(1, 540) = 1.05, p = .31; perceived controllability, M = 3.60 vs. 3.45, F(1, 540) = 1.87, p = 0.17
Note that we did not include a control condition in the design. It was almost impossible to think of the possibility that people would perceive doctors or juries as something else other than human doctors or human juries. Therefore, we assume that the human condition serves as a proxy of the control condition in our investigation.
Attention and manipulation check
We used one attention check item in the middle of the survey to immediately disqualify participants. We asked them to select “Extremely Likely” in the item. For the manipulation check, we asked participants: “Which of the following made the decisions in the situations that you read?” and provided a response option between humans and AIs. We removed 61 individuals who failed to pass the attention (n = 40) and manipulation check (n = 21).
Dependent variable: fairness
Adapting from prior measures (Campbell, 1999), participants were asked (a) whether they consider the doctor’s treatment (jury’s verdict) as fair and (b) whether the situation is fair. The response varied from 1 (very unfair) to 5 (very fair) (M = 2.49, SD = 1.24, r = .80).
Mediated Variable #1: perceived consistent performance
Participants were asked to indicate the degree to which they evaluate the consistent performance of the actor by answering the following question: How much do you think the doctor (the jury) performed the task consistently in the scenario? The response ranged from 1 (not at all) to 5 (extremely) (M = 2.71, SD = 1.39).
Mediated Variable #2: perceived controllability
Perceived controllability was assessed using an item: How much do you think the doctor (the jury) has control in determining the outcome of the medical treatment (the lawsuit)? The response ranged from 1 (not at all) to 5 (extremely) (M = 3.53, SD = 1.22).
Results
Analytic approach
To test the proposed model of mediation, we used the PROCESS SPSS macro, which uses Ordinary Least Squares (OLS) path analyses (Hayes, 2017). As we assessed two mediation paths, we used the PROCESS Model 4. This analysis utilized a bootstrapping approach, providing the confidence interval (CI) for the indirect effect of having AI or human agents on the evaluation of the agent’s fairness through perceived consistent performance and perceived controllability. The bootstrapping method randomly selects cases from the sampled data. We generated 10,000 datasets, and each dataset estimated the indirect effect of the potential mediator. The effects are deemed significant if the CIs do not include zero.
Overall human and AI comparison
Before testing the mediation hypothesis, we compared the human and AI group condition. People perceived that human agents (M = 2.72, SD = 1.26) were fairer than AI agents (M = 2.28, SD = 1.19), F(1, 601) = 17.43, p <.001.
Testing the mediation hypothesis
The primary goal of this study is to identify the causal mechanism of positive or negative evaluations of AI agents, compared to human agents. This study focused on two mediating factors, perceived consistent performance and perceived controllability. To test the proposed mediating model and estimate the indirect effect, we used the PROCESS Model 4 (Hayes, 2017). We entered two manipulated conditions (Human vs. AI) as an independent variable and fairness as a dependent variable. Perceived consistent performance and perceived controllability were entered as mediators.
We predicted that these two mediators play significant roles but in a different direction. That is, while the belief that AI performs in a consistent manner results in negative evaluations of AI, the belief that AI does not have much control over the outcome results in positive evaluations of AI in the same scenario. The PROCESS analysis confirmed these predictions (H1 and H2). Table 1 summarized the direct and indirect effects of the AI condition on fairness assessment through two mediators, perceived consistent performance and perceived controllability. As noted in the first row in Table 1, the total effects of the AI condition, compared to the human condition were negative, indicating that people’s fairness assessment of the actor was even more negative for the AI agent than the human agent. Then, the indirect effect through two mediators showed the opposite direction. First, the negative indirect effect through perceived consistent performance indicated that people made harsh evaluations of the AI agent because they perceived that the initial perfect scheme of the AI was significantly violated. Second, the positive indirect effect through perceived controllability indicated that people in the AI condition perceive the actor in the scenario to have less control over the outcome than those in the human condition, contributing to a less negative fairness assessment. When comparing these two indirect effects, perceived consistent performance seemed to show stronger mediation effects than perceived controllability. This reflects the idea that people may have not-so-clear beliefs about who should be responsible for the AI-driven negative results.
Total and indirect effects of interactions with AI on fairness evaluation (Study 1)
. | Effect . | SE . | CI . |
---|---|---|---|
Total effects | −0.44 | 0.11 | [−0.65, −0.23] |
Indirect effects | |||
Controllability | 0.03 | 0.02 | [0.00, 0.06] |
Perceived consistent performance | −0.10 | 0.04 | [−0.18, −0.04] |
. | Effect . | SE . | CI . |
---|---|---|---|
Total effects | −0.44 | 0.11 | [−0.65, −0.23] |
Indirect effects | |||
Controllability | 0.03 | 0.02 | [0.00, 0.06] |
Perceived consistent performance | −0.10 | 0.04 | [−0.18, −0.04] |
Note. Unstandardized coefficients reported. Standard errors in parentheses. 95% CIs in square brackets.
Total and indirect effects of interactions with AI on fairness evaluation (Study 1)
. | Effect . | SE . | CI . |
---|---|---|---|
Total effects | −0.44 | 0.11 | [−0.65, −0.23] |
Indirect effects | |||
Controllability | 0.03 | 0.02 | [0.00, 0.06] |
Perceived consistent performance | −0.10 | 0.04 | [−0.18, −0.04] |
. | Effect . | SE . | CI . |
---|---|---|---|
Total effects | −0.44 | 0.11 | [−0.65, −0.23] |
Indirect effects | |||
Controllability | 0.03 | 0.02 | [0.00, 0.06] |
Perceived consistent performance | −0.10 | 0.04 | [−0.18, −0.04] |
Note. Unstandardized coefficients reported. Standard errors in parentheses. 95% CIs in square brackets.
Study 2
Rationale of Study 2
The major finding of Study 1 was to examine two psychological mechanisms through which users evaluate the fairness of the AI agent’s decision after negative consequences. While perceived controllability has been well theorized and demonstrated in attribution theory, much less has been done about perceived consistent performance. Thus, we conducted a follow-up experiment (Study 2) to examine the significant role of this concept. Specifically, the goal of Study 2 is to ascertain that the perfection scheme is uniquely working with the AI agent, not with the human agent.
First, like in Study 1, we showed two scenarios (legal and job interview contexts) where people experience negative consequences from either a human or AI agent. Then, we further manipulated the amount of violated expectations (perfect scheme) for human and AI agents. For example, the high violation of perfect scheme conditions presented the scenario where their success was 97% expected (thus, the failure significantly violates the expectation). On the other hand, the low violation of perfect scheme conditions presented the scenario where their success was only 50% expected (thus, the failure does not significantly violate the expectation). The logic is, people will be more frustrated when the perfect scheme is violated and this tendency is uniquely relevant in the case of the AI agent condition. Thus, we hypothesized the following prediction:
H3: People will consider the AI’s decision unfair as their expectation of the AI’s performance is more significantly violated, compared to less significantly violated. This pattern will occur to the AI agent but not to the human agent.
Method
Participants
Like Study 1, we used a national U.S. sample from Dynata. The study initially comprised 621 respondents, but the attention check led to a final sample of 599 participants. Their mean age was 46.66 (SD = 16.13), and 50.0% were female. About 45.8% of the sample had 4-year or higher-level college education, and the median category of household income was between 50,000 USD and 74,999 USD. We used the same attention check item used in Study 1.
Procedure and manipulation
Participants were randomly assigned into one of eight groups, 2 (AI vs. human agent) × 2 (high vs. low violation of perfect scheme) × 2 (two situations: legal and job interview). However, as our analysis yielded identical results across the two decision situations, we combined the two situations in our statistical analysis. All participants were presented with a vignette about the negative consequences of legal or interview situations but were manipulated in terms of the agent (i.e., human vs. AI) and the level of violation of expectation (high vs. low). The structure of the vignette is similar to one used in Study 1, but the manipulation of the level of violation of expected success was added. The following illustrates two versions of vignettes for the interview scenario. We used a job interview scenario instead of a medical scenario because the AI system is more widely used in the job interview screening procedure.
When the level of violation of expected success is high:
You applied for your dream job. Experts in the field anticipated that you would get this job 97% because of your outstanding industry experiences and academic background. A HR (human resources) person interviewed you for about 30 minutes. Unfortunately, the result of the interview was not desirable. You did not get a job offer.
When the level of violation of expected success is low:
You applied for your dream job. Experts in the field anticipated that you would get this job 50% given your outstanding industry experiences and academic background. A HR (human resources) person interviewed you for about 30 minutes. Unfortunately, the result of the interview was not desirable. You did not get a job offer.
After reading vignettes, participants were asked to fill out survey questions.
Measures
Fairness
We measured participants’ perceived decision fairness using the same two items in Study 1. The response varied from 1 (very unfair) to 5 (very fair) (M = 2.50, SD = 1.17, r = .83).
Results
To assess whether the evaluation of the agent (human vs. AI) is contingent upon the violation of the expected performance, we conducted a two-way ANOVA where perceived fairness was the dependent variable and the agent type (human vs. AI), the violation of the expected performance, and their interaction functioned as fixed factors. Figure 1 illustrated the results. To confirm our predictions, we should observe the interaction effects between two manipulated factors on perceived fairness.
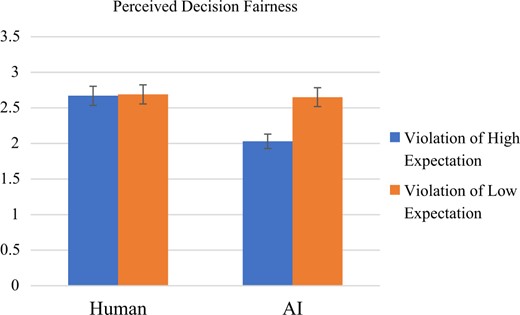
Fairness assessments by the level of violation of expected success and Human/AI experimental condition (Study 2).
Main effect
The ANOVA results revealed the significant main multivariate effect of two manipulated conditions, the agent type (human vs. AI) and the violation of the expected success on perceived fairness. First, the agent type has significant influence on the dependent variable, F(3, 595) = 13.45, p < .001, partial eta2 = .02. Specifically, univariate tests showed that the AI agent generated lower perceived fairness (M = 2.33, SD = 1.18), F(1, 597) = 18.25, p < .001, compared to the human agent (perceived fairness: M = 2.33, SD = 1.18). This confirmed the finding of Study 1, indicating that in general, people make harsher assessments for AI-driven failures.
Second, the manipulation of violation of expected success also produced the main effect, F(1, 595) = 11.99, p < .001, partial eta2 = .02. When the perfect scheme was highly violated, the evaluation of the agent seemed to be harsh, yielding lower perceived fairness (M = 2.34, SD = 1.16), compared to when the perfect scheme was significantly violated (M = 2.67, SD = 1.15), F(1, 597) = 11.88, p = .001.
Interaction effect
To ascertain whether the effects of violation of the perfect scheme on fairness assessments only occur for AI agents, not for human agents, we need to find a significant interaction effect between the agent type and the violation of the perfect scheme. Our results found a significant interaction effect, F(3,595) = 10.02, p = .002, partial eta2 = .017. As shown in Figure 1, individuals tended to make similar evaluations of human and AI agents when the violation of the perfect scheme was low (low violation). However, individuals tended to make significantly harsher evaluations for the AI’s decision in case the negative consequence was never anticipated (high violation). Therefore, these results support H3.
Discussion
This article set out to identify and examine the working mechanisms through which users assess AI for AI-driven negative outcomes. The results from Study 1 and Study 2 suggest that two psychological paths, perceived violations of expected consistent performance and perceived controllability of AI, significantly determine the fairness judgment of AI-made decisions. Specifically, we found that users were sensitive to the violation of the perfection scheme for AI. When users perceived that AI failed to deliver an expected performance, they tended to make harsher assessments of AI failures, compared to human failures. Additionally, participants perceived that AI had less controllability for negative outcomes, compared to humans, which in turn, showed tolerable evaluations of AI failures. Theoretical contributions are discussed below.
Our findings generally suggest that participants had the tendency to make more punitive evaluations of AI’s failure, compared to humans’ failure in our experimental scenarios in both Study 1 and 2. After experiencing the same disastrous events, participants reported their judgment that AI agents’ decisions were unfairer than human agents’ decisions. While the literature is mixed concerning the user’s preference of AI and human agents, our results indicate that many individuals still have reluctance and distrust of AI use in high-stakes decision contexts. The findings particularly resonate with previous research that indicated that participants become increasingly aversive for the use of AI after realizing the imperfection of AI performance (Dietvorst et al., 2015). On the other hand, the current findings are at odds with the evidence of algorithmic appreciation where users prefer taking algorithmic advice to human advice (Logg et al., 2019). This discrepancy may be attributed to the fact that our scenarios included high-stakes decision cases (e.g., legal, medical, hiring decisions), compared to Logg et al.’s (2019) research (e.g., forecasting and music recommendations). Overall, it is beyond the scope of our research to offer a generalizable conclusion of whether people prefer humans to AI or vice versa. As mixed literature suggests, such preference could be highly context-dependent. It is unclear whether algorithmic aversion witnessed in this study is due to the experimental scenario’s seriousness, participants’ exposure to AI’s error, or unfamiliarity of participant’s interactions with AI in such contexts.
Rather, the primary goal of this study is to demonstrate the psychological principle that leads to users’ evaluation of AI failures. We believe that such underlying principles are much less susceptible to varying contexts than people’s preference between AI and human agents. Understanding principles will also inform us how and why people’s judgment of AI differ. The first mechanism we focused on was the role of the perfection scheme about AI and violation of the expectation. By linking automation bias and algorithmic aversion in a sequential chain, we reason that users provide penalized responses to AI-driven failures when users’ initial high expectations of AI's consistent performance (automation bias) are violated (algorithmic aversion).
Study 1 showed that people were increasingly sensitive to the violation of the perfection scheme when the agent was AI. Participants reported that the actor’s performance was evaluated as less consistent when the actor was an AI agent than when it was a human agent. Then, such discriminating perceptions for the same negative outcome resulted in the belief that AI’s decision was unfairer than humans’ decision. The results suggest that people are more disappointed when facing AI’s inconsistent performance, which leads us to assume that people have high expectations of AI’s consistent performance.
In Study 2, we wanted to demonstrate that users’ evaluation of AI is significantly impacted by the level of prior expectation and that this tendency is uniquely shown in the AI condition, not in the human condition. The findings confirmed this by showing that participants’ fairness assessment was particularly poor when the AI agent was supposed to show perfect performance (97% condition) than when the AI agent was reasonably expected to show poor performance (50% condition). Interestingly, such initial baseline expectations did not influence participants’ evaluations for the human agent. Taken together, this study indicates that the violation of the perfect scheme plays a significant role uniquely in the evaluation of AI-made decision or performance, but not in the evaluation of human-made decision or performance. It also suggests that one may attempt to decrease algorithm aversion by lowering the people’s expectation of AI or disclosing the possibility that AI can err.
Another key mechanism for AI evaluation was triggered by how people perceive AI’s controllability over the outcome. Following the attribution literature, people are expected to make increasingly harsh evaluations for the negative result when the agent has full control over the outcome. In contrast, when users attribute the same negative outcome to something outside the agent’s control, they emancipate the agent from responsibility. Our results showed that AI was perceived to have less control over the outcome, compared to humans, and this perception led to more positive or tolerable evaluations of AI-driven unsatisfactory outcomes. This reflects the idea that people often attribute AI failure to other entities such as programmers, organizations who employ AI, or users themselves who decide to interact with it (Shank & DeSanti, 2018). Additionally, the findings are in line with the effort that AI systems should be designed to encourage users’ participation, collaboration, or partial control over the outcome (Sundar, 2020).
Our findings suggest that despite AI’s human-like features, many people still consider AI’s machine-like features when responding to AI’s performance. Given that perceived controllability could be a manipulatable belief and people’s perception of AI will also vary over the development and adoption of AI, it will be interesting to see the changing role of perceived controllability in the evaluation of AI.
Overall, our findings highlight intriguing phenomena that indicate AI’s distinct qualities that other technologies lack. Unlike other technologies, AI is recognized to think and act like a human being with a high amount of autonomy, allowing AI to make informed, independent judgments while also making unanticipated mistakes (Guzman, 2020; Sundar, 2020; Westerman et al., 2020). To demonstrate AI’s human-like nature, we utilized high-stakes scenarios in which human experts had a monopolized position and where the decision may have life-changing repercussions. Our combined findings from Studies 1 and 2 indicate that, despite AI’s half-human, half-machine nature, users still place a high value on machine-like features when making key AI decisions.
Several limitations should be noted. First, although we used multiple decision contexts to avoid biases from research with single-stimuli design, additional empirical evidence would enhance the confidence of our findings. As AI is still new in many contexts, people may have varying perceptions and limited prior experiences about AI, which poses a challenge to the generalizability of the findings. Second, although we attempted to maximize the experimental control across human and AI conditions, there are some areas where the control could have been further executed. For example, while we provided some concrete information about human agents in the experimental scenarios (e.g., Harvard graduate), we offered less information regarding the expert level of the AI agent. We were cautious to disclose possibly confusing information about the AI agent because there is no publicly accepted criteria that demonstrate the AI agent’s expert level. Furthermore, because AI decisions are based on massive amounts of data and intricate algorithms, we attempted to construct a human agent with the maximum level of intelligence and authority. For example, the memorable Go match in which an AI-based Alphago defeated Sedol Lee, a world champion Go player, four to one, reminded us of how a human agent should be presented in a comparable manner. Future experiments with varied stimuli will increase our confidence in the interpretation of the findings. Third, some of our measures used single-item measures, creating some concerns for measurement validity and reliability. Moreover, as participants were exposed to a single vignette, it was difficult for them to evaluate how consistent the performance was. Finally, we assumed that people’s existing machine heuristics will be almost automatically activated when they are exposed to AI-starring scenarios. However, as cues do not always trigger strong heuristics and even the same cue may promote different heuristics across individuals, it is recommended that future research treat the activation of heuristics as a variable and directly measure it (see for a review, Bellur & Sundar, 2014).
Data Availability
The data underlying this article are available at https://doi.org/10.7910/DVN/9NYMH0.