-
PDF
- Split View
-
Views
-
Cite
Cite
Kim M Unertl, Laurie Lovett Novak, Courtney Van Houten, JoAnn Brooks, Andrew O Smith, Joyce Webb Harris, Taylor Avery, Christopher Simpson, Nancy M Lorenzi, Organizational diagnostics: a systematic approach to identifying technology and workflow issues in clinical settings, JAMIA Open, Volume 3, Issue 2, July 2020, Pages 269–280, https://doi.org/10.1093/jamiaopen/ooaa013
- Share Icon Share
Abstract
Healthcare organizations need to rapidly adapt to new technology, policy changes, evolving payment strategies, and other environmental changes. We report on the development and application of a structured methodology to support technology and process improvement in healthcare organizations, Systematic Iterative Organizational Diagnostics (SIOD). SIOD was designed to evaluate clinical work practices, diagnose technology and workflow issues, and recommend potential solutions.
SIOD consists of five stages: (1) Background Scan, (2) Engagement Building, (3) Data Acquisition, (4) Data Analysis, and (5) Reporting and Debriefing. Our team applied the SIOD approach in two ambulatory clinics and an integrated ambulatory care center and used SIOD components during an evaluation of a large-scale health information technology transition.
During the initial SIOD application in two ambulatory clinics, five major analysis themes were identified, grounded in the data: putting patients first, reducing the chaos, matching space to function, technology making work harder, and staffing is more than numbers. Additional themes were identified based on SIOD application to a multidisciplinary clinical center. The team also developed contextually grounded recommendations to address issues identified through applying SIOD.
The SIOD methodology fills a problem identification gap in existing process improvement systems through an emphasis on issue discovery, holistic clinic functionality, and inclusion of diverse perspectives. SIOD can diagnose issues where approaches as Lean, Six Sigma, and other organizational interventions can be applied.
The complex structure of work and technology in healthcare requires specialized diagnostic strategies to identify and resolve issues, and SIOD fills this need.
LAY SUMMARY
The article describes how we developed and tested tools to understand problems with how clinics work and with software used in clinics. Our team built on existing research and theory to develop Systematic Iterative Organizational Diagnostics (SIOD). Using SIOD, we did workshops with healthcare teams, observed in clinics, interviewed people, and collected data created as people use software. We analyzed all the data to build a picture of what was happening in each clinic and why. We used SIOD in several clinics, learned about problems with how clinics worked, and found causes of these problems. For example, ordering important tests in one clinic was confusing and complicated. This caused extra work, frustration, and delays. Many problems that we found were caused by the combination of work and technology, because software did not do what people needed and work in the clinics was not efficient or effective. We handed off suggestions on how to fix the problems to leaders who could make changes. We want to give the SIOD tools to other people because our results show SIOD can help improve how clinics work, which helps healthcare teams and patients.
BACKGROUND AND SIGNIFICANCE
Healthcare organizations are under enormous pressure to improve access, reduce expenses, and optimize operational processes.1 Achieving peak efficiency and effectiveness is challenging, as healthcare organizations are highly complex sociotechnical systems.2 Making changes to optimize functionality in one area can lead to unintended negative consequences in other areas.3,4 As new health information technology (IT) is introduced into an organization, conflict can arise between routine practices already in place and process requirements related to new technology.5,6 An evaluation of current workflow and operational practices is critical to ensure that health IT can be smoothly integrated, but too often is lost in the rush toward change.7–9
Health care is not alone in struggling to address work optimization and to resolve issues related to technology implementation. Multiple fields, including manufacturing,10 financial services,11,12 education,13,14 and hospitality,15,16 have applied a variety of process improvement approaches to identify organizational issues, to locate waste within processes, and to optimize service delivery. Although process improvement systems abound, major systems in widespread use around the United States include Business Process Management,17,18 Kaizen,19–21 Lean,22,23 Six Sigma,24–26 and Theory of Constraints.27–29 Each process improvement system has its own main foci, methods, and outputs (Table 1). Systems are also frequently combined to capitalize on common goals and for a more comprehensive assessment (eg, Lean Six Sigma30,31)
Process improvement system . | Origin . | Main emphasis . | When applied; who is involved . | Methods . | Potential outcomes . |
---|---|---|---|---|---|
Business Process Management17,18 | Operations management | Development, maintenance, and optimization of repetitive ongoing core business processes | Used as part of a transition to new processes, to improve existing processes, or to automate processes; management-driven | Process management life cycle consisting of designing processes, modeling processes using software, executing the processes, monitoring the processes, and optimizing processes based on data | Identification and optimization of core processes |
Kaizen19–21 | Organizational theory and quality management, grounded in culture | Culture to support continuous process improvement | Continuously; involving all employees | Cycles of reviewing the current state, suggesting and implementing potential improvements, and evaluating change | Incremental improvements, occurring over time |
Lean22,23 | Initially developed in and applied to automobile manufacturing (Toyota) | Improving manufacturing process flow through removal of waste (eg, overproduction, delays, defects) | Applied as part of process improvement efforts; multidisciplinary team including front line workers | Toolbox of methods including value stream mapping, Kanban, standardized work, and additional methods | Reduction in waste while maintaining productivity |
Six Sigma24–26 | Introduced in the 1980s at Motorola; popularized by GE | Statistical analysis of process performance and quality data with the aim of decreasing variation | After identification of a business problem; leaders who have completed Six Sigma training and additional employees | Quantitative data analytics. Major steps: define, measure, analyze, improve, control (DMAIC) | Decreased variation in processes and outputs |
Theory of Constraints27–29 | Manufacturing operations and business management research | Identifying and resolving constraints that limit efficiency of interdependent processes | Iterative cycles of constraint identification and resolution; management, finance, sales/marketing |
| Increased capacity and throughput |
Process improvement system . | Origin . | Main emphasis . | When applied; who is involved . | Methods . | Potential outcomes . |
---|---|---|---|---|---|
Business Process Management17,18 | Operations management | Development, maintenance, and optimization of repetitive ongoing core business processes | Used as part of a transition to new processes, to improve existing processes, or to automate processes; management-driven | Process management life cycle consisting of designing processes, modeling processes using software, executing the processes, monitoring the processes, and optimizing processes based on data | Identification and optimization of core processes |
Kaizen19–21 | Organizational theory and quality management, grounded in culture | Culture to support continuous process improvement | Continuously; involving all employees | Cycles of reviewing the current state, suggesting and implementing potential improvements, and evaluating change | Incremental improvements, occurring over time |
Lean22,23 | Initially developed in and applied to automobile manufacturing (Toyota) | Improving manufacturing process flow through removal of waste (eg, overproduction, delays, defects) | Applied as part of process improvement efforts; multidisciplinary team including front line workers | Toolbox of methods including value stream mapping, Kanban, standardized work, and additional methods | Reduction in waste while maintaining productivity |
Six Sigma24–26 | Introduced in the 1980s at Motorola; popularized by GE | Statistical analysis of process performance and quality data with the aim of decreasing variation | After identification of a business problem; leaders who have completed Six Sigma training and additional employees | Quantitative data analytics. Major steps: define, measure, analyze, improve, control (DMAIC) | Decreased variation in processes and outputs |
Theory of Constraints27–29 | Manufacturing operations and business management research | Identifying and resolving constraints that limit efficiency of interdependent processes | Iterative cycles of constraint identification and resolution; management, finance, sales/marketing |
| Increased capacity and throughput |
Process improvement system . | Origin . | Main emphasis . | When applied; who is involved . | Methods . | Potential outcomes . |
---|---|---|---|---|---|
Business Process Management17,18 | Operations management | Development, maintenance, and optimization of repetitive ongoing core business processes | Used as part of a transition to new processes, to improve existing processes, or to automate processes; management-driven | Process management life cycle consisting of designing processes, modeling processes using software, executing the processes, monitoring the processes, and optimizing processes based on data | Identification and optimization of core processes |
Kaizen19–21 | Organizational theory and quality management, grounded in culture | Culture to support continuous process improvement | Continuously; involving all employees | Cycles of reviewing the current state, suggesting and implementing potential improvements, and evaluating change | Incremental improvements, occurring over time |
Lean22,23 | Initially developed in and applied to automobile manufacturing (Toyota) | Improving manufacturing process flow through removal of waste (eg, overproduction, delays, defects) | Applied as part of process improvement efforts; multidisciplinary team including front line workers | Toolbox of methods including value stream mapping, Kanban, standardized work, and additional methods | Reduction in waste while maintaining productivity |
Six Sigma24–26 | Introduced in the 1980s at Motorola; popularized by GE | Statistical analysis of process performance and quality data with the aim of decreasing variation | After identification of a business problem; leaders who have completed Six Sigma training and additional employees | Quantitative data analytics. Major steps: define, measure, analyze, improve, control (DMAIC) | Decreased variation in processes and outputs |
Theory of Constraints27–29 | Manufacturing operations and business management research | Identifying and resolving constraints that limit efficiency of interdependent processes | Iterative cycles of constraint identification and resolution; management, finance, sales/marketing |
| Increased capacity and throughput |
Process improvement system . | Origin . | Main emphasis . | When applied; who is involved . | Methods . | Potential outcomes . |
---|---|---|---|---|---|
Business Process Management17,18 | Operations management | Development, maintenance, and optimization of repetitive ongoing core business processes | Used as part of a transition to new processes, to improve existing processes, or to automate processes; management-driven | Process management life cycle consisting of designing processes, modeling processes using software, executing the processes, monitoring the processes, and optimizing processes based on data | Identification and optimization of core processes |
Kaizen19–21 | Organizational theory and quality management, grounded in culture | Culture to support continuous process improvement | Continuously; involving all employees | Cycles of reviewing the current state, suggesting and implementing potential improvements, and evaluating change | Incremental improvements, occurring over time |
Lean22,23 | Initially developed in and applied to automobile manufacturing (Toyota) | Improving manufacturing process flow through removal of waste (eg, overproduction, delays, defects) | Applied as part of process improvement efforts; multidisciplinary team including front line workers | Toolbox of methods including value stream mapping, Kanban, standardized work, and additional methods | Reduction in waste while maintaining productivity |
Six Sigma24–26 | Introduced in the 1980s at Motorola; popularized by GE | Statistical analysis of process performance and quality data with the aim of decreasing variation | After identification of a business problem; leaders who have completed Six Sigma training and additional employees | Quantitative data analytics. Major steps: define, measure, analyze, improve, control (DMAIC) | Decreased variation in processes and outputs |
Theory of Constraints27–29 | Manufacturing operations and business management research | Identifying and resolving constraints that limit efficiency of interdependent processes | Iterative cycles of constraint identification and resolution; management, finance, sales/marketing |
| Increased capacity and throughput |
Applying these process improvement systems to healthcare has proven challenging.32,33 Many systems described in Table 1 originated in manufacturing, presenting difficulty when applying them to a service-oriented industry such as health care, where new technologies affect collaboration in ways that generate unintended consequences.34 Healthcare as an institutional sector is not as amenable as manufacturing to top-down templates for designing process improvements, because after designed changes are implemented there is a ripple effect of emergent changes in practice.35,36 Barriers such as the complexity of healthcare delivery,37 necessary process variability to cope with acute patient care situations,38 and differences between the goals of manufacturing and health care39,40 have hampered efforts to achieve expected benefits of using standard process improvement systems in health care.
Researchers in healthcare and in medical informatics have made progress in assessing organizational issues, identifying contextual factors that impact healthcare work, and understanding interactions between health IT and healthcare organizations.41–46 Focus areas have ranged from identifying organizational issues in health IT implementation to studying the unintended consequences of health IT on workers and organizations.47–49 Professional organizations such as the American Medical Informatics Association (AMIA) and the International Medical Informatics Association (IMIA) have maintained longstanding interests in these topics, including working groups focused on People and Organizational Issues (AMIA) and Organizational and Social Issues (IMIA) that were established more than 25 years ago. This depth of prior informatics research provides an exemplary foundation on organizational aspects of informatics interventions, while continuing informatics research provides a strong emphasis on specific aspects of health IT design and implementation.50–53 However, opportunities remain to continue advancing the understanding of informatics as a component of a holistic system, the impact of health IT on organizations and individual workers, and the tools available to researchers and practitioners to diagnose organizational issues.
The research question that motivated this research and operational project was how can healthcare organizations build on existing process improvement frameworks and the foundations of organizational research in informatics to systematically diagnose key organizational issues with an emphasis on health IT and to identify evidence-based pathways for improvement? The element of diagnosis is key. In other words, how can we assess the overall health of the “patient,” (ie, the organization) and identify problems to address, before, during, and after the administration of the “medicine” (ie, process improvement methodology)? To this end, we developed and applied an integrated set of assessment methods known as Systematic Iterative Organizational Diagnostics (SIOD). The purpose of this article is to report on the development of SIOD, to describe the outcomes of initial applications within one organization, and to describe how this approach can be implemented widely, with attention to its articulation with other process improvement systems such as Lean and Six Sigma.
DESIGN PROCESS FOR SIOD
Over the last 4 years, our team iteratively developed a comprehensive diagnostic method combining social science methods with data analytics. Our initial discussions with clinical stakeholders suggested that the primary process improvement framework used at the institution, Lean,22,23 left important questions unanswered. Concerns voiced by our clinical partners included components addressed by Lean (eg, waste, inefficiency), but also broader concerns about overall clinic functioning, including questions about cultural, process, and technology issues. Given our knowledge of the context, environment, and the broad concerns of our clinical partners, we drew on two theoretical frameworks to guide the development of SIOD: Reddy’s54–56 organizational psychology work on diagnosing team problem solving effectiveness and the theoretical underpinnings of practical participatory evaluation.57 We built the components of SIOD based on Reddy’s team assessment approach, combined with the core components of the practical participatory evaluation framework: (1) conditions, (2) process characteristics/mechanisms, (3) process results/process use, and (4) results/use of findings.
Building on the theoretical frameworks, we used an initial structure typical to many process improvement approaches as the foundation of SIOD: entry into the field site, data collection, analysis, and feedback. We explored a variety of methods and tools to support entry and data collection processes. We also added components to the frameworks to address needs to develop deeper contextual knowledge, to extend data analysis, and to deliver more meaningful reports to the clinical sites. As we applied SIOD to different clinical contexts, we used lessons from each application to revise and extend SIOD’s stages and components. The SIOD process was designed to use well-established methods in a systematic way to diagnose current organizational issues at a holistic level, incorporating people, process, and technology components. Key design principles included engagement with healthcare team members and management, integration of a variety of data collection and analysis methods, and a holistic perspective focused not only on specific aspects of care delivery but also on each clinical context as a whole system. The current version of SIOD consists of five main stages: Background Scan, Engagement Building, Data Acquisition, Data Analysis, and Reporting and Debriefing (Figure 1).
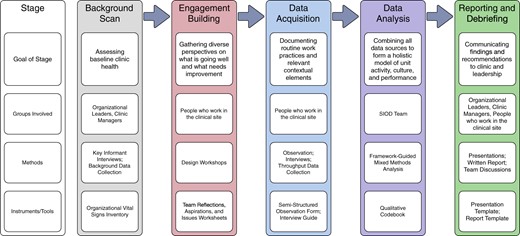
The goals of the Background Scan phase were to gather foundational information about the characteristics of the clinical environment and to build rapport and trust needed for next SIOD stages. Two primary methods were used for this phase. We collected baseline quantitative and descriptive data about the clinical environment, such as staffing model, patient load, throughput, staff roles, and characteristics of the patient population. We also conducted open-ended key informant interviews58 that served to establish rapport and to assist with establishing the goals of the SIOD process from each clinical site’s perspective. In addition, our team gathered information about any recent or current quality improvement projects to avoid duplication of effort and to understand the outcomes of previous work.
During Engagement Building, the research group engaged as many members of the healthcare team as possible at each clinical site through design workshops based on concepts developed by Reddy.56 In addition to building engagement and rapport with the clinical site, this stage also allowed us to continue gathering contextual information and to broaden our team’s understanding of the perspectives of healthcare team members at the site. We used a variety of tools to gather data in this phase, including individual worksheets (see Supplementary Appendix) and whiteboards to document the collective comments of the participants. Initial sessions separated groups based on role, giving each group (eg, nurses, physicians, administrative staff) opportunities to discuss concerns about the reporting hierarchy or other sensitive issues.59 A primary facilitator guided each group through the discussion, with additional facilitators on hand to assist and document.
In the Data Acquisition stage, qualitative and quantitative methods were used to collect detailed data about clinic workflow, roles, spatial layout, and other contextual factors. Observation and interviews were the primary methods in this stage, with a semi-structured observation template and semi-structured interview guide (see Supplementary Appendix) to guide data collection while allowing flexibility for specific contexts or subjects. Observers asked questions of people being observed as time and circumstances allowed. Questions helped to clarify observations and add details about decision-making, while minimizing disruptions to work processes. Field notes were transcribed and uploaded to Dedoose,60 a qualitative data analysis tool, where they were collated with other data. Quantitative data focused on markers of throughput and workflow, such as appointment scheduling (eg, appointment scheduling patterns for individual providers).
In the Data Analysis stage, qualitative and quantitative data were initially analyzed separately and then combined to identify gaps in the data acquisition process and to triangulate patterns across data sources. This stage was when diagnosis occurred, when reported issues (symptoms) were analyzed alongside objective data on performance and other process data. Based on these additional data, the goal was to diagnose causes of problems participants reported and of additional problems identified by the SIOD team. For example, in one of our projects, clinic staff reported that sub-departments of a clinic experienced different workloads, partially due to staffing issues. However, by analyzing additional qualitative and quantitative data, we identified that an outdated patient triaging algorithm was resulting in a bottleneck in one of the sub-departments in the clinic. The result was a backup of patients in one sub-department and excess capacity in another. During the initial development of SIOD, qualitative data were analyzed using an iterative open-ended approach,61 which led to the development of a codebook for application across data sets. While this codebook provided important continuity across multiple sites, we continued refining the codebook over time to address additional themes and concepts from new sites. Notably, Data Acquisition and Data Analysis stages overlapped to a degree, as the team identified additional needs for information or questions that needed deeper investigation during Data Analysis, leading to iterative cycles of data collection and data analysis. As part of the Data Analysis stage, the team began to identify potential improvements/solutions for issues identified in the data. These initial improvements/solutions were compiled and used in the final SIOD stage.
The Reporting and Debriefing stage involved presenting our findings to multiple stakeholder groups. Reporting sessions provided a form of member checking,62 where the assessment team verified our interpretation of the data with people from the clinical site. Major stakeholder groups included the sponsoring organizational management representatives, clinical site leadership, and staff/providers from the clinical site. This stage served four purposes: handing off recommendations to leaders with the authority and resources to implement change, determining which recommendations organizational and site leadership want to pursue, sharing cross-organizational issues, and assessing the SIOD process as a foundation for further process development changes. As each project ended, our team also reviewed the SIOD process, identifying areas where new methods of data collection and analysis were needed and assessing our approaches for engaging with participants.
Work that attempts to diagnose organizational issues through a broad assessment should address all of the elements described above. The SIOD stages may be considered inclusive, yet when applied in specific contexts there may be more granular stages or topics emphasized in each stage. SIOD is novel because it blends methodological (qualitative, quantitative) perspectives, operates at multiple organizational levels to produce holistic analyses and solutions, and addresses accountability in its dissemination phase.
APPLYING SIOD TO CLINICAL SITES
As of August 2019, our team has applied the SIOD approach in two ambulatory clinics and one multidisciplinary clinical center (MCC). In addition, the group used components of SIOD during a longitudinal evaluation of an organization-wide large-scale health IT implementation. We have included a case study of the application of SIOD to the MCC in Box 1, and process evaluation data related to the case study in Table 2. The case study described in Box 1 represents an upper boundary of the time and resource requirements for applying SIOD, due to the complexity of the clinical context. While the SIOD application to the MCC was ∼300 h total, estimates of time required for each individual ambulatory clinic application of SIOD were 25–30% of the MCC time, or 75–90 h each.
Case study of the application of SIOD to a multidisciplinary clinical center
A senior leader with dual oversight in both academic and clinical parts of the organization approached the SIOD team in October 2017, requesting the team’s expertise and guidance on addressing organizational challenges experienced by a complex MCC. The MCC has a complex organizational structure. The portion of the MCC that the SIOD team studied included multiple clinical specialties, as well as related services (eg, radiology, pharmacy, procedure area) that reported to different portions of the organization. The leaders who requested the SIOD team’s assistance identified concerns with workflow, efficiency, and patient throughput. Due to SIOD team commitments related to a large-scale health IT transition across the entire organization, the project was shelved until June 2018.
As our team re-engaged with the MCC, we started the first phase of SIOD, the Background Scan. Members of the team (NML, JWH) met with clinical leaders, across organizational levels and within the MCC, and gathered current information on staffing and other aspects of the clinic. The Background Scan meetings and data provided the SIOD team with updated information on pressing MCC concerns after the large-scale health IT transition, which remained consistent with their pre-transition concerns.
With support from MCC leadership, the SIOD team organized multiple design workshops as part of the Engagement Building phase. We held six workshops organized by role, with some crossover between groups to fit scheduling needs: radiology technicians, nurses, nurse practitioners, patient service specialists (eg, front desk staff), research nurses, and medical assistants. We also held three one-on-one workshops with physicians, using the same data collection instrument as the group workshops but held individually due to scheduling constraints. There were 42 participants total across the nine workshops. Two to four SIOD team members conducted each workshop: one team member led the workshop and other team members provided logistical support, fielded questions, and assisted with data collection. After each workshop, key artifacts (eg, worksheets, summary notes) were transcribed and analyzed for main themes and potential data collection focus areas.
We then moved into the Data Acquisition stage, conducting 21 individual interviews and engaging in direct observation for ∼11 h across seven working groups/areas. Each interview involved one or two members of the SIOD team, applying a semi-structured interview guide to gather perspectives on workflow, technology, and other organizational topics. Each observation session involved a member of the SIOD team observing members of the MCC staff and recording notes.
Interview recordings and observation notes were transcribed and analyzed during the Data Analysis stage using a previously developed qualitative codebook, although significant codebook additions were required due to the scale and multidisciplinary structure of the MCC. Data collection and data analysis were iterative, meaning that data were analyzed and then we collected additional data to answer specific questions emerging from the analysis. Two team members (JWH, CS) completed the majority of coding, but data collection and analysis were extensively discussed during team meetings to ensure all team members were in agreement with data collection needs and with the analysis. We identified a core set of recommendations, grounded in the data, for initial improvements/solutions and collaboratively ranked potential solutions by difficulty of implementation and potential impact in the specific context of the MCC and parent organization.
Finally, in the Reporting and Debriefing stage, SIOD team members held four meetings with different groups to disseminate the results of our analysis. First, team members met with the director of the MCC to share our findings and as a form of member checking. Our team then met with senior organizational clinical leaders, including the MCC director, and with the goal of handing off recommendations. The final two meetings were held with physicians and staff of the MCC, and included member checking of our analysis and a robust discussion of next steps. The final report out meeting from the SIOD team to MCC staff was held in June 2019. The timeline for the project extended beyond the original timeline, due to both resource constraints for the SIOD team and organizational constraints at the MCC. Approximately 40 individuals from the MCC and organizational leadership participated across the four meetings.
The SIOD team has continued to follow-up with the MCC leadership to assess how our recommendations have been applied and to gather additional feedback on our processes. Although some recommendations have already been implemented (eg, holding an all-team meeting to build engagement and alignment), other recommendations will take longer to fully resolve (eg, addressing alignment across parts of the MCC that report to different parts of the organization). This reflects the complex, long-term nature of work at the intersection of organizations and technology. Table 2 summarizes process evaluation components for this case.
Process evaluation data from application of SIOD to a multidisciplinary clinical center
Process evaluation category . | Supporting data . |
---|---|
Process duration from initial contact to final report |
|
Time spent on each SIOD stage |
|
Team composition |
|
Team expertise | All team members had prior experience with qualitative methods and organizational studies; the graduate student also had expertise in data analytics. |
Other available resources beyond staffing |
|
Organizational support components |
|
Process evaluation category . | Supporting data . |
---|---|
Process duration from initial contact to final report |
|
Time spent on each SIOD stage |
|
Team composition |
|
Team expertise | All team members had prior experience with qualitative methods and organizational studies; the graduate student also had expertise in data analytics. |
Other available resources beyond staffing |
|
Organizational support components |
|
SIOD: Systematic Iterative Organizational Diagnostics.
Process evaluation data from application of SIOD to a multidisciplinary clinical center
Process evaluation category . | Supporting data . |
---|---|
Process duration from initial contact to final report |
|
Time spent on each SIOD stage |
|
Team composition |
|
Team expertise | All team members had prior experience with qualitative methods and organizational studies; the graduate student also had expertise in data analytics. |
Other available resources beyond staffing |
|
Organizational support components |
|
Process evaluation category . | Supporting data . |
---|---|
Process duration from initial contact to final report |
|
Time spent on each SIOD stage |
|
Team composition |
|
Team expertise | All team members had prior experience with qualitative methods and organizational studies; the graduate student also had expertise in data analytics. |
Other available resources beyond staffing |
|
Organizational support components |
|
SIOD: Systematic Iterative Organizational Diagnostics.
Over the course of applying the SIOD approach across multiple contexts, we developed a coding schema grounded in the data, used for qualitative data analysis and integrated into presentations at the end of SIOD assessments. The schema includes five major categories: putting patients first, reducing the chaos, matching space to function, technology making work harder, and staffing is more than numbers.
Putting patients first focused on the connections and gaps between the concept of patient-centered care and day-to-day requirements of clinical work. Across all clinical sites that we worked with, staff and providers were focused on delivering the highest quality patient care in an equitable fashion to all patients. The resulting personal connection with patients is a key distinction between health care and other industries. Building rapport with the staff in the early period of the engagement resulted in extensive reporting of their experiences and barriers to patient-centeredness that they encounter.
Reducing the chaos focused on the concept that although adaptability and organizational resilience are strengths, rapidly changing contextual factors (eg, new health IT features, new processes for collecting information, rapidly evolving patient therapeutic regimens, workarounds for technology or workflow issues) can lead to variable, inefficient, and confusing workflow.
Matching space to function recognized the fact that space is a finite resource, existing spatial allocations are the result of a long history of political negotiations, and that healthcare organizations need tools to optimize how available space is used.
Technology making work harder focused on how technology or the implementation of technology increases difficulty in clinical work. Although health IT has potential to make clinical work easier and build connections across healthcare teams, in some cases health IT increased workloads, disrupted work practices, and introduced communication challenges.
Finally, Staffing is more than numbers focused on the idea that staffing is not just about the number of people working in a clinical site, but rather how staff are deployed (eg, scheduling), how staff roles are defined, and how satisfied staff are with the working environment. Although in many cases, strategies to optimize staffing structures were a main finding, in one project we also identified a strong need for additional staffing, as the site was so substantially under-resourced that strategies to optimize workflow and workload could not feasibly be implemented.
The core of the coding schema has been consistent. However, the complex MCC site required additional sections in the coding schema. For example, due to its multidisciplinary nature, components of the center reported to different parts of the organization, leading to discordant priorities in different parts of the center and disconnects related to staffing, budget, and workflow. Impact of organizational structure was not an area previously addressed in our coding schema and was incorporated into the schema as part of the SIOD engagement with the center. Across all categories in the coding schema, SIOD uncovered unintended consequences of well-meaning previous interventions or changes and identified knowledge that might assist with preventing or minimizing future unintended consequences. This was especially notable with changes made to improve a specific process or functionality in an area of a clinic, which were successful in improving that specific process/area but had downstream impacts on other people, areas, and processes (eg, additional work in another area, delays in other processes, loss of information). By taking a holistic perspective toward clinic functionality when applying SIOD, our team was able to highlight connections among people and processes in different parts of a clinic or center, providing information crucial to avoiding unexpected consequences in future interventions.
For each SIOD project, we identified recommendations for improvements, which our team handed off to clinical and organizational leadership. Examples of recommendations are shown in Table 3. Recommendations spanned a variety of topics, with the scope of some recommendations being clinic- or center-specific (Local) and some recommendations potentially for application across the entire organization (Global). Our data analysis process also identified cascading chains of recommendations, where acting on a higher-level proposal would also resolve multiple smaller issues. Each recommendation was mapped onto a 2 × 2 matrix63 with axes of potential implementation effort (low to high) and potential impact (low to high). The quadrants of the matrix (eg, “high potential effort, high potential impact”) provided guidance to assist leadership with prioritizing interventions aligned with available resources to maximize benefits. For example, the recommendation from Table 3 to “Implement electronic ordering for complex laboratory tests” was a technology-based solution that would require high effort to implement but also had a high potential impact, along with a cascade of problems that would be resolved involving delays due to incomplete information, front desk staff job satisfaction, and physician workload.
Diagnosis . | Recommendation . | Potential effort . | Potential impact . | Scope . | Cascade? . |
---|---|---|---|---|---|
Patients having difficulty opening some exam room doors; nurses unable to tell if these rooms are available | Remove door closers on exam room doors | Low | Moderate | Local | No |
Individual physicians are not full time in a clinic/center and sometimes create individual processes that conflict with overall clinic/center processes | Develop strategies to integrate physicians more fully into overall standard clinic/center processes | High | High | Local | Could decrease effort involved in optimizing processes; could improve flexibility of staffing support across the clinic/center |
Front desk staff using manual process to assist with complex test ordering; complaints and delays due to incomplete information and errors | Implement electronic ordering for complex laboratory tests | High | High | Global | Could assist with staff training and retention; could decrease delays related to information |
Length of patient education appointments and volume of information presented can be overwhelming for patients | Develop/purchase patient education videos to complement nurse practitioner training | Low | Moderate | Local | No |
Patients and informal caregivers have difficulty getting timely completion of paperwork; lack of clear chain of responsibility for paperwork completion | Develop Family Medical Leave Act and Disability paperwork tracking | Moderate | High | Global | No |
Satisfaction levels of front desk staff; issues with staff retention | Identify and implement career growth pathways for front desk staff | High | High | Global | Could resolve additional issues with use of staff pool and training |
Diagnosis . | Recommendation . | Potential effort . | Potential impact . | Scope . | Cascade? . |
---|---|---|---|---|---|
Patients having difficulty opening some exam room doors; nurses unable to tell if these rooms are available | Remove door closers on exam room doors | Low | Moderate | Local | No |
Individual physicians are not full time in a clinic/center and sometimes create individual processes that conflict with overall clinic/center processes | Develop strategies to integrate physicians more fully into overall standard clinic/center processes | High | High | Local | Could decrease effort involved in optimizing processes; could improve flexibility of staffing support across the clinic/center |
Front desk staff using manual process to assist with complex test ordering; complaints and delays due to incomplete information and errors | Implement electronic ordering for complex laboratory tests | High | High | Global | Could assist with staff training and retention; could decrease delays related to information |
Length of patient education appointments and volume of information presented can be overwhelming for patients | Develop/purchase patient education videos to complement nurse practitioner training | Low | Moderate | Local | No |
Patients and informal caregivers have difficulty getting timely completion of paperwork; lack of clear chain of responsibility for paperwork completion | Develop Family Medical Leave Act and Disability paperwork tracking | Moderate | High | Global | No |
Satisfaction levels of front desk staff; issues with staff retention | Identify and implement career growth pathways for front desk staff | High | High | Global | Could resolve additional issues with use of staff pool and training |
SIOD: Systematic Iterative Organizational Diagnostics.
Diagnosis . | Recommendation . | Potential effort . | Potential impact . | Scope . | Cascade? . |
---|---|---|---|---|---|
Patients having difficulty opening some exam room doors; nurses unable to tell if these rooms are available | Remove door closers on exam room doors | Low | Moderate | Local | No |
Individual physicians are not full time in a clinic/center and sometimes create individual processes that conflict with overall clinic/center processes | Develop strategies to integrate physicians more fully into overall standard clinic/center processes | High | High | Local | Could decrease effort involved in optimizing processes; could improve flexibility of staffing support across the clinic/center |
Front desk staff using manual process to assist with complex test ordering; complaints and delays due to incomplete information and errors | Implement electronic ordering for complex laboratory tests | High | High | Global | Could assist with staff training and retention; could decrease delays related to information |
Length of patient education appointments and volume of information presented can be overwhelming for patients | Develop/purchase patient education videos to complement nurse practitioner training | Low | Moderate | Local | No |
Patients and informal caregivers have difficulty getting timely completion of paperwork; lack of clear chain of responsibility for paperwork completion | Develop Family Medical Leave Act and Disability paperwork tracking | Moderate | High | Global | No |
Satisfaction levels of front desk staff; issues with staff retention | Identify and implement career growth pathways for front desk staff | High | High | Global | Could resolve additional issues with use of staff pool and training |
Diagnosis . | Recommendation . | Potential effort . | Potential impact . | Scope . | Cascade? . |
---|---|---|---|---|---|
Patients having difficulty opening some exam room doors; nurses unable to tell if these rooms are available | Remove door closers on exam room doors | Low | Moderate | Local | No |
Individual physicians are not full time in a clinic/center and sometimes create individual processes that conflict with overall clinic/center processes | Develop strategies to integrate physicians more fully into overall standard clinic/center processes | High | High | Local | Could decrease effort involved in optimizing processes; could improve flexibility of staffing support across the clinic/center |
Front desk staff using manual process to assist with complex test ordering; complaints and delays due to incomplete information and errors | Implement electronic ordering for complex laboratory tests | High | High | Global | Could assist with staff training and retention; could decrease delays related to information |
Length of patient education appointments and volume of information presented can be overwhelming for patients | Develop/purchase patient education videos to complement nurse practitioner training | Low | Moderate | Local | No |
Patients and informal caregivers have difficulty getting timely completion of paperwork; lack of clear chain of responsibility for paperwork completion | Develop Family Medical Leave Act and Disability paperwork tracking | Moderate | High | Global | No |
Satisfaction levels of front desk staff; issues with staff retention | Identify and implement career growth pathways for front desk staff | High | High | Global | Could resolve additional issues with use of staff pool and training |
SIOD: Systematic Iterative Organizational Diagnostics.
A major component of all SIOD applications involves a direct and tight hand-off of these recommendations to the parent organization. For example, in one case, the hand-off included a review of each recommendation at a senior leadership level, with specific individuals assigned to each recommendation and a request to present updates on a quarterly basis in organizational reporting channels. In another case, a senior manager was assigned to oversee recommendation implementation, which included the manager developing a spreadsheet to provide help with coordination and updating on each recommendation.
Throughout all applications of the SIOD process, our team focused on identifying aspects of the methodology that delivered the most benefits, determining areas for methodology improvement, and refining data collection instruments and data analysis process. During and after each application of SIOD, the SIOD team conducted elements of a formative evaluation of the SIOD process. The team met frequently during each SIOD application and held additional discussions to monitor the effectiveness of the SIOD tools and processes and to gather feedback to enhance the processes. Between SIOD applications, the team remained in contact with our clinical partners to understand the impact of the process and recommendations and to identify suggestions for future applications to additional clinical domains. A summative evaluation of the SIOD methodology is a long-term goal of the SIOD team.
HOW SIOD FITS WITH OTHER PROCESS IMPROVEMENT SYSTEMS
The SIOD process was designed to deliver comprehensive diagnostic assessments, informed by three resources: (1) contextual knowledge specific to each clinical environment, (2) broader organizational knowledge, and (3) expertise in clinical workflow, organizational studies, and clinical informatics. A major emphasis was identifying connections across areas within the clinical environment and to the organization as a whole. For example, when examining the impact of health IT on physician workflow, our team also identified ways in which front desk and nursing processes affected physician workflow and vice versa, how physician workflow directly and indirectly affected other areas of the clinic. Throughout the discovery process, SIOD was designed to engage employees at all levels in problem identification, both to ensure representation of different viewpoints on issues and to increase receptivity to future changes. We used methods to gather both internal viewpoints (eg, design workshops, interviews) and to add externally interpreted detail (eg, observation, quantitative metrics). Although several existing process improvement systems include components of discovery, such as value stream mapping in Lean, the focus on systematic problem identification in situ from diverse perspectives and on identifying system-level connections among clinical areas and processes were two major distinguishing factors of SIOD.
SIOD was also designed for smooth hand-off of identified issues to leadership for decisions regarding if, when, and how recommended changes might be implemented. In several of our SIOD projects, our recommendations included a need for additional data gathering and analysis using other process improvement systems to help the organization identify pathways to implement needed change. For example, one clinic used a scoring system to assess cases based on resource utilization, but the scoring system was outdated and did not reflect current practices and actual resource utilization, resulting in inaccurate staffing decisions. We recommended that the clinic undertake a project to redevelop their scoring system, work that was out of scope for the SIOD engagement. In addition, potential solutions identified through SIOD were flexible, allowing for the development of a variety of evidence-based solutions, including job analysis, process redesign, and technology changes. For example, when specific workflow issues were identified, methods from Lean were brought in after our SIOD process was completed to map out needed workflow changes and pathways to change implementation. When gaps related to lack of data-driven feedback strategies were identified, tools from Six Sigma offered a more quantitative approach to solution development based in data analytics. When issues related to the integration of health IT into clinical work were identified, methods from software engineering and medical informatics were needed to modify technology to better meet the needs of the clinical environment.
The purpose of SIOD was not to provide an end point in process improvement, but rather to improve problem diagnosis, to deliver initial recommendations, and to provide data that could drive additional process improvement work (Figure 2). For example, as shown in Figure 2, issues that are identified through SIOD that are related to process efficiency (eg, workflow related to appointment scheduling) could be directed to a Lean process mapping event,64 to gather a multidisciplinary team that can focus on mapping out an existing process, identifying bottlenecks and gaps, and determining specific steps for improvement. In addition, or as an alternative approach, tools from Six Sigma could be used to focus on specific aspects of an issue identified through SIOD. For example, if SIOD diagnosed issues with delays in patient care introduced by errors in complex laboratory test ordering, the organization could conduct a DMAIC cycle65 to define the problem, quantitatively measure aspects of the process including order error rate and time to various aspects of care delivery, analyze the quantitative data to determine root causes of the issues, implement improvements to address the root causes, and then control over time to ensure new processes are followed and errors are reduced. The goal of SIOD is not to replace these other process improvement systems, but rather to provide a first stage of problem diagnosis to better hone the application of other systems.
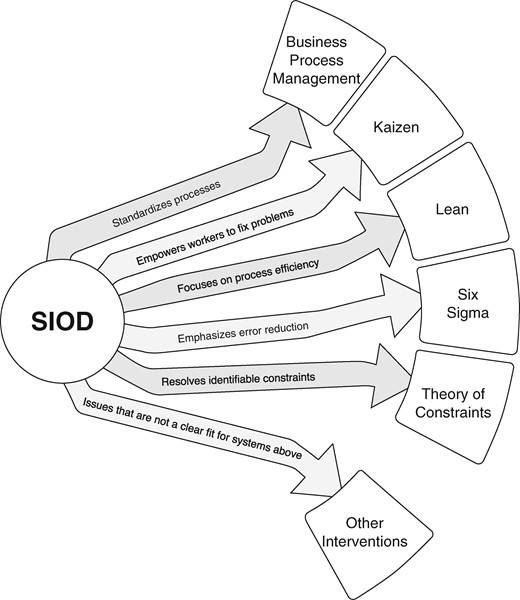
Potential Connections between SIOD and Other Progress Improvement Systems.
Using a multimethod approach, the SIOD methodology contributed a “big picture” assessment that diagnosed specific problematic areas and helped the organization focus resources where most needed. For example, documenting the intense productivity pressures experienced by both physicians and staff in one clinical site revealed sources of internal strife and a blame-oriented culture. Through the SIOD process, our team helped the clinic’s management see the linkage between productivity pressure, environmental stress, and employee turnover. In turn, management was able to reflect on possible solutions, take our analysis forward to request additional resources, and plan strategies to build teamwork and collaboration. In all of the scenarios described here, SIOD filled in a substantial gap related to problem diagnosis, enabling other process improvement efforts to move forward with improved direction and prioritization.
REPLICATION OF THE SIOD PROCESS IN OTHER ORGANIZATIONS
Healthcare organizations spend millions of dollars implementing health IT systems, but without alignment to organizational requirements and integration into clinical workflow, these investments fail to deliver the intended benefits. In our case, the SIOD methodology connected academic biomedical informatics researchers embedded within the organization to organizational diagnostic needs. Such academic-operational integrations hold promise for future management projects that are evidence-based, data-driven, and integrated with health IT. Several key drivers of success were identified during application of SIOD to date, and are needed to transfer this approach to other organizations including:
Support from organizational leadership. Involvement of organizational leadership extended throughout the process, from identifying clinical sites in need of diagnostics to recommendations hand-off.
Inclusive approach to data collection. Identifying approaches to get everyone on the healthcare team involved in providing feedback was critical, given the holistic view of clinical functionality embedded in the SIOD process. Issues were not just related to a single role but to the full clinical ecosystem, including the health IT. Taking a holistic perspective was especially important for uncovering unintended consequences from previous interventions and for identifying knowledge helpful for preventing unintended consequences from future interventions. Other specific tools have been developed for assessing organizational readiness, such as the ORCA,66 ORIC,67 and ORC68 measures, quantitative measures from Implementation Science that are well-suited for their purpose. The inclusive mixed-methods approach to data collection and the holistic perspective of SIOD goes beyond readiness assessment to develop a deeper understanding of how organizational culture, people, processes, and technology interact, interactions that can lead to issues in overall clinic functionality and can impact the capacity of an organization to implement change.
Handing off recommendations to people with capacity and ability to take action. The recommendation hand-off portion of the reporting phase was especially important, as multiple clinical staff members discussed frustration with past process improvement efforts where they had provided feedback but no action was taken. Implementation of recommendations was beyond the scope of SIOD, which served primarily to diagnose issues and provide change recommendations, but we were able to hand those recommendations directly to people with the ability to take action. SIOD provided a comprehensive assessment that identified for leadership which areas of change might have the largest potential impact and the potential effort associated with them, helping them to prioritize next steps.
Advance planning to overcome barriers to rapid diagnosis. The pace of organizational change was rapid, requiring fast collection, analysis, and presentation of data. However, there are many barriers to rapid organizational assessments that individuals working in this area need to understand. Conducting assessments in an organizational setting required substantial coordination of activity and substantial time and effort from personnel. Proactively identifying assessment team members, providing training on data collection and analysis methods, and ensuring adequate resources for administrative aspects of SIOD implementation were all key components to overcoming barriers to applying SIOD to other clinical contexts. The speed of our internal applications of SIOD was often slower than the SIOD team and our organizational partners would have liked, due to a variety of barriers. Based on our experience, two key individuals are critical to rapid diagnosis: a point person on the SIOD team to coordinate the team’s efforts and a central contact at each clinical site being assessed to coordinate scheduling at the clinical site. In the case described in Box 1, the SIOD team added a point person, but lacked a central contact at the clinical site, resulting in some scheduling delays. Not all aspects of speed are within a team’s control, however, such as competing demands for team resources that delay project initiation and high-priority clinical projects that interrupt access to the clinical context. The strategy must be to identify needed team resources and to streamline all processes as much as possible to account for unanticipated sources of interruptions and delays.
An organizational team with contextual knowledge and relevant methodological training. Team composition varied throughout the SIOD applications, but one consistent factor was that team members had exposure to methods for organizational studies. Although leaders of the SIOD team had extensive experience in this area, other members included students with course experience and staff with complementary skills/training. The SIOD team was embedded within the organization, which was an important component for understanding historical and contextual factors impacting capacity for change. Although this contextual knowledge can be developed by groups outside of an organization, having an open-ended internal team within the organization to conduct studies at the intersection of technology and organizations and make recommendations assisted with ensuring recommendations and associated ratings were contextually appropriate. This strategy builds dynamic capacity by encompassing historical knowledge of how changes are affected and propagated within the organization, providing organizational learning needed to support the goals of a learning healthcare system.
Study limitations
The SIOD process was developed by a team with significant expertise in organizational research and studying interactions between health IT and clinical work. While our core team’s expertise has been beneficial during the development of SIOD, this raises questions of whether other organizations or groups newer to these concepts and methods or with different levels of organizational support can effectively apply the SIOD approach. A study of other teams applying SIOD outside of our organization would be the ideal evaluation of this concept. Until we are able to complete that evaluation, as a proxy, we have engaged graduate students in biomedical informatics in several of the SIOD applications, demonstrating that individuals newer to organizational studies in informatics can effectively apply these concepts and methods. Broader educational components, such as workshops at AMIA conferences or an AMIA 10 × 10 course, might be a helpful additional approach and are worth pursuing to eventually lead to a cross-organizational evaluation of SIOD. Although our team has competed formative evaluation components throughout SIOD development and application, a full-scale summative evaluation of SIOD has not been completed to date. The complexity of research at the intersection of technology and organizations, including the lengthy timeframe of implementing some recommendations, makes the design of a summative evaluation challenging, but this is an important next step. Finally, although the SIOD team has always had the goal of rapid diagnosis and recommendation delivery, each application of SIOD has encountered barriers to delivering on this goal. As we continue moving forward with the SIOD project, we continue to work on approaches to speed up the process of problem identification and recommendation delivery.
The SIOD process itself has several important limitations. The ability to develop meaningful findings through SIOD requires in-depth access to clinical sites, willingness to share their experiences from staff and clinicians, and resources to collect and analyze data. Obtaining access can be a significant barrier, highlighting the need for building connections between teams interested in applying SIOD, clinical leadership, and partners at clinical sites. The SIOD process was designed to build trust between the team and people at the clinical site, but organizational culture and other organizational barriers might make people uncomfortable with sharing their experiences. Taking a multimethod approach can help address some of these potential issues, as can providing ways (eg, individual meetings) for people to share information that they do not feel comfortable sharing in front of others. Although SIOD does provide recommendations for next steps, SIOD is not intended to be an end point in process improvement and it is crucial to have plans in place to hand findings off to people who can continue moving forward to resolve issues. As a result of this, an indirect limitation of SIOD is that its eventual impact is dependent on organizational leaders accepting the results, which can be difficult when findings identify underlying organizational issues. The key drivers of success identified in the section on replication of the SIOD process in other organizations are critical to addressing these potential limitations.
CONCLUSION
Our team developed a systematic approach to diagnose organizational issues, including issues generated by the interaction between health IT and work practices. After applying the SIOD approach across multiple ambulatory clinical settings, we identified common patterns related to technology-work mismatches, need for data-driven decisions on contextual factors such as staffing and space allocation, and gaps between the goals of patient-centered care and the requirements of day-to-day work. SIOD can be adapted with specific focus areas depending on organizational needs. We continue to apply SIOD to new clinical environments and have established a toolset for use across organizations. Next steps include transfer of SIOD to other organizations and other teams and summative evaluation to complement the existing formative evaluation of the SIOD process.
FUNDING
Portions of this work were supported by the Evelyn Selby Stead Foundation.
AUTHOR CONTRIBUTIONS
KMU contributed to the design of the work, data collection, data analysis, interpretation of data, drafted the initial version of the manuscript, and led revision of the work. LLN, CVH, JB, AOS, JWH, TA, CS, and NLM contributed to the design of the work, data collection, data analysis, interpretation of data, and provided feedback and contributions to versions of the manuscript. All authors approved the manuscript and agree to be accountable for all aspects of the work in ensuring that questions related to the accuracy or integrity of any part of the work are appropriately investigated and resolved.
SUPPLEMENTARY MATERIAL
Supplementary material is available at Journal of the American Medical Informatics Association Open online.
ACKNOWLEDGEMENTS
The authors acknowledge biomedical informatics graduate students and postdoctoral scholars in Dr Lorenzi’s “Healthcare System and Informatics” course for their assistance in the early stages of development and application of this work. The authors thank the staff and providers in all of our clinical sites for their participation in this project. The authors appreciate feedback from William Stead and Kevin Johnson on early drafts of this manuscript. The authors also acknowledge Bryan Steitz for his contributions to data analysis for the case study described in the manuscript. The authors also acknowledge the peer reviewers who provided thoughtful and constructive feedback, leading to a substantially stronger manuscript.
CONFLICT OF INTEREST STATEMENT
None declared.
REFERENCES
Dedoose. https://www.dedoose.com Accessed September 9,