-
PDF
- Split View
-
Views
-
Cite
Cite
Sripriya Rajamani, Hanna Chakoian, Aaron Bieringer, Anna Lintelmann, Jeffrey Sanders, Rachel Ostadkar, Amy Saupe, Genny Grilli, Katie White, Sarah Solarz, Genevieve B Melton, Development and implementation of an interoperability tool across state public health agency’s disease surveillance and immunization information systems, JAMIA Open, Volume 6, Issue 3, October 2023, ooad055, https://doi.org/10.1093/jamiaopen/ooad055
- Share Icon Share
Abstract
Public health information systems have historically been siloed with limited interoperability. The State of Minnesota’s disease surveillance system (Minnesota Electronic Disease Surveillance System: MEDSS, ∼12 million total reportable events) and immunization information system (Minnesota Immunization Information Connection: MIIC, ∼130 million total immunizations) lacked interoperability between them and data exchange was fully manual. An interoperability tool based on national standards (HL7 and SOAP/web services) for query and response was developed for electronic vaccination data exchange from MIIC into MEDSS by soliciting stakeholder requirements (n = 39) and mapping MIIC vaccine codes (n = 294) to corresponding MEDSS product codes (n = 48). The tool was implemented in March 2022 and incorporates MIIC data into a new vaccination form in MEDSS with mapping of 30 data elements including MIIC demographics, vaccination history, and vaccine forecast. The tool was evaluated using mixed methods (quantitative analysis of user time, clicks, queries; qualitative review with users). Comparison of key tasks demonstrated efficiencies including vaccination data access (before: 50 clicks, >2 min; after: 4 clicks, 8 s) which translated directly to staff effort (before: 5 h/week; after: ∼17 min/week). This case study demonstrates the contribution of improving public health systems interoperability, ultimately with the goal of enhanced data-driven decision-making and public health surveillance.
Lay Summary
Many of the information systems currently used in public health have limited capabilities to exchange data electronically using nationally recommended standards (also called as interoperability). The Minnesota Department of Health, the state public health agency, uses the Minnesota Electronic Disease Surveillance System (MEDSS) to support disease surveillance, and the Minnesota Immunization Information Connection (MIIC) to support immunization practice and monitoring. The exchange of data between these systems was manual and hence a novel tool for standards-based data exchange was implemented in March 2022. The tool was developed by collecting user needs (n = 39) and mapping MIIC vaccine codes (n = 294) to corresponding MEDSS product codes (n = 48). Currently, 30 vaccination data elements including demographics from MIIC, vaccination history, and vaccine forecast are being imported into MEDSS. The tool was evaluated using quantitative and qualitative methods by assessing user time, clicks, and number of queries and by soliciting feedback from users. Comparison of key tasks demonstrated efficiencies in the access of vaccination data. It took 50 clicks and 5 h/week of staff effort before the tool was available and was decreased to 4 clicks and ∼17 min/week after the tool was implemented. This case study demonstrates the value of improving electronic data exchanges in public health with the goal of better decision-making using data and enhanced public health surveillance.
BACKGROUND
Public health infectious outbreaks including the COVID-19 pandemic with >96 million cases and >1 million deaths in the United States (as of October 2022),1 the M-pox2 and measles3 outbreaks and a case of paralytic polio leading to state emergency declaration4 demonstrate the significant human and societal impact of infectious diseases. One critical element in response to these public health emergencies is vaccinations as a means to curb the severity and spread of vaccine-preventable diseases. Particularly for emerging infections or infections where vaccination requirements are enforced as highlighted with COVID-19, data exchange across disease surveillance systems (DSS) and systems that track vaccinations in a population (the immunization information systems [IIS]) is a critical capability. The COVID-19 pandemic also has underscored the importance of data exchange across vital statistics systems (birth, death data) with IIS and DSS. Unfortunately, data exchange capabilities across information systems in public health agencies across the United States remain rudimentary.5–10 As noted by LaVenture et al,10 the Minnesota Department of Health (MDH) has over 31 information systems that support public health functions each with key features based on program needs and public health funding and goals. A number of important factors result from and contribute to the data exchange issues in many of these information systems. These include a lag in timely reporting of data and receipt of data in mostly nonstandardized formats which is compounded by limited interoperability of information systems within public health agencies.10
Current health information technology (HIT) policies and position papers11–14 identify the need for and endorse information systems that are interoperable and support the full breadth of today’s electronic healthcare ecosystem. The pandemic has propelled efforts in public health data and information system modernization led by the Centers for Disease Prevention and Control (CDC) through its Data Modernization Initiative (DMI).15 Despite the increased emphasis on use of health standards with these systems,16 there is a paucity of case studies focused on implementation of standards-based interoperability capabilities in public health information systems. Interoperability in public health is an emerging area, with prior studies focusing mainly on exchanges between electronic health records (EHRs) in clinical care, clinical laboratories, and public health, with emphasis on electronic laboratory reporting (ELRs),17–19 electronic reporting of immunizations20,21 and electronic reporting of mandated infectious diseases22–25 including electronic case reporting.26 These previous interoperability studies involving public health have typically revolved around IIS and EHRs,27–30 highlighting a gap in studies which provide insights on intrapublic health information systems interoperability.
There is an urgent need for better exchange of data between systems for disease surveillance and systems containing vaccination records to create a holistic view, save resources, and improve public health outcomes. The MDH31 hosts the Minnesota Electronic Disease Surveillance System (MEDSS)32 used for disease surveillance and the Minnesota Immunization Information Connection (MIIC)33 which contains vaccination records. This case study presents details on the development, implementation, and evaluation of an interoperability tool for electronic exchange of vaccination data between MEDSS and MIIC managed by MDH, the state public health agency in Minnesota (MN).
METHODS
Systems overview: MIIC and MEDSS
The MIIC system is the state’s IIS, and serves as a central repository for vaccination data for the State of Minnesota.33 MIIC has been operational since 2002 and currently holds data for approximately 130 million immunizations for 8.6 million individuals (as of September 2022). MIIC has a wide user-base with 6029 organizations as registered users ranging from primary care clinics, specialty providers, nursing homes, long term care facilities, retail pharmacies, and hospitals. The role of MIIC has been critical, including during the COVID-19 pandemic with numerous new entities submitting data and completing reports to check vaccination status for their client population. A unique aspect of many IISs including MIIC is their ability to forecast vaccines based on national recommendations or clinical decision support for immunizations (CDSi).34
The MEDSS system is the state’s DSS, and receives case reports of reportable infectious conditions and ELRs for supporting case management, contact tracing, and outbreak investigations. MEDSS was implemented in 2009 and contains 12 399 230 events (as of September 2022).32 This includes positive/suspected reports for any public health reportable condition and both positive and negative reports for COVID-19. The system has a user base across state programs, local public health agencies, and clinical partners with varying access and roles. An estimated 71 995 cases were reported in 2017 and an additional 73 181 cases were reported in 2018.32 With the onset of COVID-19 pandemic, over 26 million tests (both positive and negative results, including multiple tests on individuals) have been reported to MEDSS (September 2022).35
To meet public health surveillance needs specifically for case management and follow-up, it is essential to know the vaccination status of individuals with certain public health reportable conditions and have relevant data available in the DSS. But MEDSS lacked a personalized query functionality to retrieve vaccination data from MIIC for a specific individual or a particular disease. Hence 2 manual methods were used prior to interoperability tool implementation: (1) query individual records in MIIC based on an individual events in MEDSS and manually enter all vaccine data for that individual from MIIC into the MEDSS system; (2) match COVID-19 cases in MEDSS with corresponding records in MIIC using a bulk query process weekly, and then manually upload the matched files in a single batch process. Both processes were laborious and time-consuming for staff, and the bulk queries were prone to occasional record mismatch.
Interoperability tool overview: development, implementation, and evaluation
An interoperability tool between MEDSS and MIIC was designed by informatics staff (n = 3) from MEDSS and MIIC program teams in collaboration with the MEDSS vendor. The development began with eliciting requirements from stakeholders including the MDH infectious disease epidemiologists who are the main users. During requirements gathering, the use of nationally recommended standards by CDC (HL7 query/response for message36,37 and SOAP/synchronous web services call for data transport38) to retrieve immunization data from MIIC to MEDSS were identified as critical aspects of the interoperability tool. The query was designed to be synchronous, in real-time and the criteria for person matching was based on first name, last name, date of birth, and mother’s maiden name, with records >1 match to be presented to the end-user for reconciliation. It was decided by consensus that an existing solution will be adapted and the tool from North Dakota (ND) Department of Health was chosen for customization due to use of the same vendor product as MEDSS. Mapping of MIIC vaccine codes (n = 294) to MEDSS disease codes (n = 48) was completed to align vaccines with relevant diseases. This vaccine mapping was designed to be adaptable and kept updated by the MEDSS program informatics team without vendor dependency. The process also included mapping of needed fields from HL7 response message into a vaccination form (labeled as MIIC Query) in MEDSS. Tool design, vaccine mapping, subsequent testing, and evaluation required numerous (n = 44) vendor and stakeholder discussions over 2 years (2021–2022) with delays due to the COVID-19 pandemic. Informational meetings (n = 5) open to all users were held prior to tool implementation (go-live) to demonstrate the functionalities. A mixed methods approach was used for evaluation of the interoperability tool.
Quantitative methods involved analysis of staff time and clicks for both pre- and postimplementation provided by a lead epidemiologist who tracked the time and clicks for vaccination data access from MIIC. This analysis was conducted on a non-COVID reportable condition and is based on analysis done for pertussis, a reportable infectious disease. The use of the tool was evaluated by review of MIIC data access from MEDSS through SQL queries of the MIIC database to access MIIC log data. Qualitative methods involved input from epidemiologists (n = 9) who lead select infectious disease surveillance programs and are informed users of the interoperability tool. The feedback was collected through semistructured discussions in Microsoft Team meetings (n = 3) with notetaking and transcription done by the lead author (SR) with support of an informatician (HC) from the MEDSS program team. Dedoose v9 (Los Angeles, CA) was used for managing and analyzing the qualitative data and themes were identified by authors (SR, HC) including current tool feedback and needed future enhancements and were reviewed by the epidemiologists involved in the study (n = 9). Approval from the Institutional Review Board (IRB) at the University of Minnesota was obtained.
RESULTS
A total of 39 requirements were identified, ranging from formats to display vaccination history, repeatable fields for multiple doses, certain noneditable data fields (for data quality), and vaccine forecast data from the MIIC CDSi. Supplementary Appendix A presents the final list of interoperability tool requirements (n = 39 across 15 categories). The mapping document aligning the MIIC vaccine codes (n = 294) to corresponding product codes in MEDSS related to vaccine-preventable diseases (n = 48) is shared in Supplementary Appendix B. This mapping includes various vaccine codes (CVX [vaccines administered] code,39 MVX [manufacturers of vaccines] code,40 CPT [Current Procedural Terminology] code41), vaccine description, vaccine trade name, and vaccine status (active/inactive). Supplementary Appendix C displays a HL7 query response along with identification of fields supported by MIIC,36,37 of which 30 data elements were chosen by user consensus for inclusion in the MIIC Query form embedded in MEDSS and is noted in Supplementary Appendix C.
Figure 1 displays the overview of the MEDSS to MIIC query process. MEDSS is a person-centric system where various public health reportable infectious conditions are mapped to an individual. The tool is presented as a vaccination query button to the MEDSS user (end-user) in the main demographic section when an individual record is open. The new tool generates an HL7 standards-based query36 from MEDSS to MIIC which is synchronous and real-time based on the demographic profile of the individual, and returns the individual’s vaccination profile including the vaccination history and vaccine forecasting information (CDSi).
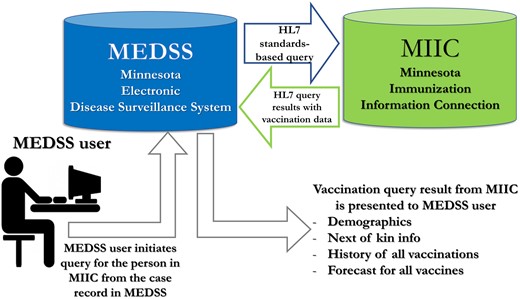
Figure 2 shows a screenshot of the query results that is presented to the end-user. It includes a total of 30 data elements to support decision-making and public health surveillance. The query results start with the demographics as contained in MIIC (client name, MIIC ID, date of birth, gender, address, multiple birth, birth order, mother’s maiden name). The patient demographic segment and next-of-kin data (if available) are also included. This is followed by vaccination history with information on vaccine group, CVX code,39 CPT code,41 date administered, administration organization, administration notes (01 = historical [vaccine given elsewhere]; 00 = vaccine given in site), vaccine level funding eligibility, lot number, and manufacturer. There are also sections for vaccine refusal, and immunity due to history of disease and/or presence of antibodies. Finally, the vaccination forecasting data (also called as CDSi) is presented. The CDSi comprises of vaccine name, dose number in series, recommended date, and earliest date.
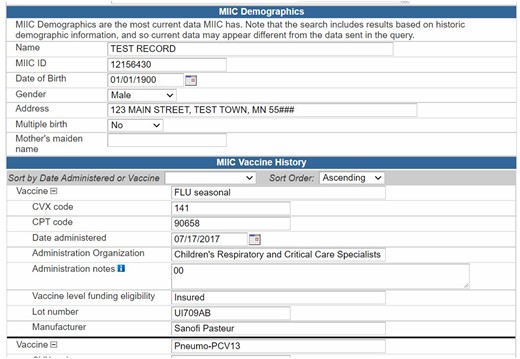
The interoperability tool was implemented in March 2022. Table 1 presents the evaluation of the interoperability tool with assessment of metrics related to time estimates, number of clicks for data retrieval, estimation of data errors, and staff effort with manual processes. Prior to implementing the interoperability tool, it took 50 clicks and 134 s (2 min, 14 s) to access an individual’s vaccination data based on user input. After implementation, only 4 clicks and 8 s were required to access an individual’s vaccination data. During weeks with high volume of MIIC access, the staff time was reduced from 5 h/week to ∼17 min/week.
Metrics . | Preinteroperability tool implementation . | Postinteroperability tool implementation . |
---|---|---|
Time estimate for retrieval of an individual vaccination record | 134 s (2 min, 14 s) | 8 s |
Average number of clicks to complete process of getting vaccination data from MIIC and entering data into MEDSS | 50 clicks | 4 clicks |
Estimate of data entry errors | ∼300 errors to fix annually | Eliminates data entry and imports MIIC data |
Estimated staff time per week of work for non-COVID conditions | 5 h | ∼17 min |
Staff effort for manual process | 1 epidemiologist, 2–3 student workers for every year | No specific staff assigned; data accessed as needed with interoperability tool |
Metrics . | Preinteroperability tool implementation . | Postinteroperability tool implementation . |
---|---|---|
Time estimate for retrieval of an individual vaccination record | 134 s (2 min, 14 s) | 8 s |
Average number of clicks to complete process of getting vaccination data from MIIC and entering data into MEDSS | 50 clicks | 4 clicks |
Estimate of data entry errors | ∼300 errors to fix annually | Eliminates data entry and imports MIIC data |
Estimated staff time per week of work for non-COVID conditions | 5 h | ∼17 min |
Staff effort for manual process | 1 epidemiologist, 2–3 student workers for every year | No specific staff assigned; data accessed as needed with interoperability tool |
Metrics . | Preinteroperability tool implementation . | Postinteroperability tool implementation . |
---|---|---|
Time estimate for retrieval of an individual vaccination record | 134 s (2 min, 14 s) | 8 s |
Average number of clicks to complete process of getting vaccination data from MIIC and entering data into MEDSS | 50 clicks | 4 clicks |
Estimate of data entry errors | ∼300 errors to fix annually | Eliminates data entry and imports MIIC data |
Estimated staff time per week of work for non-COVID conditions | 5 h | ∼17 min |
Staff effort for manual process | 1 epidemiologist, 2–3 student workers for every year | No specific staff assigned; data accessed as needed with interoperability tool |
Metrics . | Preinteroperability tool implementation . | Postinteroperability tool implementation . |
---|---|---|
Time estimate for retrieval of an individual vaccination record | 134 s (2 min, 14 s) | 8 s |
Average number of clicks to complete process of getting vaccination data from MIIC and entering data into MEDSS | 50 clicks | 4 clicks |
Estimate of data entry errors | ∼300 errors to fix annually | Eliminates data entry and imports MIIC data |
Estimated staff time per week of work for non-COVID conditions | 5 h | ∼17 min |
Staff effort for manual process | 1 epidemiologist, 2–3 student workers for every year | No specific staff assigned; data accessed as needed with interoperability tool |
Assessment of tool use conducted by analysis of MEDSS to MIIC query data using logs of MIIC queries since implementation (March 2022–September 2022), pointed to use by epidemiologists with ∼130 queries/week for individual vaccination data access during high use (Figure 3). This implies the number of times an individual vaccination record from MIIC was needed and accessed through the interoperability tool related to public health reportable conditions in MEDSS.
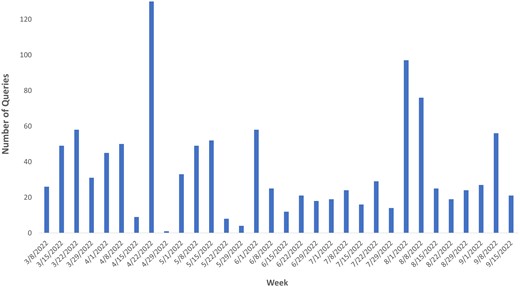
Table 2 presents synthesis of user feedback with input solicited across the various infectious disease program areas and also from the long-term child follow-up program for noninfectious conditions. Representative end-users are satisfied with this interoperability tool with one of the epidemiologist’s evaluations noting “MIIC-MEDSS exchange is the best.” This demonstrates the utility of the electronic exchange of vaccination data and storage of this data in MEDSS to support case management and disease surveillance.
Representative feedback about the interoperability tool from epidemiologists
Themes (n=# of users) . | Sample quotes . |
---|---|
Appreciation of interoperability (n = 9) |
|
Vaccine data availability (n = 6) |
|
Stakeholder requirements Met (n = 6) |
|
Future system enhancement needs (n = 3) |
|
Attend to on-going needs/requirements (n = 4) |
|
Themes (n=# of users) . | Sample quotes . |
---|---|
Appreciation of interoperability (n = 9) |
|
Vaccine data availability (n = 6) |
|
Stakeholder requirements Met (n = 6) |
|
Future system enhancement needs (n = 3) |
|
Attend to on-going needs/requirements (n = 4) |
|
Representative feedback about the interoperability tool from epidemiologists
Themes (n=# of users) . | Sample quotes . |
---|---|
Appreciation of interoperability (n = 9) |
|
Vaccine data availability (n = 6) |
|
Stakeholder requirements Met (n = 6) |
|
Future system enhancement needs (n = 3) |
|
Attend to on-going needs/requirements (n = 4) |
|
Themes (n=# of users) . | Sample quotes . |
---|---|
Appreciation of interoperability (n = 9) |
|
Vaccine data availability (n = 6) |
|
Stakeholder requirements Met (n = 6) |
|
Future system enhancement needs (n = 3) |
|
Attend to on-going needs/requirements (n = 4) |
|
DISCUSSION
Findings and implications
The Building Back Better report42 funded by the Robert Wood Johnson Foundation and a recent U.S. Government Accountability Office (GAO)43 report emphasize the need to transform the US public health system to protect health and achieve health equity. These reports highlight the deficient data infrastructure and identify the lack of interoperability as one of the main challenges. With this interoperability tool between a disease surveillance system (MEDSS) and an immunization information system (MIIC), the efficiencies gained have been demonstrated. The data access burden for an individual vaccination record was decreased to 8 s, and overall effort reduced from 5 h/week to ∼17 min/week with cost savings due to less staff time. It is better to be able to import and update current vaccine data and save it within MEDSS to support case management activities performed by epidemiologists.
With the increasing requirements for electronic data exchanges across public health information systems,44,45 there is a need to implement and assess interoperability. The interoperability tool decreased the number of processes/clicks to retrieve data along with elimination of manual data entry and resultant data errors. The query is generated in MEDSS based on an existing case of a reported infectious disease to public health. The quality of demographics in MEDSS and MIIC and their matching is foundational to data exchange using the interoperability tool. Future tool enhancements will depend on user consensus regarding needs, evaluating the impact on data quality across systems with increasing data exchange driven by interoperability enhancements, and exploring the applicability of the FHIR standard for IIS data access.46,47
Achieving Healthy People 203048,49 which sets national goals for reducing the infectious disease burden requires efficient electronic data exchange between immunization and DSSs in public health. Immunization history and forecasts in MEDSS will facilitate surveillance/follow-up and management of vaccine-preventable diseases. This public health interoperability implementation has facilitated infectious disease surveillance at the state level as MEDSS and MIIC are hosted in the state public health agency, with potential for broader national impact through collaboration.
Strengths and limitations
This data exchange tool was built using national standards36,38 (HL7 standard for query/response, SOAP/web services for data transport, and CVX, MVX, and CPT codes for terminology), a foundation for interoperability. The tool was adapted from an existing solution in ND as MN uses the same disease surveillance software (Maven Disease Surveillance and Outbreak Management System)50 which resulted in savings on development time and costs. The collaborative work with the vendor resulted in meeting MN specific requirements, and designing the interoperability tool such that future enhancements can be done by MEDSS informatics team without being vendor-dependent for all changes. Ability to add new vaccine codes, add/update mapping to MEDSS disease codes and update the mapping of HL7 fields to the MEDSS vaccination form are some of the enhancements that can be completed by the MEDSS informatics team without vendor dependency. Engaging with end-users (epidemiologists) from the start ensured that the project had buy-in and also delivered a product (interoperability tool) which met user expectations. This case study also addresses the paucity of research around HIT in the public health context.
Development and implementation of this interoperability tool required significant informatics workforce expertise and may pose a limitation for some public health departments. Collaboration with other public health agencies and adopting/adapting existing solutions will be helpful. Partnerships with academia and workforce programs funded by the Office of the National Coordinator for HIT (ONC)51,52 will assist in building an informatics-savvy workforce. The tool needed to be iteratively designed and tested with end-users and getting epidemiologists’ time in midst of the COVID pandemic and monkeypox outbreaks was challenging.
The tool is based on HL7 nationally recommended standards by CDC (HL7 query/response for message36,37 and SOAP/synchronous web services call for data transport38). Due to the utilization of national standards, the overall approach in building the tool can be applied to other information systems that respond to queries, generate responses, and are able to receive standardized data synchronously. Hence the lessons learned from developing/deploying this tool are applicable to other public health interoperability projects. These implementation details are intended to support other public health agencies in their strategy to interoperability due to generalizability of tool design, methodology, and findings. This case study demonstrates the potential significant contribution of improving public health systems interoperability, ultimately with the goal of enhanced data-driven decision-making and public health surveillance.
FUNDING
This work was supported by funding from CDC through the Epidemiology and Laboratory Capacity for Prevention and Control of Emerging Infectious Diseases (ELC) cooperative agreement. This work was also funded by a grant from the Agency for Healthcare Research and Quality (AHRQ) (grant number 1R03HS028693-01). The content is solely the responsibility of the authors and does not necessarily represent the official views of the funding agencies.
AUTHOR CONTRIBUTIONS
SR and GBM led the drafting of the manuscript and all authors read and approved the final version. All authors (SR, HC, AB, AL, JS, RO, AS, GG, KW, SS, and GBM) contributed to the content in this manuscript.
SUPPLEMENTARY MATERIAL
Supplementary material is available at JAMIA Open online.
ACKNOWLEDGMENTS
The authors express gratitude to the Minnesota IT (MN.IT) team at the Minnesota Department of Health (MDH), the immunization information systems team at the North Dakota Department of Health, and to the University of Minnesota Center for Learning Health System Sciences, a partnership between the University of Minnesota Medical School and the School of Public Health.
CONFLICT OF INTEREST STATEMENT
None declared.
DATA AVAILABILITY
Data in the disease surveillance system (MEDSS) and in the immunization information system (MIIC) are reported to public health and cannot be shared for ethical/privacy reasons.