-
PDF
- Split View
-
Views
-
Cite
Cite
Megan E Salwei, Carrie Reale, Workflow analysis of breast cancer treatment decision-making: challenges and opportunities for informatics to support patient-centered cancer care, JAMIA Open, Volume 7, Issue 2, July 2024, ooae053, https://doi.org/10.1093/jamiaopen/ooae053
- Share Icon Share
Abstract
Decision support can improve shared decision-making for breast cancer treatment, but workflow barriers have hindered widespread use of these tools. The goal of this study was to understand the workflow among breast cancer teams of clinicians, patients, and their family caregivers when making treatment decisions and identify design guidelines for informatics tools to better support treatment decision-making.
We conducted observations of breast cancer clinicians during routine clinical care from February to August 2022. Guided by the work system model, a human factors engineering model that describes the elements of work, we recorded all aspects of clinician workflow using a tablet and smart pencil. Observation notes were transcribed and uploaded into Dedoose. Two researchers inductively coded the observations. We identified themes relevant to the design of decision support that we classified into the 4 components of workflow (ie, flow of information, tasks, tools and technologies, and people).
We conducted 20 observations of breast cancer clinicians (total: 79 hours). We identified 10 themes related to workflow that present challenges and opportunities for decision support design. We identified approximately 48 different decisions discussed during breast cancer visits. These decisions were often interdependent and involved collaboration across the large cancer treatment team. Numerous patient-specific factors (eg, work, hobbies, family situation) were discussed when making treatment decisions as well as complex risk and clinical information. Patients were frequently asked to remember and relay information across the large cancer team.
Based on these findings, we proposed design guidelines for informatics tools to support the complex workflows involved in breast cancer care. These guidelines should inform the design of informatics solutions to better support breast cancer decision-making and improve patient-centered cancer care.
Lay Summary
The goal of this study was to understand breast cancer care team workflows (ie, the flow of information, tasks, tools and technologies, and people) to inform the design of decision support tools that better address the needs of patients, their family caregivers, and clinicians facing complex decisions after diagnosis. We observed 20 diverse breast cancer clinicians performing routine care at a large breast center over 79 hours and 118 patient visits. We identified 10 workflow-related themes that presented challenges and opportunities for decision support design. From these themes, we proposed relevant design guidelines to address these challenges. We found that patients with breast cancer managed a large amount of complex information from different sources during a time of high stress and under time pressure. Patients faced numerous decisions of varying difficulty along their cancer journey, many of which were interdependent. Patients’ values, preferences, and personal factors also played an important role. Existing decision support tools do not adequately support this complex decision-making process. Informaticians can apply this deeper knowledge of breast cancer care workflows to enhance decision support tools that advance patient-centered care.
Background and significance
Approximately 310 000 women in the United States are diagnosed with invasive breast cancer each year, while 1 in 8 women will be diagnosed in their lifetime.1 Following breast cancer diagnosis, treatment decision-making can be challenging as there are often multiple clinically appropriate treatment options with differing benefits, risks, and impacts on patient short- and long-term quality of life.2 For instance, one common decision is which surgery to undergo, either a lumpectomy, that removes part of the breast, or a mastectomy that removes the whole breast. Both options have equivalent survival outcomes as long as lumpectomy is followed by radiation therapy.3 Patients can struggle to make these treatment decisions, as what they consider “optimal” treatment depends on their individual values and preferences as well as their understanding of each option’s clinical evidence. For instance, in the example above, one patient may value keeping her breast tissue and choose a lumpectomy while another patient may want to avoid daily radiation therapy treatments and therefore choose a mastectomy. Patients often make these decisions under time pressure and during a period of high emotional burden (eg, stress and fear), which can hinder decision-making and the ability to remember information.4,5 Not surprisingly, studies have found that few patients make informed decisions6,7 and less than 50% of patients believe their treatment plan aligns with their values and preferences.8,9
Shared decision-making, a process that involves joint deliberation and collaboration between patients and clinicians, is the optimal approach to ensure complex cancer decisions are patient-centered10 and has been shown to improve care quality and patient satisfaction.11–13 Decision support tools (ie, decision aids) can support shared decision-making by clarifying the available options and their respective benefits and harms and eliciting patient values and preferences.14,15 Numerous decision aids have been developed to support shared decision-making in breast cancer.16–18 For instance, decision aids have been developed to support the surgical decision described above by providing a side-by-side comparison of lumpectomy with radiation versus mastectomy and the differences between each option.19 Decision aids have been found to improve patient knowledge and satisfaction with care decisions and reduce unnecessary surgical treatment and decisional conflict.18,20 However, widespread implementation remains limited, partly due to challenges integrating these tools in clinical workflows.21–23
Given these persistent issues, we need to better understand the complex workflows involved in treatment decision-making so that we can design tools that integrate in the process. Previous studies have explored various aspects of breast cancer workflows.24 Clinical team interactions have been studied using electronic health record (EHR) messaging data25,26 and observation of multi-disciplinary team meetings.27,28 Several studies have explored patient and clinician information exchange during breast cancer appointments29,30 and during the management of symptoms from chemotherapy,31 as well as how breast cancer patients share information with each other.32 Grub and McMullen33 conducted interviews and observations to understand how medical information and patient values and goals were shared during breast cancer surgical consultations. They found that clinicians prioritized sharing medical information, and that patients needed to process large quantities of information in a short timeframe for their values and goals to be incorporated in decision-making.
It is still unclear how breast cancer workflows support or hinder decision aid use. Workflow is defined as “the flow of people, equipment (including machines and tools), information, and tasks, in different places, at different levels, at different timescales continuously and discontinuously, that are used or required to support the goals of the work domain.”34 This is grounded in the Systems Engineering Initiative for Patient Safety (SEIPS) model,35,36 in which the work system influences the processes of care (or workflows), which in turn lead to system outcomes (eg, quality of decisions, patient safety, burnout). Observation of clinical workflow is a common method used in the design of information technologies37 and can provide “a better understanding of the functions and features a specific health IT application must have in order to optimize and support these workflows” (p. 514).34 In this study, we conducted observations of clinicians to gain an in-depth understanding of the workflow involved in breast cancer treatment decision-making; we used this information to identify design requirements for informatics tools for breast cancer decision-making.
Methods
Setting
We conducted observations of clinicians at one Breast Center from February to August 2022. The Breast Center provides comprehensive services for breast health and is part of a National Cancer Institute-designated Comprehensive Cancer Center at an academic medical center in Tennessee. This research is part of a larger project aimed at designing a COMputeried PAtient-centered Collaborative Technology (ie, COMPACT) to support personalized decision-making for newly diagnosed breast cancer patients (https://digital.ahrq.gov/ahrq-funded-projects/computerized-patient-centered-collaborative-technology-compact-support). This study was approved by the Vanderbilt University Institutional Review Board (IRB #212373).
Data collection
We purposively sampled clinicians to ensure a diverse representation of roles, which helped to understand the full range of clinical workflows. Table 1 details the clinician roles observed with a description of each role. To identify study participants, the medical director of the Breast Center emailed all clinicians to inform them of the study and its goals. The study PI subsequently emailed the clinicians to solicit participation in the study and provided the study information sheet. For clinicians interested in participating, the PI arranged a date and time to meet and observe the clinician during their shift.
Clinician role, description, and corresponding frequency and duration of observations.
Role . | Description . | Number of observations . | Duration (h) . | Patient encounters . |
---|---|---|---|---|
Surgical oncologist | Performs surgery to remove cancer in the breast and lymph nodes | 5 | 22.6 | 39 |
Medical oncologist | Provides systemic therapies to treat cancer, such as chemotherapy, endocrine therapy, and immunotherapy | 5 | 23.9 | 36 |
Radiation oncologist | Provides radiation therapy to treat cancer | 2 | 7.5 | 10 |
Plastic surgeon | Performs surgery to reconstruct the breast after removing the tumor | 1 | 4.2 | 6 |
Nurse practitioner | Collaborates with surgical and medical oncologists to provide care relating to cancer surveillance and treatment | 2 | 5.6 | 10 |
Registered nurse | Provides information and hands on care during cancer treatment | 2 | 8.5 | 7 |
Radiologist | Reviews and interprets imaging tests, such as mammogram, MRI, and ultrasound | 1 | 3.5 | 6 |
Genetic counselor | Provides counseling on genetic testing options to identify germline mutations | 1 | 1.5 | 2 |
Rehabilitation physiatrist | Diagnoses and manages impairments of cancer and cancer treatment to improve patient function and quality of life | 1 | 2 | 2 |
Total | 20 | 79.3 | 118 |
Role . | Description . | Number of observations . | Duration (h) . | Patient encounters . |
---|---|---|---|---|
Surgical oncologist | Performs surgery to remove cancer in the breast and lymph nodes | 5 | 22.6 | 39 |
Medical oncologist | Provides systemic therapies to treat cancer, such as chemotherapy, endocrine therapy, and immunotherapy | 5 | 23.9 | 36 |
Radiation oncologist | Provides radiation therapy to treat cancer | 2 | 7.5 | 10 |
Plastic surgeon | Performs surgery to reconstruct the breast after removing the tumor | 1 | 4.2 | 6 |
Nurse practitioner | Collaborates with surgical and medical oncologists to provide care relating to cancer surveillance and treatment | 2 | 5.6 | 10 |
Registered nurse | Provides information and hands on care during cancer treatment | 2 | 8.5 | 7 |
Radiologist | Reviews and interprets imaging tests, such as mammogram, MRI, and ultrasound | 1 | 3.5 | 6 |
Genetic counselor | Provides counseling on genetic testing options to identify germline mutations | 1 | 1.5 | 2 |
Rehabilitation physiatrist | Diagnoses and manages impairments of cancer and cancer treatment to improve patient function and quality of life | 1 | 2 | 2 |
Total | 20 | 79.3 | 118 |
Clinician role, description, and corresponding frequency and duration of observations.
Role . | Description . | Number of observations . | Duration (h) . | Patient encounters . |
---|---|---|---|---|
Surgical oncologist | Performs surgery to remove cancer in the breast and lymph nodes | 5 | 22.6 | 39 |
Medical oncologist | Provides systemic therapies to treat cancer, such as chemotherapy, endocrine therapy, and immunotherapy | 5 | 23.9 | 36 |
Radiation oncologist | Provides radiation therapy to treat cancer | 2 | 7.5 | 10 |
Plastic surgeon | Performs surgery to reconstruct the breast after removing the tumor | 1 | 4.2 | 6 |
Nurse practitioner | Collaborates with surgical and medical oncologists to provide care relating to cancer surveillance and treatment | 2 | 5.6 | 10 |
Registered nurse | Provides information and hands on care during cancer treatment | 2 | 8.5 | 7 |
Radiologist | Reviews and interprets imaging tests, such as mammogram, MRI, and ultrasound | 1 | 3.5 | 6 |
Genetic counselor | Provides counseling on genetic testing options to identify germline mutations | 1 | 1.5 | 2 |
Rehabilitation physiatrist | Diagnoses and manages impairments of cancer and cancer treatment to improve patient function and quality of life | 1 | 2 | 2 |
Total | 20 | 79.3 | 118 |
Role . | Description . | Number of observations . | Duration (h) . | Patient encounters . |
---|---|---|---|---|
Surgical oncologist | Performs surgery to remove cancer in the breast and lymph nodes | 5 | 22.6 | 39 |
Medical oncologist | Provides systemic therapies to treat cancer, such as chemotherapy, endocrine therapy, and immunotherapy | 5 | 23.9 | 36 |
Radiation oncologist | Provides radiation therapy to treat cancer | 2 | 7.5 | 10 |
Plastic surgeon | Performs surgery to reconstruct the breast after removing the tumor | 1 | 4.2 | 6 |
Nurse practitioner | Collaborates with surgical and medical oncologists to provide care relating to cancer surveillance and treatment | 2 | 5.6 | 10 |
Registered nurse | Provides information and hands on care during cancer treatment | 2 | 8.5 | 7 |
Radiologist | Reviews and interprets imaging tests, such as mammogram, MRI, and ultrasound | 1 | 3.5 | 6 |
Genetic counselor | Provides counseling on genetic testing options to identify germline mutations | 1 | 1.5 | 2 |
Rehabilitation physiatrist | Diagnoses and manages impairments of cancer and cancer treatment to improve patient function and quality of life | 1 | 2 | 2 |
Total | 20 | 79.3 | 118 |
At the start of each session, the researcher explained the goal of the observation, gave clinicians the study information sheet, answered questions about the study, and obtained consent. The researcher then observed the clinician as they interacted with patients and conducted other routine work activities (eg, placing orders), asking follow-up questions for clarification when possible. The observation form was based on the work system model38–41 and included free-text fields to capture the people, tasks, tools and technologies, and physical environment observed over time (ie, workflow).34 We piloted the observation form during one clinician observation. Based on this pilot test, we made slight modifications to the organization of the form to streamline data collection.
One human factors engineer conducted each observation, using a tablet computer with smart pen to take notes. Throughout the observation, we recorded all roles that clinicians interacted with (eg, patients, schedulers, other clinicians) and the information exchanged during the interactions. For instance, when a clinician was in an exam room with a patient, we wrote down the information the clinician told to the patient as well as any questions or comments that the patient and their family member(s) had for the clinician. We also included notes about individual activities that clinicians performed such as reviewing patient charts and responding to messages and any tools or technologies used to complete these activities. Patients and their family caregivers (if applicable) provided consent to be observed upon the clinician entering the exam room. Table 2 depicts an example of the observation form and data collected. The blank observation form is in Appendix S1.
Time . | Location . | Tasks and roles . | Tools/technologies . | Barriers . |
---|---|---|---|---|
2:43 | Radiology reading room | Radiologist and surgeon discuss an MRI for a patient. Surgeon gives the radiologist the MRN for another patient and they pull up her images. They discuss placement of a Magseed. |
| |
2:48 | Surgical oncology workspace | Surgeon stops to get nurse and goes to see patient. | ||
2:49 | Surgical oncology exam room | Surgeon asks how the patient is doing. The patient said her mom died last week. She has no family history of breast cancer and is healthy. Surgical oncologist logs into the EHR and shows the patient the screen. They review her imaging together. The surgeon points to the printed-out pathology report from the patient’s biopsy. Explains the patient is ER/PR positive and HER2 negative, low grade, low rate, and tumor is very small. This is very treatable. [Surgeon writes on the back of the pathology report]. She explains the 3 types of treatment. Surgery, radiation which will be after surgery, and medical. The medical oncologist will talk to you about this. For surgery, you can do lumpectomy plus radiation or mastectomy. These have the same chance of survival. With both, we will also do a sentinel lymph node biopsy. This takes out the lymph nodes and sends them to the lab… (conversation continued) |
|
Time . | Location . | Tasks and roles . | Tools/technologies . | Barriers . |
---|---|---|---|---|
2:43 | Radiology reading room | Radiologist and surgeon discuss an MRI for a patient. Surgeon gives the radiologist the MRN for another patient and they pull up her images. They discuss placement of a Magseed. |
| |
2:48 | Surgical oncology workspace | Surgeon stops to get nurse and goes to see patient. | ||
2:49 | Surgical oncology exam room | Surgeon asks how the patient is doing. The patient said her mom died last week. She has no family history of breast cancer and is healthy. Surgical oncologist logs into the EHR and shows the patient the screen. They review her imaging together. The surgeon points to the printed-out pathology report from the patient’s biopsy. Explains the patient is ER/PR positive and HER2 negative, low grade, low rate, and tumor is very small. This is very treatable. [Surgeon writes on the back of the pathology report]. She explains the 3 types of treatment. Surgery, radiation which will be after surgery, and medical. The medical oncologist will talk to you about this. For surgery, you can do lumpectomy plus radiation or mastectomy. These have the same chance of survival. With both, we will also do a sentinel lymph node biopsy. This takes out the lymph nodes and sends them to the lab… (conversation continued) |
|
Abbreviations: ER = estrogen receptor; HER2 = human epidermal growth factor receptor 2; MRN = medical record number; PR = progesterone receptor.
Time . | Location . | Tasks and roles . | Tools/technologies . | Barriers . |
---|---|---|---|---|
2:43 | Radiology reading room | Radiologist and surgeon discuss an MRI for a patient. Surgeon gives the radiologist the MRN for another patient and they pull up her images. They discuss placement of a Magseed. |
| |
2:48 | Surgical oncology workspace | Surgeon stops to get nurse and goes to see patient. | ||
2:49 | Surgical oncology exam room | Surgeon asks how the patient is doing. The patient said her mom died last week. She has no family history of breast cancer and is healthy. Surgical oncologist logs into the EHR and shows the patient the screen. They review her imaging together. The surgeon points to the printed-out pathology report from the patient’s biopsy. Explains the patient is ER/PR positive and HER2 negative, low grade, low rate, and tumor is very small. This is very treatable. [Surgeon writes on the back of the pathology report]. She explains the 3 types of treatment. Surgery, radiation which will be after surgery, and medical. The medical oncologist will talk to you about this. For surgery, you can do lumpectomy plus radiation or mastectomy. These have the same chance of survival. With both, we will also do a sentinel lymph node biopsy. This takes out the lymph nodes and sends them to the lab… (conversation continued) |
|
Time . | Location . | Tasks and roles . | Tools/technologies . | Barriers . |
---|---|---|---|---|
2:43 | Radiology reading room | Radiologist and surgeon discuss an MRI for a patient. Surgeon gives the radiologist the MRN for another patient and they pull up her images. They discuss placement of a Magseed. |
| |
2:48 | Surgical oncology workspace | Surgeon stops to get nurse and goes to see patient. | ||
2:49 | Surgical oncology exam room | Surgeon asks how the patient is doing. The patient said her mom died last week. She has no family history of breast cancer and is healthy. Surgical oncologist logs into the EHR and shows the patient the screen. They review her imaging together. The surgeon points to the printed-out pathology report from the patient’s biopsy. Explains the patient is ER/PR positive and HER2 negative, low grade, low rate, and tumor is very small. This is very treatable. [Surgeon writes on the back of the pathology report]. She explains the 3 types of treatment. Surgery, radiation which will be after surgery, and medical. The medical oncologist will talk to you about this. For surgery, you can do lumpectomy plus radiation or mastectomy. These have the same chance of survival. With both, we will also do a sentinel lymph node biopsy. This takes out the lymph nodes and sends them to the lab… (conversation continued) |
|
Abbreviations: ER = estrogen receptor; HER2 = human epidermal growth factor receptor 2; MRN = medical record number; PR = progesterone receptor.
Observation notes were saved on a password-protected computer. One researcher transcribed all observation notes in a Microsoft Excel spreadsheet with each tab representing one observation. We uploaded the transcribed notes from each observation into Dedoose, a qualitative data analysis software.
Data analysis
Two human factors researchers, one of whom is also a nurse informaticist, coded the observations in an inductive process.42 To do this, we each independently coded one transcript and then met to discuss the coding, clarifying the codes and their definitions. We continued coding the transcripts, meeting to discuss and resolve discrepancies each week, and adding codes as new concepts arose in the data. Once we coded all observations, we met to review our codes and identify key themes emerging in the data relevant to the design and implementation of decision support tools. We grouped the themes into the 4 aspects of workflow described by Carayon et al.,34 namely, the flow of information, tasks, tools and technologies, and people. Finally, we reviewed the themes and identified design guidelines for decision support tools.
Results
Clinician observations and visit types
We observed 79 h of clinical care and 118 patient encounters across 20 observations of various clinical roles (see Table 1). Seventeen out of the 20 clinicians were female: 15 clinicians were White, 2 were Black, 3 were Asian, and 1 clinician was of Hispanic, Latino, and/or Spanish origin.
Table 3 shows the phases of treatment observed according to the visit type. We found that treatment decision-making occurred across almost every visit types. We observed 33 new patient visits, which are visits when a patient is meeting a clinician for the first time. New patient visits with the surgical oncologist, medical oncologist, radiation oncologist, and plastic surgeon typically focused on reviewing the patient’s diagnosis and making a treatment plan. We observed 75 return visits, which can serve a variety of goals; often these visits are focused on surveillance of patients currently in treatment to monitor side effects and symptoms (eg, during chemotherapy) and can also be visits for routine screening (eg, mammogram, breast exam) after diagnosis. Post-operative check-ins with the surgical team are another type of return visit that occur approximately 2 weeks after surgery. We observed 4 instances in which a return visit was scheduled to re-discuss treatment options in cases when a decision could not be made in the first visit. We observed 2 visit types for radiologists: a visit for taking a biopsy in the breast and a visit for performing an ultrasound. For genetic counselors, new patient visits focused on the decision to get genetic testing. Return visits focused on reviewing genetic testing findings.
Role . | Type of visit . | Number of observations . |
---|---|---|
Surgical oncologist | New patient visit | 9 |
Return visit | 26 | |
Surgery planning | 3 | |
Post-operative check-in | 4 | |
Surveillance | 19 | |
Visit for non-cancer breast surgery (eg, atypical hyperplasia) | 4 | |
Medical oncologist | New patient visit | 8 |
Return visit | 28 | |
Radiation oncologist | New patient visit | 9 |
Return visit | 1 | |
Plastic surgeon | New patient visit | 4 |
Return visit | 2 | |
Nurse practitioner | Return visit | 10 |
High-risk patient surveillance (not yet diagnosed with cancer) | 3 | |
Surveillance | 7 | |
Registered nurse | New patient visit | 1 |
Return visit | 6 | |
Surgery planning | 1 | |
Surveillance | 5 | |
Radiologist | Biopsy | 1 |
Ultrasound | 5 | |
Genetic counselor | New patient visit | 1 |
Return visit | 1 | |
Rehabilitation physiatrist | New patient visit | 1 |
Return visit | 1 |
Role . | Type of visit . | Number of observations . |
---|---|---|
Surgical oncologist | New patient visit | 9 |
Return visit | 26 | |
Surgery planning | 3 | |
Post-operative check-in | 4 | |
Surveillance | 19 | |
Visit for non-cancer breast surgery (eg, atypical hyperplasia) | 4 | |
Medical oncologist | New patient visit | 8 |
Return visit | 28 | |
Radiation oncologist | New patient visit | 9 |
Return visit | 1 | |
Plastic surgeon | New patient visit | 4 |
Return visit | 2 | |
Nurse practitioner | Return visit | 10 |
High-risk patient surveillance (not yet diagnosed with cancer) | 3 | |
Surveillance | 7 | |
Registered nurse | New patient visit | 1 |
Return visit | 6 | |
Surgery planning | 1 | |
Surveillance | 5 | |
Radiologist | Biopsy | 1 |
Ultrasound | 5 | |
Genetic counselor | New patient visit | 1 |
Return visit | 1 | |
Rehabilitation physiatrist | New patient visit | 1 |
Return visit | 1 |
Role . | Type of visit . | Number of observations . |
---|---|---|
Surgical oncologist | New patient visit | 9 |
Return visit | 26 | |
Surgery planning | 3 | |
Post-operative check-in | 4 | |
Surveillance | 19 | |
Visit for non-cancer breast surgery (eg, atypical hyperplasia) | 4 | |
Medical oncologist | New patient visit | 8 |
Return visit | 28 | |
Radiation oncologist | New patient visit | 9 |
Return visit | 1 | |
Plastic surgeon | New patient visit | 4 |
Return visit | 2 | |
Nurse practitioner | Return visit | 10 |
High-risk patient surveillance (not yet diagnosed with cancer) | 3 | |
Surveillance | 7 | |
Registered nurse | New patient visit | 1 |
Return visit | 6 | |
Surgery planning | 1 | |
Surveillance | 5 | |
Radiologist | Biopsy | 1 |
Ultrasound | 5 | |
Genetic counselor | New patient visit | 1 |
Return visit | 1 | |
Rehabilitation physiatrist | New patient visit | 1 |
Return visit | 1 |
Role . | Type of visit . | Number of observations . |
---|---|---|
Surgical oncologist | New patient visit | 9 |
Return visit | 26 | |
Surgery planning | 3 | |
Post-operative check-in | 4 | |
Surveillance | 19 | |
Visit for non-cancer breast surgery (eg, atypical hyperplasia) | 4 | |
Medical oncologist | New patient visit | 8 |
Return visit | 28 | |
Radiation oncologist | New patient visit | 9 |
Return visit | 1 | |
Plastic surgeon | New patient visit | 4 |
Return visit | 2 | |
Nurse practitioner | Return visit | 10 |
High-risk patient surveillance (not yet diagnosed with cancer) | 3 | |
Surveillance | 7 | |
Registered nurse | New patient visit | 1 |
Return visit | 6 | |
Surgery planning | 1 | |
Surveillance | 5 | |
Radiologist | Biopsy | 1 |
Ultrasound | 5 | |
Genetic counselor | New patient visit | 1 |
Return visit | 1 | |
Rehabilitation physiatrist | New patient visit | 1 |
Return visit | 1 |
Workflow themes
We identified 10 key themes related to breast cancer team workflow that presented challenges to decision support design (see Table 4). Figure 1 depicts the 4 components of workflow and the corresponding themes.
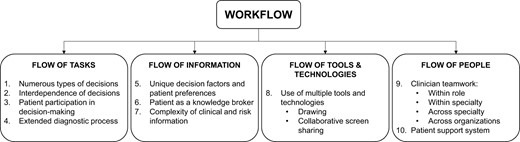
Workflow components, themes, and associated challenges to decision support design.
Workflow . | Theme . | Challenges to decision support design . |
---|---|---|
It is challenging to… . | ||
Flow of tasks | 1. Numerous types of decisions |
|
2. Interdependence of decisions |
| |
3. Patient participation in decision-making |
| |
4. Extended diagnostic process |
| |
Flow of information | 5. Unique decision factors and patient preferences |
|
6. Patient as a knowledge broker |
| |
7. Complexity of clinical and risk information |
| |
Flow of tools and technologies | 8. Use of multiple tools and technologies |
|
Flow of people | 9. Clinician teamwork |
|
10. Patient support system |
|
Workflow . | Theme . | Challenges to decision support design . |
---|---|---|
It is challenging to… . | ||
Flow of tasks | 1. Numerous types of decisions |
|
2. Interdependence of decisions |
| |
3. Patient participation in decision-making |
| |
4. Extended diagnostic process |
| |
Flow of information | 5. Unique decision factors and patient preferences |
|
6. Patient as a knowledge broker |
| |
7. Complexity of clinical and risk information |
| |
Flow of tools and technologies | 8. Use of multiple tools and technologies |
|
Flow of people | 9. Clinician teamwork |
|
10. Patient support system |
|
Workflow components, themes, and associated challenges to decision support design.
Workflow . | Theme . | Challenges to decision support design . |
---|---|---|
It is challenging to… . | ||
Flow of tasks | 1. Numerous types of decisions |
|
2. Interdependence of decisions |
| |
3. Patient participation in decision-making |
| |
4. Extended diagnostic process |
| |
Flow of information | 5. Unique decision factors and patient preferences |
|
6. Patient as a knowledge broker |
| |
7. Complexity of clinical and risk information |
| |
Flow of tools and technologies | 8. Use of multiple tools and technologies |
|
Flow of people | 9. Clinician teamwork |
|
10. Patient support system |
|
Workflow . | Theme . | Challenges to decision support design . |
---|---|---|
It is challenging to… . | ||
Flow of tasks | 1. Numerous types of decisions |
|
2. Interdependence of decisions |
| |
3. Patient participation in decision-making |
| |
4. Extended diagnostic process |
| |
Flow of information | 5. Unique decision factors and patient preferences |
|
6. Patient as a knowledge broker |
| |
7. Complexity of clinical and risk information |
| |
Flow of tools and technologies | 8. Use of multiple tools and technologies |
|
Flow of people | 9. Clinician teamwork |
|
10. Patient support system |
|
Flow of tasks
Numerous types of decisions
We identified approximately 48 types of decisions discussed with patients across their breast cancer journey (full list in Appendix S2). These decisions ranged from major treatment decisions (eg, receiving chemotherapy) to more “minor” disease management decisions (eg, wearing a cold cap during chemotherapy to prevent hair loss). Common decisions included what type of surgery to receive (eg, lumpectomy or mastectomy), what type of reconstruction to get (eg, implant-based or DIEP flap), changing medications due to side effects, and testing for genetic mutations. We also found variability in the types of decisions each patient needed to make. For instance, one patient needed to decide whether to proactively get a chemotherapy port placed during surgery or wait for the surgical results to decide. If she opted to place the port during surgery and then did not need chemotherapy, she would have a scar from the unnecessary port. However, if she did not get the port placed during surgery and ended up needing chemotherapy, she would need to undergo another procedure to place the port at a later date. This decision was specific to this patient’s case and was not experienced by other patients.
Interdependence of decisions
In addition to the multitude of decisions made following breast cancer diagnosis, we found decisions were interdependent and evolved over time. This resulted in patients making treatment decisions with incomplete information on the downstream implications of that decision. Figure 2 depicts an example of the interdependent nature of breast cancer treatment decisions. In this case, a patient with stage 2A, triple negative breast cancer was deciding between a lumpectomy or a mastectomy; the surgical decision would influence the patient’s future need for radiation and potentially reconstruction. However, when the patient was making the surgical decision, she had not yet discussed radiation therapy with a radiation oncologist (eg, to discuss duration of treatment and side effects) or reconstruction options with a plastic surgeon. We found that patients often brought up questions about other treatment modalities when making decisions. For example, in Figure 2, the patient asked the surgical oncologist about the frequency and duration of her chemotherapy regimen, which is managed by her medical oncologist.
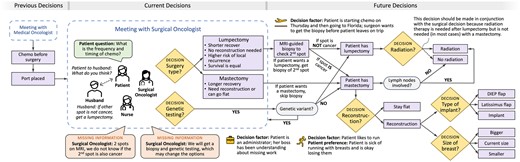
Example decision-making process for a 50-year-old female patient with stage 2A, triple negative breast cancer. Following diagnosis, the patient met with a medical oncologist, agreed to receive neoadjuvant chemotherapy before surgery (the standard of care in this case), and had a port placed. The patient and her husband then met with a surgical oncologist to discuss surgical treatment options, either a lumpectomy or a mastectomy. The surgeon explained that these 2 options had equivalent survival outcomes, but the lumpectomy had a higher risk of local recurrence. The lumpectomy would have a quicker recovery and would not require reconstruction. In this case, the patient had 2 suspicious spots on her MRI, one of which was already confirmed as triple negative cancer. The team did not know whether the second suspicious spot was cancerous or benign. If the patient preferred a lumpectomy, she would need to get a second biopsy to verify if the second suspicious spot was cancerous. If it was not cancer, the patient could continue with a lumpectomy and radiation. If the second spot was cancerous, the patient would instead need a mastectomy, followed by reconstruction (if desired). Following discussion with her husband, the patient decided to proceed with the lumpectomy pathway. The patient was traveling later in the week after starting her first chemo treatment in 2 days. The team needed to coordinate how to get the patient’s MRI-guided biopsy before she started chemo and left for Florida. The patient also needed to get genetic testing, the results of which may subsequently influence her treatment choice, especially the surgical approach.
Patient participation in decision-making
We found varying levels of patient participation in decision-making, spanning the shared decision-making continuum.43 In some cases, the physician would share information on the treatment options, risks, and benefits and then would make a clear recommendation on what option to choose (ie, “informed non-dissent”). In other cases (53 instances coded), more like “patient-driven decision-making,” the patient was left to decide between 2 (or more) feasible options presented by the physician (example in Figure 2). Patients and their family caregivers frequently participated in decision-making by asking questions, most commonly about the treatment timeline and next steps. Two patients were seeking second opinions, representing one component of the larger decision-making process. We identified 13 instances of decisional conflicts in which there were disagreements on the treatment plan. For instance, a patient and her aunt disagreed about whether the patient should receive chemotherapy or not.
Extended diagnostic process
We found that breast cancer diagnosis was an extended process often involving numerous steps and procedures (eg, MRI, ultrasound, additional biopsies, genetic testing) over days and weeks following the patient’s initial diagnosis. For example, following initial diagnosis of invasive ductal carcinoma, a patient may undergo an MRI to check for additional suspicious spots in the breast and lymph nodes. Because of this extended diagnostic process, relevant information (eg, lymph node involvement) was often missing when patients discussed treatment options with physicians, which at times increased uncertainty in the decision-making process. For instance, in Figure 2, the patient was discussing treatment options with a surgical oncologist, but she required additional diagnostic work-up to inform the decision. Specifically, the team did not know whether a second suspicious spot on the patient’s MRI was cancerous or if the patient had any genetic mutations (eg, BRCA1), a finding which would significantly affect the surgical approach. This missing diagnostic information was necessary to make a final surgical decision.
Flow of information
Unique decision factors and patient preferences
We identified numerous patient-specific factors (100 instances) that influenced the treatment decision or the execution of that decision. These “decision factors” went beyond disease-level characteristics, such as cancer sub-type (eg, triple negative), and instead, covered the broad spectrum of patients’ lives that had practical implications for the decision-making process. One common decision factor was the patient’s work; for instance, one patient was self-employed and wanted to wait to have surgery until her business lease was up at the end of the month. Another patient was a CEO and wanted to make sure she could continue working during treatment. Upcoming life events were another common decision factor. A patient’s son was graduating from high school, and she wanted to understand how her treatment would affect this upcoming event. Another patient wanted to ensure her treatment decision (eg, mastectomy) would not limit her ability to hold her new grandchild. Patient hobbies also played a role in decision-making. For instance, one patient golfed several times a week and wanted to select the reconstruction option that would have the least impact on her golf performance. Patients also wanted to know how their treatment would affect their other care needs (eg, planned cataract surgery). We found many instances (64) in which the patient explicitly communicated their values and preferences during the decision-making process. For instance, patients expressed their preference for one treatment over another (eg, mastectomy vs lumpectomy) and to not receive certain treatments (eg, chemotherapy). Appendix S3 includes a full list of observed decision factors.
Patient as a knowledge broker
We found that patients played a critical role in information sharing between members of the large cancer team, filling multiple knowledge broker roles44 including retrieving, transferring, and reminding care team members of information (74 instances coded). Clinicians routinely asked patients to share information that other care providers had told the patient. Patients were also asked to relay information from one clinician to another. For instance, a surgical oncologist identified sub-clavicular lymph node involvement, which needed to be radiated along with the patient’s breast and axillary lymph nodes. The patient was getting radiation closer to home and the surgical oncologist told the patient to make sure she tells her local radiation oncologist to target the extra lymph nodes during treatment. Finally, clinicians asked patients to verify whether tasks were completed (eg, genetic testing).
Complex clinical and risk information
We identified a multitude of complex clinical and risk information communicated to patients while making treatment decisions. In one case, we identified 14 complex terms, including drug names (eg, carboplatin, Lupron, Kadcyla, Taxol) and clinical concepts (eg, endocrine, receptors, hormone blocking, toxicity) used during a medical oncology visit. The use of complex clinical terms and medical jargon often led to patient confusion. For instance, a patient had been under the (incorrect) impression that she had metastatic disease when she was previously told the cancer had spread to her lymph nodes. In another case, a surgical oncologist warned a patient that they may see the term “partial mastectomy” as well as “lumpectomy” in her chart; the surgeon explained that these terms mean the same thing. Patients repeatedly struggled to understand their pathology report due to the use of complex medical terms.
Clinicians regularly shared risk information with patients during the decision-making process, most commonly the patient’s risk of recurrence with and without certain treatments as well as comparisons of risk between different treatment options (82 instances coded). For instance, a medical oncologist explained to a patient that their risk of recurrence after surgery with no treatment is 10% while their risk is 5% or “cut in half” if the patient takes hormone therapy after surgery. Clinicians also used risk information to discuss uncertainties (81 instances coded). For instance, a surgical oncologist told a patient there is a 5–15% chance that there is cancer in addition to the identified inter-ductal papilloma. The patient had the choice of surveillance or excising the mass to check for cancer cells. Clinicians also communicated personalized risk information to patients. A surgical oncologist explained to a patient that her risk of another breast cancer was 20%, which is higher than the average risk of 12.5%.
Flow of tools and technologies
Use of multiple tools and technologies
We identified 23 different tools and technologies used by patients and clinicians during treatment decision-making. Technologies included the EHR and patient portal, telephones (clinician and patient smart phones, office phones), radiology systems (to view and discuss patient mammogram and MRI images), ultrasound, SOZO® lymphedema scanner, clinician laptops, and fax machines. Clinicians also used several technologies to gather information on patient tumor and genetic factors: DCISionRT©, Oncotype DX®, MammaPrint, and RNA genetic testing. Clinicians accessed these test results through different websites that were not integrated into the EHR, creating barriers to clinical workflow.
Paper documents and drawing. Clinicians used paper documents to exchange information. A paper pathology report with the patient’s biopsy results was often employed in the treatment decision-making discussion. Clinicians presented the paper pathology report to patients and walked patients through their diagnosis (eg, receptor status) with the paper report as a visual aid. We found that 6 clinicians drew images and text on paper documents to help patients understand treatment options. The radiation oncologist used the whiteboard in patient rooms to visually depict information.
Collaborative screen sharing. We identified 12 instances of clinicians using the computer screen in the exam room to share information with patients and their family members. Most commonly, clinicians used the computer to show patients their imaging tests, such as an ultrasound or bone scan. Clinicians also used the computer to show patients their blood test results, medications, family history of cancer, and historical measurements for lymphedema tracking.
Flow of people
Teamwork
Not surprisingly, almost every task observed in the clinic involved some form of teamwork. We identified 77 instances of teamwork within roles (eg, 2 medical oncologists discussing a patient’s treatment plan), 135 instances of teamwork between different roles within the same specialty (eg, a surgical oncologist and a nurse discussing a patient’s background), and 55 instances of teamwork across specialties (eg, a surgical oncologist and radiologist discussing patient images). We found that medical oncologists and surgical oncologists often collaborated on treatment plans in-person and via the EHR. We also identified instances of intra-organizational teamwork, such as a medical oncologist working together with a patient’s rheumatologist and cases of inter-organizational teamwork, where clinicians worked with other clinicians from outside institutions to manage patient care. For instance, the radiation oncologist collaborated with a patient’s local radiation oncologist so that the patient could get treatment closer to home. We found that the roles and responsibilities of different members of the care team frequently led to confusion. In particular, patients did not understand what part of their treatment each clinician was responsible for (eg, medical oncologist vs surgical oncologist).
Patient support system
Patient family caregivers also played a central role in the decision-making team. Patients frequently relied on family caregivers to take notes, ask questions, and give input on treatment decisions (58 instances coded). For example, as shown in Figure 2, a patient asked her husband what he thought about getting a lumpectomy versus a mastectomy. The husband recommended the patient get a lumpectomy if a second suspicious spot on her MRI was non-cancerous. The patient agreed and decided to get a lumpectomy.
Discussion
In this study, we analyzed and described the complex workflow involved in breast cancer treatment decision-making. While decision support technologies can improve shared decision-making for breast cancer treatment, workflow barriers have hindered widespread use of these tools.17,21 From observations of 20 diverse breast cancer clinicians, we identified 10 themes related to workflow that have implications for the design of decision support tools in breast cancer care. Overall, we found that patients with breast cancer must manage highly complex information from multiple specialties and different care team members that includes technical medical language, mathematical concepts (eg, prognosis and risk), and uncertainty. A multitude of decisions are made following breast cancer diagnosis, and these decisions are often interdependent, involve multiple clinical specialties, and evolve over a prolonged period during treatment. Furthermore, patients must manage these difficult decisions under time pressure and at a time of emotional crisis.4 Existing decision support tools do not adequately support this complex process.
Based on these findings, we propose design guidelines (see Table 5) for informatics tools to better support patients (and clinicians) as they manage breast cancer care. Observation of patient–clinician communication is a valuable method to understand the perspectives of users and derive design considerations for future information systems.45 While breast cancer patient information needs have been widely studied,46 limited understanding of team decision-making and clinical workflows has hindered implementation and use of patient-centered decision support tools.23 Previous studies have investigated workflows and user needs to identify design requirements for health information technology use at chronic disease clinics37 and for the development of an antibiotic clinical decision support system.47 To the best of our knowledge, this is one of the first studies to link a detailed workflow analysis of breast cancer teams to specific design guidelines for informatics tools.48
Workflow . | Theme . | Design guidelines . |
---|---|---|
Systems for breast cancer shared decision-making should… . | ||
Flow of tasks | Numerous types of decisions |
|
Interdependence of decisions |
| |
Patient participation in decision-making |
| |
Extended diagnostic process |
| |
Flow of information | Unique decision factors and patient preferences |
|
Patient as a knowledge broker |
| |
Complexity of clinical and risk information |
| |
Flow of tools and technologies | Use of multiple tools and technologies |
|
Flow of people | Clinician teamwork |
|
Patient support system |
|
Workflow . | Theme . | Design guidelines . |
---|---|---|
Systems for breast cancer shared decision-making should… . | ||
Flow of tasks | Numerous types of decisions |
|
Interdependence of decisions |
| |
Patient participation in decision-making |
| |
Extended diagnostic process |
| |
Flow of information | Unique decision factors and patient preferences |
|
Patient as a knowledge broker |
| |
Complexity of clinical and risk information |
| |
Flow of tools and technologies | Use of multiple tools and technologies |
|
Flow of people | Clinician teamwork |
|
Patient support system |
|
Workflow . | Theme . | Design guidelines . |
---|---|---|
Systems for breast cancer shared decision-making should… . | ||
Flow of tasks | Numerous types of decisions |
|
Interdependence of decisions |
| |
Patient participation in decision-making |
| |
Extended diagnostic process |
| |
Flow of information | Unique decision factors and patient preferences |
|
Patient as a knowledge broker |
| |
Complexity of clinical and risk information |
| |
Flow of tools and technologies | Use of multiple tools and technologies |
|
Flow of people | Clinician teamwork |
|
Patient support system |
|
Workflow . | Theme . | Design guidelines . |
---|---|---|
Systems for breast cancer shared decision-making should… . | ||
Flow of tasks | Numerous types of decisions |
|
Interdependence of decisions |
| |
Patient participation in decision-making |
| |
Extended diagnostic process |
| |
Flow of information | Unique decision factors and patient preferences |
|
Patient as a knowledge broker |
| |
Complexity of clinical and risk information |
| |
Flow of tools and technologies | Use of multiple tools and technologies |
|
Flow of people | Clinician teamwork |
|
Patient support system |
|
This study highlights the complexity of treatment decision-making workflows in terms of the number of decisions that patients make throughout treatment and the interdependence of these decisions. Patients often made treatment decisions that influenced subsequent decisions without the bigger picture of how these decisions were related (eg, choosing lumpectomy implies a later decision of radiation therapy). Decision aids typically focus on one decision or one care specialty (eg, surgical oncology) and do not support consideration of several treatment options at one time.17,18 Furthermore, previously developed decision aids have not accounted for the full scope of decisions patients face during breast cancer care.18 Future research is needed to determine if and how decision support tools could incorporate the broader scope and the interdependence between various decisions to support patient-centered care.
We highlight the need for informatics tools to consider the diverse and unique decision factors that are crucial to patient-centered decision-making. Breast cancer decision-making not only varies depending on tumor characteristics (eg, size, position, receptor status), patient physiology (eg, menopause status), genetics (eg, BRCA carrier), and patient preferences, but also is influenced by the broader context of a patient’s life such as beloved hobbies and important social engagements (eg, a child’s graduation). Prior studies have highlighted how some of these factors influence treatment decision-making. Grub and McMullen33 detailed how a patient brought her violin to a clinic visit to ensure her range of motion and ability to play would be minimally disrupted by her breast cancer treatment. In a systematic review, Lu et al.46 found that a key information need of breast cancer patients was the resumption of normal life, such as if and when patients can return to work. Decision support could help elicit and incorporate these factors during treatment decision-making. However, the variable nature of these patient-specific factors presents a challenge to decision support design. One study developed a calendar where patients and clinicians could view and plan for important life events (eg, a daughter’s wedding) during treatment.49 Further research is needed to determine how to optimally incorporate such factors into decision support tools.50
We found that patients were key knowledge brokers in the care team, responsible for relaying information to and between different members of the clinical team and for monitoring the completion of important tasks (eg, genetic testing). Prior studies have found that patients frequently serve as knowledge brokers,44,51 filling structural holes (eg, missing information) due to fragmented healthcare systems, such as during hospital discharge52 and emergency department visits.41 Given that patients only remember 50% of what clinicians tell them during clinical encounters,30,53 a better system for tracking and relaying information between team members is needed. Furthermore, breast cancer patients already undertake significant “work” during their treatment journey on top of managing a disease that can hinder cognitive and physical capabilities.54–56 Consistent with prior studies,49 we found inadequate system support for clinicians including limited feedback loops to notify clinicians of completed tasks (eg, genetic testing), which resulted in additional cognitive workload for clinicians to remember and track down necessary information. Informatics solutions could eliminate some of this burden on patients (and clinicians) and improve the reliability and safety of information transfer within breast cancer teams.
Informed by the workflow themes and the challenges and design guidelines outlined in Tables 4 and 5, future informatics solutions should better support personalized decision-making in breast cancer. For example, a cancer journey map could be developed that supports team collaboration and treatment planning for each patient. The journey map could visually represent each step in the process and the various decisions patients need to make along the way, with improved representation of the interdependencies between treatment decisions and how each option will integrate in the patient’s broader life. Leveraging the fact that some clinicians regularly create drawings to communicate complex concepts in patient conversations, future decision support systems could incorporate a library of visual templates for easier annotation during discussion as well as allowing the care team to upload paper drawings for patient’s future reference. Such informatics solutions should align with the International Patient Decision Aid Standards (IPDAS) guidelines15,57 and human factors design principles.58 Future research is needed to evaluate the proposed design guidelines and work with end users—clinicians, patients, and their family caregivers—to develop and test potential informatics solutions.
One limitation of this research is that it occurred at one academic medical center in an urban setting. The findings may not represent workflows at other breast centers. We used written observation notes rather than recorded, verbatim text. It is possible that certain aspects of clinic workflow were not recorded. While we observed a wide range of breast cancer roles and visit types, we had a limited sample of some phases of treatment and visit types. We conducted these observations from the perspective of clinicians, and therefore, aspects of the patient visit and experience at the clinic may have been missed. As such, we did not collect information on patient sociodemographic characteristics or health literacy. Future studies should conduct patient-centered observations to better understand the workflows and experiences of patients across their breast cancer journey. While observations are helpful for understanding workflow, they are limited in their ability to capture cognitive processes and the cognitive nature of decision-making. Interviews with patients and clinicians should be conducted to better understand the cognitive processes of treatment decision-making and inform the design of decision support tools.
Conclusion
Breast cancer treatment decision-making is a complex process that involves a large diverse team and numerous preference sensitive decisions that are made in a time pressured and informationally dense environment. Our findings shed light on the unique challenges of breast cancer treatment decision-making as well as the opportunities to better support patient and clinician work. Informatics solutions, aligned with the identified design guidelines can support patient-centered breast cancer care and contribute to improved patient outcomes.
Author contributions
Megan E. Salwei contributed to the research conceptualization, data acquisition, data analysis, data interpretation, initial drafting, and editing of the manuscript. Carrie Reale contributed to the data analysis, data interpretation, and editing of the manuscript.
Supplementary material
Supplementary material is available at JAMIA Open online.
Funding
This work was supported by the Agency for Healthcare Research and Quality (AHRQ) through grant numbers K01HS029042 and K12HS026395. The content is solely the responsibility of the authors and does not necessarily represent the official views of AHRQ.
Conflicts of interest
The authors have no competing interests to declare.
Data availability
The data underlying this article will be shared on reasonable request to the corresponding author.